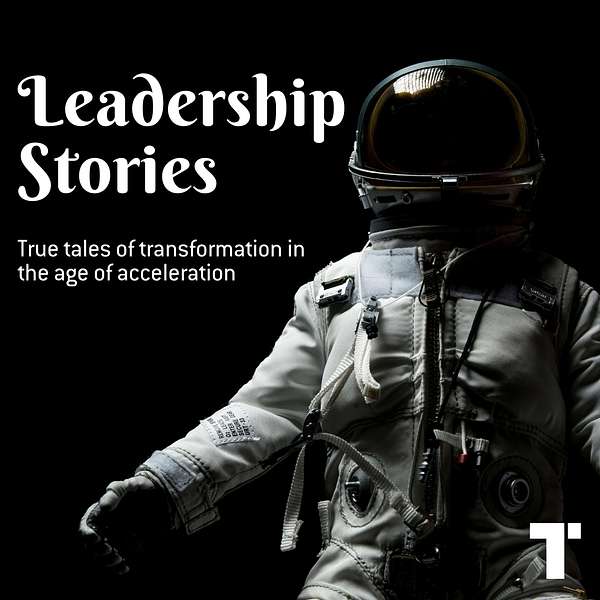
Leadership Stories
Leadership Stories
The Next Industrial Revolution with Craig Hayman, CEO of AVEVA
.One of the most fascinating events this year was the crisis in the Suez Canal, which I think in many ways was a reminder of not just how fragile our supply chain infrastructure is, but just how complex all the systems that run our world in fact are. Why is complexity becoming such a big issue now for business leaders in organizations?
Craig Hayman:Mike, the most amazing thing about complexity is when it works, it's, no one really notices. it's when something goes wrong across these disparate systems as we saw in the Suez canal, whether that was the wind or the engine power or the human talent there. When they don't all come together then things can go wrong pretty rapidly. I think about this as a, the data, the siloed systems, the network of decisions that really every day make these industrial problems go away. When they don't, they're right, right in our face.
Mike Walsh:I'm talking today with Craig Hayman who's the CEO of AVEVA. And AVEVA is a really interesting company. And it's one that I think if anything has become... its mission in the world has become more important as a result of the pandemic, but just for everyone else, could you give us a little bit of an explanation of of what AVEVA is and really what its mission is?
Craig Hayman:Yeah. AVEVA is a company spun out of Cambridge University in the UK, and we really are focused on the industrial sector. So this is the companies that produce the staples of our lives, the food and medicine and energy. And what happens is this sector is seeing an accelerated transition to digital. About five years of innovation has been crammed into the last 10 months. And so really what's happening is we're starting to see these very open, these very agnostic systems that you are able to work together. You don't have the time to rip and replace them as we've seen in the middle of the pandemic. So what AVEVA does is we provide software that works across these different systems in an agnostic way, you click our software in there and in a few weeks you see something or learn something that you didn't know. As an example, in Southeast Asia as a chemical company, SCG Chemicals, and they want to increase reliability so they bring together all of this data across these different systems and they put that in hands of their workforce. And so that, that... it's complicated to build petrochemicals. And so this helps those who've tasked with that very difficult job, do that. And what it does, it lets you build a model that you can then share with other companies in the petrochemical space. Here's another example EDF, which is a power company based in Europe. We've saved them over a hundred million pounds by identifying a failure before it occurred to help them optimize what is a very important renewables operation.
Mike Walsh:I think that's important to remember because, when we often think about digital transformation, people tend to focus on the consumer internet. They think about digital channels or mobile apps and in many ways they underestimate just how, how critical the digital acceleration of the industrial sector is. It actually stands to be much bigger than the consumer internet doesn't it? What, why is this the case?
