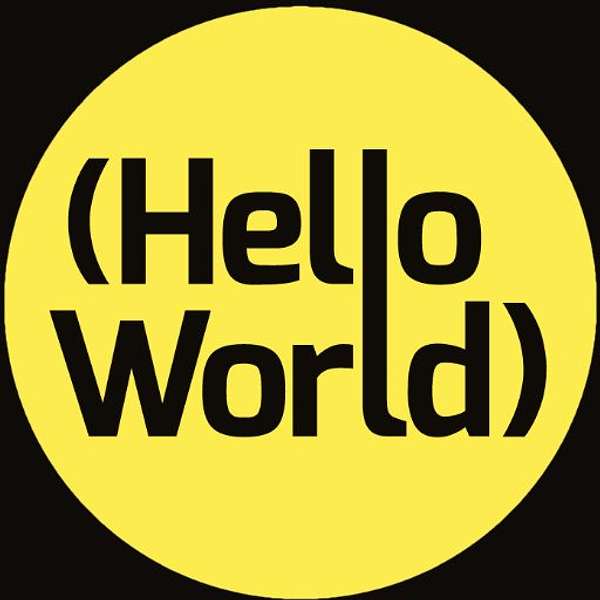
Hello World
Are you an educator who’s passionate about computing and digital making? If so, this is the podcast for you!
Join hosts from the Raspberry Pi Foundation as we chat with teachers, researchers, and learners from around the world to discuss the latest research, debate the big questions, and provide practical tips to help you engage and educate young people in computing. We’ll be releasing a new podcast series to coincide with each new issue of the Hello World Magazine to continue the conversation.
We’d love to hear from you! As we trial new episode formats, welcome additional hosts, and endeavour to produce regular episodes, we’d really appreciate your honest feedback about what you find informative, engaging, and, most importantly, helpful. Drop us an email on podcast@helloworld.cc and subscribe to the Hello World Magazine for free at http://helloworld.cc
Hello World
How do we engage learners in data science?
Understanding data has always been an important skill, but particularly so now as data is all around us and interacts with so many parts of our lives. Analysing and interpreting data can be challenging for learners, particularly if they don't see the value in doing so. Providing them with engaging and relevant opportunities to work with data is crucial.
Full show notes:
https://helloworld.raspberrypi.org/articles/how-do-we-engage-learners-in-data-science
But I think, you know, whether or not you're, I don't think many of us would look at a spreadsheet full of data and like, get Buzz of excitement about what that data could be showing us.
Rebecca Franks:James would by the way
James Robinson:Is this, you dodging doing some ballet on the Come on, I wanna see your routine.
Ben Garside:I can if you want to.
James Robinson:Yeah Welcome to Hello World a podcast for digital making. I'm James Robinson, a content creator for the Raspberry Pi Foundation, specialising in Computing Pedagogy. As Carrie Anne is away, taking a well-earned break. Today, I'm fortunate to have another amazing Educator stepping in to join me as co-host for this episode.
Rebecca Franks:That's a lovely introduction. Hi, I'm Rebecca Franks. A learning manager for the Raspberry Pi Foundation, specialising, in secondary Computing, education, which is 11 to 16 year olds. If you want to support our show, then subscribe wherever you get your podcasts and leave as a five-star review.
James Robinson:It's great to have you with me, Rebecca and up. Today, we're asking how do we engage our learners in data science. Understanding data has always been an important skill, but in particular now is data is all around us and we interact with it in so many parts of our lives. Developing our learners data Literacy is an important part of modern education, but why should they care and how do we best support them? Rebecca, I'm afraid it's time to put you on the spot. So first of all, what do you think we mean, when we talk about data science and data literacy, and how do we make it relevant for our learners.
Rebecca Franks:Okay. Well, I would say that data science many different means and finding the value in that data and that value can be found through many different forms, but the most common would be a data visualisation which isn't always what we would traditionally called a graph that we might have produced in our maths or science lessons at school, but it can be so much more than that. And I am sure we will learn more about that from our guests as well. Then I would say that being data literate means having the tools to look at the data that is presented to us and question its validity. And I feel that nowadays we are exposed to so many facts and figures in the news and on social media that it's becoming really hard to see the facts behind the fiction. And by developing our data literacy skills. We can learn to look at the source of the data. The representative samples that were used. And the also the ethics, which is really important behind the data collection in the first place. And this can help us to make more informed decisions in our day-to-day lives.
