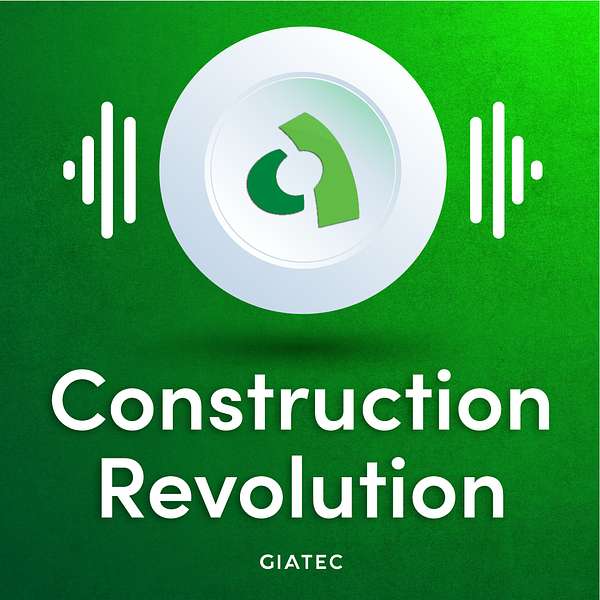
The Construction Revolution Podcast
The Construction Revolution Podcast
Unlocking AI's Potential in Construction
In this latest episode, host Sarah McGuire sits down with Sean Devine, Founder and CEO, XBE, to explore the transformative role of AI in the construction industry. With a background in logistics and technology, Sean brings unique insights into the challenges and opportunities surrounding AI adoption in construction.
Sean highlights untapped opportunities and discusses how XBE is leading the charge with innovative solutions, including AskConcrete— a groundbreaking answer engine powered by decades of NRMCA data, designed to tackle complex questions about ready-mixed concrete.
From identifying industry limitations to exploring what companies need to do to embrace AI effectively, Sean offers a compelling roadmap for innovation. He also shares his journey in founding XBE and his mission to optimize heavy construction operations with cutting-edge technology.
Tune in to gain valuable insights into how AI is shaping the future of construction. Don’t miss this enlightening discussion!
Podcast Transcript
Sarah McGuire:
Hello, concrete revolutionaries, and welcome to yet another episode of Building Better with AI. I'm your host, Sarah McGuire, and today we are going to be talking about AI and unlocking its true potential in the construction space. Today I'm joined by Sean Devine, Co-Founder and CEO of XBE, an integrated platform designed to optimize every aspect of your heavy construction process.
Sean has a background in logistics and technology. And before joining the sector in 2016, founded and sold another company, Partage, a semi-automated freight brokerage. Sean launched XBE, which leverages AI to drive innovation in the construction industry. Today Sean is focused on transforming both the company and its product through cutting-edge AI solutions. Sean, thank you so much for joining us and welcome to the podcast.
Sean Devine:
Thank you for having me.
Sarah McGuire:
So Sean, the reason why I wanted to have you on this episode to talk about truly unlocking the potential in this space, is because when we look around the construction industry right now, we're seeing a lot of buzz around AI. But it's very rare to see companies that are truly applying this technology in an extremely practical way with real-world results.
And I found that to be the case with your organization, so I found that you would probably be much more insightful about this than most. But also, I know that on our side as a company, that has been one of our biggest challenges, AI is showing us so much opportunity, but actually being able to use that in a practical way is a whole other set of challenges.
So I'm really excited to dive into this with you. But first, I'd really love to start by just giving you the floor to share your story of how you got into this industry, but also the space in AI, and just telling us a little bit more about what XBE does.
Sean Devine:
Sure. So, I started Partage. I started Partage a long time ago, but I started XBE in 2016 after selling Partage. And when I first got into the business, it was for a pretty simple reason, which is I knew from Partage, that could apply a set of techniques to optimize transportation management in niche areas to a lot of success. And Partage was focused on partial truckload and related modes of transportation.
And I was looking for a similarly sort of niche part of the logistics industry that hadn't benefited from some of the tech that was delivered to the broader logistics world, and construction materials logistics fit that bill. So it was an area that was a bit of a backwater in terms of capability. It had very particular needs, they weren't solved well enough by nonspecific platforms, and so I built XBE.
At first, this is back in 2016, for the first three years, to focus on providing a better solution for construction material logistics. So that's things that go in dump trucks or pneumatic trailers, or asphalt and rock and powder cement. The materials that are moving as part of the horizontal construction value chain, so that's where we started.
But then in 2018, we started to expand up the value chain into the supply chain, into the overall set of problems related to coordinating heavy construction. And the reason for that was saw that for every $1 that we could save someone by directly managing their construction materials logistics better, there were like $4 or $5 that were wasted.
Because the upstream coordination across functions, so between construction and materials, and trucking and equipment, was poor, so the planning wasn't that great. The scheduling work wasn't that great. The collaboration across those areas wasn't that great. So if we wanted to achieve our vision of optimizing logistics, we had to attack the whole problem.