Craig Hayman:Yeah. Look you think about late in the 20th century there was a one of these revolutions around a sort of binary and digital and that digitalization created the tools we're using today, desktop productivity tools but digital transformation to retail, to financial services, to telecommunications. And so what you have here is this outline, this industrial sector, which is the[inaudible 00:04:31] the very last industry to use digital at scale. And so what we've discovered is, look, if you use this, the digital capability get the data that's there, visualize it and something amazing happens when you put data in front of people together, people look at it and say,"Oh, I didn't know that," you get a little bit of some insights, some shared insights. You agree and you say,"Oh, what do we do about that?" And then you galvanize actions, people gives them the power for them to affect change. And then they work about improving their business. Now that could be a big topic, important topic now about sustainability. It could be not just driving down the cost, but reducing the amount of power that you use or the amount of emission that you put back into the into the atmosphere. And so you give people the data, you give them the analytics and they can take waste out of it. Now, we think about this this idea of data and machine learning, which leads you to artificial intelligence, which is rev- revolutionary, transformational. But we think about that artificial intelligence in the hands of people to help them improve performance. And we call that performance intelligence. And performance intelligence is putting... taking the data, the AI and putting it in the hands of people. It's just a quick example. You might've started your day like, like me drinking a cup of coffee perhaps with some Starbucks beans. We deliver the software to help them support their sustainable coffee manufacturing vision. So you think about this coffee bean it's roasted based on the altitude, it was picked up uh, it was picked from based on the moisture content in it, and based on the taste that you want that we all want in our coffee. And we'll have very different tastes. So we allow them to give them automate the recipe of how to roast and give them this sort of shop floor to top floor visibility, all of the coffee bean the roasting process, and then into the operations business. I know it's a pretty... to get that level of consistency across 30,000 stores in 80 countries is no small task.
Mike Walsh:I think you often underestimate just how difficult something as basic as consistency is. And I didn't know about the Starbucks example. I'd actually heard that there was a very complex algorithm that Coca-Cola uses to even make its orange juice consistent because of the seasonal variety of the produce. And one thing that really intrigues me though, is that I don't think it's anything new about the importance of data in industrial manufacturing and processes. We've been doing time and motion studies for the last hundred years, but what is really difficult here is it seems for many years this data has been kept in very siloed, very proprietary systems, and it hasn't allowed you to do things that we've been able to do in the consumer internet, which is really scale up and connect these more complex ecosystems.
Craig Hayman:Yeah, look when Tim Berners-Lee began the work around what is now the internet that allowed us to connect different systems together so that we as consumers and originally scientists could navigate those different systems in a standard way. Now let's think and we've all benefited that from that in, in our lives. Now think about the industrial sector and you've got different companies manufacturing different products, some of which have got proprietary recipes or ingredients or manufacturing facilities, and they change hands quite frequently as companies move around market trends and tastes. So you end up with these over 20 years, 20 different systems all using different technologies. So in any given day, it's a real challenge to try to figure out how to make these things work together. Now what happened in, in our industry, and companies would say,"Look, hey, if you use my single system, buy my thing, your life will be better. My product is the best and it will connect to everything." It never happens. And in fact, there's nothing in human history to indicate that one thing would solve everything. So you say, really, I need to connect these disparate systems. And so that's what we do. We harvest data from your existing systems. We bring it together with analytics to give it to the team. And by the way, the data is our customer's data it's not ours. So this idea is back to the internet, we have the data in front of us and that can help us run our lives. Similarly, let's do that in an industrial sector. Let's put the data and the insight into the hands of the people who have these difficult jobs running their facilities. Also let's visualize it in creative ways like augmented reality another example, GlaxoSmithKline great example. Operational data management seems pretty dry together with our system platform to automate manufacturing, bringing plants and processes, informations together with analytics, again pretty dry, boosting efficiency rate, accelerate time to market, pretty dry. Except when you think about what they're manufacturing vaccines. And so when you're manufacturing vaccines, you wanna get the most out of these disparate systems. You have no time to rip it out and replace it. And so they're delivering for us back as consumers and we're thankful for that, but we're helping them leverage what is already there installed footprint around their manufacturing facility for vaccines.
