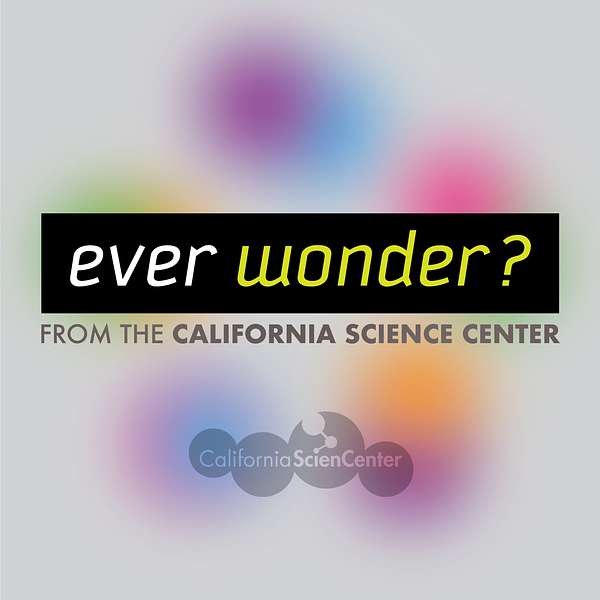
Ever Wonder? from the California Science Center
Ever Wonder? from the California Science Center
REBROADCAST:......if robots can be biased? (with Ayanna Howard)
The field of robotics has advanced a LOT over the past couple of decades, and part of that has to do with advances in the fields of computer science and artificial intelligence. Algorithms that help robots function and interact with the world are all around us, from the search engines we use to the facial recognition function in our phones.
But these algorithms can have problems. This past September, for example, Twitter users discovered that photo previews, which use machine learning to crop photos to the most interesting part, appeared to favor white faces over Black faces.
We know that humans aren't perfect, but… Do you ever wonder if robots can be biased?
Ayanna Howard (@robotsmarts) is a roboticist, professor, director of the HumAnS Lab, and chair of the School of Interactive Computing at Georgia Tech. She is a leader in the field and has many accomplishments, but one area of her work that caught our eye is her research on how algorithms and robots can be biased.
Have a question you've been wondering about? Send an email to everwonder@californiasciencecenter.org to tell us what you'd like to hear in future episodes.
Follow us on Twitter (@casciencecenter), Instagram (@californiasciencecenter), and Facebook (@californiasciencecenter).
Perry Roth-Johnson: 0:06
Hello! This is Ever Wonder? from the California Science Center. I'm Perry Roth-Johnson. So if you've been listening to the past couple episodes on robots, you've heard about a few kinds of robots that might challenge your view of what"counts" as a robot. The field of robotics has changed a LOT over the past couple of decades, and part of that has to do with advances in the fields of computer science and artificial intelligence. Algorithms that help robots function and interact with the world are all around us, from the search engines we use to the facial recognition function in our phones. But these algorithms can have problems. This past September, for example, Twitter users discovered that photo previews, which use machine learning to crop photos to the most interesting part, appeared to favor white faces over Black faces. We know that humans aren't perfect, but... Do you ever wonder if robots can be biased? To find out more, I talked to Ayanna Howard, a roboticist, professor, and chair of the School of Interactive Computing at Georgia Tech. She is a leader in the field and has many accomplishments, but one area of her work that caught our eye is her research on how algorithms and robots can be biased. Let's get into it. All right, Professor Ayanna Howard, you are a roboticist and professor at Georgia Tech, where you are the chair of the School of Interactive Computing. Welcome to the show!
Ayanna Howard: 1:32
Thank you. I'm excited about this conversation!
Perry Roth-Johnson: 1:36
I am too! We're really, really pleased to have you with us. Maybe we'll start on a fun note. What's your favorite robot?
Ayanna Howard: 1:43
Rosie, of course!
Perry Roth-Johnson: 1:44
Rosie from The Jetsons?
Ayanna Howard: 1:46
Rosie from The Jetsons. And I'll explain why. Um, so Rosie epitomizes what I think about robotics. Um, something, a machine that interacts with us that understands us, uh, that is almost essential to enhancing our quality of life. But at the end of the day, uh, if, if anyone remembers Rosie, if you did something wrong, she will tell you off. Right? And so I think, you know, she, she made the family a better family.
Perry Roth-Johnson: 2:15
Does a robot need to be something that has mechanical components like a Rosie, a Mars rover, or the robot dogs made by Boston Dynamics that are in all those viral videos? Or, are voice assistants like Alexa, Siri or Google Assistant robots too?