And one thing led to another and then XBE ended up being the big platform that it is now over the next five years after that. In terms of the AI question, we did some amount with machine learning all along. But back about two years ago, back when it was GPT-2 to 3 range, it became clear that the scaling laws of AI should mean that the capabilities of models, both in terms of their world knowledge and what you could do with that and the cost of inference, were progressing at a rate that meant that whatever was true today, was going to be quite a bit different in three months and six months, and so on and so forth. And that while it wasn't clear exactly how that was going to play out, it seemed like we were at the front end of a possibly pretty long curve of continued improvement.
So I just made a decision, which is we were going to start building as if the future was already here, because by the time we got there it would be. And that one choice then led to a series of product innovations. Some in hindsight, they seemed small, but we've always seemed like we were early. I think we always have been early releasing things right at about the first moment that you could.
And it wasn't exactly because we had superhuman reaction skills. It was more that we were building in anticipation of things just continuing to get smarter and cheaper. So sometimes we were done a little early, sometimes we were done and had to wait for the actual improvements to happen, sometimes we're a little bit late.
But because we've been skating to where the puck is going for the last two-ish years, it's meant that we were pretty much on the forefront the whole time.
Sarah McGuire:
Skating towards the puck. You've already said actually quite a lot of interesting things that draw a lot of parallels to our company as well. We're in the same industry but completely different spaces. And this is what we're telling a lot of companies all the time, is to me there's no winning the game of AI. It is solving the biggest problems and then applying AI to it.
So we're both doing that in different spaces that'll ultimately complement each other. And what you said about going through the entire vertical of and going upstream, and being able to capture everything that's happening, this is what we've seen as well. Is that when we're talking about at Giatec, we're talking about optimizing concrete mixtures, but we can't just put all of this data into a platform and allow you to optimize.
It goes up the value chain, and it's actually a whole ecosystem that draws people to being able to do that. So we're seeing how that needle gets moved forward, but then we see, "Oh, well, right now if I'm not able to control what's happening at my production level at my batch plant, I'm not able to actually optimize as far as I want." And there's so many other facets that go into that.
So I love what you said there, because it's very similar to what we're seeing is that we're really only scratching the surface, and that's really a mission that we need to work on together as a whole organization. I'm curious to know when you're talking about going up the value chain and XBE being this bigger platform, are you working on fixing all of those aspects yourself as an organization?
Are you partnering with a lot of organizations to bring this together? How are you going about having one big platform to optimize the logistics space?
Sean Devine:
Yeah, so our strategy is it's a mix of two things. So first, it's to go wide and deep in every area that we can. Back in the very beginning of XBE, I called XBE a logistics management platform for heavy construction. And then that was for the first three years, and then so let's say 2016 through 2018. And then from 2019 to 2023, we were operations management for heavy construction.
So not just logistics, but the integration of logistics, construction, manufacturing equipment to some degree, both in the short-term and long-term scheduling and backward-looking analytics. So that was operations management and that's what we have called ourselves basically up until now, up until six months ago.
And just we had our user conference this past week, Monday and Tuesday, and someone asked a question during a panel of me, which is, "Hey, you've called yourself operations management for heavy construction, but it's starting to feel like that's a little limiting. That's not quite it anymore." And it was an interesting timing for that question because I agree, and I've been thinking about this all year.
And we haven't really publicly changed the way we describe ourselves, but privately, we call ourselves business management platform, which is sort of a ridiculous sounding phrase. It sounds like something out of the Lego Movie or just, I don't know, business management seems silly sounding.
Sarah McGuire:
And vague.
Sean Devine:
Yeah. What we found is that setting the fence of what we do, sort of integrating the problem within the confines of the physical operations was too limited.
Because the work that we do now extends backwards to link operations back into estimating and pricing, and forwards, which links operations into cash flow forecasting, those two primarily.
Sarah McGuire:
Got it.
Sean Devine:
So in the vertical pillar, you've got operations in the middle, but then it's sitting right dead center of the business with a crossbeam that connects it upstream to estimating and downstream to FP&A, so we see the business now as business management. It's just a long way to say that every day that goes by, it all integrates further.
And our mission generally, is to treat the problem as a single problem, not a bunch of little point solution issues that are loosely integrated. Now to point two, there are other systems that exist, even if we could do it all, which we can't. But even if we could do it all, people would have other systems to do various parts and that's fine.
So we have an API that is extremely robust. So, 100% of the functionality that our client application exposes to end users, uses our API on the backend to communicate with our server. There's not a single exception to that rule, so we are the biggest users of our API. But that API is accessible, both to us for integration purposes, and to customers for integration purposes.
So what that has enabled us to do is integrate either with upstream systems that are pushing data into XBE, or downstream systems where we're pushing data after things happen within XBE. So whatever the collection of systems that a company uses is, that's fine. Because given the API strategy, even though we'll tend to be the big, huge thing in the middle.
Whether they're adopting 100% of what we do or 30% of what we do, and whether that changes over time, we integrate well and happily with any of those upstream and downstream systems to cover the rest.
Sarah McGuire:
No, that's really clear, because that also brings me to my next question and something that you spoke about earlier. We know that when you are bringing together one big platform where people can have all of these insights, the ability for that data to speak to each other is crucial. That has been some of the bigger challenges that we found in this industry as well.
There's all these new-age technologies coming out that are giving people more insights on what's happening with their concrete. But when it doesn't all come together in one place where they can see it and visualize it, forget about AI too. Some of this is just basic algorithms at the end of the day. What good is all of this data if we're not finding a way to translate it into information in a way that we can actually take it?