Mike Walsh:Let's talk a little bit more about insights because there's really an open question now about what's gonna drive the next wave of productivity. And there is some, let's be honest concern that things like automation and artificial intelligence are really gonna be about ripping out costs and people and essentially finding ways of squeezing productivity out of human beings essentially through algorithmic management. But your argument is, I think is that the real next leap in productivity is gonna come from better insights and augmenting and empowering people.
Craig Hayman:Yeah, I think I think about this as industries where you've squeezed all of the productivity out you end up there's very few other places to go other than labor, reducing the labor requirement or trying to frankly squeeze the efficiency of labor capability. This is the markets that are heavily already digitalized we're already seeing parts of this. But in the industrial sector, there's very little It's a bit strange, actually. It's the first industry to to start using digital, but was almost a hobby for 20 years. So here's an example. You might have a car... a customer told me this example, you might have a car, it's your family car, and you drive it quite carefully. If you're a Formula One race car driver, which I'm not, you don't drive that car carefully. You you... I would imagine you drive the living daylights out of that thing. You drive it around and lap as hard as you can, and you just know when to bring it in to change the tires or whatever else it is. The difference is in, you don't drive your car like a Formula One car, because you want it to be reliable. What if you could drive it as hard and as fast as a Formula One car because you had better insight into it? And that's what we do for equipment. We allow our customers to run them as hard and fast as they need so that they then know when it's gonna fail before it's gonna fail for maximum performance. And you take that information and you put it in hands of the operator, the person who's in charge of that piece of equipment. They're still in charge of the same piece of equipment. They're still driving. They still have bring their skill and capability, but we're giving them more information. We're giving them the dashboard. We've seen this at companies like[inaudible 00:12:52] at SCG Chemical, at EDF. And this is this AI, artificial intelligence. Back... a little bit back to my car point. I remember when maybe 10 years ago you got in a car and you get this check engine light and you take it to the dealership and there will be nothing wrong with it, but it costs you a hundred dollars, a hundred pounds to, to check it out. That's a false positive. So that's an early sign of technology. Most cars are better than that now. In the industrial sector, there were a few years ago, a lot of false positives. People were saying, I think that's a problem and the people who knew better said, actually there is not. But the data, the information has gotten better. There's more information, and the analytics have gotten better. And and so now these false positives have been reduced dramatically. If you happen to go in a new big city and you go to one of these Starbucks roastery, so that you can see the coffee being roasted, you'll see our software in there manufacturing that safely, or a vessel going through the Suez canal. We manufacture... our software manufactures these massive vessels in the ship yards, like the building blocks. It's crazy. They're flipping and turning the large steel plates with floors attached to it like they're blocks. And the precision is incredible of how they slot together aircraft level precision. This is just an example of putting that data in the right place.
Mike Walsh:I think it makes sense intuitively that the scale of the things that you're manufacturing or producing at the industrial level requires a whole new set of data-driven capabilities. What to me is a little bit more complex is how that changes us potentially. And what are the new skills and capabilities required by individual leaders and team members at these next-generation industrial organizations that you described. This surely is more than just everyone going and trying to learn TensorFlow or learn artificial intelligence. What... for everyone, what do you see as the sort of the shift in the cognitive stack that I guess we all need?
Craig Hayman:Yeah. We've seen the creation of new jobs, new roles, like chief digital officers, or we've seen of data scientists. And we're seeing those roles being applied to really complicated tasks like the energy transition. Building a self-driving car is a very difficult challenge, but actually the challenge that the data scientists at BP have to support the energy transition and to support delivering energy for the world throughout a transition, it's this combination of bringing geophysics experts together with software from uh, AVEVA in this case together with the data science. That's a new skill. The domain is still the same. You still have to understand energy, but you're applying it in new ways. Another example is,
Mike Walsh:Let's talk about that for a second though, Craig. So I find that really interesting'cause you essentially, what BP I guess realized is that they had lots of people with math skills and very deep domain experience they just were missing I guess a small piece of knowledge which allowed them to connect that to the next generation systems.
