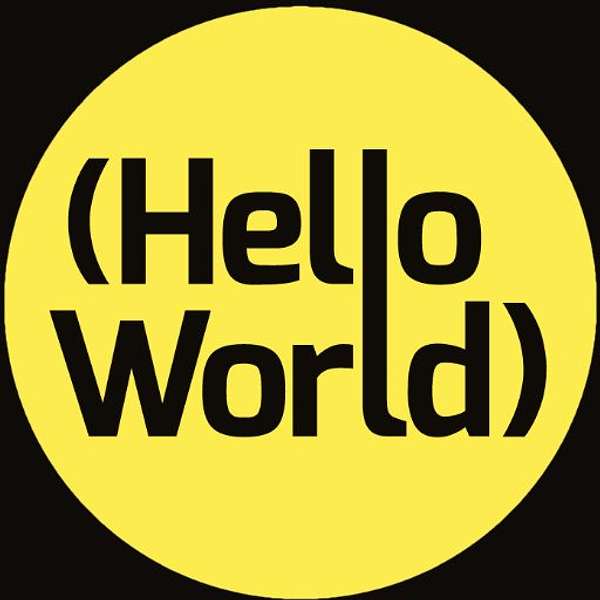
Hello World
Are you an educator who’s passionate about computing and digital making? If so, this is the podcast for you!
Join hosts from the Raspberry Pi Foundation as we chat with teachers, researchers, and learners from around the world to discuss the latest research, debate the big questions, and provide practical tips to help you engage and educate young people in computing. We’ll be releasing a new podcast series to coincide with each new issue of the Hello World Magazine to continue the conversation.
We’d love to hear from you! As we trial new episode formats, welcome additional hosts, and endeavour to produce regular episodes, we’d really appreciate your honest feedback about what you find informative, engaging, and, most importantly, helpful. Drop us an email on podcast@helloworld.cc and subscribe to the Hello World Magazine for free at http://helloworld.cc
Hello World
How can AI help us tackle climate change?
This week we're exploring the impact of artificial intelligence (AI) on the global challenge of climate change. The relationship between these two topics is complex as, like any digital technology, AI consumes energy and has potential negative consequences for the climate. However, its judicious application can drive all sorts of insights and innovation that help us combat climate change. It is not, however, an instant solution that can fix all the challenges we face.
Full show notes:
https://helloworld.raspberrypi.org/articles/how-can-ai-help-us-tackle-climate-change
AI's an incredibly powerful tool and it can be applied to challenges across many sectors.
Ben Garside:Do you think you have to be a coder to work in AI?
Marla Fuchs:A diverse team will get you the best results.
Ben Garside:I think a lot of young people might see AI as being something quite cool.
James Robinson:I identify as code adjacent. Welcome back to Hello World a podcast for educators, interested in Computing and digital making. I'm James Robinson, Computing educator and professional development Pioneer.
Ben Garside:Hello. I'm Ben Garside a learning manager at the Raspberry Pi Foundation, a former Computing teacher and master of multiple browser tabs currently proudly standing on 11 tabs open James, and it's only Monday.
James Robinson:That's an achievement Ben!
Ben Garside:It will only get more. As ever we really value your comments and feedback which you can share at helloworld.cc/podcastfeedback.
James Robinson:This week, We're exploring the topic of artificial intelligence and its relationship with our climate emergency. Now our listeners, may not see an immediate connection between AI and the climate. So Ben, can you elaborate a little for us?
Ben Garside:Yes. So I see AI primarily as a technology that is helping us solve problems and what really Powers AI or more specifically machine learning models is data and lots and lots of it. But luckily, we live in a society where vast quantities of data being generated. So, I see that AI can use that data to help us solve climate problems. That data could be gathered from things like; multiple weather stations across the globe; satellites above the Earth; or maybe even smart devices in our own homes that could show us how to save money on our energy bills and in turn, reduce our carbon footprint. It could be used to find ways to improve renewable energy technology like making our solar panels more efficient. But what I'm also slightly worried about is and I'm really hoping our guests today can reduce my fears on this one is that I think there's a significant environmental cost of running machine Learning models in Big Data Centres that all require limited resources to run such as massive amounts of electricity or water. But James as an enthusiastic about AI and saving the planet I'm really excited to hear more from our experts today who are hopefully going to make us feel very positive about the future.