So I think you've touched on that really, really well. And I'm curious to know from your side, because it was super painful for us and continues to be very painful for us, how challenging is it to get access to all of that data that you need for your customers? And can you talk to me about some of the challenges that you dealt with when, sure, you have a great API available to all of your customers and you might be the biggest user of that, but just because you have that amazing technology, does not mean the platforms that you're trying to get that data out also has that. So I'm just curious to hear about your experience amalgamating that data.
Sean Devine:
First off, the quality of the data in other systems is a bit of an unknown quantity, and all data is not created equally. It's easier to create data now than it's ever been. So one big difference between data that was created or managed in XBE, where we have some decent idea for various reasons of the quality of that data. There's a big difference between that and data that's sitting in another system, and many of those systems are permissive.
They have given users what they want, which is the ability just to scribble all over the data everywhere. And that's great on the one hand, which is like it's convenient, but on the other hand, it sort of robs the data of meaning. So there's one problem, which is to either normalize that data or cleanse it or score it and something. So, we have to deal with the fact that there's a quality issue.
Or at the very least, we don't know what the quality is, and oftentimes there's also a quality issue. It's one issue to deal with. Now, XBE is a very opinionated platform and understands the domain very well. So I think our ability to spot outliers and deal with that data cleansing, harmonization process is pretty good, but that's not a trivial issue.
I think a second one is our customers tend to be big companies in their region, but they're regional. So they're not big companies in the big, wide world sense of big companies.
Sarah McGuire:
Yeah.
Sean Devine:
And their technical abilities, therefore, are relatively limited. Even our bigger customers, which again, are pretty decent-sized organizations for what they are, they wouldn't necessarily have, at least by default, a lot of success doing the integration work themselves. So that's part two, which is not, we have to be cognizant of data quality risks.
We also have to provide the ability for them to integrate easily into our platform, which may mean doing the work for them, or it may mean making it easy for them to do the work. And it's often from the former, sometimes the latter, so that is also an issue. I think in terms of the access question, I don't know that we have actually found that to be that hard, in that companies want their systems to work well together.
I think the larger the organization, the more IT is going to inject itself into the middle of the conversation, create various level bosses to get through in order to do that sort of integration. Since most of our customers are large, regional companies, they tend to not have an IT-first culture anyhow. So they're going to tend to have the business owners driving, pulling through the organization in terms of what they want.
And the business owners want integration, so I think that's the primary reason why that hasn't, in most situations, been a challenge. I think where it can be a challenge is that oftentimes, we are integrating with our customers' suppliers. So it's not just that we're getting data directly from the company that has a commercial relationship with us, but we are getting data from multiple third parties on their behalf.
So early on, because our credibility would've been lower and we weren't very known in the industry, that took some more work in selling. Now that's less true, just because most suppliers would've seen us somewhere before and we know what we're doing on it. So there's a certain convenience that comes with that kind of familiarity.
But I'd add that into as another hurdle to overcome that depending on the timeline that I just said, used to be a bit of a challenge, but not a huge one now.
Sarah McGuire:
Okay. Well, that's very promising for someone like me to hear. For us, we've been doing this for about a year, and we've established ourselves really well with a lot of the suppliers that believe in the same things that we do. API access, availability, get the data for the customer. One of the challenges that we had, and you touched on this already, you said that you started building things as if the technology was already there in preparation for it coming.
And we've done the same thing, because we've built this really capable technology with the most new-age technology that exists. But we are really reliant on those other technology suppliers to be able to grab the data for our customers and bring it all into one place. And they didn't have the benefit of doing that because they're much older companies than we are. We had the privilege of being able to start with the latest and greatest.
They are working on a lot of older-aged systems. And currently, right now, it's about 12% of at least the ready-mix industry that is fully on cloud-based solutions that allow us to actually plug things in. Now, there are ways that we can integrate into on-premise systems, but it's very clunky, not super robust. Also, we're hedging our bets that people are on their way into the cloud, so that we'd prefer to be a part of that movement.
And then have us be able to create that seamless experience for people as they bring it all into one place, so we're not having to redo the work for them in the future.
Sean Devine:
I would say on the percentage of cloud-based versus on-premise integrations, I would think our experience matches that. I would say 90% of our integrations are with on-premise systems. It's a huge mix. We're integrated with hundreds of systems, so everything you can imagine is in that list. But the default would be that we're integrating into an old on-premise platform.
Our point of view about that is that you meet people where they are, and we're not going to pull. I don't think we're going to pull them anywhere and they're going to go on their own. And if someone could, I think we would have a shot at it because we're a big operations management system historically. So we're the system that other people would be integrated with to some degree.
So we have some gravity, I think we could pull, but things go at their own pace and I would prefer to just meet people where they are as necessary. So that means we've just gotten good at dealing with whatever it is, and part of it's like the networking can be tricky. It's all sorts of things you think wouldn't be hard, but how do we get through the networking?
Do we have a way to get through and we're going to tunnel and pull the data? Are you pushing to us? If you're pushing to us, who's manning the agent that's running that's going to do it? And damned if you do and damned if you don't on that. But we think it's easier to just accept whatever reality exists with the upstream and downstream systems, than to try to affect it all that much.