Craig Hayman:Yeah, exactly right. The domain is the same but the challenge is is, has been reframed. So let's think about green hydrogen. So green hydrogen is really important. Hydrogen is very good energy source for us the world here, and green, it's called green hy- hydrogen comes from essentially water H- H2O you apply energy into it and you get the hydrogen out of it simply, that's, and so you need the energy to come from somewhere. So when you take that energy from a solar farm or a wind farm, or from the the tide that becomes and apply that into the water you get hydrogen, and that's a green hydrogen project. It's quite difficult. It's quite complex to do that safely and at scale. As the it's green energy is a little bit intermittent and it literally goes up and down based on the sun and the wind. So how do you build one of those facilities that perhaps could float? How could you build one of those facilities? How do you design it? So we are helping companies like Technip Energies in France do exactly this, design it, construct it, deliver it and help them operate it. It could be as simple as a piece of that facility called a flare and reducing the size by about a third to save the equipment and materials and construction. I just my final example, the one I like as a Duke Energy. They're in the U.S. and they have a difficult challenge. They produce energy and they have these big turbines that produce energy. And we detected that one of these that we're looking at the data, not just from the turbine and everything else around was that something was amiss. Similar to getting in your car and you go, it sounds a little bit different today, but we're looking at hundreds of data points around this turbine. And we said to Duke"Hey, there's something wrong with it. It's probably gonna fail within this timeframe." They called in the manufacturer, and the manufacturer said,"There's nothing wrong with the turbine." because we were looking at more data than just the turbine itself, and we said no, it's really going to fail." So they stopped people. You don't just turn these things on and off. They took it down to a low utilization level prevented human access to it so that it was safe and then took it out at the next window. They found loose aluminum parts inside of it, and helped them save$34 million in what could have been a catastrophic failure. They won the award for some of the work they're doing in taking data to prevent these types of failures.
Mike Walsh:When you think about the complexity, once again that, that runs these systems, it's no surprise that there's an emerging industrial technological stack I think here that's much less proprietary. It's more driven by the cloud. It's AI powered, it's... And I think critically it's connected to new ways of working. And if anything, the pandemic has accelerated in the last 18 months that, that shift to not only using data in new ways, but collaborating, making decisions with the other humans in your organization side by side with more automated systems. I think you've got potentially a unique viewpoint on this when you look at your customers. So what have you seen in terms of the future of work and how that's been driven by the emergence of new data and and smarter machines essentially?
Craig Hayman:One of the great things we were talking about, the internet early. One of the great things is you get to consume a lot of these lessons that, that others have made before you by reading about them. So one of them is in Japan, the, what was originally the kanban approach which was, if you're running a manufacturing line don't ship a part or component to an assembly station until the assembly station needs it, it seems pretty straightforward. But that's a innovative idea. And these feed built back... bringing this feedback loop so that these systems that are disconnected are now connected. So now let's think about this more broadly, and think about data flowing through a manufacturing facility. And I think of data like the rain, it falls from the sky somewhere, and it falls onto a leaf perhaps, it flows through a river, into an ocean it has a flow to, it has a tide, it has a value. And if you look at that water in the stream, or in the[inaudible 00:21:26] in the ocean, you can figure out how you might fish or how you might put in a an energy, a way to capture the energy from that water flowing through this. This is the same way, the way that data flows through an industrial setting. If you look at that data, if you can look at it in its totality, you can understand its scope and its scale, and you can drive efficiencies. And again, it has to be open, it has to be agnostic. It's owned by the people running those environments. And now is the one example I think of they use data to drive their systems, right? They use data in the way that they run in energy production the same way that you would use it in a regular production of some sort of discrete product.