James Robinson:I agree with you in terms of that explanation I think it's really important. And as you say, it's all around us and we want our learners our citizens of the future, to be able to consume data, critically, to be able to make up their own, their own mind, based upon the the data that they are being presented with and to be able to see through data that is perhaps being used to convince them or persuade them where maybe actually it's not being used as as well as it could be. And you mentioned visualisation. I'm sure as you say, we're going to hear from our guests, but I think one of my favourite books and I haven't taken it off the, my yet coffee table, haven't picked it up and looked at it for a while. It's called information is beautiful, and it is a really fantastic book. And I think that's an updated version where they take lots of big data sets and they visualise it and demonstrate it in really interesting and innovative ways. And for me that just sort of really kind of shows the power of visualisation that the data. So and I think tapping into the data and the matters to our learners will help encourage them to be excited about data and see the value in being data literate and to help us explore this question further. We're joined by two. Fantastic guests who between them have ample experience teaching and writing about data science. Our first guest is Simon Johnson teacher author and Raspberry Pi certified educator and host of #CasChat, a regular Computing education focused Twitter chat. Simon recently wrote an article about contextualising data science for issue 16 of Hello World magazine, Simon. Welcome. and what do you think if you went as data science fit into the Computing curriculum?
Simon Johnson:First of all, thank you for having me having It's an honour. With regards to your question. I think at it's heart data science is solving problems. So the ability to think analytically and to recognise patterns are key components of computational thinking. Also, if you think about data science, if your using an algorithms to extract knowledge to understand data, and structure data, but as we will talk more about, it also helps to provide a context to many of the concepts we teach. I'd like to go back to what Rebecca was saying or alluding to about visualisation is one of the problems with the Computing. Curriculum is that is and it's going to sound a bit silly. But there's too much focus on the Computing side.
James Robinson:And so, what does that mean in practice? I mean, when we talk about what steps, can we talk about giving students context? What do we mean by that? What steps can Educators take to give their content more relevance to their Learners and believe me? I know what about this. I'm a trained maths teacher and you know "why we learning this?" was a very frequent question particularly with abstract concepts in maths. So yeah, what can what can educators do Simon?
Simon Johnson:Oh, completely agree. I feel for maths teacher sometimes, I remember asking my teacher."Why are we doing Pythagoras, when am I going to use Pythagoras, in the rest of my life. I'd start by asking yourself a couple of questions, key questions, and by answering those questions, you can help your students relate to the subject. So, the first question would be, does this relate to students aspirations or common interests. I'll kind of answer these questions myself in a moment. The second one is, "Does it involve skills that I can use in the workplace?" or the students can use the workplace? And finally, can it be linked to popular culture? So if we look back at the first question, does this relate to students aspirations or common interests? There's a bit of controversy about this and about using students aspirations in the interest in subjects. So there's kind of two ways of thinking about this. The first of the student centred or student Centric approach, and the other one is contextualisation. I used to do a lot of PBL, so if you've not used PBL, PBL is big in the states, it's quite popular in the UK as well.
Rebecca Franks:What does that mean for our listeners? What does PBL mean?