Sarah McGuire:
Well, maybe we'll have to touch base a year from now and I'll have to let you know if we've also decided to succumb to that reality, or if we're doing well on the cloud. We'll see. I think the reality is, is that for us with this newer technology, and now I want to jump in with AI.
Is that there's enough companies for us right now to work with that are in that space, that will help us prove that the AI that we've created is practical, and that takes time in itself. We don't just want to have 100 customers on tomorrow that are left to their own to figure out how to use the AI that we've created, because we need to guide them on that journey, so it takes time.
Sean Devine:
And to be clear, we're cloud-based. It's just like we connect to anything under the sun.
Sarah McGuire:
Yes. And frankly, I was in a meeting yesterday where someone said, "I don't understand why you won't connect onto the on-premise systems. You're cutting so much out for yourself." And we're like, "We know, we have boldly decided to do that, at least for now."
But new technologies evolve all the time, new mindsets, all of that, so we'll touch base in a year on that one. Going into the world of AI now and just it's exciting. Because when I first asked you to come on with us to talk about this subject, you had not launched what you just launched a couple of days ago, so this is very cool.
And before we jump into your experience working with AI in this industry, can you just set the foundation as to what SuperPower is, and who is Kayla?
Sean Devine:
Oh, yeah. So first SuperPower, and let's start with the problem we were solving, because you said it earlier and everything starts with that. So the problem that we were solving that ended up leading to SuperPower, I hinted at in things that I've said already, which is on the bright side, XBE is the big, huge, ultra-capable operations management platform that big contractors use. So that's great.
Every month, it becomes wider and deeper, more capable, but there's this trade off that happens, which is the more capable the platform is, and just think about it in terms of surface area. The larger the surface area is of the application, on the good side, that means we can solve more problems. On the bad side, it means the harder we are to use and that is an inevitable march.
So just even if you have perfect usability, which we don't, but let's pretend we did. If you have perfect usability, if you're expanding at a pretty constant rate, your usability is going to go down, because it's just more stuff to understand. More screens, more buttons, more behaviors, more interfaces, et cetera. So over the last few years, it had become a pretty difficult trade in that there's constant pressure from two sides.
We have literally daily pressure to just keep adding more, just integrate more of the business. That's pressure one. The next sentence will be make it simpler, and these things are at odds. You can't square the circle. Well, you couldn't square the circle. In the current, the typical enterprise SaaS interface, as it gets bigger, it gets more capable and harder to use.
So we were to a place where I think it was pretty clear we were going to end up in some weird middle, and I hate the middle. I like one side or the other. Like Mr. Miyagi said, "You end up in the middle, you get squished like grape." You got to get to the one side or the other, and we already clearly had made our bed in terms of being the big, capable platform. So I didn't want to see us reduce the rate at which we were adding capabilities.
I think that that's central to value creation, but we had to solve this usability problem, which again, back to skating where the puck's going, we could just see was going to get worse. Wherever it was now, it's going in one direction. So the key moment happened in July when we said, "What if we just accept that there's no way to solve the problem? It's unsolvable."
That fundamentally, if we keep building out more capabilities, that usability is going to go down. And that one moment of accepting that that was an unsolvable problem, caused us to solve it, which is we added a new application on top. Which is built on the same infrastructure as XBE, but is a brand-new, totally separate application.
So we said one, how can we make a simple application on top that gives access to all the smarts of XBE, but in a way where, as the capabilities increase, the footprint of this app on top doesn't. It has a constant interface. Also, how can we piggyback on what people already know, so that there's no cognitive load in learning in the first place?
So we built an app that looks an awful lot like Instagram or other social media apps, where our platform, which again knows basically everything about the operations of big, heavy construction companies. It has a Hot Feed, and that Hot Feed is a feed just like you'd know from Instagram of posts, relevant content from all of the things you care about.
All of the projects and the customers, and the truck and the job production plans, and the time cards, and the on and on and on, that they know about themselves. The data is in really good shape for the reason I said, and so they're constantly posting into the feed, information about themselves that's relevant to users. And then when those posts, just like a content creator would on Instagram.
When they create those posts, we know, due to machine learning and relationship between the resources, we know who should have that information in their feed. So maybe there's a new post and it's about the material site start timing of a given job today. And we say, "Well, these 49 people are the people that care about that job today because of what we know. So let's stick that all in their feed, and so on and so forth."
So that's innovation number one, we call it the Hot Feed. And there's a huge AI component to this, which is that even though the platform understands the relationship between things, actually generating content off of that information that's compelling to a person, turns out AI is really helpful at that. So we assemble the data so that it makes sense, and then push that through AI models to generate posts, to generate content, that is compelling and easy to access.
And then we said, "Well, you know what else is great about these social media apps? You can tap into the post and ask the community a question. You can comment on things," so we added that. And then we said, "Well, you know what? Wouldn't it be great if the community included a very capable bot that knew how to do all sorts of things?" And that's Kayla you asked about.
So for example, let's say there's a post about the job starting 20 minutes late, and I tap into that post, and I say, "What was the start timing on this job over the last five days? Give me the date and the minutes offset." And in the background, people are listening, like you may be listening, and you may have something to chime in, but the app is listening.
