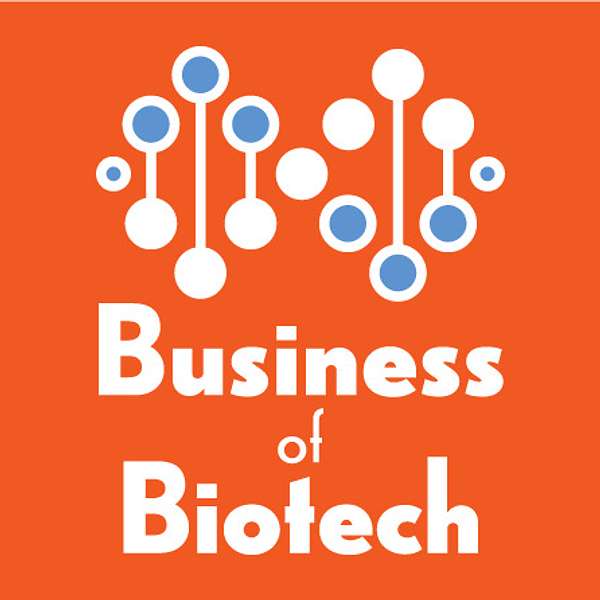
Business Of Biotech
The Business of Biotech is the pod dedicated to leaders of emerging biopharma firms. SUBSCRIBE to our new newsletter at www.bioprocessonline.com/bob. We bring you insight into organizational, finance and funding, HR, clinical, manufacturing, and regulatory challenges you’ll face as you navigate your company from an idea to success in the clinic. Each episode features guest commentary and best practices from accomplished founders and biopharma industry luminaries. The Business of Biotech is produced by Bioprocess Online and Life Science Connect and brought to you by Cytiva.
Business Of Biotech
A $1B AI Bet With Foresite Labs' Vik Bajaj, Ph.D.
We love to hear from our listeners. Send us a message.
This past spring, Xaira Therapeutics launched with $1 billion in backing, a who's-who mashup of Silicon Valley and biopharma stars led by Marc Tessier-Lavigne in the C-suite, and a mission to develop AI-generated drugs. On this week's episode of the Business of Biotech, we're digging into the company's origin story and its place on the greater techbio landscape with one of the men bearing responsibility for Xaira's launch. Vik Bajaj is Managing Director at Foresite Capital Management (which led Xaira's funding) and Cofounder and CEO at Foresite Labs (which helped incubate it, along with ARCH Venture Partners). He takes us behind the scenes, and shares his worldview on the role of advanced computing technology in drug development.
Access this and hundreds of episodes of the Business of Biotech videocast under the Business of Biotech tab at lifescienceleader.com.
Subscribe to our monthly Business of Biotech newsletter.
Get in touch with guest and topic suggestions: ben.comer@lifescienceleader.com
Find Ben Comer on LinkedIn: https://www.linkedin.com/in/bencomer/
There isn't much in the way of biotech that our guest on today's show has not done. Since earning his PhD in physical chemistry at MIT, he's hopscotched across several intersections of the high-tech life sciences and financial industries. No-transcript on the opportunities that present themselves when science, technology and money converge. I'm Matt Pillar. This is the Business of Biotech, and on today's episode we're digging into that convergence of tech, science and money with Dr Vic Bajaj, a guy who's got a hand in a startup called Xaira Therapeutics out of the Foresight Labs ecosystem that's got some serious backing. We're going to get to know Vic and his biotech worldview and, more specifically, we'll dig into the big bets he's placing on technologies like AI and ML, demonstrated by the mansion the billion-dollar mansion Xaira is building in a burgeoning industry full of cottages. Vic, welcome to the show.
Vik Bajaj:Oh, thank you, Matt. Very nice to meet you and I've been looking forward to this conversation, as have I. Yeah, Very nice to meet you and I've been looking forward to this conversation.
Matt Pillar:As have I. Yeah, and just for the listeners reference, before we started recording here, vic and I were bantering a little bit about political influence on biotech, which wasn't on the bingo card for today's discussion but may make its way to our discussion after hearing some of your thoughts and opinions. But where I want to start is in the here and now. As I said, I rattled off quite a list of experiences that you've had in the space from day one and currently you've got broad responsibility across three distinct, I guess, operating units or divisions or facets of foresight. So I want to kind of get a lay of the land for the intersection of your work at foresight, capital management, foresight labs and Zara Therapeutics. Am I pronouncing that correctly, by the way, zara? Yes, yes, yes, okay, I want to get a lay of the land of the intersection of those and sort of the chronology of your work in each of them launched jointly with my colleague and friend, bob Nelson at Arch, along with David Baker's group at the Institute for Protein Design.
