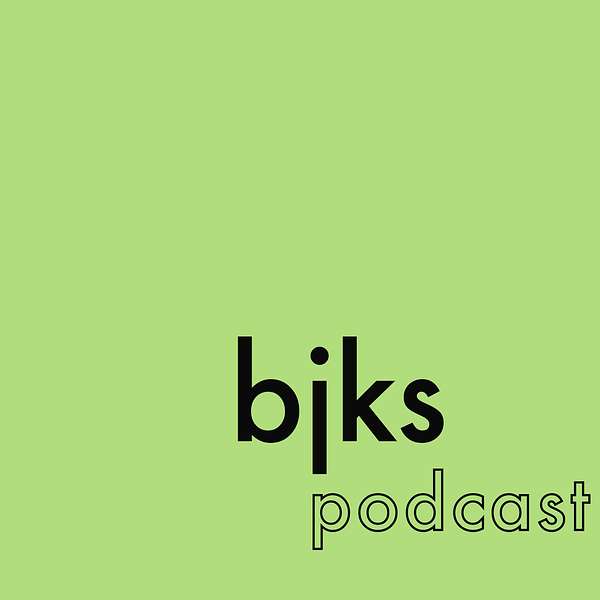
BJKS Podcast
A podcast about neuroscience, psychology, and anything vaguely related. Long-form interviews with people whose work I find interesting.
BJKS Podcast
87. Rick Betzel: Network neuroscience, generative modeling, and collaborations
Rick Betzel is an Associate professor at India University Bloomington. We talk about his research on network neuroscience, how to find good collaborators, Rick's path to network neuroscience, and much more.
Support the show: https://geni.us/bjks-patreon
Timestamps
0:00:00: What's the purpose of connectomics if understanding a species' entire connectome (as in C elegans) doesn't allow us to fully understand its behaviour?
0:03:57: Rick's very very linear path to network neuroscience
0:19:41: Multi-scale brain networks
0:43:40: Collaborations (between people who collect data and people who analyse data)
0:52:33: The future of network neuroscience: generative modeling, network control, and edge-centric connectomics
1:13:15: A book or paper more people should read
1:15:55: Something Rick wishes he'd learnt sooner
1:18:01: Advice for PhD students/postdocs
Podcast links
- Website: https://geni.us/bjks-pod
- Twitter: https://geni.us/bjks-pod-twt
Rick's links
- Website: https://geni.us/betzel-web
- Google Scholar: https://geni.us/betzel-scholar
- Twitter: https://geni.us/betzel-twt
Ben's links
- Website: https://geni.us/bjks-web
- Google Scholar: https://geni.us/bjks-scholar
- Twitter: https://geni.us/bjks-twt
References
Akarca ... (2021). A generative network model of neurodevelopmental diversity in structural brain organization. Nat Comm.
Barabási (2003). Linked.
Barabási & Albert (1999). Emergence of scaling in random networks. Science.
Betzel (2022). Network neuroscience and the connectomics revolution. In Connectomic deep brain stimulation.
Betzel & Bassett (2017). Multi-scale brain networks. Neuroimage.
Betzel & Bassett (2017). Generative models for network neuroscience: prospects and promise. Journal of The Royal Society Interface.
Betzel ... (2012). Synchronization dynamics and evidence for a repertoire of network states in resting EEG. Front comp neuro.
Bullmore & Sporns (2009). Complex brain networks: graph theoretical analysis of structural and functional systems. Nat Rev Neuro.
Cook ... (2019). Whole-animal connectomes of both Caenorhabditis elegans sexes. Nature.
Feltner & Dapena (1986). Dynamics of the shoulder and elbow joints of the throwing arm during a baseball pitch. J Appl Biomech.
Lindsay (2021). Models of the mind.
Nieminen ... (2022). Multi-locus transcranial magnetic stimulation system for electronically targeted brain stimulation. Brain stimulation.
Oh ... (2014). A mesoscale connectome of the mouse brain. Nature.
Rubinov & Sporns (2010). Complex network measures of brain connectivity: uses and interpretations. Neuroimage.
Scheffer ... (2020). A connectome and analysis of the adult Drosophila central brain. Elife.
Sporns (2016). Networks of the Brain.
Van Den Heuvel & Sporns (2011). Rich-club organization of the human connectome. J Neuro.
Watts & Strogatz (1998). Collective dynamics of ‘small-world’networks. Nature.
White ... (1986). The structure of the nervous system of the nematode Caenorhabditis elegans. Philos Trans R Soc Lond B.
Winding ... (2023). The connectome of an insect brain. Science.
Yan ... (2017). Network control principles predict neuron function in the Caenorhabditis elegans connectome. Nature.
[This is an automated transcript that contains many errors]
Benjamin James Kuper-Smith: [00:00:00] Today I'd like to start, uh, maybe with a slight provocation. I hope that's right. Um, you know, we'll be talking about network neuroscience, um, in this episode. And, uh, I wanted to kind of start with an introductory question that kind of questions to some extent the field. So from what I understand, I'm not, you know, from the field, but from what I understand, we have had most of the connectome of C. elegans since the mid eighties and the entire thing since a few years ago. Uh, but it's obviously still quite a lot of stuff we don't understand about how these worms with three headed neurons. how they behave. So kind of, I thought, my provocation is like, what exactly is the purpose of network neuroscience if understanding the entire thing still leaves out quite a lot?
Rick Betzel: Yeah, I think it's like genomics is never going to give us the full picture, right? It's a The way I think of connectomes is, it's kind of, it's a constraint, it's a, it's a network constraint on the, on [00:01:00] possible kinds of interactions. It doesn't prescribe everything. You know, if you, if you know how the, how the wheel of your car connects to the, the, the, drive shaft connects to the, the actual wheels on the, you know, it doesn't tell you how the car drives. But it places some constraints on the way you can interact with a car, the way the car functions, the way it ultimately works. So I think connectomes are a lot like that. They constrain which parts of the brain can, I'm going to use this word kind of, kind of loosely, like talk to one another, communicate with one another. no ability to communicate in the absence of a direct connection. It's not entirely true, but that's kind of the game that we play. So it constrains communication, it constrains how signals propagate in the brain. so it's putting some limits on the space of all possible activation profiles that a brain can have on the repertoire of brain states. And I think understanding how the wiring enacts those constraints is still a really important and frankly unknown [00:02:00] question. With C. elegans, like whole brain imaging is only a relatively recent advance. So we've had the connectome, but now we can situate it alongside stimulation studies, studies that record from hundreds of neurons in a behaving worm. So I think it's still an open question. What is the connectome going to contribute and exactly how? But I think understanding those constraints, understanding how the wiring activations, constrains Functional coupling and how that is supports ongoing adaptive behavior that that's that's the quest. We have hints of resolution, but far from a complete
Benjamin James Kuper-Smith: So the, the kind of, you know, idea being we have, especially in the human brain, so many billion neurons and yeah, let's, let's, let's just like that whole, like that infinity of permutations, can we somehow make that a little more manageable?
Rick Betzel: little more manageable. And in case of the [00:03:00] human connect them and even some of the other. So in the last year. We have whole brain cellular resolved drosophila connectomes now hundreds of 120, 000 neurons or so, maybe in the next decade, you'll have your first mammalian connectomes at the same spatial resolution. But there's also this question of like, can we do we need that full dimensionality? Right? I mean, like, at some point, the brain has whatever 85 billion or trillion neurons or whatever, but effectively, what is the scale? That that is important. What's the effective resolution of the connector? And if I want to address questions about. Whole brain activation states or something like that. I would wager that I don't need of those dimensions and I could, there's some core screening that we can, that we can, uh, take advantage of, um, to hopefully, again, address some of these, these big questions about what the connectome is doing.
Benjamin James Kuper-Smith: I'd like to kind of, this was more [00:04:00] like a little introduction to the topic in a sense. Uh, I'd like to kind of now, uh, get into the topic via the way you got into it. And I have to admit, I'm a little bit confused when I look at your CV. Uh, it's, it doesn't look like a completely linear path. Um, so, uh, so just, I mean, my question is basically, how do you go from physics to human biomechanics?
And then, I mean, what I especially find interesting is that Uh, at first I thought when I saw human biomechanics, I thought, Oh, maybe this is like, I don't know, the department called it this, but it's actually something different. Like in, yeah. Then I saw your supervisor when I wrote it down, uh, your supervisor's most cited paper was about.
Uh,
Rick Betzel: jumps probably
Benjamin James Kuper-Smith: uh, dynamics of the shoulder and elbow joint of the throwing arm during a baseball pitch. Yeah.
Rick Betzel: Yep.
Benjamin James Kuper-Smith: So very much biomechanics. And then somehow within a few years of that, you, you find yourself in the, in the lab of one of the most cited neuroscientists working on like a hot emerging topic. How exactly [00:05:00] did that happen?
Rick Betzel: Yeah, it's a it's a weird story. So I'm a first generation student. When I was looking for schools, I had no idea what I was I was doing back as an undergrad. I was also fairly talented in baseball. So that was that factored into my decision. And I ended up going to a school close to my home, um, because I happened to go to like baseball clinics there.
Turns out it was a good school. And I thought I could get by on. Academically on my like just intrinsic abilities.
Benjamin James Kuper-Smith: Okay.
Rick Betzel: I majored in physics cause I liked it and I was really good at it in high school, but I did not try very hard in college. I failed lots of classes and part of this was like misalignment of priorities.