And Kayla, who's our personality for the collection of these bots, says, "Hey, I know how to answer that question." And then goes to work, looks at the history of posts, because she has access to the Hot Feed. So she can read all of the things in your feed, not to mention do other things. But she can read that and then just post back an answer and say, "Oh, I see that here's the answer for you."
But it doesn't stop there, because sometimes you want to ask questions and get answers in response to something. Sometimes you just want to ask questions out of context, which you can do right at the top level of the feed. And sometimes you want to actually ask for action.
So a simple example, let's say right in that moment, you said, "I think it'd be great if XBE added any incidents at the related plant that happened around the start time of that job. I'd like to add that information into the start timing post if you had it." So Kayla says, "Hey, it looks like you're making a feature suggestion for the platform. Do you want me to add that for you?"
Or if she finds another feature suggestion that's similar to that one that's been made before, she'll say, "Hey, someone already suggested this good idea. Do you want to upvote that?" And you get the idea. So we've got the Hot Feed, which is this unified, single place that you can go to see any information that is relevant to you and interesting.
And then we've got Kayla, who is a multi-skilled bot, that knows how to do all sorts of things, get you information or take action on your behalf, and that's SuperPower. And SuperPower is a fundamentally big deal because it flips the equation on its head. Whereas before, the increase in capability reduced usability just fundamentally. Now, every day we add new content types, and we add new skills to Kayla, and the interface remains literally the same.
It does not change at all. It's a Hot Feed and it's a social media app, so that stays completely constant, but the content and the skills go up, which makes usability go up, not down. Because instead of there being this sparse space where you wish that the post had things that it doesn't have. Or you wish she had skills that she doesn't have, the post or the feed is hydrated with more information and she's more capable.
So instead of this just constant pain of, on the one hand we're helping, and on the other hand we're hurting. We've aligned the interests and said, "Okay. On top of the aircraft carrier that is XBE, we have added an ability for most people to interface with it, in a way that they literally already know that gets easier every day." And there is AI powering literally every inch of the whole thing.
Sarah McGuire:
So this leads into the whole concept of how do we get AI to be practical, applicable and trustworthy, I think is another one? With all of these things that SuperPower's the platform and then Kayla, backed by Kayla's doing, are you finding it challenging?
And if so, what are those challenges in getting people to trust what they're seeing in front of them, and then be able to take those actions? Because I think that is the biggest concern with AI is like, "How do I trust that what Kayla is showing me is accurate? What about these actions that are being taken?"
Because certainly, that's the big learning curve that we go through and we really take our time with that, so I'd love to hear your insights on that.
Sean Devine:
Yeah, two answers. So I was pretty concerned, I mean, anyone should be concerned about this point for all the reasons you know. And I'll tell you how we were approaching it and then what's true now, so how we were approaching it is that we built pipelines to check the output to.
The models have gotten a lot better about hallucinating, so the problem is probably 85% lower than it used to be, even a year and a half ago. So a lot of it's been solved by models getting larger, and the RLHF being pretty effective in helping it avoid some traps. But 15% is still like, let's say, it started at 100 and now it's 15, that's still a meaningful issue.
Sarah McGuire:
For sure.
Sean Devine:
So we built a pipeline that for most of these where we would check the generated text against. We would have the models check the generated text and determine if they thought that they saw anything that was off.
And if it was really important to run multiple model runs and then look for consensus as another technique you can use, if the cost of being wrong is pretty high.
Sarah McGuire:
That makes sense.
Sean Devine:
So that's going to get you through a decent amount of the problem. I'm just going to add some cost and latency so it's not free but that's going to get you through a decent amount, but it's still an issue. Now, that said, o1 came out last week, and I believe the reasoning models will basically solve this completely.
We launched SuperPower Monday night at our customer event and offered a preview to everyone to use a live version right now. And I believe that o1, this is OpenAI's o1 models, so there's o1-preview and o1-mini, they became available Thursday night, the prior Thursday night. And we did some testing in the final hours because we were in a scramble to finish everything.
We did some testing to check the quality of the output, both as it relates to content synthesis and decision-making, because SuperPower has a lot of decision-making going on in it about what to do. Which skills do I enact? How do I orchestrate all this work? There's a whole orchestration layer in the middle, which is very decision-making central. So we swapped the o1 model in for the GPT-4, primarily GPT-4 class model.
Usually, it was GPT-4o that was being used for most of this, and immediately saw the challenges that we had been contending with that we had added these additional capabilities to attack, gone. So two days before we launched the product, we swapped out the GPT-4 for o1-mini in many cases that are decision-making centric. Because it's just obviously way better, and especially as it relates to evaluating reasoning about instructions and making a decision.
So our general approach now is the one-two punch of o1 for reasoning-centric ideas, or GPT-4 in some cases. And then we'll put the output of that back through a strict mode model, to force the output into parameters that fit a JSON schema so we can call a method with them. And the combination of that means that I'm actually not really worried about this much anymore.
Sarah McGuire:
Okay.
Sean Devine:
That's not to say it's a completely solved problem. I think hallucinating is you could never fully solve it, because you're going to hit sparse parts of your pre-training or post-training for that matter.