James Robinson:That's really interesting, Ben, I think like so many of the things that we talk about both within Computing but also on this podcast, quite regularly, Is that any technological developments They come with many benefits and many drawbacks. And so it's not as simple and clear cut as saying well this is a good thing or a bad thing. We have to take the benefits and the drawbacks and we have to weigh them quite carefully to think about the impact that technology is going to have. Well, I'm pleased to say that you don't have to rely on me and Ben to help explore and solve some of these challenges we're joined today by two fantastic guests. So let me first welcome Sims Witherspoon, who's co-founder of the Centre for AI& Climate also known as CAIC and Sims is also Product Manager, working on the application of artificial intelligence to solve challenges that drive climate change. So Sims, first question for you really have how have Ben and I done at capturing the positive and negative impacts of AI on the climate, you know, and what more could you add?
Sims Witherspoon:I mean you've done a great job James but there is, I can add some more detail. I think that one of the things that I think about it as as we start off, is that AI is an incredibly powerful tool and it can be applied to challenges across many sectors. You know I'm sure we'll get to specifics in a bit and I really appreciate that Ben started with data because it all does start there. But I think if we zoom out, the way I really like to capture the positive impacts of AI on climate is to kind of present the opportunity in three parts. So, the first one being that AI can help us understand the problems we face and the impact on our ecosystems, you know, it can help us model the effects of climate change and also develop solutions to mitigate those effects which you know Ben mentioned earlier. It can help us also optimise current systems, you know, we can't just start everything over from scratch today. We need life to continue and we need to develop and have software only solutions, that help us run current systems and infrastructure. And AI is one of these Solutions, you can think about, you know, reduction of energy consumption. It can help us optimise the way we use energy, which has a carbon footprint. And then I would say the third bucket behind helping us understand the problems we face, helping us optimise current systems is maybe what most people think of, when they think of AI, which is accelerating breakthrough technology, you know, AI can help us develop these new technologies that will help us reduce our impact on climate, for instance, you know, new energy sources, like fusion. And I think those three aspects of AI helping us to understand to optimise and also to accelerate breakthrough science through and through across climate science, across mitigation, across adaptation. Even if we're drilling down into individual sectors and thinking about, you know, electricity system for agriculture or transport. So I really like thinking about it in that framing because I think it shows how just generalisable and how AI is really a multi-use tool. Now obviously, you know, I would be remiss to skip over the possible negative impacts here, as you'll have duly mentioned. And I think that one of the ones I see actually is techno solutionism, you know AI is not a silver bullet. It's not going to solve all of these problems for us on its own. It's a tool that we need to use and a tool that many individuals need to use in concert. You know, we need to be talking to the communities that are going to build these tools to actually make sure that we're building something useful that they want that will get used because, you know, technology is not really impactful just on its own, it needs to be deployed and used in order to have impact, you know, we talked to academics and scientists who are really the domain experts in this space to understand where we are applying AI and, and how to keep those systems safe and how to build them responsibly. You know, we need to understand from Regulators, what the regulatory constraints are going to be what they're concerned about as policy makers, and individuals, kind trying to look out for the for their constituencies. And I mean even businesses you know you hear all this information around you to Green, Green capitalism. And what we need to do to leverage capitalist incentive structures to make sure that economically all of these pieces you know, fit together. And so it really becomes this, this intricate like really multi-faceted conversation with many different players who need to be working together. And so techno-solutionism I think is a particularly dangerous aspect of the conversation but also you know like most powerful tools AI can, it can do harm as well as good, right? It can be used to develop new technologies that will actually increase our impact on the climate rather than help mitigate or adapt or address loss and damage. So, again it goes back to being safe and responsible. And I think just the last thing that I'll mention around the negative aspects is that it does require compute which requires energy and given energy is not carbon-free yet. You know, as long as that remains the case until our grids are running on carbon-free energy 24/7, there will be Associated emissions with any technology that uses energy AI included. So we can think about addressing that in a couple of ways starting with decarbonising the grid and also just being really responsible and how we are deploying AI how we're training models, how we're running inference and, and how we are being responsible about experimentation as well.