Ayanna Howard: 2:30
Yeah. So the definition of robot has evolved and I would claim that an intelligent agent, intelligent voice assistant like Siri, um, is a robot. And I'll explain why. So if you think about what a robot needs to do, uh, a robot has to be able to sense the environment. Uh, Siri understands voices. So that's an aspect of sensing the environment. They have to then have a brain to process this information, uh, which voice assistants do. They take your voice, and they look at, oh, what is it that you want me to do? But what's key and why I would say a virtual agent is also a robot, is that they have to do a, what I would call a physical action, something that allows us to interact. So playing music? Think about it. It's, it's replacing a physical action. So typically we go to the radio, we turn the station. Just because they do it in a virtual format versus a physical format. The output is still the same. You have to figure out the volume, you have to figure out the station, it's sensing the environment processing based on that environment and then creating a function that impacts the physical world in some way or form or fashion.
Perry Roth-Johnson: 3:42
Mmhmm. Would you say, uh, that was true many years ago--like, I don't know, 10, 15 years ago--or has our notion of robotics changed over time?
Ayanna Howard: 3:51
Our notion of robotics has changed. So when I started in this field, uh, more than 15 years ago, um, there was roboticists, which I was. And then there was this whole field of artificial intelligence. And as a roboticist, I used AI. So I used neural networks and computer vision, but I never called myself an AI person. Um, and it was because back then, uh, roboticists and the things that we could do, um, were really about the physical components and the hardware and, you know, maybe getting AI to work and it didn't work almost ever. Whereas, whereas AI was really, um, the computation wasn't necessarily there. So I would say that the kinds of tasks that they were attacking were readily about fundamental knowledge and that's evolved because computation has gotten much better, which means that you can do all of those fundamental things that you used to just dream about. And you can do them with robotics as, as the component or the entity. And so the field has those two robotics and AI have blended together quite a lot.
Perry Roth-Johnson: 4:56
So it sounds like the hardware and the software really become deeply integrated. And those fields of study are deeply intersecting right now.
Ayanna Howard: 5:04
They're deeply integrated, they're deeply intersecting. And even the concept of hardware, right? It's it's this aspect of a self-driving car. Is it really a car or is it just a AI system that happens to have wheels? Right? And so it's this, it's this blend. Yes.
Perry Roth-Johnson: 5:24
In your view, how do robots have the potential to help people, um, or maybe even harm people if they're not designed properly?
Ayanna Howard: 5:33
Yeah. So I'll, I'll take the first part of that, which is help. And I usually link it to quality of life. Um, so these are the things that we are accustomed to that we think are almost our rights. So a lot of us believe that every child should be educated. Uh, we, we don't really think about the fact that, well, maybe there are some environments that don't have teachers or don't have access to the internet to log on to courses online. Um, and so what robotics does is it fills those roles where, um, you might have a person there if you had that access and resource. And robots provide it, provides that access and, and levels the playing field. And so this is in education, it's in healthcare, um, and it's in access to transportation and, and these functions of life, um, are the helping part. Now the harm is that if our systems aren't created with thinking about the unique natures of people, it also means that there could be a potential of harm. And I'll give an example of healthcare. Women, as an example, exhibit signs of heart attacks a little differently than, than men. Um, and for years there, wasn't the wealth of studies. And so think about, I'm designing a robot--a healthcare robotic system--that's at the hospital, a patient comes in and it quickly tries to triage, you know, is this person having a heart attack or whatever. And it's designed based on this data that has how people are supposed to exhibit. Basically, women would die, right? Because it just wouldn't have that data. And so that's where the harm happens is because you replace these functions with robots, assuming that it's going to be perfect, but it's not, if it's based on bad data.
Perry Roth-Johnson: 7:24
What you just described was in one of the papers that you co-wrote in 2017,"The Ugly Truth About Ourselves and Our Robot Creations: The Problem of Bias and Social Inequity". And in addition to this medical example, you talked about, uh, search engines delivering job postings for well-paying tech jobs, to men and not women. Facial recognition systems, having problems, identifying nonwhite faces, and these problems still continue. Just last month, Facebook announced that it's going to study whether its algorithms are racially biased. What is, uh, algorithmic bias, uh, this idea that technology might have bias?