I, I played baseball. I thought I was the most important thing on earth and it came at the expense of my academics. I was like I said, I failed, I failed differential equations. The first time I took it, I failed quantum mechanics. The first time I took it and it was just an immaturity issue. I mean, honestly, that was the biggest problem [00:06:00] and it took me a long time to kind of write this ship, so to speak, but when I was graduating, I can't tell me to graduate.
So I didn't have good grades. I didn't really know the process of graduate school. I had a friend I was rooming with at the time and he's like, yeah, I'm taking the GRE. I'm like, what's the GRE? I thought professors were just like people who, um, really liked teaching. I just stuck with it or something. I didn't know what that trajectory
Benjamin James Kuper-Smith: what, GRE for non Americans?
Rick Betzel: The Graduate Readiness Exam or something that, some acronym, and it's historically the scores have been used to kind of tier people into grad school. If you have a higher score, ostensibly you're better prepared for graduate school. Although that's not really true, of course, like a lot of standardized tests, it's pretty biased.
I was totally unprepared for that. I didn't really know what I wanted to do. So I thought, Hey, I could combine baseball. This thing that I really liked with physics, this thing I used to like, but turns out I'm not really good at, at the academic sense. but it took me, it took me a year. I went to a community college kind [00:07:00] of like brushing up on biology courses. I got a little more serious about my academics at that point. Like community college was really useful because it wasn't Oberlin where I went to. to my undergrad. It was little chill, a little more chill. Like, I felt smart again, was for my, good for my confidence. Um, and then I got into, I got into a biomechanics program a year later and I wanted to, I wanted to study the mechanics of baseball, specifically pitching.
I don't know, I don't know what you know about baseball, but like it's really expensive. Like the players earn millions and millions of dollars. the pitchers, people who throw, they put a lot of stress on ligaments in their arm and their shoulder, so if you could figure out ways to spare injury, or just understand what the heck is happening from a physical standpoint, from a mechanistic standpoint, it'd stand to save people lots of money. And so I got my master's. I even wrote a couple papers. They're out there. They're not on my cv. Like I kind of like Hi, hi to these things, [00:08:00] Because, because like you said, you, you look at the CV and it's like, what the heck is this? This, this really stands out. And, and I, I even stuck around after, after my master's, I said, I said, well, I'll try a PhD.
And I shifted a little bit. So I was still in biomechanics, but I was doing a little more like motor behavior and on a whim. I took Olaf Sporn's class. This is back in 2012 is right after he wrote his networks of the brain book. I think this is the first time he's offering a course on network neuroscience and it just kind of clicked for me.
I don't know if you know, not everybody has this experience. I think we're like. Some, some cosmic alignment happens. There's like, I just, I felt like I could into it very naturally about networks. It turns out I couldn't, I was kind of fooling myself, but at the time I felt, wow, I just get this. And it was easy to take the tools and apply them to data because a couple of years previously that, um, Olaf and Mika Rubinoff had just published their brain [00:09:00] connectivity toolbox.
This was like very easy to use. I had lots of data because it was starting to become publicly available. I had lots of questions and I could just like run with it. And so I asked Olaf, like, Hey, can I join your lab? And he's like, no, and I asked him, like, I asked him again, a year later, like, we're trying to like work on at a time publishing my course project. And he still said no, but somehow I got a key to his lab. Um, and I kind of just like hung out there. Just trying to learn by osmosis. and finally he leveled with me and basically was like, look, if you want to join, you have to go through the right channels. You have to leave your current program, reapply. And I did that. And fortunately, uh, I was admitted the next like graduate cycle. So it was a, it was a long meandering journey. Um, but in the end I got to Olaf's group. And it's like, honestly, the. The protracted nature of the transition was actually good because it allowed me to kind of smoothly jump into his lab smoothly move in as opposed to like [00:10:00] right out of school admitted into his group without any background knowledge without having ever interacted with his with him or his lab.
So it ended up being, um. Quite nice to have gone slowly, let's say, and taking my time to get into the group. It was awful waiting, like those two years, like, can I do work in here? But eventually, once it worked out, it was really pleasant.
Benjamin James Kuper-Smith: But why, why did he not want you? Because you had no prerequisite knowledge apart from his course or?
Rick Betzel: I'm not sure. I don't, I don't think it was so much of a no that he didn't want me. At the time, he didn't have any graduate students. This is right after Chris Honey had graduated. Um, so there's like a little gap. Hey, Olaf was doing a lot of work with Martin Vanden Heuvel, doing like rich club stuff. So he had plenty of external collaborators, um, and very young, capable collaborators. Graduate students take up time. They're expensive. I don't know. I could come up with a lot of reasons. I've never asked him why, uh, why this didn't happen sooner. Um, we're now colleagues, so I [00:11:00] suppose I could, like we, we have shared lab space, and um, I don't know why. That's a good question, I'd love to know.
No,
Benjamin James Kuper-Smith: so, but why did you, I mean, you just said you took his course on a whim. That sounds like one hell of a whim. Uh, like, did you have any exposure to, to network science or anything like that? I mean, it just, it sounds like you just got into it, like, I don't know, you got the, the, went into lecture theater at the wrong time or something, then it's just this completely different course.
Rick Betzel: it was very strange, like I, so my uh, my advisor at the time, he had like, he told me about OLAF, he was kind of like, he was a new, my advisor when I took OLAF's course, was a new hire. Um, it's kind of like looking for collaborators around the university and he, he, he knew Olaf's work because they had just published all the, some of the first human connectome maps, um, and analyses.
And I think Olaf was kind of rising at IU at the [00:12:00] time. It was the, it was the beginning of large scale conatomics, large scale network neuroscience. He's like, you should take that course, kind of. There's a couple others that he steered me towards, um, I, I had no experience, no background, didn't really know what I was getting into. And again, because it's this cosmic alignment, it just suddenly kind of, it clicked. It's like, oh, I got this now. It was the right time for me, I think, wise, and the topic happened to be hot. And it was not a deliberate. like, take advantage of the newness of network neuroscience and kind of comics, but it was ended up being a good serendipitous thing that happened.
Benjamin James Kuper-Smith: I mean, I suppose, like, how, how much of a hot topic, I mean, was it clear at the time, or was it just, like, now in hindsight? Uh, you know, because, you know, maybe it would have just fallen away, because you, you know, I don't know, couldn't be done methodologically, or something like that, I mean.
Rick Betzel: Yeah. Again, it wasn't a conscious decision. I didn't I wasn't thinking like, long [00:13:00] term, like, boy, I need I need a field that has. The power to stick around for a few decades, it was just something that was genuinely interesting to me. I was surrounded in that first course I took by lots of other people who were genuinely interested in the field. And maybe they knew better than I did, they recognized, they saw something in networking neuroscience. But it felt like very contagious, like a lot of um, a lot of widespread interest, a lot of enthusiasm for the topic. And I kind of fed off of that a little bit too. Um, it's like, wow, everybody else likes it.
I like it too, I guess. It helped that I, again, I, I felt like I had this good intuition about it, but I don't know that that was necessarily the, looking back on them, quite confident in fact, that my intuition, whatever I thought that I had was, was not exactly right and not as strong as I thought it was.
Benjamin James Kuper-Smith: But it worked out in the end.
Rick Betzel: myself a bit. It worked out in the end. I needed the PhD. I need the immersion. You can only get so much out of a semester's long course. But hanging out [00:14:00] in Olaf's lab, it really reinforced for me, you know, the need to dig deeper. And it's
Benjamin James Kuper-Smith: Are there?
Rick Betzel: more about,
Benjamin James Kuper-Smith: Yeah. Are there, from your, from, from your path, any, what do you advise? Like if an undergraduate comes to you and says like, I'm interested in this topic. Uh, do you, I don't know. Do, do you, do you think people should, should also change track quite drastically or go, I mean, like, yeah. What do you, what do you kind of make of this when you advise students and that kind of thing?
Rick Betzel: yeah, to me, if I'm being rudely honest with myself and with you, I think, I think networking neuroscience, human networking neuroscience may have its apex. I think, I think in a sense that it is, it has gone, even in the short time that I've been in the field from kind of fringe. fairly mainstream.
There's a journal. There's a There's workshops like you. You can't throw a rock at a imaging neuroscience or [00:15:00] neuro image paper and find and miss like a network neuroscience paper. Somebody's doing connectivity. Somebody's doing networks. It's kind of ubiquitous. So in that sense, I think if the goal is to like. Advance the field. I think that's very difficult to do. And I'm very frank with students in my group about that. Like, there's still room to kind of push the bubble, but all of the very low hanging topics are somebody's touched them already. I feel like somebody has done human neuroscience so you have to have some good questions.
If you're going to stay in that field, I think you have to you have to have something that motivates you. That's. Other than, hey, this is new, because it's not really that new anymore. The easy stuff has kind of been done. It has its own, it's a mature discipline. It has its own distinct subdomains. I think people really need to have a question that motivates them.
And that's, for me, like, when I'm thinking about new students or the students that are working in my group right now. They all have that, and I think that's I probably wouldn't have admitted them if I just said, I like [00:16:00] networks, what I did to Olaf. And maybe, actually, maybe that's why he didn't, uh, he wasn't so enthused about me when I first asked him to join the lab.
I didn't have a question, I didn't have knowledge. I acquired a little bit more, I could see, you know, where I would fit in. And I think, yeah, the students in my group are like
Benjamin James Kuper-Smith: but don't you kind of need. The experience to get at a good question. I don't know one thing. It seems, I just basically just finished my PhD. It seems to me that it, you know, it takes a while of, it takes like a while of working in the field, right. To figure out like, Oh, this is actually a question that's interesting.