James Robinson:Thank you Sims. Ben, do you want to introduce our second guest?
Ben Garside:Also, joining us is Marla. Marla is an Executive Program Director for The Institute of Computing for Climate Science (ICCS), based at the Department of Applied Mathematics and Theoretical Physics within the University of Cambridge. So, welcome Marla.
Marla Fuchs:Hello.
Ben Garside:And so Marla. What do you think? How are they, how do the positive and negative impacts of using AI for climate action weigh up?
Marla Fuchs:I think you guys have covered a lot in the last couple of minutes, and I mean, I think obviously in the work that I do, I think that the positives outweigh the negatives. And I think that was kind of the resounding course that everybody is coming together with. But I think it's there, one positive that I don't think was stressed very much yet was using data to inform policy, and I think that's a, that's a really powerful tool and one that we haven't exhausted yet. So, and I think that that can really make a difference generally speaking in the global South especially, I think that that's something that well International policy, but affecting the global South, the people most affected by climate change and then negatively, I think, I something what, you know, there were a lot of negatives that were brought up, but one of one of them that wasn't brought up, was unintended bias. And so again, that's something that's I think we need to be cognisant of as we move forward with AI and it's starting to get a little bit more understanding, but I think it's something that's really important and I think also just that you know, AI is getting better but there still isn't necessarily creativity within it and so what you ask AI. So again if we kind of go back to kind of a policy space and we think about, if you ask AI to identify policies that reduce carbon emissions in London to nearly zero, it would find one when we were all locked down the carbon emissions in London bottomed out, they were really low. That was really, really great for the environment. However, what it doesn't consider is that in that identification, there are a lot of other costs that that took place, that didn't that we didn't ask AI about. And so, I think that we just have to be careful. You know, oftentimes we're if we're asking the right question.
James Robinson:I think that comes back to, there was something you both mentioned there in your answers, which is this sort of that actually the technology is just one aspect of this. You know, we talked about policy, we talked about the adoption and the awareness and the understanding by Society at large and by governments and so on. And I think, you know, and within your three buckets of things there Sims you were talking about the one that's probably most or best understood or expected of AI. Is this sort of creating and developing and helping us develop new Solutions whereas actually that's that's just one strand of this. So I thought that was really interesting that there is a real human societal kind of roll going on here. Yeah I found it very interesting. Ben, did you want to, you want to come in with a question?
Ben Garside:Yeah. Marla, I'd like to explore that unintended bias a little bit more if that's possible because I think when I think of unintended bias, I often think of things that are directly might affect people around us such as facial recognition systems, or maybe I might be biased in recruitment because of AI. How does that relate to climate science?
Marla Fuchs:Well I think it relates to all science. You come with your data set and with your, with your background, you come with bias. And and so I was actually just had a talk and I can get you a reference for the academic who's giving the talk later, but I just went to talk last week where they were looking at data images, a data science with images. And if you do a Google search for a jay, most of the jays that come up are the North American Blue Jay by a huge huge percentage, right? The world does not consist mostly of North Americans Blue Jays. So, you know, your data set is extremely biased. If you're going to then try and build something off of that. And so I think that's just a really for me that was a really good way of showing that the data that's out there and the data that's being harvested, is is really biased, even if we don't really think about it being that way.