Vik Bajaj:David Baker, as you know, was awarded the Nobel Prize for his work last month, and our model as investors is a little bit more operational than you would expect from an investment firm making sure that they're launched with the right operating infrastructure and contributing, where we can, some elements of scientific platforms and other things that we've spent years developing. But our model, then, is to essentially hire our replacements and make sure that we can move on quickly to the next thing while the company is in the hands of its permanent management, in this case under a brilliant CEO, mark Tessier-Levine, and a team that includes both some of the most accomplished AI developers and practitioners and some of the most accomplished drug developers in the world. So that is in very good hands. Um, and Bob and I, as representatives of Arch and Foresight, are are on the board. Um, but the company is uh now run by the stellar management team. Uh and uh we uh try to help them where we can.
Matt Pillar:Yeah, yeah, well, I, I'd like to get the uh get, get some. So, so, let's, let's go back to Foresight Labs then I'd like to get the get, get some. So, so let's, let's go back to Foresight Labs then, because I'd like to dig into some of the I guess machine at Foresight Labs that that produces companies like Zara. I'm wrong. As I understand it, foresight's sort of focus is on developing companies around these core technologies that you mentioned, technologies like AI and ML and you know, I mean I can make inferences looking at your LinkedIn profile.
Matt Pillar:As I said, google Life Sciences Verily like yeah, this guy's coming at it with a bit of a tech bio bend or bias, but tell us why, rationalize that for us, because I tried to make a play on words in the intro around, you know, building a mansion in an industry full of cottage, right, a little play on the cottage industry thing. But we see this cottage industry right now of small players, just a highly fractionalized industry of super small players that are not nearly as well-funded as Zara. Industry of super small players that are not nearly as well-funded as Zara. Coming at molecular discovery and design from an AI angle, you might even look at it and go. You know, nascent, borderline, saturated, are we looking at like where's the value? So I'm curious about your take on that and what differentiates the approach I mean, besides dollars, what differentiates the approach that you guys are taking around building companies, around this foundation of AI and ML? Long-winded question, I know.
Vik Bajaj:No, but a good one. First of all, we're broadly focused on things that actually accelerate product development. I don't have to tell or remind your audience, but it's worth remembering that our industry is very peculiar. We have a 10-year product development cycle that is characterized by late stage failures you know it's not for the faint of heart by late stage failures, you know it's not for the faint of heart and part of that is really governed by our resort to empiricism at essentially every stage of the process, and there have been exceptions to that. The transformation of oncology from an entirely empirical discipline where broadly cytotoxic therapies were tested in broad patient populations, to a completely different practice of precision oncology where we understand the molecular and genetic drivers of cancer, we produce drugs that are precisely targeted to those drivers and we both test and eventually deploy them in patients whose tumors actually harbor those drivers. That's an example of a transformation of the industry from something that is very empirical and artisanal to something that is not quite but increasingly engineering-like, in the sense that there are predictable modular elements and predictable outcomes when those modular elements are employed right. So what we're broadly interested in is how can you accelerate drug discovery, drug development and even healthcare delivery. We can come back to that. That is the whole infrastructure that consumes 20% of US GDP and increasing with miraculous outcomes, but still nowhere near good enough. So breaking that down, part of it is the long process, sometimes decades long, of understanding disease biology, understanding what targets cause disease rather than just are associated with disease. Those are the best drug targets. The second part of the process is actually making molecules, of whatever kind, that engage those targets in ways that reverse their malfunctions or induce a function that counteracts the disease process. Then, given that drug against a target that we believe to be real and causal in disease, you have to test the drug in clinical trials and you want to do that in a patient population that's as small as possible, so the trial is as fast as possible and where the success rate is high. And then you'd like to deploy that in a healthcare system, ideally, that's tuned to the needs of the individual patient.
Vik Bajaj:In oncology we now have, at least scientifically, the ability to manage the entire patient journey. We can measure who is at risk for cancer. We can target, as we did at Grail, people at highest risk for screening. That is increasingly sophisticated. Once we find the cancer, we can measure other properties of the cancer to target therapies to it. We can watch those therapies succeed or not, and then we can even watch for recurrence using increasingly sophisticated assays. So, again turning to oncology, how can we accelerate that product development cycle and how can we do something similar for all disease? That's the question we're asking. In an ideal case that would be precise, tailored to the needs of the individual, and it would also allow us to intervene in disease early, maybe ideally preventing it before the harm is done, or at least preventing the most deleterious consequences.