It may be even if other people don't think so, right. I mean, it's, it's not that obvious.
Rick Betzel: it's not obvious, but yeah, I think like the mainstream nature of network neuroscience now, it's like, it's, get some by osmosis, you get some exposure and some experience, but if you just like read old neuro image papers from like the last five years, you'll learn a little bit more than I think that I did reading neuro image papers back in like 2012 because it was [00:17:00] just just wasn't the dominant topic.
But now it's everywhere. So there's the baseline knowledge is a little bit higher for people wanting to do work in this space. It's easier to get your hands on data. It's easy to get your hands on. Tools. It's easy to find lots and lots of papers about even the most arcane topics within network
Benjamin James Kuper-Smith: Hmm.
Rick Betzel: It feels that way, at least. Yeah.
Benjamin James Kuper-Smith: so, so what did you, so Olaf let you in. What did you then work on? What was your kind of question topic?
Rick Betzel: The first paper I wrote was on time varying connectivity with EEG. It's my only scalp EEG paper. It took rounds of revisions. Not an exaggeration. Six or seven. It's been many years now. But either six or seven rounds of revisions. It was the most brutal process. vowed to never do EEG again. And I haven't. Uh, at least at Scalp EEG, but I was very interested in like the notion of [00:18:00] change, like I said, and that first paper was like how networks change over time using EEG to assess that, but I'm still very interested in change and that was ostensibly like the theme of my thesis was. timescales of network change.
So we did a lot of work on the so called dynamic connectivity, these like rapid to moment changes, um, and usually functional connectivity. But we also started looking at longer timescales, um, like developments and ultimately the human lifespan too. So we did some early work in what the lifespan changes and, uh, and differences in, in. Structural and functional connectivity that kind of that theme has really stuck with me even to up to this point. Yeah, we, we, we did a lot of dynamic things back then.
Benjamin James Kuper-Smith: Yeah, it's, it's funny. It seems to me that that's a very, it seems to me that that's kind of a, a classic, like sec, like in a way, second generation research in the field topic. The first is like, what are [00:19:00] networks? The second is like, how do they change? Right.
Rick Betzel: change? Exactly. Exactly. And even generationally, like if you look at like the, some of the original, I say original, but like the, the human network neuroscientists, the first people doing human network neuroscience, it's like, well, Olaf and Ed were right there at the beginning. There's other people around and Olaf and Ed probably being the most visible. Olaf trained Chris Honey, uh, Ed trained Danny Bassett, and then there's this next generation, which like, you're exactly right. They figured out the networks in that first, that first group. And then there's us who came, I think in wave two or 2. 5, who are asking some of the different questions and the questions have evolved since then.
Certainly. Yeah.
Benjamin James Kuper-Smith: I mean, so you mentioned, uh, the, the, that you worked on different scales of network. Um, this is, you know, one of the topics I had that I'd like to talk about a little bit, particularly, maybe you had, I mean, you can kind of take it wherever you want, but, uh, the, the, the paper of yours that I kind of read in parts was a review with Danny Bassett about multiscale brain networks.[00:20:00]
Uh, so maybe what, what kind of, I mean, you already mentioned time, uh, but maybe could elaborate a little bit about the time and kind of what other kind of scales. Can networks
Rick Betzel: Yeah,
Benjamin James Kuper-Smith: have, yeah,
Rick Betzel: so that paper was intended to kind of be really be an olive branch to all of neuroscience to say, like, networks are super flexible, almost irrespective of where you're doing your science, what organisms you work with, what imaging modalities, what's, there's a there's a network for you. And I think that's, that's what we kind of meant by multi scale. And that paper, we outlined several axes that we thought be considered scales along which we could, you know, we could embed any network analysis. So you mentioned time, right? We can think about networks changing, like a single network changing over a very rapid time scale, like sub second reconfigurations all the way up to time scales of the human lifespan.
So very, very short to very, very long, but we can also [00:21:00] consider spatial scales. And we talked a little bit about this in the beginning. Right. There's a nanoscale or microscale connectomes, you know, where the nodes represent individual neurons or cells and the edges typically represent synaptic coupling between pairs of cells. The finest possible scale that we usually consider. But there's also coarser versions of the network of brain networks. There's a so called mesoscale or we kind of build inter aerial maps of axonal projections. This is often done invasively. A good example is the Allen Institute's mouse connectome. made publicly available. It's viral tracers in a lot of mice they get aggregated then by, by area. So, you know, if we put a tracer in area X, can kind of figure out where the projections from that area are to other areas. Um, and that's still invasive. And then at the very coarsest scale, there is, of course, the fusion imaging [00:22:00] data and tractography, which we can acquire non invasively.
So those of us working with human data, this is, uh, in human subjects, this is really exciting. Um, but it allows us to reconstruct this very large scale, uh, and get estimates of very large scale myelinated white matter pathways between different brain regions. a spatial component as well. And we also identified a kind of like a network scale, um, along which. You can consider different levels of granularity. And when we talk about networks, we talk about these two fundamental components, nodes and edges, circles and lines. That's like the finest elements in our network, like the node or edge level. But we can also consider the network as a whole.
We can look at things like, um, and make measurements on the mean shortest path, on average, how many steps does it take to go between any pair of nodes. It's kind of a global measure. You get back one number that describes your entire network, plus the coarsest possible resolution. But in [00:23:00] between, there's these so called analyses that focus on, like, community structure, subnetworks embedded within the network, within the broader network.
So it's not exactly properties of individual nodes, not exactly properties of the entire network as a whole, but collections of nodes and edges, subnetworks, um, embedded within the larger network. Um, and we kind of tried, we, our claim, I guess, was that you can situate basically any network analysis. Within this morphous space of scales, spatiotemporal and network. Or graph theoretic scales. And yeah, that was the big point, I think, primary point of that paper. And we walked through some examples, I think, in paper. Focusing on so called multi scale modularity. Like this notion that brain networks are modular. They can be divided into internally dense and externally sparse sub networks. But that within any one of those sub networks, those modules, they can be divided further. [00:24:00] Um, it's kind of like modules within modules within modules. And also, I think we focused on this notion of a rich club as well. if you read the papers on rich clubs, they say there's like, there's one rich club, it's a group of nodes that are highly connected, but also connected to one another. But it turns out, even there, there's a range of rich clubs that have are having degrees of exclusivity. Some are the hyper connected nodes, that are also connected to one another, but there's, there's a kind of a graded version of that. There's some, these are just nodes that are pretty well connected, but tend to be connected to each other.
So it's not a, there's not, there's not one rich club. It's a, it's a scale. It's a spectrum.
Benjamin James Kuper-Smith: I have a kind of fairly generic question. Let's say about the human brain are the different types of the brain. Do they have very different kind of topological structures or is it kind of similar? I mean, I remember roughly, uh, for my masters, we had like one about one lecture about how the cerebellum is just organized in a [00:25:00] very different way.
But that was not from a network perspective or anything like that. So I'm just curious. I kind of, is it Yeah. Are there like, can I, can you give like a broad answer to that? Or is it like very specific to each area or,
Rick Betzel: Yeah. So I'll, I'll give you, I'll give you two comments. Actually, I'm going to, I'm going to extend this in a slightly different direction initially, but I'll come back to your question. One of the phenomenal things about network science is that it is super flexible. The framework, the modeling framework is super flexible and super generic. It can take any system. If you can, if you can discretize it into and those elements can interact with one another, you can represent it as a network. So one of the very interesting Observations from even early on and like network neuroscience, like two decades ago, is that many of the these like so called organizing principles, these features that we think are really important functionally and human brains, for instance, like modules, rich clubs. drive to reduce wiring costs, efficient [00:26:00] processing paths, these are big important functional principles. We see them not just in human data, them when we look at those inter aerial mesoscale connectomes, the same sets of features. We see the same sets of features when we take, when we, when we analyze micro scale connectomes, which kind of either hints that either these are super generic properties, like. trivial for a network to have, or it's a really profound observation that they, that these types of network structures, they support, you know, they, they support adaptive behavior, all of it, even, even a worm exhibits a surprising repertoire of behaviors. And so we be surprised that there is a rich club there, some, some integrative unit for, you know, pooling lots of different sensory inputs together, integrating them and coming up with some motor plan or something like that. We see it down there. We also see it in human data. So I think of that is we're just speaking to kind of the universality of some of the network properties. I think I think that's quite [00:27:00] striking still, I think it's important point
Benjamin James Kuper-Smith: mean, just briefly, it also goes beyond brains, right? I mean, that's the,
Rick Betzel: Absolutely. I mean, like circles and lines.
If you can take a system and represent it as discrete elements that pairwise relationships, then yeah, you can, you can study a model it as a network. And in fact, some of these same organizing principles seem to be pretty ubiquitous across all real world networks for the majority of real world networks.
Small world is probably probably being the most, uh, most obvious property
Benjamin James Kuper-Smith: so I mean, like from that perspective, yeah. As you said, like, it's not that surprising that brains would have them in general,
Rick Betzel: yeah,
Benjamin James Kuper-Smith: because it seems like, yeah, any kind of complex, any like network that leads to some sort of complex output or something like that, yeah, seems to have those properties.