But I think that the fact that the models can now recursively introspect their output before it comes out, means they can follow instructions really well.
And that dramatically reduces the likelihood that they'll go down a path early in their answer, that sets them on a course for a problem later. Because if they do, they'll notice and restart and fix it.
Sarah McGuire:
I think it's interesting to hear all of these different almost safety buffers and factors that have been put in here, but it does seem like things are progressing so quickly, that these are correcting themselves in a really profound way. I mean, on our end with this, one of the things that we do with all of our customers is that when we get all of their data in one place, there are so many different factors.
You have Kayla, we have Roxi, and she rejects a lot of data that just doesn't quite make sense. And we can actually pull that up for companies and say, "This is all the data that's not being fed through the system, and this is why." And it ends up pointing out other places in their organization that they can go and patch up, which is kind of interesting.
But then on the other hand, we've added just anywhere where maybe Roxi could get it a little wrong, there's friction in a system. Just to make sure that it's intentional, we're reviewing, we're seeing what we're doing. And it seems like there's a little bit of that on your end as well, but that really, truly things seem to be taking off so quickly, that you might not need that as much anymore.
So Sean, I have to ask because everyone gets their AI names from interesting places. Ours came from, we have SmartRock, and so we created Roxi back in the day. So where did the name Kayla come from?
Sean Devine:
My oldest daughter, her name is Kayla, and she works for XBE. And at the time that we released Chatbot back a year and a half ago to answer questions about XBE, and this is the thing that predated Hey NAPA and AskConcrete. It first came, this chatbot that had been trained on our release notes and newsletters and glossary terms, and it knew everything about XBE.
And because at the time Kayla worked in customer success and was the most expert person about the product in many ways. So people would always write in Slack, "Hey, Kayla, how do I X, Y, or Z?" So we decided to, given that we made a bot version of her answering on Slack those questions, we decided to call it Hey Kayla. Now, just like Siri started as Hey Siri, and then ultimately dropped the hey, so it goes with Kayla.
And now she's just Kayla, because she not only can answer questions about XBE, but she can about our customer's businesses and take actions, and is listening in the background. You don't have to say hey anymore, and so we decided to keep the likeness of Kayla, but now rebrand it as just the plain name, Kayla.
Sarah McGuire:
Now, when Amazon came out with Alexa, after a couple of years of that, women who were actually named Alexa came out and said, "This is ruining my life."
They were a little overdramatic, but so your daughter does not mind having a bot named after her, and the confusion that it might cause sometimes?
Sean Devine:
Well, not only does she not mind, but when we were creating SuperPower, we weren't quite sure what we were going to call these capabilities. Because as it is with a new product, it takes you a bit to figure all the details out.
So we were considering some other ideas, and she got kind of bothered and sent me a text and said, "Oh, I'll miss Kayla. I'll miss, Hey Kayla, that's too bad." So no, very much the opposite.
Sarah McGuire:
Got it.
Sean Devine:
Two thirds of our company is based in India, and that's always been true, so still true, and she's taken multiple trips to India with me. And while Kayla is a very typical, born in the '90s name in the US, in India, it means banana in Hindi.
So imagine a name here in the US that is just the most basic name. And then all of a sudden, you go to a different place and it's like if someone was called cotton candy over here, just like a wild name.
So she went over to India with me a couple of times and would introduce herself as Kayla, and often would get this amazing reaction of people being like, "Oh my God, your name is Banana?" Literally, bananas.
Sarah McGuire:
Oh, wow. That's so funny.
Sean Devine:
It took her name from something that she didn't dislike, I don't think, but wasn't exactly fond of because it's common. And now it's a name she likes a lot because it means bananas and that's funny, and so the original Hey Kayla icon was this little robot banana.
Now the new one, it got a bit of a glow up, and so it's sort of this abstract woman with a banana, and so it has a life of its own. But no, a long way to say, she's not upset about it. In fact, it's become part of her identity.
Sarah McGuire:
Yeah. I guess when you put it all in that way, in that context as well, it's definitely flattering. It's not like she's a random woman named Kayla that is now being confused by a chatbot.
It's genuinely based off of her, so I guess the credit is due. That makes sense.
Sean Devine:
That's right. It was flattering in the first place, and now it sort of is something she's known for. In fact, at our conference that we just had, she stood up at the end of the SuperPower announcement and earnestly asked, she took the mic and said, "Hey, this is Kayla for those that don't know me."
And then asked a question about what Kayla could do. And it was just like a peak moment of at first, we were attempting to build this bot that would approximate her knowledge. And then a couple of years later now, she's standing up asking what the bot is capable of that's beyond what she can do.
Sarah McGuire:
Amazing. So Sean, I guess I'm more curious to know, do you have any examples of companies seeing things in front of them where it might be perfectly accurate, but just questioning what they're seeing?
Sean Devine:
I think that that's a problem independent of AI that exists in AI. Given that this podcast is listened to by people in construction, I'll give a very construction example. Which is oftentimes companies will say, "Hey, my team, if they know that there's a problem, then they'll do something about it. They just have to have visibility."
And then you mentioned that, "Well, the foremen can see the trucks lined up at the paver every day." Literally, right in front of them, like 50 yards down the road, he sees a stack of four trucks and says, "You're under trucked," like it's just not true. In other words, there is a real trust of the things that are right in front of you because they conflict with your narrative.