Vik Bajaj:So in that framework, we are convinced I'm convinced, have been for a long time that the methods of modern large-scale computation at first, and now machine learning in the last five years or more, are maturing to the point where they promise to do for our product development cycle what they've done in the technology industry and the finance industry and other parts of industry that have been thoroughly transformed. So that's what we've been really engaged with. And the question is to understand when are those tools mature? When are the data sets even? When do they exist? When are they mature enough that those tools can be built? And then, finally, how do you arrange that toolkit in a company that's actually capable of doing product development. Otherwise you have to wait five, six, seven, eight years to understand if your toolkit is even working.
Vik Bajaj:So those themes are ones that we exercised in detail at Zara because the idea was to create a vertically integrated biopharma company whose mission from the beginning is to use this new and emerging toolkit to accelerate product development.
Vik Bajaj:And the pillars of Xera, the people in Xera, the funding structure reflects all of that. We brought into Xera at inception efforts that, like David Baker's, that have a 10-year legacy of validation, or the Illumina intramural programs in data generation using perturbative experiments, functional genomics, that were also very advanced. And we did that so that these tools and technologies, as much as they'll contribute to a future pipeline of machine learning models, they're mature and ready to contribute to product development today, learning models that are mature and ready to contribute to product development today. And so we also brought into Zara really seasoned product developers like Debbie Law from BMS, who truly understand how to harness this infrastructure and turn it into drug programs that benefit patients. So that was the model and kind of set of themes that led to the model and and you know, kind of set of themes that led to the model.
Matt Pillar:What's the, what's the stage of, of, of, I don't know maturation that we're at in terms of you know people like her, who, who, who understand the ecosystem, the tools and have the capability to bring it all together. And I asked that question, uh, because I get the sense, you know, when you put your ear to the ground and listen to you know uh, groups of of of, uh scientists and biotech on in in forums. For instance, you go go on the biotech forum on Reddit, the subreddit, the biotech subreddit. You ever, you ever do that.
Vik Bajaj:The biotech subreddit. You ever do that.
Matt Pillar:No, I try to avoid that. But I'm guessing that you're about to tell me why I try to avoid it. But yes, what some ground level chatter around AI and machine learning and biotech. There remains a lot of skepticism and much of that is surprisingly maybe not surprisingly around a younger cohort who maybe haven't been around the block in industry as much. It gives me the sense that industry is driving much of this innovation, as opposed to academia. I'm wondering if academia is still kind of stovepiped in its approach to professing, teaching and preparing tech bio workers. You tell me, what does that landscape look like? What's the funnel look like for companies like Zara and other companies that Foresight Labs is going to churn out? They rely on people who understand these intersections.
Vik Bajaj:Well, there's a lot to unpack in the question yeah, I ask these long-winded multi-point questions.
Vik Bajaj:No, but let's take it one at a time. Right One is you don't have the freedom to construct an organization from a hypothetical training pipeline that takes 20 years to create. Right. And one thing I learned at Google in the life sciences program there, which we should come back to because it's like an AT&T bell labs environment that I feel really laid the foundations for a lot of what you're seeing in the space now is how difficult it is to get different kinds of people to work together, and why. What are the problems you have to solve to do that.
Vik Bajaj:So I remember at Google at one point after we had launched a first clinical study and there were a couple of hundred people on the team, a mixture of wet lab scientists, software engineers and other kinds of engineers and clinicians. I surveyed the team and I asked them this question when do you think the first result of this study will impact clinical care of patients? And of course, the clinician said well, this is an observational study, we'll learn something from here. It's going to lead to discoveries those have to be tested. That's a decade, two decade long cycle before there's any impact. And scientists are more optimistic. They're thinking about biomarkers, molecular factors, things that could impact product development. They thought maybe in five years we'd have some novel endpoints for a clinical trial or biomarkers from these observational studies. Software engineers thought that this would happen in a matter of months, that we would derive insights from a observational clinical study that were going to be transformative in healthcare. And you know, what that reflects are the different product development cycles of each of those disciplines, their culture, their instruction, their training. We talk about bilingualism and all of these things. That's fine, that's important, but most important is to understand that in biotech and healthcare we literally have a decade long product development cycle, and for software engineers that cycle is measured in weeks and months. So getting those people to work together requires bridging those worlds, understanding the best of not sacrificing scientific rigor but also not changing the way that software engineers work, in fact importing that process much more into the practice of science.