Mike Walsh:So what's sort the next step beyond this. Are we gonna see the emergence of a new kind of industrial internet that, that leads to a much more open networks data-driven ecosystem? Because even though we see with the work you're doing with many customers, there are now more open systems, we haven't really gone to that next stage of a well-defined semantic, industrial web yet.
Craig Hayman:Yeah. I think of I don't know if you remember when you first used the search maybe with Yahoo or something online you know you'd get a, you'd type something and you'd get a couple of some ca- human categorized list of places to go. And then you search and you get maybe you search for let's say George Washington, a famous figure, and you might get a a series of websites that have information about George Washington. And a Wikipedia page where all that knowledge has by a person or multiple people who have been stitched together to, to create knowledge out of that data. What we're seeing now is that knowledge being the graph of that knowledge being created automatically, if you are online now and you search George Washington, you'll see somewhere on your screen, a knowledge graph of that famous person and it's pretty good, it's pretty good. So this is a way of taking the data and allowing you to navigate that data, even though it comes from disparate sources. What we're focused on in AVEVA is building that knowledge graph for the industrial world, so that maybe you're constructing a ship and then later when you're operating that ship, and you're like,"Oh, I wonder what that is," you can then navigate to the design of that ship and understand the design constraints around that particular subsystem, or, and you can extend this into, across any type of asset. Maybe what's the safe operating envelope to, to operate that facility around it. We've done this in the middle of the pandemic, but one, one company, one customer of ours is ADNOC. They are a national oil company, produces energy and they created a digital twin of their entire operations. They did this in eight weeks. We snap in, we visualize all that data. I It's beautiful visualization. It just looks stunning. And they can now visualize bring their teams together and look through all of that data, hundreds and thousands of data points often in real time and they can make decisions. Financially we talked about productivity earlier. So the productivity benefit of this system for them, they are saving a hundred million dollars every month. That's over a billion dollars a year in economic value that's being created. It was always there in their systems but now by pulling that data out, that knowledge graph out and putting it in the hands of the people who can do something with it it's just a phenomenal productivity boost.
Mike Walsh:It makes sense when you think about the way we design and build things now, whether it's cargo ships or buildings it's increasingly a digital process. So you have these very sophisticated CAD files, but also these objects that contain information about the operating characteristics of those modules. But what you're talking about is something that's even more interesting, which is you can start to build it almost a template of what a highly efficient factory looks like, or what a a wind farm operating at its right capacity looks like, or what a jet engine, its tolerances look like, and then actually start to understand those interactions in the real world. In a sense, you're not just tracking the data, you're starting to build a blueprint of what success or failure looks like as well.
Craig Hayman:Let's just think about in a manufacturing facility you've got, or go back to kanban, Japan. You don't just have the machines, and you're trying to make sure those machines keep working. You apply some people who's in charge of improving how they work together. You, if you, whatever your favorite sport is, whether it's soccer or baseball or basketball, you have a coach and the coach is constantly tuning and advising the team how they can work better to increase their performance. I think this is where we have customers like Enel Energy or[inaudible 00:26:52]. They have these teams who are constantly advising the teams who are operating them to use analytics, to optimize the operation and reduce the sort of lost downtime, et cetera. I think where we go eventually is that these, some of these systems can be run autonomously, but for now you're helping the teams with around predictive maintenance. They can ask questions like when will this fail, and could I last until the next scheduled planned downtime so I could do, I don't have to do my repair now, I could actually wait and make sure I do that repair right now... I'm sorry when the downtime is as planned? What's the optimal time to perform the maintenance to... It's not, you'd be surprised some of the optimizations are that we find people would say of course that's obvious. And many of them, they say, yeah, we know about that we are already working on it. But often some of those insights for people like Enel are just astounding in how just a little tip, a little coaching advice to the teams there on the ground can really transform how people work in these facilities.
Mike Walsh:We spoke at a number of points in our conversation about sustainability, and I think this is a, probably one of the best examples of the challenges of complexity, because, as you mentioned, a lot of alternate energy sources can be intermittent, which creates incredible difficulties for trying to predict output and to plug those into more traditional manufacturing systems. So with this renewed focus on on, on that sustainable world, this is not something that has to happen necessarily at the expense of productivity and efficiency though. I know you've got some thoughts on this.