Rick Betzel: I agree. It's also it's so it's like early on network neuroscience. There's like this big flurry of papers that have kind of like [00:28:00] world, small world, small world. But now I think it's the field has pivoted a little bit and understand that some of some of these findings, some of these some of these properties aren't that aren't that spectacular in some sense. It's like you see it everywhere. So that's it's exciting. We can't write a paper and say we saw small worldness here because it's almost really It's almost trivial. We expected that. We have very strong priors that say it should be there. So maybe it's not as profound as we imagine or enough to carry a paper any longer.
Benjamin James Kuper-Smith: So is that, is that, uh, you mentioned you'd get back to the, my original question. Does that then, does that then mean that, yeah, I mean, every brain area has the same properties. Is that kind of,
Rick Betzel: No, I don't think that's true at
Benjamin James Kuper-Smith: okay.
Rick Betzel: So I think whole brains, I think, have a lot of shared properties for me. Person to person, across phylogeny, I think, I think we will find lots of shared global, with some of these like local Properties like communities will find communities will [00:29:00] find these kind of like organizing principles that are common, but there's still a lot of heterogeneity across the brain. The most obvious can think about heterogeneity is in terms of interconnectivity. So from node to node area to area. You can make very simple measurements, like count up the number of connections it makes or the total weights of those connections, and that number varies wildly across certainly across cortex.
And as you extend the basal ganglia and cerebellum to that number changes, it's a very coarse measurement. It's not telling us where those projections go. It's not telling us where that area is connected to, but it's kind of a measure for the proxy for the capacity to exert Influence or be influenced by I in my course I use the example of like Beyonce's account on Instagram, right?
She has like How many billion followers that's an important note in that in that in that online social network, right? a huge capacity to influence any anything that she posts gets [00:30:00] disseminated to like the world or a third of the world or why I don't know how many people are anymore quarter of the world ostensibly like It's a big broadcaster by virtue of being highly connected And then there's my Instagram account, which has like, whatever, my family and my, some of my friends from college, can say the same thing as Beyonce, but it doesn't have that same capacity to be broadcast worldwide.
And so I think, I think we think about the brain in that way a little bit, right? There are the, this actually comes back to our, in the very first discussion that we had, wiring, it doesn't tell us exactly about the communication. It doesn't tell us how the brain is working necessarily. It's telling us it's placing some limits or some bounds and expectations on.
The capacity to do something in this case to exert influence for highly connected, all things being equal, have a great capacity to influence. If you're weakly connected, you make only a handful of connections. may not be as influential unless those connections are just the right the right partners.
Um, I think [00:31:00] that's a very clear example of. Heterogeneity across the brain. There's, they're not, not all areas are equal. And, and being highly connected is, is only part of that. Right? It's like, like I mentioned a moment ago, it's also who you're connected to. We think of these, at least in human brain networks, as being composed of these kind, like functional modules, these brain systems, like we, the, the terms are even enshrined in the literature.
Kind of like there's a default mode, there's a module for vision, there's a module for. It's not a motor processing. There's another one for this control network. We use those names as shorthand and they're functionally evocative. So it makes us think of what some of these areas collectively might be, might be doing, you can be very weakly connected, but if you form a bridge between some of these systems, you might not be influential any longer, but you're in this, this highly integrative position.
where you have the capacity to interlink, uh, information, say process in a visual network with motor information or auditory information. And now [00:32:00] you're important, but in a different way. And of course, this measure of importance, um, varies across cortex as well. And so it's not just dependent upon the number of connections you make, um, but really how you are positioned with respect to some of these, um, It's called functional systems or modules.
I think I think that's another big insight that human neuroscience has contributed. Human network neuroscience has contributed.
Benjamin James Kuper-Smith: Is the number, I'm wondering, I mean, especially in the human brain, like, I was wondering whether maybe then some of the metrics you mentioned, like how many connections something has, whether that's then quite misleading, or can be it to some extent, because I mean, I don't know whether this is true or not, but let's see if you take like a sensory processing area probably has, You know, it's super important for that one thing to give it to other thing, but in this network, it might not come out as that critical.
Rick Betzel: yeah, is why we have this this multitude of network measures and why they've gained so much prominence. They, you know, the number of connections [00:33:00] indexes one possible function, the capacity to influence, but it's not giving you the complete story. The measure that indexes how your position between modules, the participation coefficient, can give you complimentary insight. Other measurements based on how important a node is with respect to, like I say, a communication process unfolding along the network. Like how, a node tend to appear on lots of shortest centrality. Tends to be correlated with its degree, but it's not a one to one correspondence. You can find a node. It's positioned exactly between two modules, so anytime they want to talk, the path has to go through that node, but it might be, it doesn't have to have lots of connections itself. So there's a tendency for some of these measures to be correlated, but there's a, you know, you can find near orthogonality if you allow for lots of measures to be considered.
If you consider lots of measures, they're not always correlated. They can give you unique insights, I think, [00:34:00] into the architecture and hopefully brain function too.
Benjamin James Kuper-Smith: Just to kind of brief aside, are these networks measuring, is it always neurons or is it also astrocytes? Or, um, I don't know much about astrocytes, but I once took a course where they said it was very important
Rick Betzel: yeah,
Benjamin James Kuper-Smith: and highly neglected. Uh,
Rick Betzel: uh, with the human data, it's neither, right? Because it's, it's, we usually work with whole brain data. We usually want a whole brain. And at that resolution, there's only a handful of techniques that can grant us a whole brain MR, EG, MEG, which is a whole brain picture. But the voxels themselves are, you know, a couple of millimeters by a couple of millimeters or.
And somewhere in there, there's hundreds of thousands of neurons. So for human data, it is never neurons. So there's a question of, you know, if your questions, if your research questions require. connectivity. Well, maybe you should find a different organism than humans [00:35:00] because we don't have those data and probably won't for a while. The connectomes that I, that I am aware of at the micro scale where you in principle could have astrocytes or glial, yeah, other, yeah, other, other cell types. They've all focused on neurons, generally speaking. So I don't, I don't know. I mean, it would be an interesting question and I'm sure somebody is thinking about networks of this type, but I'm not aware of efforts to. To build those kinds of maps, but it would be interesting. I agree. I think maybe we could talk about this at some point later on in this discussion, but I think network neuroscience is actually poised for a revolution or a renaissance of sorts. Well, we've done human MR and networks are based on human MR 20 odd years now, you know, we're getting close to 20 years. And they're, the networks we reconstruct are imprecise. MR is noisy. a very coarse scale. Functional MRI, which has been really the workhorse, is a slow, it's a slow assessment of a slow signal that is an indirect [00:36:00] measure of neuronal population activity. But now, within the last, like, two or three years, we're starting to see all of these nanoscale connectome data sets become available.
A great example is this Drosophila connectome that was published this summer. 000 neurons. The techniques are, I'm sure, being scaled as we speak to mammalian data, or eventually will be. But it's going to be a second round for network neuroscience. So I think it's delivered some on some of the promises with the human data.
But there's a lot of like, again, coming back to our first question, what are we going to do with networks? If we had one, what can we learn? And I think there might even be an incompatibility of scale with some of the tools and the human MR data that isn't such an issue when you go down to the nanoscale, where we're the units, the neural elements, the neurons are more or less unambiguously defined notion of a path in a, in a neuronal network, I think has different meaning than it does in a, in a human [00:37:00] network. So a path in a neuronal network is a, is literally a multis, synaptic pathway. the human network, I don't really know what it means if I'm going from region to region. It takes me three steps along white matter. I, it's, it's, it partly depends on how we course grain, our data, how we choose to, partially human brains have and, and it's not as obvious what the correct parcels, the correct neural elements, the correct nodes in our network should be.
I think. So I'm quite excited about these new data that are gonna become available and are becoming available at the, at the nano scale. I think it's. Our field is poised. We've developed lots of tools, lots of techniques, lots of intuition, but it's poised to start investigating these really fine scale data. And it's really a kind of a put up or shut up. Can we learn something with all these tools we've developed? not, then maybe we need to start thinking about our tools, too. There's a network neuroscience [00:38:00] framework. Like, build a network science for neuroscience. Does that make
Benjamin James Kuper-Smith: Do you still see a future in MRI in your sense then, or is it, I mean, let's say, yeah, is it just higher resolution is gonna solve the thing or is that just a bit more precise of the same thing?
Rick Betzel: I think me, I'm speaking in a very biased way here, but I think the future of human MRI And maybe part of the error along the way, but the future, I think, is the precision mapping. I think it's the, these dense sampling studies where we have lots and lots of data from individual brains. And just like, just like we do with monkeys, like when you, you can publish an NL4 study with monkeys, and you have a deep, deep knowledge of that individual. They repeat tasks thousands and thousands of times. They reach, they get their little, little sugar droplets, their juice. But human MR historically has really washed away a lot of [00:39:00] that, uh, inter individual variability for the sake of, you know, really the ease of analysis, forcing everybody's brains to look like a template brain, warping my brain to look like yours so that we can compare, choosing the same sets of parcels, the same sets of nodes.
Again, it facilitates analyses, it facilitates comparisons. But it also obscures, I think, some of the meaningful inter individual variation. And these, you can see the dense sampling studies that require lots and lots of data from a very small number of individuals. I have, I have some hope that there is, that that kind of holds the key, that general framework. I think about it in terms of like precision medicine. Like if I went to a physician, I really want them to prescribe to me Drugs that work for me,
Benjamin James Kuper-Smith: Yeah. Yeah.
Rick Betzel: history. They need to know my family history. They need to know about me. I think, you know, when we take a human brain, we can do something similar like that, right?