Vik Bajaj:So now the second question you asked was about the training environment, or how people are trained. That's changed a lot too right, when I was in grad school, in the quantitative disciplines people had software engineering experience, but it wasn't really in the quantitative physical science disciplines, but it wasn't really rigorous in an engineering sense unless, like you know, a subset of us. You had worked outside of science in the software world and been exposed to that in some way. Right, that was normal. Today, that's not normal at all In people in biological AI, people in fields of statistics, biology, computer science that are touching machine learning.
Vik Bajaj:Actually, this generation of scientists has a a understanding of all of the tools that industry uses for software engineering and software development. They had the mission to release tools that other people can use. They understand the open source community. They understand to some extent and they're receptive to rigorous software engineering practice release cycles, release calendars, requirements-based development, testing-based development. So that's changed and it's probably taken 15 years for that to change. So I think the workforce that is coming in now today is way more informed in the disciplines that we need and that actually, to your third point, reflects the practice of science in academia.
Vik Bajaj:It's no accident this year that two Nobel Prizes in two different disciplines went to AI and ML.
Vik Bajaj:I mean, when I came to Google a long time ago, I was giving an all hands meeting and I mentioned to all of the Google employees that you know the best scientists. They are attracted to environments where they have new tools to accomplish their science, because, as scientists, it's not just what we find, but even the very questions we ask. The exploration you can conduct is circumscribed by the tools that you have available, at least as experimentalists, to understand the world around you, and it was clear then that the tools that Google had developed for completely different purposes were going to be the best scientific tools of my generation of scientists, and that's why we all went there. That's played itself out in academia. So there is no part of academia today which doesn't use that toolkit to accomplish science, and we could go into 20 examples of that. But basically, academic science is aligned with the kind of themes that we were discussing before. The training environment reflects that, so there's a robust pipeline of people who understand that that are coming into companies that we've started, like Sarah.
Matt Pillar:Yeah, I get the sense that in the time that you've spent since earning that physical chemistry degree time spent at earning that physical chemistry degree, time spent at Google and elsewhere you now lean decidedly into the software engineer mentality camp, where five months ought to be a Maybe. Five months is aggressive. But you get my point. If you had to pick, where does Vic Bajaj live right now in terms of you know, reality, product life cycle development, uh. Product development timeframes, uh. Versus where we want to be.
Vik Bajaj:So, um, this goes to I think something you mentioned before was implicit in your last question, right which is like when is any of this stuff actually going to accelerate product development? Think about it now. We have to hold ourselves to the utmost standard of evidence. Let me give you an example of what we did at Grail, right, grail is running now one of the largest clinical trials ever attempted I think, probably the largest outside of vaccine trials, right? Why does the trial have to be that large?
Vik Bajaj:Well, the goal of our product at Grail was to develop a blood test that's capable of screening for many, many different cancers, test that's capable of screening for many, many different cancers. That means that you need to show that you can detect all those cancers. And also, it would be unconscionable to tell the patient that, well, you have cancer somewhere in your body. We don't know where it is Now you have to do some kind of workup to find it. That would be very unconscionable. So it also has to be exquisitely precise about the site of origin unconscionable. So it also has to be exquisitely precise about the site of origin. Now, if you want to do that for 20 different cancers, you are faced with two facts. One is that every cancer is slightly different and you have to study all of them because you have to be able to tell them apart. So it is not one disease but dozens of diseases that are related but different. So that immediately means that you need a much larger patient population because you have to have a representative number of all of these cancers.
Vik Bajaj:Second, we want to see to prove that the product works. Healthy people get cancer that the test can detect earlier. Now cancer kills an enormous number of people. It's a sad fact, but the reality is the chances of any healthy person getting cancer in a year are exceedingly low. So that means now you have to enroll even more people because you have to have statistical power. You have to anticipate that you will see this number of healthy people get cancer and 20 different cancers in a reasonable clinical trial timeframe, which in this case is three years.
Vik Bajaj:So those things that I've all recited are scientific facts about statistics. They're scientific facts about human biology that it's very unlikely that machine learning can impact or change, and we can't pretend that this toolkit will absolve us of the responsibility to generate the most reliable evidence for interventions that are incredibly expensive economically. We're always going to have to generate that evidence. So one question that we're asking is how can you systematically accelerate the generation of medical evidence? There's many ways to do that. There's many ways that machine learning can be applied to do that. There's also many ways that just business model innovation can be applied to do that as well, and that's equally important. But you can never shrink that. I mean never, say never. But with no foreseeable data set, no foreseeable technology, could you shrink that to a five month process and be sure that it's safe and effective, which is ultimately the responsibility that you know we're held to?