Simon Johnson:So PBL is Project based learning and it's It covers multiple disciplines and the idea is students work on a project. So they have a project manager. They have to do research a topic and then they'll just create a design, And then they'll the pitch, their idea. Very similar to like Dragons Den, and as well as focusing on their topic students are assessed on their collaborations. Their skills, team skills or that listening skills. It's usually focused on an interest. Another example is genius hour. So genius hour came from big companies like Google where they give their employees a day in the week where they could work on their own project, their own passions and things like that. I think was Google Docs came out of genius hour. So, a lot of schools have took on this idea, where students can then on a Friday afternoon, possibly work on a project that interests them. The problem with that approach is and I used to do this, when teaching, Photoshop, I'd say right this week. We're going do Photoshop and you're going to create an album cover of your favourite artist, and I find that the children would spend half the time actually, just finding pictures of the artist rather than focusing on the skills. Whereas with a Contextualisation, you're using something that's relatable to the students. So, for example, if your working on a project on creating infographics, you could use mobile phone habits. so get the students to interview each other. Ask each other about how they use their mobile phones. Whether it's for gaming for watching video or actual texting. Who uses their phone to text any more. But then use that and create data visualisation. So you're using something that they can relate to but they're not going to focus too much on that interest. The other question to ask yourself is, does it involve skills they can use in the workplace. I love this quote, "when I grow up. I want to be a data scientist said no child ever". But a recent report by LinkedIn. I think is a 2020 emerging jobs report. Highlighted that data science roles are starting to appear across almost every industry and it's one of the biggest growing sectors. To give you some examples of few seasons ago. Liverpool Football Club won the Premier League. And the went on this really magnificent unbeaten run and a lot of that was owed to data science. So they actually employed mathematicians and data scientists to analyse the players and the style of play and try to predict how and where best to place players in certain situations to get better outcomes, which is quite interesting. And students are not aware of this. Ask yourself. Can it be linked to popular culture? So a lot people are probably already aware that data analytics and data science and AI is involved in things like Netflix so whenever you watch a video or film on Netflix. It will take that data to decide what other shows to purchase based on popularity or to commission or also give you ideas or links or suggestions on what to watch.
Rebecca Franks:That's really interesting. So when you're given us loads of ways to contextualise it already, that's that's a really really useful thank you.
James Robinson:Yeah, absolutely. lots to think about there. I think probably time to bring in our second guest. And so also with us today is Ben Garside a former a secondary teacher who develops learning materials here at the Raspberry Pi Foundation. Ben wrote the secondary data science units for Teach Computing Curriculum as well as a recent article, entitled "Data visualisations, for inquisitive minds", which was in issue 16 of the Hello World magazine. So Ben, same question, but with a follow up to you, so where does data science fit into the Computing? Curriculum? And why do you think think visualisation is a powerful way to engage our Learners.
Ben Garside:Where, in where the data science fits into because if you were to look at our English, our English National Curriculum program of study, interestingly, there's no mention of data science anywhere. So what I thought I'd do when I found that out. I thought I'm just going to go to find out what a definition for data science is. So I did what everybody does and went straight to Wikipedia. So Wikipedia says data science is an interdisciplinary field that uses scientific methods processes, algorithms to extract knowledge from noisy structured and unstructured data to gain actionable insights. So actually, when you look at those terms, you know, there's a lot of common Computing terms in there. And if you look at what the outcomes are for, you know, actually the English National Curriculum is that we want our students to be able to analyse problems to be able to use abstraction, logic, algorithms, apply information technology to solve problems and be responsible and creative users of information communication technology. So I think whether or not you're a teacher in England or anywhere else in the world. All of these are, you know, these common Computing terms are together I think, encompass all the skills required to be a data scientist. You know, so it's about analysing data to solve problems or collecting and preparing data, analysing it to probably possibly by using algorithms in order to provide insights. So suppose where it fits into the curriculum is it's whenever students start learning about Computing. I mean, younger children can be taught that there are fundamental differences between data and information. And they can take a very simple project of collecting data doing some analysis on it and just finding a different way to present that data from its raw formats. And then, of course, the children progress throughout School I think these skills can be developed further by looking at larger data sets or exploring maybe how programming can fit, fit into this, you know, how to use programming to clean data or analyse and present it. Or explore, how it relates to other things such as machine learning and artificial intelligence. And as mentioned earlier by, I think Rebecca, I think it's really important aspect of data science, shouldn't be overlooked. Is that kind of impact on society and its responsible use making people aware that the data is being collected by a bunch of companies and and it might be providing insight sometimes with the benefit to you. So if you've got like a step counter on your smart watch or a mobile phone, you know, it provides you with insights, which is great because that's going to help your Fitness levels for what about when those insights aren't for you, you know, like a social media company who collect data about their users and how are they analysing the data? And and he's actually going to benefits from the insights is providing. In my opinion, given all of that. I think the grounding for data science, definitely lies within within Computing, but I think what's been touched on by Simon, it's it's not just it's not just a remit of computer science. You know, for example, how much data would a geographer or a scientist work with on a daily basis? What insight to a historical event can a historian provide if they were equipped with these data science skills. So, yes, I think it fits very comfortably with in Computing and shouldn't just be seen as a Computing skill alone.