If I know enough about [00:40:00] your brain, I can hopefully tailor a treatment, tailor an intervention, like a neuropsychiatric disorder, based on detailed knowledge of an individual's brain. It also just informs our understanding of variation between individuals, too. I think that part is very much clear already. One brain is not the same. You know, two brains rather are not are not alike. And those differences are meaningful, systematic, and, uh, and it can inform some of the translational aspects of the network neuroscience. And ultimately, we're not going to do single cell resolution for the clinic, I think, right?
Like, we need something that we're going to get us. I don't do clinical work, but my understanding is it's very difficult to get patients in the magnet. It's very difficult to get them in the magnet for a long period of time. Um, so. I mean, it's got to be short, it's got to be something that's easy to do, and MR still might be the easiest, but we're not going to get single cell resolution at any time from a clinical cohort, right?
Like, [00:41:00] that'd be the, that's way, way, way down the pipeline, I think.
Benjamin James Kuper-Smith: I mean, it's interesting that, uh, as I mentioned before we started recording, I just talked to Peter Banduccini and I also asked him like kind of question, like what he's most excited about. And I think if I remember correctly, or. At least he mentioned it a few times throughout the episode. He was really interested also in this like, deep, detailed measuring of individual people and that kind of stuff.
Rick Betzel: I mean, in parallel, there's, there's changes, I think, um, in how in the acquisition, people are fine, fine, fine tuning acquisition, fine tuning the magnets, 70 is becoming more widespread, which can has a promise of reducing the amount of time needed to get, we have somebody in the magnet to acquire good data and resolving structures that are not obviously resolvable. With the traditional three T magnets. So I think there's, kind of in Peter, uh, Peter's wheelhouse a little bit, but it's, uh, yeah, I I think that's also a [00:42:00] very exciting development.
Benjamin James Kuper-Smith: Yeah. Are you, um Just, I've, I've always been, I don't know exactly why, but I've always been really fascinated by these new MEG systems that come out, um, the OPM MEG, um, I guess the part of the why I'm fascinated by it is because it just, you know, takes a huge machine and puts it in the size of a helmet, but is, is that, how much does MEG allow you to do with network neuroscience?
Because I guess in terms of, you know, you mentioned, for example, with patients, uh, it might be difficult to get them in the MRI and that kind of stuff, but, That then obviously impedes with some of the progress you mentioned about, like, deeply understanding someone's brain. Do you think that something like the MEG system could be of use, or is it not really the data you need for at least network neuroscience?
Rick Betzel: I, I have to be brutally honest. And MEG is one space, like one data modality. I have literally never, ever, ever touched, I know, I know There are lots of, so I, I. For me, I'm a very, like, in the weeds kind of PI. I, like, I've carried [00:43:00] that over from grad school. Like, I, I reserve judgment until I, like, touch the data.
I, like, had some experience with it. I know there are lots of people who, who do MEG, and I, I suspect there's even people doing network analyses with OPMs. Uh, or data acquired. I don't know enough about it to be truly to be to say much more. It seems useful. It seems like it circumvents some of the issues associated with like scalping, e.
g. in terms of the ability to reconstruct sources and the phenomenal temporal precision. But I couldn't honestly. Give you a I wouldn't want to give a strong answer here.
Benjamin James Kuper-Smith: Yeah, I mean,
Rick Betzel: it's it's it's outside of my space for
Benjamin James Kuper-Smith: Yeah, I guess you don't have to be an expert on every imaging modality in every species.
Rick Betzel: Yeah, I wouldn't I would hardly call myself an expert on imaging modalities. Like we, that's one of the peculiarities of my group is that we what kind of data parasites, right? We piggyback on lots of other people's extremely hard work and deep understanding of [00:44:00] the acquisition and the pre processing and post processing and the network reconstruction. We're almost like data scientists. In some sense, like we have, depending on who I'm talking to, I'm either a network scientist with a neuroscience problem or a neuroscientist with a network science problem, but we in the grand scheme, the enterprise of network neuroscience, we're really at that tail end, like, everybody's the data, done all of their thoughtful quality checks and processing, we do some processing,
Benjamin James Kuper-Smith: now it's safe for you to use.
Rick Betzel: Exactly. We're very
Benjamin James Kuper-Smith: I
Rick Betzel: and we, we, we, we, we love our collaborators who are doing the acquisitions, doing the data collection and, and working with human subjects and every intermediate step. But we, we, we benefit and I think they benefit too from, from our downstream experience and abilities.
Like,
Benjamin James Kuper-Smith: hope so.
Rick Betzel: so I acquired, I've collected myself one, the data for one study. And I kind of told myself after [00:45:00] that, I would, I would never do it again I'm like,
Benjamin James Kuper-Smith: Just not your thing, or? Okay,
Rick Betzel: maybe it was the nature of the study that, um, itself, that kind of pushed me away from it. But when I was at Penn for my postdoc, uh, at the very, very end, somehow I got interested in the gut microbiome.
I don't know why I thought like, this would be really fun to do. Um, and so we actually got a seed grant to collect some data and I really appreciate that Danny let me do this because I really didn't have the experience or pedigree that should allow me to do this, but they were like, go, go collect the data.
And so I did the MR, but it's a microbiome study.
Benjamin James Kuper-Smith: exactly.
Rick Betzel: kits for collecting samples to put it politely. Um, and they're college kids. It's like, you can just imagine all of the ways this went bad. Um, In the end, we never published anything. Fortunately, some of the MR data was usable and actually made [00:46:00] its way into a paper that I was only tangentially involved in, but I think that experience of like collecting the data and then this really crappy, excuse the pun,
Benjamin James Kuper-Smith: Very good.
Rick Betzel: um, kind of soured me on the rest of the data collection enterprise.
So
Benjamin James Kuper-Smith: I'm glad a paper came out of it. At least I am right. Otherwise that would have just been a weird hobby.
Rick Betzel: be a really weird hobby. I think at that point, I don't know if you can call it a hobby. If that is, if, you know, microbiome data collection is your hobby, it's uh, there's other words for what that
Benjamin James Kuper-Smith: Well, don't judge people.
Rick Betzel: I'm not judging. That's fair. That's fair. I should not, right. Yeah. Not, not, not something I want to do
Benjamin James Kuper-Smith: Okay, but, okay, well, that's, yeah, that's, uh, that's an unusual introduction to data collection in neuroscience.
Rick Betzel: Yeah. say the least.
Benjamin James Kuper-Smith: Yeah, okay. Um, but does, I mean, um, Kind of one question I have is that like you, you [00:47:00] know, during data collection, I'm assuming, I hope, you learn something about your data and about what you're doing.
And I guess you are by default kind of missing out on that aspect. Do you just go, well, you know, everything has a blind spot and that's mine. Uh, do you just talk a lot to the collaborators about their, how they collected it or how kind of do you deal with it?
Rick Betzel: I think it's a little bit of both to be honest. Like I think we recognize, I recognize. My lab recognizes that there are, there are things that we do very well, or at least I think we do better than the typical lab. And that's the network modeling. I like to think because we're a small lab. We're also not, don't, we don't bend to the current trends.
So, so, so to speak, we get to explore. Kind of the network neuroscience questions that are of interest to us. We get, we get, we get to do the network neuroscience. We're very, we're very good at that. And it's kind of exploration, curiosity driven research, but that means that we can be good at that and probably not other things.
And the acquisition is something that, that we are, we are keenly aware of current [00:48:00] trends acquisition, how careful we need to be with data and quality, it's also something that people get PhDs and there's labs that focus on that. And if we can align ourselves or work with groups. Who, who are very careful about their data and how it's acquired and how it's processed and how they do quality assurance. That's when I think when, when the collaborations work the best, and I think that's where we, that's where we fit in, right? It's, uh, finding good collaborators who do thoughtful research and understanding that we don't have to undertake every aspect of the research pipeline, right? We show up at 1 point, we can do our analyses and our collaborators and in discussions with them.
We talked about this, but they're thinking about the data that we're analyzing downstream. I think this best. Like, when I first started in his lab. I'm gonna curse here, but this is what he said. It was like you put network neuroscience is not magic shit in shit out If you put in bad [00:49:00] data, the insights you get are also going to be bad There's the network neuroscience isn't a rescue for you know It doesn't salvage bad data and it's just as susceptible to any other technique as any other technique To crappy data if you give it something bad, you're gonna get The bad results in the end. So I think we're keenly aware of that and for us it means finding good collaborators. Paying attention to what other people are saying are artifacts or issues or ways that say like motion creeps into your data. we try to be thoughtful about that in short.
Benjamin James Kuper-Smith: How do you find a good collaborator of that sort? I mean, let's say, you know, let's say this, yeah, it doesn't have to be network neuroscience, but you're just trying to do some sort of theoretical work. You're not collecting data, but you're very good at data analysis, that kind of stuff. How do you find someone who, who does do the data collection well?
How do you identify that? Especially if you don't have the expertise yourself to judge it.
Rick Betzel: Yeah, yeah, I think a couple ways. I think like [00:50:00] on one hand we've had collaborations where it's like literally your reputation precedes you kind of thing where it's like this is a group that has historically been very thoughtful in this space processing and acquisition. And we really like what you're doing in this paper.
Do you have any interest in tagging along, helping us out here? So we've done, worked like that. But I think just for collaborations in general, I don't like doing science with people I don't like. Which, there's very few people I dislike, but I like, another way to put that is I like doing science with people I enjoy doing science with and just talking to and like hanging out with and chatting with.