Matt Pillar:Yeah, it's. Uh, that may be a an interesting foray to uh, to touch on some of the discussion we were having before we hit the record button uh, around political influence, because I'm curious, as you describe this, um, you know some of these, I guess, um facets or factors that play into the development time when you're running a biotech business. I'm thinking about the fact that we're in a, you know we're at an inflection point, at least seemingly we're at an inflection point looking at the next four years around the way that agencies like the FDA conduct business, like there's. You know, based on some of the appointments we've seen in the last few days, there's a likelihood that at least there's going to be a strong influence for change.
Matt Pillar:Can one, do you see that as an opportunity? Do you see that as an opportunity for you know HHS and FDA and CDC, to kind of take a look around and say what can we be doing differently to enable at least work in lockstep with the efficiencies that we see coming out of industry? And so another two-part question One, do you see an opportunity there? And two, do you have reason to hope or speculate that those agencies, FDA in particular, and it's the role it plays in drug development. Do you have reason to believe that they can enable some of these embrace, even some of these efficiencies? Because this is revolutionary technology. We see it play out over the next several years. It could have very real implications on how fast you expect a company that you're investing in, like Zara, to move through the clinic.
Vik Bajaj:So I mean I'll try to lay out the positive potential and then maybe talk about some things that would impede that right. So one is that, as much as we complain about the regulator at times, what the regulator does, especially in the United States, is largely focused on what it's supposed to focus on safety and efficacy. And the arguments that the regulator invokes are not unlike the arguments that I was reciting in relation to Grail's clinical trial size. Right, there's a quantum of evidence that's needed to prove a claim and that quantum of evidence is not totally independent of how you generate the evidence. But you have to satisfy that right and that's what the regulator does. And for the most part, if you have innovations take cancer, biomarkers, basket trials in cancer. We could recite a lot of examples there's a path to convince the regulator that what you're doing is reasonable, that it represents a well-defined patient population, that you are causing much more good than harm by doing whatever it is that you're doing that's innovative. You know the regulator doesn't care if we use machine learning to automate the drudgery of preparing all the materials that go into clinical trials. In fact, those things reduce errors and there's a path to include them. That's relatively straightforward If we have novel clinical trial endpoints, time and time again there are paths to include them that are appropriately difficult, but they're not opaque, they're not mystical.
Vik Bajaj:That said, there's also areas where the regulator can improve, can accelerate, and there we should take comfort that there's been, you know, bipartisan set of individuals who are committed to that principles all of them, you know. I'll name two that I work with personally. One is Rob Califf, who is a dear friend and was a mentor to me when he was at Google. He is extremely committed to the idea of accelerating the generation of clinical evidence without sacrificing rigor in every way possible. There's probably no human on the planet who's run more large clinical trials than Commissioner Califf has and Rob has, but he's very committed to innovation at every step of the process. And the other one, scott Gottlieb, different party. He's someone who we work with at Zara. He is equally committed to innovation, creating paths for these new tools and technologies to come forward, actually creating business incentives to work with the regulator in unique ways.
Vik Bajaj:So I'm kind of comforted that there's this whole bipartisan infrastructure set of individuals that start with the basic scientific premise that we have to prove these things are safe. We have to prove they're effective. And then let's pull every lever, let's consider any innovative path to demonstrate those two things more and more quickly, to just accelerate how much we learn the difference overall between people from different parties who've held that role, because they're all committed to the same things. Now what happens if that premise is challenged, that it's no longer science-based? There are some other principles that are being served, that are mixed up in the regulatory process. That's what I worry about, because the outcome there is not something that I could predict and it's not clear that then people would be following the same incentives and rules and in that framework, it's not clear how the professional staff at the FDA would even react to different kinds of incentives and pressures staff at the FDA would even react to different kinds of incentives and pressures so that I'm worried about because it's so unpredictable.
Vik Bajaj:But what is predictable is, if you start from that patient-centered, science-based premise, that people of radically different political persuasions all find ways consistent with their politics and their worldviews to make the process more efficient.
Matt Pillar:Yeah, Uh, so many, so many should have such a uh uh, uh a row. Now I don't want to say Rosie, but a bipartisan and tempered approach.
Vik Bajaj:Well, that's what we've had. Uh, until well, we don't know what we have today. Yeah, we don't know what we had, for sure until today.
Matt Pillar:I don't know what we have, don't know what we're getting. Uh, we'll know that tomorrow. Uh, we'll know that the next day. Getting back to Foresight Labs and Foresight Capital Management, you know we've had this discussion around the importance of the sort of tech core of companies that you're interested in and funding and Zara, what else? What other criteria do you apply? And you can answer this from either perspective, you know, as a capital management company, or the Foresight Labs perspective what other criteria do you apply to the determination of companies, modalities, technologies that you're interested in investing in? Is it modality-specific? Is it indication-specific? Are there geographic sort of guidelines? Just give us some sense for the MO there in terms of your investment criteria.