James Robinson:Maybe a question. Maybe we could sort of drill in to data a little bit more as well. Because I think both of you kind of mentioned the the importance that data plays in any work involving machine learning AI in this space, the quality and the quantity of data that we're working with is crucial. So what what is the role of data in all of this? What kinds of data do we need to be exploring in order to help us tackle the climate crisis? Where do we source that data? And how do we ensure that it's of good quality and we're removing some of those unintended biases?
Sims Witherspoon:Yeah so I think that with with data one of the things that we think about in AI for climate is that there's a massive Gap in climate critical data sets. A lot of the information is siloed, it's owned by entities that, you know, for good reasons need to keep its access constraints, really strict, but I think for researchers, if there was a way to get access to that data in a safe responsible way, there is a lot that we could do with it. So there's there's actually a wonderful paper that was written by climate change AI available on climatechange.ai CCAI's website that lists climate critical data sets and they have encouraged individuals or entities that own these data sets, which if we had access to, would unblock critical obstacles in research and Innovation for applying AI to climate, to kind of share those and make them available for for researchers. So I think quality or sorry access is one of them. Quality is really the next thing, you know, to the point that Marla made earlier, once you get a data set, you're not ready just to you know plug and play with it. You really have to work with domain experts to make sure it's representative of the actual system or space or whatever problem that you're that, you're diving into. And you also have to make sure that you've actually collected all of the data you need to solve a certain problem, right? Because what we've seen sometimes is when we go in and to the real world and we are defining what we call an objective function. So something you want the AI to do, a goal let's say, we will be talking with Partners who haven't actually started collecting or trending or archiving the data streams they need in order to you know, eat meet that goal or to in order to understand whether or not they are pulling the right levers in order to reach that goal. And so we actually say there's a ton of leg work be done on data before you're even ready to engage AI starting with figuring out what it is you want to do with it. Making sure you have the data you need to do that thing. Making sure it's of a quality standard and a cleanliness that allows you to work with it in a responsible way. And so I really think of data as like the homework before you start diving into into the problems.
Marla Fuchs:I think that's this is where interdisciplinarity comes in and and so I think you can remove some of those biases or reveal some of those biases, by having interdisciplinary teams. So you make sure that you have people from different backgrounds, different geographies on those teams, but you also have different expertise. So that's so the work that we're doing is getting climate scientists and data scientists to work together, which is interdisciplinary. And now, to a huge portion of the world, those two disciplines sound really, really close together, but, you know, they're not and they don't think of themselves as even cousins. They think of themselves as very, very distantly related. So that getting having those conversations and having those collaborations is also I think the way to improve that.
James Robinson:What are the kind of data sets that we're talking about here? And I think, whether that is you know the data that we currently already have access to that we might want to be exploring or Sims you mentioned the kind of critical data sets that maybe we have we have challenges around accessing. What is that kind of data that we, you know, we want to be exploring. I mean, it might not be that it's just like there's One answer to that, there's probably many, many, many different data sets, but what are the kind of things that we are that kind of data that we're manipulating here to achieve some of these outcomes?
Marla Fuchs:Yeah, I mean, just even within our small program within once, you know, one of the four teams that we're supporting within the Virtual Earth Research Institute, they're just looking at Sea ice and how it moves. So that, I mean, that's, you know, there are a lot of people working just on that within our program. So the data set, you know, the data that they're looking at is very complicated.
James Robinson:Yeah no that sounds intres.. really Interesting, it'd be really nice to kind of have a play around with some of this data. And Ben maybe we should, oh sorry Sims go for it.