Craig Hayman:Yeah. It's big, a big topic. And frankly, there are some great books out there that certainly I've read recently. Bill Gates has a great book trying to think through energy consumption, energy production, energy storage, and you realize that digital has a huge role to play in driving enabling the sustainability. But I think, look we're in a time of transition here. It's really not the first time that we're thinking about how we're shifting from one energy type to another. We did this a hundred years ago as we shifted to, to oil or even before that, when we we shifted from whaling for oil for light. We, as a human species have shifted multiple times in, in how we consume energy and how we require energy. One of our customers in the UK National Grid just tipped over 50% of the energy that they produce and distribute is for, is from renewable energy sources. And we helped them manage that. Another of our customers Henkel you might use their products in your home. And so you can we're saving them eight million pounds per year. We're cutting their energy usage by 60%. Some of our facilities of the, where our software has been used on have won awards from the world economic forum for sustainability, these factories whether they're in the U.S. or Indonesia you can reduce energy, reduce downtime by, by, 40 plus percent in some situations. And you mentioned it earlier, Mike the cloud. course where you can... the cloud lets you bring those best practices and ideas together, wherever you are in the world. And one of our customers Veolia Water they produce water facilities to produce clean water for either manufacturing or for humans. And they use our software to share best practices around the world in the design of those water facilities, or food. They also make specialty food based on where you are in your life, whether you're a newborn or you're sick or you're late in life, we all have different nutritional needs. So they use our systems to bring better, better efficiency and sustainability into their supply chain. You'd be hard pressed to go about your day certainly where you're not interacting or consuming or using some core elements, some of the staples of our lives that you didn't wasn't touched by, by, our software. In Georgia Gwinnett County purification of the drinking water 70 million gallons of water per day using our cloud technology to streamline the production against pretty rigorous standards while reducing the energy required to do it.
Mike Walsh:I still find it ironic that despite the fact that we started this conversation with the discussion of the crisis in a canal, which, there's no doubt of its critical importance to the global economy was still very much an innovation that came, I think a century and a half ago. So as we start to really look forward to 2030 and beyond, given everything we've just spoken about, what do you think are the new kinds of challenges and the new kinds of, let's say AI powered problems that we're likely to also face as leaders as we look into the future?
Craig Hayman:Yeah. I'll start with, you mentioned us as leaders and as a human race. It's not just technology Mike. I would say what we're doing here we're interacting, we're chatting trust and partnership and innovation. These three things go hand in hand. It's by working together on that road together though, I think we, we find a shared success. I love the Suez canal point. It's really sparked my this and this Littlehampton, this 80 meter ship in Littlehampton I went back and did a bit of looking and Littlehampton is a little... I grew up near there actually, it's a little port on the South coast of England. It's it's in the Domesday Book, which you know, was about, 1080 is when the Domesday Book was written. And you think about the revolutions that, that physical place has gone through as a small sort of fishing port, the 1760s, the first internet first industrial revolution mechanical production, steam and water, the 1870s the network rail, the telegraph the net workout technological revolution. And then as we mentioned earlier, we're 10 years into the beginning of the fourth industrial revolution, the industrial internet of things. This is about the manufacturing of bringing data and the internet and productivity improvements into an industrial production in a way that is, does it with less emissions, with less energy and better quality products. And then finally, you mentioned this point about, leaders. I don't know about you Mike, but this last year has probably been the toughest year. So now we're entering a world of post pandemic 2021 and beyond. And the question is, how do we further this for the sort of the grand challenge as leaders? I think that's quite exciting and it's actually quite uplifting.
Mike Walsh:Craig, it's been wonderful talking to you today. Thank you for being on the show. And it's been really insightful.
Craig Hayman:Thanks, Mike. It's great seeing you.