Vik Bajaj:So it depends on the kind of company and the criteria for company creation and investing in companies.
Vik Bajaj:They overlap, but the emphasis is different.
Vik Bajaj:So one thing I think that distinguishes our practice at Foresight Capital and it's something that the founder of our firm and my colleague, jim Tannenbaum, has emphasized from the beginning, long before I was here is measurable product quality, and so, for example, we have a very robust therapeutics team led by Michael Rome and Dorothy Margulski, and they are among the world's experts at evaluating the quality of a product as it's nearing a clinical experiment, predicting how will it work, seeing all of the dimensions, from the molecular to the clinical to even the commercial, connecting those to understand is this a product which will fundamentally benefit patients and are there any barriers to its adoption?
Vik Bajaj:So that product orientation which is in the firm since its founding, infuses even some of the more long range speculative things that we create and we do, including big idea companies like Zara, because in the end, there's this foundation of accountability to the patient, which is driven by knowing exactly what criteria you have to satisfy for a drug to be good. So we've never lost touch with that, and that definitely animates everything that we do, even in the big ideas that you wouldn't think are grounded in that kind of product logic, but increasingly they are.
Matt Pillar:Yeah, regarding the point I made earlier about the cottage industry of companies that are coming, biotech companies that are coming up with an AI ML bend, or AI ML companies who are attempting to break into as even as a service right, like service providers who want to serve biotech companies that are doing molecular discovery, target identification design, how do you see that shaking out? It's a big, broad question and maybe it's early to say, but you know and I ask this question like fully aware of the juxtaposition of a billion dollar investment in a company like Zara. Right, you've got Zara, you've got the recursions of the world. You've got these big giants right now, big players in the space, but then you've got this sea of small players who are trying to get a piece of the pie. What do you see happening there of small?
Vik Bajaj:players who are trying to get a piece of the pie. What do you see happening there? So it's tough to say, but we could again discuss some general principles and make some guesses. So one thing that Chris Gibson and of course, now Najat Khan is there as well One thing that all of us agree on is the need for companies like this to generate data in their domains. Daphne, kohler would also agree with that right. These are almost founding principles behind those three companies that I would highlight as really principled in their approaches Recursion in CITRO and now ZERA.
Vik Bajaj:And I know that that sounds either silly or obvious, depending on your perspective, but actually there is a misunderstanding about the amount of biological data available and the importance of such data in training models to answer questions that are actually relevant for product development. And let's look at some examples In the technology world. The reason that models of larger and larger scale can be trained is because there is an amount of data available that continues to grow. It may be plateauing now, but it has continued to grow in the past, and that's because, as consumers using devices like this, whether we want to or not, we generate data which is then harnessed for the next generation of models, Whether those are new model architectures or they're just larger models using existing architectures that are working. There's actually no emergent or discontinuous behavior there. Their properties and their internal training metrics are essentially continuous properties of the amount of data and the size of the model.
Vik Bajaj:In biology we do not have data of that scale In the places that we do. Take the PDB, the protein databank. This is a database that has atomistic accuracy, right, which is really unusual and it's been curated by four or five generations of scientists literally since the mid 1950s. So that's a very unusual dataset in its accuracy, breadth, historical completeness. There's very, very few data sets like that. So one of the reasons that we're just scratching the surface in biological machine learning and things like, on the other hand, solving the protein structure problem or creating approaches to design proteins there's a gulf between those two is the quality of data available. So our thesis, and many others, is that you actually have to create those data. The world is not creating them. Pharma companies are not creating them, maybe with the exception of Aviv Ragev and her collaboration with Recursion. Nobody's creating those data at a scale that's needed to train biological models that would actually answer useful questions.
Vik Bajaj:So what's an example of a useful question, just to be really concrete about this. So you know, in human genetics we use to establish causal relationships between targets of drugs and disease, and we can do that because genetics are kind of like a randomized control trial or the closest thing that we have to that, in that genetic variation is is random between two individuals. So you can look at many, many millions of people, ideally, and you can see what these random experiments of nature are doing to their disease risk or to biomarkers in their body that are associated with disease. And because that's kind of random, like a randomized controlled trials, it allows you to make statements about causation, causality rather than just association. So it's incredibly powerful. But evolution selects against deleterious things and so it's also quite limited. Even in the limit where we have now, you know, a couple million people's individuals data available, one form of genetics or the other, we're still highly data limited. So one question is how can we discover new targets without observing them in human beings? And that requires that we ideally do a lot of experiments where we introduce perturbations, experimental changes, pings into cells, into animals, into model systems and put them all together. These are things that we can't observe in nature, they're too rare and understand. How does that relate to the human experience of disease? So that's kind of a grand challenge of machine learning for which the data are so limited that it's only been possible to solve in narrow corner cases.