Rebecca Franks:I read your article about data visualisations visualise data. And one of my absolute favourite is Genieve with "Ready salted code", where she took some data about storms that happen, and she turned it into a ballet to make such an amazing way to look at how storms do but for a ballet. So can you top that Ben? Can you top that with anything for me?
Ben Garside:I can't top it with any visualisations that But that is a really great example. Yeah, and I think.
James Robinson:Is this you dodging, doing some ballet on the
Ben Garside:Well, I think, you know, whether or not I don't think many of us would look at a spreadsheet full of data and like, get Buzz of excitement about what that data could be showing us. So I think that by the very nature of that sentence means that.
Rebecca Franks:James would by the way.
Ben Garside:But yeah, so I think we're trying to engage right? So that being said, the way I kind of like to introduce it is I would love to be able to show somebody like just raw data and then the visualisation of that data and get them to see the difference between them. I think that can be really powerful. So you talked about an example there, Rebecca. One of my favourite examples, which I mention in the article. This really famous visualisation it was created by a French engineer called Charles, Joseph Minyard. And I wrote this down it as in 1869 and he was his.. by trade he was known for creating information graphics by hand. That was way before any technology could produce any kind of fancy computer-generated visualisations, but what he did was took data known about Napoleon's, march on Russia in 1812 and turn that into visualisation. And I really like the idea of giving the students this data, this raw data about that and say, look what information, what can you get? Get any meaning from this raw data? And they might be able to say some things about it, but then you show them the visualisation and ask then to do the same thing."What meaning can you get from this?" And all of a sudden they can do more. I mean, from the raw data, you can see that you get an understanding that lots of troops are lost. Now I think if you already knew about Napoleon's March on Russia, I think you probably could have guessed that anyway, but when you look at the visualisation there that it's like a line graph kind of look, it's not a line graph, but there are two lines and one of them represents in one colour, the march on Moscow and the other line is different colour. And you know, it represents the retreat and you know, the thickness of the lines represents the size of the army. Like when you see that you think wow all of a sudden like the scale of the losses just becomes really clear. I think I think he set off is something like 40,000, no 400,000 troops and came back with 10,000 troops. Now, you know if I told you that you think that's quite a lot that they've lost. But when you see that visualised you really get like an understanding of that, you know, what scale that loss was and where it took place. And I've actually heard that visualisation being described as the, the Mona Lisa of visualisations because the you know, the more you look at it the more you see. You know there's temperatures on there showing just how much colder it got as they approached Moscow. There's geographical features on there showing, you know, towns cities rivers. And actually the river thing is really significant because you can see that on a certain day. They started with 50,000 troops. And then by the time, they cross the river, The Troop numbers dropped the 28,000. So showing that lot 22,000 men. So whilst the geographical coordinates are available in the data, you don't know where those rivers were until you see that, until you see that visualisation and understand how significant it is. What I like about that one alone is that visualisation tells a story. It's not just raw data on a sheet. It's not just an image, not in the situation. It's something that actually tells a story, and I also like the other visualisation in article as well, which is why I wrote about it by my man named John Snow. And if you're a fan of Game of Thrones, it's definitely not that Jon Snow. He did know something. So what he did is he mapped out Cholera deaths and something called a dot map. Essentially, what he did is he took a street map of the Soho area in London and he placed a dot at the location of where someone lived who had died of Cholera. What I really like about the visualisation is that a lot of people at the time thought that Cholera was caused by dirty polluted air in London, but what John Snow believed is that it was being caused by the water supply. So he wasn't creating the visualisation to discover this but he was actually using the visualisation to prove he was right. So the visualisation shows that the death was centred around this, pump on a street called Broad Street and where the local residents got their water from. So he got this he made into a visualisation and he took it to the local Council in order to convince them that this was the case. It proved the theory and as a result they shut down the water pump and you know, saved lives. I think both of those examples, I think, I find them really engaging I hope the students were too because it makes them realise the power of visualisation in comparison to just looking at raw data. The fact that, you know, you can tell a story, you can gain these insights into it. You and importantly, you can persuade people to take action, you know, ultimately, I think a visualisation can bring data to life.