Ayanna Howard: 8:01
So algorithmic bias is that-- um, and, and it's not--and I wouldn't say technology, but the decisions that come out of the technology, um, have different outcomes for different classes of individuals. Um, and so that's really what's this aspect of bias. Um, and, and so just so you know, bias is not always bad. That's the problem. As an example, um, you know, my dosage as a woman for medication should be different than a guy, right? That's an, that's an aspect of bias. You shouldn't give the same kind of dosage to, uh, two individuals, gender-wise. We know this. Um, so bias, isn't always bad, but when the outcomes are different, because you belong to a certain group, or have a group membership, then there's this algorithmic bias. And that's really the definition. And the bias is in a couple of things. It's, it's in the data that's being used to train, it's in the design of the parameters you put into the system. And it's also even in the, how you define your outputs and how you define your outcomes. Right? And so a lot of these biases, um, are some of this is based on, you know, us as developers. Some of it is based on society because of the past decisions we've made, uh, that, you know, we eventually realized, you know, we were wrong. But we have so much data that has this kind of, you know, archaic viewpoint that's being used and trained because it's available. Um, and it's, yeah, it's a problem when it impacts, um, the decisions in terms of our lives, our livelihoods.
Perry Roth-Johnson: 9:42
Mmhmm. One way you're describing that technology can maybe not become biased, but have these poor outcomes that are, that are, uh, unequally, uh, handed out to different groups is because of the dataset that might be biased because it has a lot of past ideas embedded in that data. I've heard many experts talk about this notion of de-biasing datasets. Is that possible, is that sufficient to, to fix the problem?
Ayanna Howard: 10:11
It's not sufficient. Um, and it's just one part of a possible solution. Um, and so as an example, I have a dataset and I create a system that"de-biases"; quote, unquote"de-biases". Well, there's a developer that is creating an algorithm to do the de-biasing that says, I want to look at say age, or I wanna look at gender, or I wanna look at, um, ethnicity. Well, guess what? I have just biased the algorithm. So maybe it's de-biased for those groups, but is it de-biased with respect to U.S. versus a, you know, European or Oceana viewpoint? Is it, uh, de-biased with respect to, um, LGBTQ+? Right? Like, so it's, it's never going to be fully de-biased. It's only going to be de-biased based on whatever the developer thinks is bias. So it's only one part of the equation.
Perry Roth-Johnson: 11:08
Got it. And, and the other thing coming to mind is the person writing the program intending to de-bias the original program also brings their own biases. So like how many layers of this do we have to go until we get to an acceptable level of equity in the results?
Ayanna Howard: 11:29
Yeah. And, and it's, and, and just like, if you think about the way we do it in society, uh, the problem is, is that it takes a lot of, um, inequities, right? And the accumulation of inequities before, you know, we, as a society look back like,"Oh, I guess we messed up?!" Right? That's, that's typically what happens. Um, I, I actually believe that with AI, we can do better. But what I, why do I say that? I think we can accelerate that. Like, for us, it might take a generation before we realize that,"Oh, you know, housing accommodations has bias." But with an AI system--I mean, imagine that it can process all the top different parameters and possibilities. And instead of taking 10 years, it can do it in an hour. Right? And then say,"Oh, by the way, guess what? If we project 10 years from now, this is what's going to be the outcome. What do you want to do about that?" Like, I think we can think about it a little differently when we think about trying to mitigate bias in AI.
Perry Roth-Johnson: 12:32
Mmhmm. Is another part of that, uh, something else you've, you've written and talked about is we tend to trust answers from robots more than from other humans. Uh, unpack that for me a little bit.
Ayanna Howard: 12:42
We do!
Perry Roth-Johnson: 12:42
Give me an example.
Ayanna Howard: 12:44
I, so what we have found--and this is in a number of, uh, my, my research in my lab with my students--is that when, um, an AI system, uh, provides guidance on a decision, um, individuals, humans will, will kind of say,"Oh, yeah, that makes sense. I'll follow your guidance." Even if they had a different answer before. Uh, and I'll give you a good example. There was a study, this, this was out of a different group where they looked at search results. And, um, I mean, you know, think about it. How many of you guys, when you do your search result, actually go to the second page, right?
Perry Roth-Johnson: 13:23
Yeah.
Ayanna Howard: 13:23
You look at the first answer. I mean, none of us do! Even--the most we might do is scroll down five or six. I mean, that's the most we'll do. Um, and so there was a group that did a study that basically a large majority of individuals choose the first two options on their search results as truth. Right? And they, it's not like they come back and then choose the fourth or fifth or sixth. Um, and so what they did is that they took the answers at the bottom of the page and they reversed the order.
Perry Roth-Johnson: 13:52
Oh?
Ayanna Howard: 13:52
And guess what? They still took the first two! And what's even interesting is that afterwards, they would ask like,"Well, did you see, you know, did it, did it match your expectations?" Most of them, um, and I'm just going to paraphrase would say,"Oh, well, I put in the search criteria incorrectly." I.e., internalizing the blame because,"Of course the AI system is perfect. So it must have been my fault."