Vik Bajaj:One of the things that Zara is doing is creating more data of that kind in a single quarter than the whole world has created so far, and we think that experiments of that scale are necessary. The world isn't going to produce the equivalent of the protein data bank on its own in the short term. So what does that mean? It means that these companies that have SaaS type business models or business models where they're not generating data but they're working on models they have to stay ahead of all of the model generation activity in academia, which is very hard. There's a lot of creativity there and they have to somehow access these data sets.
Vik Bajaj:If the data sets come into existence, they come into the public domain or they'll be able to access it and they can stay ahead of the academic community, then perhaps they could generate a lot of value. Actually, if they don't, then it will be companies like Zara, who are trying to generate data and harness the value of actually developing products that succeed, and my prediction is in the steady state. All kinds of companies will exist because there'll be a lot more public data. All kinds of companies will exist because there'll be a lot more public data. There'll be many, many models and more people who are able to really generate specialized model architectures for particular problems. And there'll be many companies like Xero which are harnessing all of this infrastructure for product development.
Vik Bajaj:In the short term, our bet is that the data generators are most likely to succeed, because the data simply don't exist. I'd point to even in the technology LLM space, there are companies that are worth many billions of dollars whose sole purpose is to organize the data that's freely available on the Internet and make it available to those companies that are training large models. So even there, it's not as easy as I was suggesting. In biology, it's exceedingly hard. In clinical medicine it's even harder. So that's why we think data generation is a prerequisite for all of this to be useful.
Matt Pillar:Yeah, give us a sense for the execution of data generation. Give us an idea how that happens.
Vik Bajaj:Well, I mean there's generally two or three models. So one is this PDB type model where there are individual investigators or large institutions that are generating data and they pool it in databases for the good of the public. You've seen many projects like that in oncology, for example. You've seen many well the Cancer Genome Atlas, the DepMap project, the CCLE, before that, all at the Broad. In single cell biology there's been similar projects to create a single cell atlas. That's a project that Aviv Raghav and Sarah Teichman started and ran. Czi has made major contributions to that. So that's one model where consortium of academics over a decade generate a lot of data, or over many decades, and they pool it it so.
Vik Bajaj:Another model is the model of nation states producing large cohort data, and that's happened in the UK. I'm on the board of Genomics England, for example, and the UK remains, believe it or not, the world's largest data generating entity. Most of the useful biomolecular data that we use comes from the UK, not from the United States, because there's a rich tradition of that and variants of that include companies like Regeneron or Amgen, which have sponsored very large projects to generate those data, over which for a short time they may have exclusivity, but eventually it ends up in the public domain. And then there's a third and emerging category of companies that are generating their own data and meaningfully sized data sets. That's happened in genetics, 23andme Ancestry, decode, genetics, kari Stefansson's effort in Iceland. There's very few examples of that. There's even fewer examples of companies that have generated large-scale laboratory data to train machine learning models. There are a few, including In-Citro Recursion and Genentech now, and Zara. So that's generally three ways that data get generated. I think the last one is going to be very important in the next five years.
Matt Pillar:Yeah, yeah, all right, we're going to run short on time, so I got a couple more for you.
Vik Bajaj:Sure.
Matt Pillar:I want to get back to that. Billion. Billion dollars is a lot of money, Vic. It's a lot of money. What's the plan for that? What is that funding and how many more Zaras can Foresight Labs put out?
Vik Bajaj:How many more Zaras can Foresight Labs put out? Well, there's two or three separate questions there. One is what is the right amount of money for an idea like this? And the answer is that you need a quantum of money that actually allows you to test the hypothesis. Of course, the hypothesis has to be compelling enough that a whole community of people will join with you in sharing the risk. That's what we've achieved at Zara. It's a very big idea. There's a lot of optionality. There's a lot of ways in which it could be successful, many more than there are ways in which it won't succeed. So that's how you assemble something of that magnitude. It's that the idea merits it.
Vik Bajaj:Again, going back to Grail, another example with Bob Nelson, where I was the chief scientific officer. Bob was the founding investor. You can't test that idea with a small amount of money. If you were just sitting drawing on a napkin the idea of detecting cancers early, you would conclude within a short amount of time that you need a very gigantic clinical trial and that costs what it costs. So some ideas the impact on healthcare are so great that they're worth testing.