James Robinson:That's a really great and very comprehensive Thank you, Ben. I think you talking there reminds me a little bit about how we started the show. Rebecca was talking about value and meaning, and sort of extracting value and meaning. I think that's a really key part of producing visualisations, is being able to convey that, that meaning tell that story and there's so many skills wrapped up here because you need students to be able to not only... If they're creating visualisations they need to understand the data. They need to think about all the different ways, there's some creativity within that kind of creating a visualisations. But then you also want them to be able to work with other people's visualisations and interpret that and understand, and draw conclusions from it. So I think these are really important skills that we are we're touching upon here. You mentioned the John Snow example, I am I right in thinking, that that's what part of one of the units in the Teach Computing Curriculum.
Ben Garside:You know, that's absolutely right. It is So, that unit starts off by talking about different visualisations, you know, getting them to understand that this it's not visualisations, you know don't have a particular form. I think if you were to say to, you know, student take data and make it visual. I think a lot of them might go right? Okay, I'm going to make a bar chart or a line chart or something like that. So I think what I want them to get an understanding of in that unit is the fact that visualisations can take pretty much any form, you know, you can present data. However, you like, as long as it does, those key things, you know, it brings data to life. It helps the audience to interpret it. And I guess, most importantly, it helps them gain an Insight that perhaps they wouldn't have been able to gain from just looking at that raw data. So yeah, I talked about the, you know, in that unit, we talked about John Snow with a dot, we also talked about the Minyard example. But I'd hope what they get from that is also understand that visualisations can take different forms, you know, understanding that, maybe even your sat-nav that shows your route from A to B, that's a visualisation. You know, the London, underground map is a visualisation.
James Robinson:And I guess in a way, I mean you could Right? Because I think if you're, you know, using a, taking some raw data and you're aggregating it, or you're summarising it. That in itself is a simple form of visualisation, it's not as pretty and as as creative, and as innovative as some of the visualisations that we're perhaps talking about, but I guess what's going on. There is it's it's abstracting some of the detail away from the data and bringing to the surface, the important relevant things and making that more accessible to to consumers of that data.
Rebecca Franks:I think the key is well, when we're looking learners. If... it's very different to look at a table compared to something amazing, like, you know, those those John Snow examples or the dance and things like that. It brings it to life and it makes it more appealing to our learners. It makes it more exciting. So I think moving towards sort of more of these sort of impressive visualisations is going to help our learners become more engaged with the materials.
Ben Garside:Yeah, and I think that you're right. I mean, I talked about two historical examples and you know, history interest me, but it might not All the students. So you're absolutely right Rebecca. It's about looking at this broad range of things. I mean visualisation don't also have to be these static things on a page, either still images and the website, you know, they might be animations or perhaps something that shows how something changes over time. Or it might be interactive where the audience, get to explore the data they want to. And you know, going into the the unit that's on there, there's a really good website called gapminder(.org). You can see how, you know countries have progressed throughout time so you can get students to pick something that interests them. For example, in that unit. I get them to think about where would be your best. Where would be the best country in the world to live and think about what would make that a good country. So they can come up with a country name, you know, might be Barbados or somewhere, fantastic like that. And I think on a rainy day like today, I definitely like to be in Barbados then getting them to think about, you know, what data is available to them. And how could you visualise that to prove your point? Prove the fact that Barbados is the best place to live and they can explore. Extra things like, you know, employment rates, life expectancy, how much crime there is in these places to see whether or not they were right, you know? And so in a way that interactive visualisation, something very visual it moves throughout times could show you what the country was like in 1900 compared to 2021. So, yeah, it's finding there's other ways that can, you know, appeal to them I supposed other than my historical examples.