Sims Witherspoon:Well I was I was gonna offer because I think my original answer was quite overarching so maybe one specific area would be useful to dive into as an example because there are lots of overarching datasets that are important to have you know, I mentioned satellite imagery and things like that but let's just say we're solving for some some challenge related to building in cities, you can imagine that some of the data sets that you will need, would be, lets say a city level energy consumption, the emissions data, building informations that are part of the building information that is part of the built environment, the thermal dynamics of any of those buildings, the building, you know, energy consumption levels, and their Energy Efficiency. So you can see when you start getting down into specific, problem areas, the datasets that they change in that exact instance, it's really the datasets I gave would help you optimise buildings and do some urban planning work and what really falls into probably like the future of cities or energy optimisation. But this is where, you know, you really need to be working with domain experts to a point that Marla made earlier is that as machine learning researchers or as data scientists like they're not, we're not domain experts, we need to be working with those domain experts, so we understand what are the important data sets to solve whatever challenge We're trying to apply AI too. And I think that this is to the point of the interdisciplinary collaborations. It's absolutely critical to the success of building these systems.
Ben Garside:So I thought that was really interesting and what you're trying to get across there was really that you don't necessarily have to be a computer scientist or even a data scientist to be interested in, you know, getting involved with AI in climate science. So what kind of advice would you give to young people? I think a lot of young people might see AI as being some quite cool I think they might hopefully feel very passionate about wanting to improve their Futures. So what kind of advice would you give to young people who want to explore a career in this area?
Marla Fuchs:So I always think that we do our best work when we're next to our passions. And so I think that finding a project that is focused on your passions. For instance, there's there are a lot of really cool citizen science portals that are where you click about penguins and you you know, there're photos of penguin, loads loads of photos of penguins that scientists need help Identifying, because penguins look a lot like rocks and, and that's really difficult for AI to distinguish. So, you know, that's something that takes its, you know, get kids involved with that, you can have zero attention span, you can do one photo, you can do 100 photos, you can do it anytime you want. They always need help identifying Penguins, but so, that's, that's one that I particularly like. Staying on with birds the RSPB does the Big Garden Birdwatch each year where they really drum up support and they'll send you out a little packet with the tick sheet and you can get kids, you know, 2, 3 years old doing that all the way up to teenagers. And it's just, I think it's an hour, maybe half an hour it's not very long. You sit in the garden and you, you identify the most common Birds. Think there might also be one around butterflies. Anyway, those kinds of things, are fantastic. I think also learning code it at any age is really fantastic. I was lucky enough to learn code in the 80s. On a Apple Mac, which was, you know, the size, the big old TV kind of Apple Mac, and I don't think there's anything that really makes you understand the feet of working code, better than sitting and watching your own code not work. And so I think that, even if someone weren't to carry on with code if that defeats them, it really teaches you something about code to try and do it yourself, and it can be something really simple. It can be something more complicated and I think that having that experience, just demonstrates to you what you can do, or how difficult it is to do some things, but how powerful it can be. So yeah, I I'm I'm a big fan of getting involved and just trying things. And and also, you know, things within your community are great and at least in Cambridge. And I don't know about the rest of the world at the moment but here there's a lot of different CoderDojo kind of things that you can. You can turn up to at libraries and loads of free places online or places where if you don't have a computer you can go and they're always really keen people to teach code.
Ben Garside:Can we play games of penguin or Rock at these CoderDojos?
James Robinson:Yeah, I think so. That's that's that's my next CoderDojo planned, right and we think we can all relate to that code failure experience, right? The frustration. And the enjoyment of of broken code.
Ben Garside:Yeah when you said you had the joy of learning code in the 80s.
Marla Fuchs:In the 80s if you succeeded with your code, if you got the turtle to draw a Star, then you could play a game called Oregon Trail, which is something only Americans from the 80s will probably appreciate. But they will appreciate it very deeply.
James Robinson:Before we go to Sims what kind of what languages and sort of tools do you use now in your role, what are the kind of things that are really beneficial to the work that you do?
Marla Fuchs:Oh well I mean I grew up on C++ but that's not really what the teams are using nowadays, they're using Python and Scratch. And yeah so but I think the most important thing is learning any language to start with. I think it doesn't matter what language is if you learn a language or even if you're using Minecraft, and some kind of modifications that you can do with that. I think just getting involved is the most important step.