Vik Bajaj:And then the goal is to generate optionality so that those very large scale experiments where you need that amount of money, that they're not binary, they don't succeed or fail, but rather there's optionality, there's early success, there's tactics to make sure that the risk is managed and mitigated, but you cannot get around the amount of money it takes to accomplish transformational things, and when you do, you actually introduce a risk which is greater than the sum of all of the scientific and execution and engineering risks combined, and that's simply that you're not resilient to the natural failures in any discovery process. Those are not failures. Those are things that abort unproductive paths and allow you to focus on paths that can work. If you run out of money before you learn all of that, the consequences are far worse. So I think the argument for funding these big ideas in that way it's an argument that you know, bob and others have always advanced for decades now is absolutely correct. And yes, and I didn't mean any.
Matt Pillar:I didn't mean to be too speculative. I spend so much time, you know, in the biotech world where you know a billion dollar acquisition of a 10-year-old, well-developed, phase two clinical product is big news. So to come out of the gates with that kind of money, I'm just a little bit, you know, starstruck by it. That's all.
Vik Bajaj:No, I understand, but the reality is that big ideas need that kind of support.
Matt Pillar:Yeah.
Vik Bajaj:And our job is to make sure, first of all, that we're excellent stewards of that capital, so it is not a proxy for making sharp and sometimes painful decisions, and also that we preserve a lot of optionality. So there's many paths to success, and that's a hallmark of these big ideas. And then you asked are there more like that coming? Yes, there are more like that coming. One that we are really interested in now is understanding how our deep scientific understanding of disease can actually impact healthcare delivery the gulf between what we can accomplish in the laboratory, what we can understand about an individual and an individual's experience of disease and risk for disease, and what's in clinical practice that is growing and growing and growing every year. All we know about science and apply it to maximum benefit, where right now there are enormous barriers to accomplishing that very few of them scientific. So that's an example of you know things to come.
Matt Pillar:That's a tantalizing hint you dropped out there. I'm trying to put pieces together, but I don't want to get ahead of myself. I just had this conversation, the episode that were dropped prior to this one. The drop the listeners heard last week was a conversation with Alan Shaw, where we talk a lot about that inefficiency, the fact that the United States of America in particular I mean I know you're looking at things on more of a global scale as well States of America in particular, I mean I know you're looking at things on more of a global scale as well, but you know we've got a $4 trillion healthcare problem and we don't perform very well in terms of outcomes. So am I picking up on the hint that perhaps this what you just described might be an element of a solution to that challenge?
Vik Bajaj:Yes, that's our hope, that we'd understand how to harness all of the science and technology to produce a kind of radical personalization of the healthcare product to the needs of the individual. Yeah, you know, and the science is there to do that.
Matt Pillar:Yeah, all right, we need to wrap things up here. Vic, I feel like I could talk with you all afternoon. I've got so many more questions for you, but let's just end on this. Can you offer our listeners, many of whom are new biotech leaders, new biotech builders, early stage companies, your best advice on approaching the marriage of high tech and bio in their endeavors?
Vik Bajaj:that the marriage of those two worlds. Particular manifestation of that, there's a way to measure whether or not a product development process is being accelerated or some unique insight is being generated. It's a way to measure that, so that if you don't have that, if you don't have the crucible of product development within a company, then it's not just that you don't know whether you're succeeding or not, but actually the questions that you begin to ask are not related to what's needed in the end. In the end, we want to produce better drugs that alleviate the suffering of disease and eventually cure disease, and a measure of that has to be built in at every stage of the process. If it's not, um, the process can be easily misdirected.
Matt Pillar:Yeah, sound advice, vic. Thank you for joining me. As I said, I mean, I feel like we just scratched the surface here. I think what we're going to have to do is, uh, invite you back and maybe do a multi-part series, because I feel like you've got a lot to offer our community and I appreciate what you've offered today.
Vik Bajaj:That's very kind of you and I'd. Interim President Dr Vic Bajaj.
Matt Pillar:I'm Matt Pillar and you just listened to the Business of Biotech. We're produced by Life Science Connect and its community of learning, solving and sourcing resources for all manner of life sciences professionals and sourcing resources for all manner of life sciences professionals. I invite you to subscribe to the Business of Biotype podcast anywhere you listen. Check out our videocast page under the Listen and Watch tab at bioprocessonlinecom. Leave us feedback and a review, and be sure to subscribe to our monthly newsletter at bioprocessonlinecom. Backslash B-O-B In the meantime. Thanks for listening.