Perry Roth-Johnson: 14:21
Oh, wow.
Ayanna Howard: 14:23
Which again, it goes with this aspect of, of over- trust. We believe in these AI systems, we believe that they are, um, I won't say smarter, but that they have more knowledge. Uh, and therefore what they say is probably truth. And in my own experiments, we have validated this as well.
Perry Roth-Johnson: 14:43
Mm. How did you get interested in this particular facet of your field: bias in AI?
Ayanna Howard: 14:50
Yeah, so, uh, interesting enough, I'm, I'm an electrical engineer, right? That was my, my background, my training. I did a minor in computer science. I was not a cognitive scientist. I was not a psychologist that was like far from my, my even purview. Um, but when I started working with designing robots for children with special needs, it was therapy robots for the home. What I had to do was I had to develop models of how people behave. Um, it's a mathematical model, right? So you collect data, you create these structures. I mean, it's a human, but you have to be able to predict behavior. And so you do these mathematical models. And, um, one of our very first studies, it was with, um, children with autism. And we were looking at, um, outcomes in terms of behavior therapy. And what I found is that there was a difference between the outcomes for the girls versus the boys. Now, these were, were, were fairly young kids, which of course makes no sense, right? You're like,"Hey, it's a robot! It's kids! What's going on?"
Perry Roth-Johnson: 15:51
Right!
Ayanna Howard: 15:53
It was like--and so like a good scientist, you try to kind of figure out--you know, what are the confounding factors? Maybe it's the...right? Um, and I discovered that, um, when we were collecting data to create these systems, to train these systems--we were going into the clinics, we were doing the observations. And the fact is, is that, uh, boys are diagnosed at a higher rate for autism. And so when we looked at that data that we were learning from--it was basically, it wasn't, it wasn't kids in general and clinicians, it was boys and clinicians. And we didn't even think about it because when we were looking at the outcomes and the interventions, that's when we cared about making sure that we have gender distribution and age distribution. But the learning was just like, we're just collecting data! So, right?! We're just collecting. That's what we're doing. We're not trying to change it, just go in the clinic. Um, and I realized then that if this is something that impacts me--and I, I self-identify as a woman. And if this happened and I am very thoughtful about this, then imagine what our systems are doing that are out there when you have individuals who maybe aren't aware or just are, are aren't as concerned.
Perry Roth-Johnson: 17:08
Yeah, not as careful.
Ayanna Howard: 17:08
Um, and so I thought I needed to, yeah, not as careful. And so I, I, I figured that it was my role to do this. And also I'm an engineer. I like hard problems. And this was like one of the hardest problems I could actually see at that time. I was like,"Oh yeah, we are going to try to attack this one."
Perry Roth-Johnson: 17:28
And so picking up on that, I just want to jump back into one section of your paper for a minute. Um, it seemed like the first half of this paper was describing a lot of examples of harms, uh, that bias in AI systems have led to. And the second half was considering a few, uh, thought experiments. One of these thought experiments was how bias could affect a robot peacekeeper, or a robot police officer. Which at the time, you noted it could be especially challenging with tensions from police shootings of Black people in Ferguson--because you wrote this in 2017--and elsewhere in this country. It's obviously not a new phenomenon, but since the murder of George Floyd, a larger swath of Americans seem to be paying attention to this. And you explore one idea that has a potential to help: this"litmus test" for robotics? What, what is that? And how would that help make sure a robot police officer was treating all people fairly?
Ayanna Howard: 18:19
Yeah. So, um, the example of a litmus test, it's basically like, um a truth serum, you know, if something's going right or going wrong. So right now, uh, the way that AI was used in predictive policing was, um, systems use historical data, have AI identify hotspots. And then you deploy police officers to these hotspots to basically, um, be where the criminals might be before it happens, or while it's happening. Right? Um, and so they they've seen that this is systemic. It actually is not a good thing. Um, and so an example of a, of a litmus test would be, um, one, what would your human judgment be before you use the AI system? Right? Like, let's look at your human judgment, then let's look at the AI system, right? Like which one--are they the same or are they different? Um, and, and you can do this in a couple of formats and a couple of ways. And so you can look at the judgment from current police officers, you can look at judgement from advocacy groups, you can look at judgment--right? Like from all these different constituents and compare it to the AI system to see, you know, is, is there some type of convergence or is there some divergence? Um, you can do things like, does there seem to be a, uh, propensity for targeting, you know, one area of the city, as an example? Um, but identifying this beforehand. Because one of the things, because we over-trust these systems, once it produces its answer, we're more likely to believe it. But if we carve out kind of these parameters before we deployed the system, it's almost like,"Here's my hypothesis. Oh my gosh, my hypothesis is true! Therefore, the system is biased." And so it allows us to start also thinking a little bit more.