So when we, when we look for collaborators, we're also looking for that a little bit too, somebody who's like not a pain to work with. Um, there's lots of people who do good science, but who are just kind of assholes. Like I don't want that. I want to want nice people. We try to be nice too. And we promise we'll try.
And I think, I mean, that's, that's, that's our strategy for collaborations. Like can, can we [00:51:00] mutually benefit? Is there a way of mutually benefit? And if so, are we, are we going to get along and have fun doing it in the process? And if so, that's. It's usually a recipe for a, usually a recipe for a good collaboration.
Benjamin James Kuper-Smith: Yeah, I guess if you, especially if you, if you like the people, like talking to them, you also, I'm assuming more likely to find out kind of much more about the data than if you're just like, just tell me the necessary thing and leave me alone. Right.
Rick Betzel: exactly, exactly. Yeah, I think that's actually a good point. I've been on both sides of this, where like, you really just want somebody for one very specific thing. I need to detect communities in my networks, like I've been that person for people before, but it's not very, it's not personally satisfying. And I think we've also you know, we've had similar collaborators where we've asked, like, look, we really need you for this one narrow thing, like one, one, one, one thing you do really well. You know, we can add you, add you as a co author in the middle. I, I, I, I hope they never feel bad about it. Like, it's, it's, it's not, it's not as exciting a [00:52:00] collaboration.
I think it, it leaves people. Feeling a bit jaded and maybe a little bit used to, um, which isn't fun. It's not fun science.
Benjamin James Kuper-Smith: Yeah. I guess as long as it's fine, as if it's like clear from the beginning what you're looking for. And
Rick Betzel: too, right? It was like having a good rapport with somebody. If we're having a conversation, we probably don't hate each other to start out with. And it's more
Benjamin James Kuper-Smith: yeah.
Rick Betzel: that we'll have, uh, yeah, we, we set these good boundaries and expectations and yeah, yeah.
Agreed.
Benjamin James Kuper-Smith: Okay, I wanted to ask a little bit more about, I guess, network neuroscience itself, and a little bit about the future, which is notoriously difficult to predict. But
Rick Betzel: Yeah.
Benjamin James Kuper-Smith: you had, I saw this, you had this book chapter somewhere. I'll link it in the description. I can't remember what it's called right now. But you, you mentioned kind of three points that you think are exciting for the future.
which are generative modeling, network control, and edge centric connectomics. I thought, [00:53:00] can we just, uh, yeah, a few minutes on each of them? Uh, maybe what is generative modeling in general and in the context of network neuroscience?
Rick Betzel: Yeah. So generative models are basically descriptions, like a set of rules or a set of processes that seek to generate data that ideally looks like your own data, right? So, like, generative models is a set of rules or a set of processes that when you run it, it gives you a Synthetic versions of your own data, or of data that should be like your own data, and then you get to mess around with the model, what those processes are, what those rules might be, in an effort to make the synthetic data as close to your own data as possible, by some fitness function.
And so this is something that we were really excited about in the context of brain networks. Um, so network neuroscience, we've talked a little bit about this, but like historically, I think people think of it as just like applied graph theory. You have a network, you make some measure, you make some [00:54:00] measurement on it, and it tells you something about the network.
And I think that's, that's There's there's an element of truth to that, but it's also kind of dissatisfying because those measures are just descriptive, right? It's like you calculate the small worldness of your network. It's a number. Okay. My network has this. For us and for other people I think it's what's more interesting is trying to understand the processes that could give rise to small worldness.
Understanding how the network came to look the way it did. Uh, or the way it does. And so generative models kind of give us a, a playground for exploring that this idea that we can come up with very, very simple wiring rules, like, for instance, wire together, connect brain regions that are closer to one another with a higher probability than those that are far apart. A really simple rule. But if you iterate that, and you try to build networks using that rule, whoa, they actually have a lot of the properties that we think of as being very special in real world networks. They have, they look kind of small world like. They have, maybe based on, because some nodes [00:55:00] are really, really close to one another and densely packed, you even get hubs, you definitely get modules, or something that looks like modules from this really simple wiring rule. So it gives us some hints at what the kind of overarching principles might be. That explain brain network organization. And so we and others have kind of pushed those models a little bit, trying to test different wiring rules, different, different possible mechanisms for growing brains, and then comparing them to the real ones. real brain networks. The hope then would be that like, you know, whatever, whatever models best resemble or most closely resemble the real world networks. It gives us some, some confidence that, you know, maybe those wiring rules are what explain brain network organization, or it can explain brain network organization. So very low dimensional rules gives rise to high dimensional networks with quite interesting properties that look like the real ones, which is pretty profound to me. It's not as it [00:56:00] hints that order, right? It hints that there's some, there's some prescription underlying these circles and circles and lines, these nodes and edges.
It's not just a a module, build a rich club. There's some, there's some principle that guides that growth. And
Benjamin James Kuper-Smith: Doesn't that stuff already exist quite a lot though? I mean, it's from, I don't know, the thing is, again, I've read a bit like, uh, from the outside. Um, I mean, the thing that really got me fascinated was, uh, when I read, uh, Barabási, so however you pronounce his name, um, Linked, I think is his book, uh, when that came out.
And I always thought that's kind of what they did, to some extent. I don't
Rick Betzel: this is a really great point. So that some of the some of the earliest and best known generative models are like Barabasi's preferential attachment model. It starts with a core set of highly connected nodes, and then it adds new nodes to that. And they connect preferentially to the [00:57:00] highly connected nodes in the existing network, and you just iterate this many times, and it gives rise to a network that has a heavy tail degree distribution, which is really what they were trying to replicate at the time.
They observed that real world networks don't have Gaussian distributed degree distributions. Why is this? Can we come up with a model that explains that? And that was there back in 1999. Their effort to do that. The lot Strogatz model is other famous model of small world networks. It's a generative model of how we go from complete order, a totally regular lattice network to a network that has, know, small worldness, like a high, high clustering and short path length. And there's lots of models along the way. Part of the shortcomings of those specific models is that they really don't match. They're very stylistic. They don't match some of the details that we know matter for brain networks, space being one of the one of the huge issues, depending on who you talk to the geometry of the [00:58:00] brain. either. is the singular factor
Benjamin James Kuper-Smith: know.
Rick Betzel: the large scale organization, or
Benjamin James Kuper-Smith: Hmm.
Rick Betzel: the topology of large scale brain networks. There's there's there's gradations within those two, you know, between those two extremes. But the Barabasi Albert model doesn't care about space. It's totally disembodied and same thing with the Watts Strogatz model and some of these other generative models that have come along from network science proper that are super generic, and they have their own spatial versions to geometric random graphs being a clear example. But we need models. I think if we want to understand the brain taking the network science stuff right off the shelf doesn't always lead us.
It doesn't give us quite the insight that we want. We need something that takes our domain specific knowledge, integrates it into this generative modeling framework. And then I think we can make some real progress. And there's a group actually at Cambridge right now who's doing, I mean, it feels like every [00:59:00] other week they have a new, a new model on this, like, or a new variant of it.
So they're, you know, they've added weights to the generative models. They've added, doing it now with artificial neural networks. It just, it just keeps extending and extending. And there's other groups doing work too. Um, but they seem like they've really started running with it. I'm excited to see what they do. Um,
Benjamin James Kuper-Smith: So who's that in Cambridge?
Rick Betzel: So the main person I think of is this guy, um, Daniel Akartha, I think is how you say his name. I think he just got his PhD, but he's in Duncan Astell's group, or he was, I think he just graduated. But they've pushed a lot of this work, I think that's a big part of his PhD he wrote. Probably four or five papers.
They all end up in like really good journals. So it has a lot, kind of high visibility. And they've continued this. I feel like, if those guys listen to this at all, I feel like I get a paper of theirs to review like every other month or so. And always from [01:00:00] like good journals. I'm like, these guys are doing something right.
Benjamin James Kuper-Smith: Okay, yeah, I'll put, I'll put some of those in the description, uh, if you want to check it out. Uh, just, you mentioned space as one of the like specific factors that you might need to alter or take into account maybe more, uh, with brains. Well, what are some kind of other relevant factors that you don't have in standard network science that's like really important for brain science?
Rick Betzel: I mean, I think a lot of the same factors matter, right? We want we want networks that have some hubs. We know real brain networks have that. So we need some something that kind of so the way I think about these degenerative models is like space. Allows us to form short range connections very well, the brains, all things being equal.
They want to reduce the volume of connections. We have finite volume in our skull making connections requires some commitment of material sustaining them and using them requires energy and metabolism. So we want to make short. Connections ideally, but our [01:01:00] brains have long distance connections and where those long distance connections exist are highly stereotypical.
They're not, they're not like the Watt Strogatz model where it's like we have this random, we have this very regular network and a couple random long distance connections. The long distance connections in brain networks are really stereotypical. They're, they're, they're not, they're not random. They're just very long distance. So we need some, some countervailing rule that allows for And specific long distance connection. Um, and one that we and others have found works exquisitely. Well, is this notion of homophily? Um, it's the idea that things that have similar connectivity patterns should wire together, or they have a higher probability of wiring together. And so when you combine that with the spatial rule, it turns out that you get very of the models of this of this type. You get very close fits to the observed brain networks. So you have a spatial rule that says minimize cost or reduce wiring cost. And this other thing that says [01:02:00] occasionally, you can actually eject from that, that rule and you have the occasional long distance connection. And that seems to do the trick. It's, it's, it's far from a complete picture. Cause it's, it's, it's this cartoonish model. It's not really a developmental or a growth model. And the timescale isn't right, but it, but it, again, it hints that organizing principles is that we'll do with just two, two rules. can actually recapitulate a lot of the really high level network features, so maybe, at least at this coarse, large scale, MR style network, it's not as complicated as we think.