So I think that the issue, if I was to rank the sources of the pushback on information. The number one reason is that that information conflicts with someone's identity, or that it conflicts with their incentives. That's, let's call it, those are one and two. I think identity is always number one, incentives is number two. Those two root causes, trump, call it, trust to the machine by an order of magnitude.
So I think that there are issues where there's a trust in the machine issue, either rightly or wrongly, and I think it's a mix of the two. But solving the like, "Hey, this information doesn't agree with how I see myself. Or this information doesn't agree with how I'm compensated directly or indirectly." Much bigger problem, like way, way bigger problem.
So I mostly don't see that much trouble on the latter about like, "I don't trust the machine," because the machine just has to be more reliable than their alternative source of information for it to be useful. And the competitor is not that good, which is their rule of thumb or hunch, or grapevine or whatever it is.
So I don't generally find that to be fundamentally a problem. Whereas I do think that the mismatch of information to incentives and identity is a bit more of a fundamental issue.
Sarah McGuire:
That's interesting. And maybe that's why, I asked that question from our perspective of doing this in the field that we have. Is that we've brought this artificial intelligence capabilities into the hands of quality control departments, technical management roles where they're having to generate all these insights on their materials in their mixes.
And the reality is, is that a lot of these people for 20, 30, 40, 50 years have been doing all of this stuff in their head, I would say quite well, still, there's a lot of waste that happens. There's a lot of qualitative stuff in this industry as well that just simply can't go into algorithms that people have in their minds.
But the problem that we have now, is that we're bringing this technology and we're saying, "Look at how much more you can do." And we are seeing some people are very excited about it because they see. But then other people will look at it and say, "Oh, you're showing me all of this money on the table. My boss is going to see how much money I've been sitting on."
But the reality is that you as one human, you could never do this because you have to do all of the mundane work just to generate these insights. Now we can actually give you the insights to take and have an impact on. And there's this fear of, "Well, you're showing me how much better I could be doing my job."
But the reality is, is that if you're not using the efficient tools to do your job, how could you possibly do that much with your time? It's just humanly impossible. So that's one of the gaps that we're trying to bridge, is you said it perfectly, it conflicts with their personal identity because they believe that they are experts in QC.
They are correct. They are absolutely experts in QC, but they're doing a lot of tasks day-to-day that doesn't require that expertise, and then they don't get to use that expertise as often.
Sean Devine:
Yeah. And I think that there’s, I'm of mixed minds on this point. Which is like there is a lot to learn. So people are bad at optimizing, but they're good at creating robust solutions to problems. So what we have to be careful of is sort of over-optimizing and throwing away the risk management that's embedded in the heuristics that they're using. And this is, I'm a bit obsessed with antifragile thinking, and this is core to that idea.
And this is a great thing about AI, is that it is good at understanding heuristics and human ideas. So I think that what we want first, is to not replace the rules of thumb and heuristics with perfect optimization that tends to be fragile. In other words, it's really right if the assumptions are right, but if the assumptions are wrong, it'll go to heck pretty quickly.
And people's solutions tend to be pretty good across a range of uncertainty but not optimal, and I think that we don't want to make that trait. We want to allow them to pick the right place that's either antifragile or robust against uncertainty. Instead of having the model think that the inputs are exact and precise, which they aren't, and optimizing the problem saying, "This is definitely the right answer."
When, in fact, every parameter that's going into it is a probability distribution that's unknowable. So I think that that's point one, which is we want to be careful that oftentimes the machine's solving a different problem than the person is. And we want to integrate the sensibilities and say, "Is our goal to be perfect in the universe we've imagined is going to happen, even though we don't know?"
Or do we want it to be robust or antifragile, in that it does well in the face of uncertainty? I like to think about these ideas of knowing versus noticing. We want to do a better idea of noticing, and we want to not try to know everything. It's not enough to say that, "I don't know it all." You have to say, "I can't know it all."
Sarah McGuire:
It's interesting because the whole concept of evolving as a society is to always think that we could do a little bit better and better every time. And whenever we stop to do that, then we stop progressing. In general, scientists, their whole job is to prove that they are wrong so that they can get to a new solution or new theory in whatever they're studying.
And so, if we're looking at this newer technology that's coming out, and we're not saying, "How can we use it to make ourselves better?" Or just throwing it away because we're afraid of it, then we're also just not evolving.
Sean Devine:
I mean, the way that the system gets better is by having individuals that can fail. You can't have it both ways. In other words, these two are deeply related.
Sarah McGuire:
Absolutely.
Sean Devine:
The antifragility, that means the strength under uncertainty of the system, is inversely related to the fragility of the individuals. And so, we can have a difficult time accepting that that's true, that that's the way it is.
In other words, if you want to have super strong individuals, you're always right down at the low level, you won't progress. You have to explore the random space, just like happens with genetics, explore the random space.
And then again, you don't have to know in advance which ideas are going to be wrong, you just have to notice when they're wrong and stop doing them.
Sarah McGuire:
And that's fair. I think also, especially when we're talking about the world of construction, we see that technology adoption in general is just slower in this industry. But we're dealing with very heavy building materials that if something goes wrong, it can be fatal. So not in every scenario, but we've had this as an organization with our sensors, for example, that we have that go into concrete.