Perry Roth-Johnson: 20:11
Um, are there other kinds of tools that you use in your research, um, to mitigate bias?
Ayanna Howard: 20:17
Yeah, so I am, and again, this is not, um, not everyone necessarily believes in this, but I actually believe that the diversity of individuals is actually unique and special and we should make sure that we treat people based on their characteristics and parameters. Um, and so one of the things about a lot of these AI systems is that they're generalized, right? So they have all of this data. They don't necessarily have characteristics between like age or gender or ethnicity. And so they just kind of learn, learn a function. Um, which means that they're going to be biased towards the majority of their data set. And those, uh, attributes that are minority, um, are going to be assumed by, by the general characteristics. So my thing is, it's like, you know, listen, we are unique and we are different and we are diverse. And so why not just admit that and have the AI system, um, have a additional filter for that? Uh, so we did it with respect to age is one of the examples. Uh, most of the AI systems out there are not trained based on kids' data. Of course! Like most parents aren't putting out their kid's data online.
Perry Roth-Johnson: 21:35
Right.
Ayanna Howard: 21:35
And so, I mean, it's just, you just don't do that! So what we did is we took, um, a facial recognition algorithm that was already out there and, uh, looked at how I did it on kids. And it did horribly, by the way. I mean, of course it wasn't trained on kids and their facial expressions. And so we basically added a specialized filter that basically said, um, after you go through this algorithm, that's, that's there. Um, you then parse it through this special filter, if you have any indication that they belong to a kids group. And you don't have to tell us the specific age, we don't want that knowledge--because that was, we actually had to think about privacy. But just let us know that, you know, in general, they're under the age of 18. Um, and what we were able to show was that our accuracy shot up. Just by, and it was built on top of the generalized learner. So it's not that we're saying, pull all your systems back...
Perry Roth-Johnson: 22:36
Oh, it's modular!
Ayanna Howard: 22:38
It's modular! Right?
Perry Roth-Johnson: 22:39
Cool. Which also means that if I'm, you know, if I am, um, a school teacher and I want to incorporate AI, well, most of my population is going to be kids. Let's add a specialized learner to whatever is out there. If I am in a hospital, that's in a predominantly Hispanic neighborhood, let's add a specialized learner that understands the unique needs of that demographic. That's built on top of the typical medical diagnosis systems. Um, so it allows us to then address the unique natures of our community. So the key here seems to be that whenever you have a first draft of an algorithm, especially if it's generalized, you just assume from the jump that it's biased...
Ayanna Howard: 23:24
Correct.
Perry Roth-Johnson: 23:24
...and you're not surprised.
Ayanna Howard: 23:26
You're not surprised. You're just going to assume, because it's going to be biased. You just don't know toward whom.
Perry Roth-Johnson: 23:33
Okay. And so then you need to go back and kind of double check your answers and make sure that the outcomes are what you want it to be.
Ayanna Howard: 23:40
Yes.
Perry Roth-Johnson: 23:40
Well, Professor Howard, it's been a real treat talking with you. Thanks for spending the time with us, uh, you know, walking us through this important area of how robots actually affect our lives and making sure that they're having positive outcomes on them. Uh, where can people follow you online and find your work?
Ayanna Howard: 23:58
Um, so the easiest is of course, Twitter,@robotsmarts. I tend to post all the newest articles and studies off of Twitter.
Perry Roth-Johnson: 24:07
All right, Professor Howard, thank you so much for coming on the show. Appreciate it.
Ayanna Howard: 24:11
Thank you!
Perry Roth-Johnson: 24:12
Well, that's our show and thanks for listening. Until next time, keep wondering. Ever Wonder? from the California Science Center is produced by me, Perry Roth-Johnson, along with Jennifer Castillo. Liz Roth-Johnson is our editor. Theme music provided by Michael Nikolas and Pond5. We'll drop new episodes every other Wednesday. If you're a fan of the show, be sure to subscribe and leave us a rating or review, or tell a friend about us. Now, our doors may be closed, but our mission to inspire science learning in everyone continues. We're working hard to provide free educational resources online while maintaining essential operations like onsite animal care and preparing for our reopening to the public. Join our mission by making a gift at californiasciencecenter.org/support.