James Robinson:I um. I was playing with a tool the other day out there like this, but it sucked half a day of my time because it was just really interesting. It's, a website that I've been using recently called anychart(.com) and there's loads of tools out there like this where you can program your visualisations using a JavaScript based sort of thing. You put your data in a data structure, you write some code and it uses some classes of the API to render some really interesting and engaging visualisations that are also interactive. And so that's a really great way of combining other areas of computing with the data science areas as well. So let's let's bring Simon back in now and ask both Ben and Simon: For those educators that are maybe Hearing this conversation that we're having and are slightly apprehensive or nervous. Where can they go to start to learn a bit more about data science, build their confidence. What are the first steps that Educators can take? Let's go to Simon first. Simon, what are your thoughts?
Simon Johnson:So obviously go to the National Centre earlier, there is a whole curriculum and series of lesson plans on data science. Definitely, check out the John Snow example, it's one of my favourites, too. The thing I like about that as well, is it also teaches computational thinking skills. So things like pattern recognition, see new trying to identify patterns to identify the problem. Also, decomposition, you're trying to break down the problem. It also introduces abstraction, so if you get access to some of the testimonies by some of the local people, then then does help that helps you to come to the conclusion that it's water that's causing the the Cholera. And by taking out the key points from all those Testimonies using abstraction. And then, of course, at the end you using algorithm design, to come up with a solution, I often make it, gamify it and come up with a competition to see who can come up with the best solution almost like Dragons Den pitch and get some interesting ideas. Like sealing off the entire city, definitely start with those resources.
Ben Garside:Also as well. Intel have created a free bank and the service over 70 lesson plans, cross-curricular that introduced the idea of computational thinking and data science and data analytics as well.
James Robinson:That's really good, some really good ideas And Ben, what did you want to add to that?
Ben Garside:Yeah. Well, I agree with Simon really don't Give it a go and really is don't let anything be a barrier to just give you a go in the classroom in the chances are you've already, you know, as you mentioned earlier, you know, data science encompasses so many areas of the Curriculum, the chances are you know, you look at the resources that are out there. You probably will be comfortable in delivering them. I mean the teach computer curriculum, for example, we got a whole unit on it as Simon mentioned, but you know, they also come with, you know, fully resourced and lesson plans which describe exactly what you need to do at what stage. So, you know, you feel comfortable about that too. I think data education Schools is a Project based up in Scotland funded by the Scottish government. Their website is definitely worth checking out because whilst we got some units on data science in the Teach Computing Curriculum. They focus specifically on data science and they have a full range of resources. I think from 5 to 16 so I'd highly recommend looking at their work. I mean, the most, obviously, the most recent edition of Hello World magazine has some really great lesson ideas in it and thought-provoking conversations around data science. And actually, if you're interested in exploring, like the programming side of data science there's a free open-source Python library called pandas and you can use that to prepare data as well as analyse it and visualise it. So there's lots of resources online for that but we've also got an article in which is got a really friendly beginners tutorial on how to get started with it. So I'd look at that. So yeah, there's some really good stuff out there basically, so my advice would be to just spend a little bit of time, looking at the what lessons there are out there, any tutorials, but just don't be afraid to just give it a a go with your classes.
Rebecca Franks:So there's been a lot of talk recently about the Raspberry Pi Foundation has just released a report on this giving guidance to educators about it. How do you think we can make data science, more culturally, responsive and relevant to our Learners.