It has lots of cool properties, but I can get those with very simple models. then maybe variation in those rules is actually what explains some of the variation and say modular structure or rich clubs. It's like it's something about these rules, the way they're parameterized. It gives you networks of different character.
And maybe that's what's happening. Somebody's sick or. Develops in a typically it's it's it's some some [01:03:00] variation of these rules. Something about the parameterization or the rule itself got perturbed.
Benjamin James Kuper-Smith: Hmm.
Rick Betzel: So
Benjamin James Kuper-Smith: Okay. Yeah. Yeah. Yeah. Okay, so that's generative modeling, network control, uh, yeah, maybe again, what's, what's that briefly? How do I control a network?
Rick Betzel: Yeah. I mean, you said it. That's basically the idea is we have we have a network. A connectome, for instance, and our activation patterns or instantaneous firing rates or something like that. It defines a brain wide state. Your brain is in a particular state at an instant in time. I know when I do a working memory task, I tend to elicit a particular pattern of activation. How could I change, how could I drive my brain into that pattern? So I have some arbitrary state that I start in. I know there's wiring, and I know the wiring matters for how signals propagate. If I wanted to ping my network with some input signals, and I have a target state in mind, what should those input signals look like? And that essentially is what the network [01:04:00] controllability and network control framework is concerned with. those input signals, deciding where they should go. Where you should drive the brain, how many signals you need, and is it even possible to do to enact this particular transition from one brain state to another, because there's some brain states, given certain constraints that are not accessible and essentially that's what that is what network control is asking, can I drive my brain from its current state into a desired target state with minimal effort or very, very low effort and effort in this case, being like the amplitude of the input signals over time, the integral of their Amplitude over time. So it sounds like a really theoretical space in some ways it is, but I think people are currently trying to form bridges between some of the theory and reality. There's a paper that use some of the network control principles to make predictions. About, um, if you ablated a particular neuron in C. elegans, what would happen?
[01:05:00] And based on some of the network control properties, if you ablate the ones that network control predicts to be really disruptive, it turns out it disrupts behavior more than the ones that were predicted to not be so disruptive. They've there have been studies that have linked the input energies like the amplitude of these control signals with basal metabolic rates using pet imaging. There's there's lots of applications now, but it's but it's asking this question of now that I feel like I have a good grasp on the connectome. Now that I think I have some model of how its activity changes over time. I perturb it and predict in a particular way and predict the outcome and then flip that? Can I have a particular outcome and then select the perturbations? So it's really testing how confident we are. In some of our models, because it allows us to make those kinds of predictions and then check didn't work. It still requires a closer collaboration between, like, the theorists and experimentalists to really bridge that gap.
Like, there's only so many [01:06:00] neural technologies for doing stimulation invasive way. if we're thinking about network controls. Exogenous inputs, those inputs coming from, say, TMS, but we now we need somebody who does the theory alongside somebody does a stimulation and then we can start comparing the predictions with reality like they did with C elegance. But I think it's quite exciting.
Benjamin James Kuper-Smith: Yeah, I was also a little bit surprised that you said that was so theoretical because as you were talking about it, I thought this sounds very, it sounds to me like kind of what people would. Yeah. In the future, also like me are trying to do in cognitive neuroscience, right? You're trying to get at the mechanism of how does, how do you, how does something change the brain to do something?
I mean, very, very broadly, uh, but even if we take like a specific example of like, you know, you're in one brain set and then visual signal comes in, you know, how does that perturb the network? So you do something else.
Rick Betzel: exactly. And actually, that's, that's the most, that's like the most, uh, to me, the most interesting way to think about the optimal control, like [01:07:00] some of this network control questions, right? Like we do that every time we run an experiment, we have an expectation for what these sensory and perceptual signals should do to our brain state. Um, so it's, it's, it's a little, yeah, I think that that part is very real. The part that's kind of like. fiction still like some, some of the theoretical models imagine like, okay, well, if we had 150 stimulation sites, what would those signals look like? And I think that is where it's gotten a bridge too far, right?
It's like, we don't have the ability to stimulate the entire brain at 150, 150 different sites with sub millimeter precision and no bleeding, you know, from, from site to any nearby sites. Like that part is still very science fiction to me. I, but I agree it, it, it is in line with. Very, uh, deeply ingrained ideas in, in, in cognitive and human neuroscience, for sure.
Benjamin James Kuper-Smith: Yeah, I was also curious. I [01:08:00] don't know how much you know about this, but I thought I'd ask just in case you do. Um, so you mentioned TMS already. Um, so I'm very interested and want to use kind of noninvasive stimulation in humans. I'm curious, like, how. I mean, there are obviously a few different stimulation methods out there and new ones being developed, but how specifically can you do this in this context?
Is it, I mean, because I tend, I always thought of TMS more as like, uh, like a mini temporal legion almost. Um, but yeah, I'm just curious, like what, what your, whether you have an opinion, like how precisely you can use these. Tools and humans to actually do this precisely.
Rick Betzel: I, I don't think I have a good intuition, honestly, about exactly how this would work in prac, in a practical sense. I, I don't, I don't, I don't have a strong opinion there. That being said, I, I just went to a brain, a brain modes workshop in Hamburg, like just like a week and a half ago or something like that. I heard a beautiful talk and I can't remember. [01:09:00] The speaker's name, he is, he's at a group in Finland doing multi locus TMS, and they're, and they're specifically thinking about it in the context of spreading along white matter. I have a note somewhere. I'm still kind of recovering from that trip. It was like less than whatever a week and a half ago.
I came back. Kids were sick. I had another trip. I have a note to reach out to him, because I'm very interested in this, too, like, because that, that, that particular flavor and the way he described it, it was very much in line the way I think of signals propagating and spreading along white matter from one part of the brain to the other and trying to deliberately drive the brain into a particular state. Um, and they're doing multi locus versions of this. They can stimulate multiple sites more or less simultaneously with different stimulation parameters, and they can combine different, you know, different orientations and different directions. It's really inspiring. I just haven't had bandwidth to get back to him. [01:10:00] How was his name? Risto something. Why can't I think of his
Benjamin James Kuper-Smith: Yeah, just, I'll, I'll put it, uh, if, if they have something out, I'll put it, I'll ask you, I'll send you an email and then I'll put it in the description.
Rick Betzel: sure. Sure. Yeah, they had a pre
Benjamin James Kuper-Smith: I mean, the good thing is Finnish names are always easy to identify. So
Rick Betzel: That's right.
Benjamin James Kuper-Smith: you can tell which ones are Finland.
Rick Betzel: Absolutely. Yeah.
Benjamin James Kuper-Smith: Yeah. Um, okay. So the final one is edge centric connectomics.
Uh, just briefly kind of, uh, what is that?
Rick Betzel: So, so this is something we did. We started pre pandemic and then all the papers came out during the pandemics. We didn't get a lot of feedback on it, but it was essentially a little trick that we discovered. It turns out other people discovered it before us, but maybe they didn't fully capitalize on it.
We wanted to kind of flip So rather than thinking of, Nodes connected to one another. about edges connected to one another. I know it sounds silly, like why would you ever do this? But it turns out if you build these networks, networks that are edge edge networks, you get a lot of interesting [01:11:00] things for free.
Like overlapping communities, some things like that. So we thought about ways to do this, and we discovered that if you take the bivariate product moment correlation, it's really the product of two z scored time series. If you add it up and normalize it, you get a correlation, you get a functional connection. you don't do that, you get this co fluctuation time series. And it's telling you at every moment in time these two time series, which come from two different brain regions, are co fluctuating together. You get an instantaneous estimate of their connectivity, or co fluctuation. From that, you can build these edge edge networks, it also gives you frame by frame, moment by moment resolved time varying connectivity without any windowing without any blurring, smoothing kernels or anything like that. And so we've been pushing that a little bit for the last. Three or four years trying to see just how far, what we can use this added temporal precision to [01:12:00] learn about the brain. We've discovered these brain wide events. Again, it turns out other people discover something similar before. One of the added advantages of the edge centric approach is that these edge time series, these co fluctuation time series, they're exact decompositions of functional connections.
If you add them up, you get functional connectivity back. so we can actually investigate how these individual moments in time Contribute to the to the whole brain static network. And so we've been doing a lot of work in that space studying individual differences from the theoretical properties and trying to build some understanding of what this complexity going from nodes to edges. Actually buys us. I think we have evidence that it, you know, it enhances identifiability, makes some, in some cases, the brain fingerprint stronger of an individual. We show that in some cases it can improve, you can use it to improve brain behavior, association, things like that. And there's even, there's some new work that that's [01:13:00] going to hopefully come out really soon. So this is my little teaser that
Benjamin James Kuper-Smith: Yeah.
Rick Betzel: puts this all on some very strong statistical foundations, was kind of nice.
Benjamin James Kuper-Smith: At the end of each episode, I ask recurring questions. Uh, first one is what's a book or paper you think more people should read? Could be famous, not famous, new or old? Just something you think more people should read.