Sims Witherspoon:And I would also add that, you know, it's never too late to get involved or to learn code. You know, Marla started super early, you know, in the eighties, I took my first. Well, I mean, I guess I studied JavaScript at 13 but I'm not sure that that really counts but I only started coding in Python at, you know, during graduate school which was a handful of years ago. So I started, you know, really recently and it's incredible how quickly you can pick it up if you're really interested and really dedicated to it. There's so many free resources as well. Whether that's, you know, online resources like Coursera or classes you can access from local universities that are just open or even there's so many computer scientists who are also really Keen to help develop the next generation of computer scientists. You can also kind of LinkedIn stalk and like, find people who can give you recommendations on where you can learn and it's not just basic, you know, CS as well. It's not just a basic computer science you can do machine learning. You can take a machine Learning course, you can take certification level courses online. Like you don't have to physically be somewhere you can go to a classroom to learn how to code. And I think one of things too about this. Both AI and climate is resilience is just so important. You know, it's Marla mentioned like we've all sat there and had the experience of watching our code fail or, you know, searching the hours for a bug. And I think that, that frustration is also something that a lot of climate scientists and, you know, product managers and folks who work in building applications for their real-world of this space. We, you know, we face that there are a lot of obstacles. These problems are intractable, they're incredibly complicated, they're very layered and I think that all of us have to have an amount of optimism but also a huge amount of resilience to just keep going, keep learning when the code fails, keep pushing, keep, you know, going if you hit an obstacle, find a way around it, build, build that data set you don't have, try to generate it, see if it works. And I think that that's something also that students can just keep in mind, you can start at any age, you know, doing whether it's in your free time or professionally and you just have to be curious and open and resilient.
Ben Garside:It's really interesting that you both mentioned code as part of your answers to working in a career related to AI do you think you have to be a coder to work in AI and because earlier on you mentioned policy and how important that was. Do any of the people that you work with or know of in your surroundings are they all coders or do they have other skills that are really valuable as well.
Sims Witherspoon:You absolutely do not need to be able to code to work in AI. There are so many important contributor contributors who have various skill sets, whether that is policy backgrounds, whether that's domain expertise, whether that's Operational Support, whether that's, you know, product management. There are so many different areas and so many groups of people that need to work together to build and deploy AI Solutions, you Absolutely do not need to be a computer scientist but that's my opinion. Marla?
Marla Fuchs:I agree a hundred percent. And I would actually say that it's really important that you have people, you know, the people who are cut out to code as their opus are different to people who are cut out to have other parts of that puzzle, right? And so, like you're asking me what I use for code, I don't code anything now, I talk to people who code all the time and that's their passion. It's not my passion. My passion is communicating and making things happen. And so I think, you know, you really need fantastic communicators and people who think across these disciplines to, to make an impact. And and so I think that yeah, being being code adjacent is not a problem.
Sims Witherspoon:I also identify as code adjacent, for the record, I do not code at all. I learned it. But I do not build AI applications. And hopefully my team still Finds Me useful.
Marla Fuchs:Yeah.
Ben Garside:But I think what's really important is that code adjacent is a really interesting term and I think it's probably the case for whether or not you're interested in coding or not. I think having an understanding of how these machine learning models work. You know almost like doing machine learning adjacent as well. I think it's really important, I guess.
Marla Fuchs:I think that's the case with all of us and that's why I mean, I think that really passion is the thing that's going to get you there. If you're not passionate about it, then your your stuck, aren't you? But I think that I believe very strongly that anybody who really learns about climate change, and the situation that we're in right now and nature will be passionate, and they just need to find out if they're passionate about sea ice or if they're passionate about you know, gravitational waves or you know, where there where their passion lies, but there's a huge Spectrum out there of things to be to get involved in and and there is definitely somebody looking at the thing that you're interested in for you to learn about.