Ben Garside:So I think as a teacher of data science, I'd them realise that this isn't just like a theoretical skill that they learned in the lesson and they're never going to use again, which is of course, the case with all of our lessons, but I'd want students to think about how they can apply their skills to bring about change on something that matters to them in their lives. And this kind of goes back to what Simon was talking about, you know, the culturally relevant curriculum. You know, I don't think it should be or arguably, it definitely shouldn't be something that's decided by the teacher. But, you know, it's moving them onto a project, like I say, that provides something that's relevant to them. They feel that they can use those skills to make a change, you know, for example, you know, let's say for in school litter, really bothered me. So rather going to head teacher and saying, I think there's a problem and what you gonna do about it, I could actually go and gather some data about where the litter is, where the bins are. What type of litter is being dropped and maybe if I visualise that and analyse the data I could provide that might provide me with insight such as maybe there was lots of little being dropped in the languages corridor and it turns out there was no bin within 50 meters of that area. Now. what I can do is I can I can highlight the issue to the Head teacher, but rather just be a moan. I can make a suggestion on how things can be improved and the great thing is all backed up by data. So it's not my opinion anymore. So I'm sure many teachers, listening to, this might think."Well, my students aren't that interested in litter but the principle of that idea of them, being able to investigate something like this. But actually something that's relevant to them. What you're doing is you're empowering them to use their data science skills, that you've taught them, to go gain a better understanding about something that matters to them. Maybe something that makes a difference that they could use to hopefully bring about change and that might be something simple. Like, you know, how much water they consume in the week or something, because they think they might need to drink more water. They can investigate that they might look at their mobile phone usage and what apps they're using what time of the day and, you know, they that might bring about change in their own lives. But they might also look at local data sets, you know, something that's, you know, traffic in their local area. There's so many possibilities that you can customise their learning to something that matters to them, something that really interests them. And that doesn't have to be the same for every student in the classroom. It might be completely tailored and individualised to whatever like I say, whatever matters to them really.
James Robinson:That's great. I think that relates back to started with Simon talking about context. And you know, I think when we are selecting context for our learners, I think it's really important that you explore more things from the culturally relevant guidance is, is understanding your learner's, you know, knowing what they're interested in and being able to kind of guide them towards things that interest and motivate them. Well, thank you very much. Both of you. We asked you our audience for your thoughts via social media on what data science looks like in your setting. What do students learn about and how do you introduce them to it?
Rebecca Franks:Yeah, so miss Ms Dennig has said at their visualising data sets within open processing and statistical analysis with R. I'm not sure what that is, but it'd be interesting to find out. They also have a dedicated extracurricular course in the topic. Ms Dennig says that these measures have supported students entry into both apprenticeships and university courses in the area.
James Robinson:That's fantastic R is, it is it's a language of been able to Tinker with. It's specifically for doing data manipulation. So Shasi Krishna who's a regular contributor to the podcast and talks about their experience at an international Baccalaureate level or IB and they've been looking into genetic algorithms this year and has also been using data studio to extrapolate knowledge and meaning from the raw, unstructured data sets. It is very much ongoing work, but I think it sounds like a really exciting and interesting route.
Rebecca Franks:Yeah, Matthew Wimpenny-Smith says how data is to it, so it could be as simple as labelling it and then displaying in a meaningful way that is accessible to its intended audience or such as info graphics or simple chart how to collect organise and read data, so that you can be understood in its context.
James Robinson:And I'm really grateful to Cat, Lamin for So some sort of younger Learners, I think, for her personal view is that she finds it quite a tough area of the curriculum for her. But I think a recent realisation has been there isn't just sort of death by Excel and boring spreadsheets. There is a lot of value in this area, but for primary there is a lot of focus on recognising the value of data, how it's used to inform decisions. And there's they do a lot of work on on collecting and manipulating data and understanding how that works. I think, in in that regard is quite linked to the maths curriculum as well. My thanks to Simon Johnson and Ben Garside for sharing their expertise with us today. And also sharing the experience of teaching data science. You can read their articles on this topic issue issue 16 of Hello World magazine. If you have a question for us or a comment about our discussion today and you can email via podcast@helloworld.cc, or you can tweet us @helloworld_edu. So Rebecca, what did we learn today?
Rebecca Franks:Well, what I've learnt what R is now? So maybe I can go investigate investigate that further, and I've also learned quite a few more ideas for data visualisation, which is really useful and I definitely want to read that book that you mentioned at the beginning called Information is Beautiful. So I think that's going to be added to my reading list.
James Robinson:And on my part. I mean, apart from knowing Thrones. I am really interested to go and find out a bit more about his early work in data visualisation.