Rick Betzel: So I, I, I, I bought the book a while ago about it, but it's Grace Lindsay's book on AI and artificial neural networks and intelligence. And I wish I had read it sooner, because I, I have a student who's very interested in like artificial neural networks working in my lab. like for me, the conversations, it's always difficult to keep up.
Like my training is not in that space. He's super bright and like does lots of, just, just talks to me and I just have to trust that he's. What he's telling me is true a lot of the times, but the book was written at such a level and with a lot of historical descriptions, like this is where we are now, and this is the 200 years leading up to [01:14:00] our current understanding of artificial neural networks.
I have that and I went through that. A few months ago, again, I, again, I bought it, started reading it, didn't, finish it, but finally I've gotten through it again. It's not that bad of a read, it just took me a long time for like professional reasons and having two kids to get through it, but it was a really phenomenal read. I don't think The author needs any more bumps. It sounds like that book's like wildly popular already, but like, that would be my endorsement for a book that everybody should, everybody should read, especially given, like, since that book came out, like the LLMs and all of that kind of became a cultural thing that we talk about now.
And I think having that historical perspective, uh, like artificial neural networks and AI and it was, it was a really profound read and really enjoyable to and written at a, at a level that even I could understand it.
Benjamin James Kuper-Smith: Uh, so what's a,
Rick Betzel: good
Benjamin James Kuper-Smith: what's it called?
Rick Betzel: I was looking it up. I can't remember the [01:15:00] title. Um, I'll tell you in just one second. Models of the
Benjamin James Kuper-Smith: Okay, does that work? Okay, yeah, okay.
Rick Betzel: How Physics, Engineering, and Mathematics Have Shaped Our Understanding of the Brain. a really beautiful book. really enjoyed it.
Benjamin James Kuper-Smith: but haven't gone around to read it. I kind of really like, actually, that almost every time it's funny, like these, when I ask this, this question, it's basically one of two answers. It's either something I've never heard about, Or it's something I've heard about like 200 times, and I know I should read.
Rick Betzel: Okay.
Benjamin James Kuper-Smith: It's, it's, it's not really been anything in between so far. Um,
Rick Betzel: Yeah, yeah, exactly.
Benjamin James Kuper-Smith: yeah. And so far, I don't think I've read a single one of the ones that have been recommended.
Rick Betzel: You just have a long
Benjamin James Kuper-Smith: Yeah, yeah.
Rick Betzel: will read someday.
Benjamin James Kuper-Smith: Yeah. Yeah, yeah. No, I mean, there's definitely, yeah, it's always like, oh yeah, I should read that, yes. Um, and one day I will. Um, second question, something you wish you'd learned sooner.
This could be private. Uh, from work, [01:16:00] whatever.
Rick Betzel: I think, I wish I, early on, I wish I had known that it was okay to not have an answer to something. I think that was I thought all smart people had all answers, and so when I encountered people who didn't have an answer to a question, regarded them as like, oh, they must not know their stuff. And I also felt when people asked me a question, especially early on when I felt very unsure of myself, I felt like I had to have an answer. Even if I knew it was wrong, I a lot of times like, this is what I think, this is my solution. I still find myself doing that these days.
Benjamin James Kuper-Smith: Did you do it today?
Rick Betzel: less than that. don't think so. I probably made some crazy pronouncement early on that I'll regret. About the future of imaging or neuroscience or something like that.
But no, I do it far less now. And it's something I wish I had. seriously early on, like you don't have to have an answer. In fact, admitting you don't know something, uh, it it's, it's actually quite, quite useful. It serves [01:17:00] as an impetus for a new project or trying to learn something about it, about, about a particular topic.
Um, and, and it just, it just also reinforces that we're people, right? Like not everybody. There's, there's very few people have the answers to,
Benjamin James Kuper-Smith: Everything.
Rick Betzel: well, nobody has the answer to every question. Very few people have good answers to most questions, even if they say they do. So I think being able to admit that you don't know something is really, really profound, and it takes you on a journey, the process of like trying to figure it out, if you want to learn that, and you don't know it, there's probably a good, it's probably going to take some time, but you might learn something new along the way, and maybe get some, some resolution ultimately.
Benjamin James Kuper-Smith: but I guess it's especially useful to know why you don't know something. Like the, the, like what, what part you don't know. Um, because then you're, yeah, then you're pretty far along the way already to actually understanding some of it at least.
Rick Betzel: Yeah, if you know why you don't know, now you have a pathway for, for trying to, uh, to, to, to figure it out. Right. You know, you know, the first step at [01:18:00] least, you know, agreed.
Benjamin James Kuper-Smith: Okay, and final question, yeah. Advice for PhD students, postdocs, people on that kind of transitory period.
Rick Betzel: Be bold. I guess that's kind of silly. It goes without saying, but like on projects that seem uncomfortable. This is true in network neuroscience where I feel, and I mentioned this in the beginning, where I think our field is getting crowded. It's very difficult to stand out. It's very difficult to find. some new knowledge. It's not something that nobody else knew and I think it requires being bold and like not, maybe not doing a. I have a clinical cohort. I have my healthy controls. I'm just going to do a case control study because I know I can write the paper. It will get out. I can just keep writing papers of that type for the rest of my career, but I think like trying to trying to do something bold and original. It's not always safe, right? Because, like, Our
Benjamin James Kuper-Smith: it wouldn't be bold.
Rick Betzel: Otherwise we wouldn't be bold, right? field rewards productivity. We have whole indices that [01:19:00] tell us how good we are because we write a lot of papers or something like that. I think it's easy to build a little empire of bad papers. Or mediocre papers, let's say.
But I think doing something truly bold requires some vision. And if you can do that, then you have a chance to change. Change the trajectory of your field, or at least, at least get yourself a job, right? Like you see,
Benjamin James Kuper-Smith: Yeah.
Rick Betzel: you have a way of, of standing out, right? Like
Benjamin James Kuper-Smith: Yeah, but I was about to say, isn't it, isn't doing the kind of slightly uninteresting low risk project, isn't that perceived to be the path to get a job?
Rick Betzel: yeah, I, I think, I think so. I think, I think it works, but it's, yeah, this is, this is. Purely speculative my sense is that like I really believe this people who have the academic jobs They're not necessarily the best or people have the best postdoc. They're not necessarily the best It's like a there's a tier of people who are all who see these are all basically interchangeable in terms of like research output and so what distinguishes that one [01:20:00] person or the person who got the job from the others like we, we can all get tiered that way. is it just a flip of the coin? Is that I, I know I'm not the best person for this job I have now. I, I can say that very confidently. I just happen to get in at the right time. And so how do you, how do you get into that tier and then adv advance, like nudge yourself out so that you stand out? And I think that still requires some. bold thinking and do and doing. It's easy to say that, right? If everybody knew how to be bold and do cutting revolutionary research, we'd all be doing it. And then it wouldn't be bold anymore. But I think thoughtful research that that is Maybe doesn't, doesn't fall into that safe research occasionally is, is, is needed.
Yeah.
Benjamin James Kuper-Smith: I guess so taking risks ideally with a positive average outcome. Yeah.
Rick Betzel: we would all do it. Um, I get, I [01:21:00] sometimes find myself bored too. Like I read all the papers or I want some, I personally want, want to read something new. And I would, I think that's how that's, that would go a long way for me.
In terms of how I evaluate somebody else's research, somebody transitioning. If I was looking for somebody from my lab, like, there is that tier. There's, everybody has papers, more or less. They're all kind of in the same usual set of journals. Like, what makes something stand out? Oh, you did something really interesting that I haven't seen before. kind of cool, as opposed to just like, I took network science measures, calculated modularity, and I correlated it with age, which you could do 10 years ago, but I don't know if you can do it now and write a paper, at least a paper that would stand out.
Benjamin James Kuper-Smith: Do you, you know, would you still go with a, have a safe project though? Uh, or is it, are you, uh, do you like all of your eggs in one basket? So to speak.
Rick Betzel: You need to have some safety, right? You need like, yeah. Voices. I really opened a can of worms here, haven't [01:22:00] I? Um, yeah, I think you need to have the papers, but I think like doing having something that is like your thing, the thing that like nobody else is doing, and I don't know how to find it. Read, read from outside of other outside of your discipline, like read.
But for us, there's like reading network science papers. So inspiring, like who weren't limited by. Having to, you know, acquire data or conform to some of the norms of neuroscience. Wow. Look at all the cool technical things they're doing. Maybe we can incorporate a little bit of that wizardry in neuroscience and see how far that goes and see how far that takes us. 10 years ago that felt bold. I don't know if it would be anymore, but maybe that's a way to kind of get there. Get outside your comfort zone a little bit in terms of what you're reading and get inspired by. And other disciplines, some more work. We usually don't read or we usually don't touch. I think that's that's exciting to me.
Benjamin James Kuper-Smith: Yeah.
Rick Betzel: It would be.
Benjamin James Kuper-Smith: Maybe, maybe you should go back and read some of the biomechanics papers [01:23:00] about baseball throwing. Maybe you'll get some inspiration.
Rick Betzel: I've seen I've seen network science papers in biomechanics. Now I still occasionally read them and people, especially in papers of gate where people are doing like cyclic movements. You can get like. the phase of different joints. I've seen, I've seen the, the network science
Benjamin James Kuper-Smith: Yeah.
Rick Betzel: papers.
So worlds are colliding, um, which is all for me, really exciting.
Benjamin James Kuper-Smith: Yeah. Okay, cool. Well with that, thank you very much.
Rick Betzel: Yeah. Yeah. Thank you. My pleasure.