Ben Garside:I think that nicely brings us on to our next question, which is something you mentioned Sims with the fact that young people need to have resilience but I think that's also I think young people can as we as adults do as well, can feel quite anxious about our futures to do with Climate. So bearing in mind, that there's often a lot of worrying news about climate change. How do you go about balancing the impact and with the opportunities and make people feel as though they can have an impact in their futures?
Sims Witherspoon:I think this is such a personal choice on how you deal with your own climate anxiety. I would say that for me individually, one of the things that I think about is what is within my sphere of control. What can I actually influence? Whether that is in my individual choices for how much I decide to get on a plane? What I decide to eat, how I spend my professional career. And I think that one of the things that is really important to keep in the Forefront of our minds. Is that if you are just working on a component of the challenge, try not to zoom out too far and worry about, but what happens next. But what happens next? And after that. It's more about making a start and making sure you are attempting a solution safely, responsibly something that feels just and fair for you. And those, those definitions will be different for any of us. I think one of the things that has helped me in combining AI and climate and to kind of understand how I want to think about the pros and the cons. And what's helped me deal with climate anxiety is that, you know, if we have an understanding of climate, we can help advise the next generation of scientists, you know, whether AI is even the right tool for a given Challenge and if it is how to approach it. And we can be a part of those Solutions and equally, you know, understanding AI can help young scholars really advise which are architecture's are used for the challenge as well as to develop the tools needed for those challenges and I think if we focus on the solutions we at least for me individually. I feel like I have a lever, a lever to effect real change. And yeah I think it's a really tough one though because there are really so many intractable complicated problems that I do think if you think about everything we have to do, all at once, it becomes incredibly overwhelming. So I choose to Zone in on components and try to do the best I can do with the skill set I have and and that of the people and experts working around me.
Marla Fuchs:So I guess for me, particularly with young people, I think that think Global and act locally. So I think it's great to get involved with things that are tangible as much as AI is great but when it comes to overall climate, I think that getting involved in something like a litter picking up thing at a park or, you know, the Garden Bird Watch. I think those kinds of things make you see and get out in nature, enjoy it. And I think that's a really important part of the being passionate about the climate is enjoying it. I don't think that you can spend all of your time worrying I think you have to make sure that you get out and see what's out there and also that's contagious because as soon as you know I've taken Children up mountains and seeing their faces when they first get to the top of the mountain is you know an Irreplaceable feeling and I think or seeing a waterfall. I think those kinds of things are really. They make you more committed and kind of give you that extra energy to keep going. I think in terms of especially for young children. I think there's kind of a level that needs to be set in terms of how much they they are told about the the or the way they're told about what's happening. I think that there's a lot, a lot of information out there and I think that we all need to have levels. And I think that for children as Educators and as parents, you have to set those levels. So I don't think that that's up to us to kind of make sure that that burden isn't too much for them too much too soon. But overall I think that knowing that we have an amazing amount of tools at our disposal and that actually a lot of the things that we know we need to tackle climate change. We've already figured out that we just need to deploy them and that that then's actually, we're now into the sales pitch part of solving climate change is actually really reassuring. And so I think there's there are a lot of positive stories out there too.
James Robinson:Great. And I think I mean my I was going to ask a little bit about engaging Learners and how we do that but I think we've spoken quite a lot already about kind of passion and how actually connecting young people with their local and the global kind of climate challenges is potentially quite motivating for them. And there's a way to kind of engage them in this topic. So just a more sort of slightly more specific question are there like for those students maybe that are less convinced or want some sort of idea of tangible benefits of us working in this space AI and climate are there particular examples of what the things that we can point out where we can say, here is this program that used AI? And here is the positive impact it had on the climate. Can we are there case studies that we can kind of share or talk about that spring to mind and maybe the answer is, no, I don't know. What are our thoughts on that?