They give you the strength of your concrete on your phone, instead of having to crush field cylinders. And there's a lot of skepticism around this because people are used to breaking a physical piece of concrete, seeing it broken and seeing the strength result. So putting a little sensor into the concrete and trusting that the data that you're getting on your phone is accurate enough for you to strip forms and move on to the next stage in your project, that's a big deal.
Now, it's been used for many decades. It's really reliable and there's a lot of research to show why it's actually more reliable than the other testing methods in the beginning. However, it's still getting people to a level of comfort with that, I think it is fair to have to go through that educational curve with people in a slower way just to make sure.
But as somebody who's very excited about technology, yeah, there's some frustrating days where somebody says, "But that's the way that we've always done it." And you're just sitting there going, "But this is a problem that you can solve today. I can help you solve this problem." For sure, it goes two ways.
Sean Devine:
On that point, I think that seeing the problem to solve as building solutions that are robust against what we don't know will happen. I think that the degree to which systems are designed that way, not to say like, "Hey, good news, the machine is here. Now we just will make the right, perfect choice."
Because I think that one of the things that people like in a situation, like you said, where there's a lot of risk. The reason people trust themselves is that they intuitively know that they have to buffer against things. They know they don't know it all, and so they'll stay far from the edge.
Whereas they don't trust the machines to stay far from the edge. Well, they shouldn't. Machines generally are programmed by people to be far too confident and to just go right to the limit. And then it turns out that when the limit is past the limit because the inputs were uncertain, it screwed up.
So I think that we should meet people where they are in saying that, "Hey, we are going to solve the same problem you are solving intuitively, which is to create solutions that aren't right in an expected value way. They aren't right in the predicted case, but they're right in the multiversal way. They're right across every possible option that is going to happen."
And when you start meeting people there, then they're like, "Oh, it's not that I didn't trust technology, I thought you were solving the wrong problem."
Sarah McGuire:
“Meet people where they are” is a great way to kind of close the loop back on how we started this whole conversation in the first place.
I think that's perfect. So Sean, on that, can you tell me about the super agent that you created with NRMCA?
Sean Devine:
Sure. AskConcrete is on its way, and we're really excited about it. So AskConcrete is a chatbot that's been trained on all of the proprietary content that the NRMCA has produced over its history. So hundreds of documents, thousands of pages, all of the information that NRMCA staff refers to if you ask them a question about technical matters, or business matters related to the concrete business.
AskConcrete's a chatbot that has all of that information in its library and can answer questions about anything related to concrete. We have a lot of related experience because of a project we did with the National Asphalt Pavement Association called Hey NAPA, and that's used by thousands of people. So that's state agencies and municipal leaders, and contractors and truck drivers and homeowners, everyone you can imagine.
And they use that platform to answer their questions about all aspects of asphalt. And so, we launched that a year and a half ago, and launched a version two this summer. Has had just a wonderful reception by the community, and so decided to do something similar with NRMCA.
Sarah McGuire:
And we are recording this before NRMCA's ConcreteWorks, but at ConcreteWorks in Denver this year, is when it's going to be launched. I got to see a little preview of it, and that's actually how we met in the first place this summer. And I have to say I was really impressed with the way that that works.
What I really liked is you've taken all of the data from legitimate sources, that personally, as somebody who's working in this industry myself, when I'm trying to go and look for specific numbers of all the NRMCA benchmarking surveys, for example. When I'm trying to look for something very specific, I don't want to comb through all those pages.
I just want to go into AskConcrete and say, "Give me the P&L statements for the bottom quartile of the NRMCA producers last year." I just want to be able to see that number in front of me. And not only are you able to populate that with the resources, but you actually also are referencing everything in terms of where you're getting it, which gives people that credibility of, "I can trust the source."
So I thought that was one of the things that popped out to me specifically just because of my own experience. But I'm excited to see what people say and respond to it because this year, the IT Task Group for the BAC Committee is actually going to have a booth, and they're going to be showcasing it, right, at the show?
Sean Devine:
Yeah, that's right. Yeah, we're excited too. I mean, we built Hey NAPA as a service to the asphalt industry, and it's just been an amazing experience to be that provider.
So doing the same thing for concrete now again, especially given that many of our customers are in that business as well, is pretty special.
Sarah McGuire:
Well, Sean, thank you so much for hopping on to have this call. I learned a lot from you today. I'm sure our listeners are going to learn a lot.
Is there anything that you want to say as closing statements before we wrap it up?
Sean Devine:
No. If anyone wants to catch up with me, you can go to X-B-E.com, and you'll find your way to me there.
Sarah McGuire:
Perfect. And yeah, to that point, with all of Sean's contact info, with his permission when we publish this podcast, we'll have links to your website, links to your products, some of the things that we talked about here. Maybe even a link to AskConcrete so people can find that, so that will all be made available.
And by this time, AskConcrete will have been unveiled, because we're holding off on releasing this episode until NRMCA gets their moment in the way that they deserve to. So Sean, thank you so much for being on. This was a really helpful discussion, and thanks for being part of Building Better with AI.
Sean Devine:
Thank you very much, Sarah.