Sims Witherspoon:Oh yeah, they're there are many many case studies on AI being used to benefit the climate. I mean I think if you think about climate science there are there's AI being used in our climate models, to make predictions about atmospheric physics, and weather so, we understand understand the effects of climate change and actually are able to help develop solutions to mitigate as well as adapt to those changes. In the energy sector, we are able to use AI to improve Energy Efficiency by predicting usage patterns and then optimising generation so they're less emissions. In agriculture there there are groups that are helping improve production by using, you know, AI and machine learning to boost crop yields and reduce water and fertiliser use. In transport there's examples, where AI is being used to improve efficiency and optimising routing and reducing emissions. I mean there are many many, many examples, and I think one of the really great resource that I would love to plug is climate change, CCAI's, climate change, AI's paper called, Tackling Climate Change with Machine Learning is really a fantastic resource and it's available on their website, climatechange.ai, and it lists loads of different applications across many different sectors, and I think it's a fantastic paper.
James Robinson:That's great Sims, thank you. We will definitely link out to that in the show notes. Marla, do you have any examples that you want to kind of cite or talk about?
Marla Fuchs:Yeah, I was just trying to think of a kind of more more visual learning examples for children. That's, I guess where I was at. I mean, I know academic ones for adults that I have been a part of particularly on saving water in India that was done with AI a project that I worked on there in agricultural use and that, you know, billions of litres of water have been saved and that's it. So, yeah, there are lots of really great academic examples. But for children, I was trying to think of more visual local examples like I guess more like home heating. Kind of examples that would be that would help them to understand and kind of home heating relating to money I think that would be something that kind of this age group could could do and even could do an experiment on kind between the summer and the winter. And they can see how much, how much energy their homes use in the summer and how much they use in the winter and or or temperature over the course of the year. And looking at it historically that's a, that would be something that they could see how AI is making those Trends. Because again, those Trends are really visual, right?
James Robinson:So we coming towards the end of the episode, but I think one final question that I have for both Sims and Marla is how important do you think that diversity within the workforce within the people working in AI is to the future of its development and its impact on the world?
Marla Fuchs:I think that we want the best possible teams working on this problem because it's so important, and data has shown time and again in the corporate world and every world that a diverse team will get you the best results. And so I really encourage everyone to get involved in this at any level. And sometimes that might mean that you're a minority in that group. I was a minority in my undergraduate degree, there were not very many women doing mechanical engineering at that time. And in the math department, still there, where I work now, there still aren't very many women but I think it's important that we have diversity across across the project.
Sims Witherspoon:Yet, I couldn't agree more. And I think diversity is also people from different backgrounds places around the world. We tend to see a lot of AI applications being built in, you know, these very developed countries with access to the compute and the technology and the big academic institutions, but it's actually really important that we have engagement from less developed countries particularly in the global South where a lot of the impact of climate change is going to be outsized for those communities. So whether it is diversity in gender or background, or religion, or culture, or where you're from, it's just important that in order to build systems for a global Community, we need representation of that Global community in the teams that are building the systems and in the teams that are reviewing and offering the data for those systems.
James Robinson:Well, thank you both. I think that's a really nice end to what I think's been a really interesting episode and I hope you know, I've certainly found the conversation interesting and I hope that our audience have to. So if you're listening and you have a question for us or a comment about our discussion today than you can email us at podcast@helloworld.cc or you can tweet us at HelloWorld_edu. My thanks to Marla and Sims today for sharing their experience and expertise we'll be back in two weeks time exploring more topics to do with Computing and digital making. So Ben, what did we learn today?
Ben Garside:Well, other than learning that I can probably outsmart AI when distinguishing between rocks and Penguins, for me, I've really enjoyed listening to ideas on how young people can get involved with areas of climate science that they're really interested in. No matter how small they think that problem is no matter what their background is, and just drill down and understand the impact that that the data can have on those kind of problems. So, it sounds as though a I could be a real driver of a change when it comes to climate action and that should make us all feel really positive about the future.
James Robinson:And I learned that I am I identify as code adjacent, there we are.