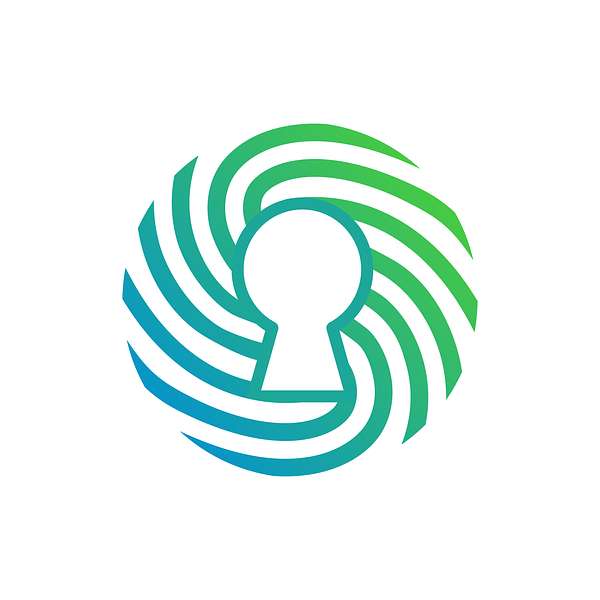
PrivacyLabs Compliance Technology Podcast
PrivacyLabs Compliance Technology Podcast
Data Management and AI Governance with David Marco, PhD
In this podcast, I explore AI governance from the perspective of data management. David Marco, PhD maintains an unrivaled pedigree and over 30 years of experience in data management. Hear his thoughts on AI governance basics, the extension of data management into AI governance and his thoughts on where we are in this hot, new and ubiquitous area. See more about David and his company at ewsolutions.com
David Marco, PhD Podcast Transcript
Paul: Hello and welcome to another podcast by PrivacyLabs!
We're honored to have David Marco here, PhD, very briefly. This is a podcast that is hosted by PrivacyLabs. We are a consultancy and marketplace. We specialize in cybersecurity, privacy and AI governance solutions and services. Let me start by asking David if you would introduce yourself, because I think that's going to be a very important preamble, if you will, into how we unfold into the conversation and drill down into the topics.
So, David, welcome!
Tell us all about your impressive background.
David: Oh, goodness! Well, Paul, thank you so much for inviting me to your podcast. This is a topic near and dear to my heart, AI governance, my background. I just welcome people, go to LinkedIn, connect with me. I've written – so I'll do a thumbnail of this. I've written four best-selling books in data management, including the two top sellers in metadata management.
I am currently LinkedIn's top voice on data governance, and I've been doing AI for quite a period of time. And my focus is not so much building Gen AI systems. My focus has been how do we utilize AI to have more successful data management programs?
I've personally built over 60 data governance and data management and advanced analytics programs. Soup to nuts. So, I'm an implementer. This is what I do all day, every day, and talking about it with pleasant people like Paul is just a side benefit. Please feel free to connect with me on LinkedIn. You can go to my company's website, ewsolutions.com. I'm very easy to find and I'm happy to be here to talk about this topic.
Paul: And I'm honored to have you!
To be honest, part of what I would recommend going to David's site and looking at what he offers, but also his impressive background, because that really is going to give us a sense of not only the credibility of the information we're going to get today, but also the foundation for what we're going to be discussing.
And I have to say, I can't imagine this being any more important now than it has ever been. So, the takeaway for me, just for some background for our listeners, David and I had a discussion. I was reaching out to professionals that were well placed, who could provide me some input on the current quest of AI governance. And we'll get to that question last in our discussion with David.
But what stood out to me the most with your background, and I think is probably one of the most important aspects of AI governance, is that you do data management. That really, the data management that we knew of, the data governance and so forth two or three years ago, is really not really different in its fundamental sense, and that since artificial intelligence is really something that takes what's there and enables it and gives it more efficiency and value, are we not then just building on what we've already got?
I really want to hear your thoughts on how that, if I have that accurately stated first, and then secondly, how you see that unfold in any issues or takeaways or insights that you think are important to understand in that, in that context.
David: Goodness, Paul, there's so much to that question and I'm going to start going through it and unraveling it, but feel free to jump in whenever you like because perfect asked a mouthful, but I have a mouthful to say on it, so I will share with you. I had the joy of actually coding and building AI way, way back. I don't want to say how long ago, because, my goodness, it was far too long ago, but anyway, and what has been interesting is really what has helped drive AI today is not only some of the new technologies, but our processing power. It is far greater today than it has ever been before.
So, we are able to utilize large natural language processors, massive neural networks to do AI stuff, for lack of a better word. And it is a natural extension. What I see, AI governance is directly impacting data governance, which is really where I was dragged in.
So, I'm currently working at a massive federal agency. They're a client partner of mine, and part of that work, they're like, hey, David, we need help with AI governance. Nobody knows how to do this stuff because it's so new, these applications are new and they're different. And Paul, I will tell you one of the key things that I bet you will resonate with for decades in advanced analytics.
So, there are six major types of analytics. One of them is automated analytics, where you try to take away decision making from a human being, where the human being doesn't have to look at it and make a decision where based on the past, based on what us AI folks might call supervised machine learning, where we could make decisions. And executives and companies have always been very hesitant to allow an algorithm to make a decision.
AI, with its massive hype, train with it, with it, with, with all these uh, different applications coming, coming out now we're seeing that hesitancy come off and that where a lot of these AI applications, they are going to create automat, they're going to have business decisions become automated, interactions with the customer becoming automated.
These are things that we never would have seen 20 years ago or even 10 years ago. Because of that, the exposure and the risk is far higher. Look at some of the regulations out there. If you've ever read a NIST RMF 1.0, it's all about risk. It's a framework on risk management. The RMF stands for risk management framework.
Paul: Yes.
David: And the whole thing, and I've had to study it in great detail, it is about understanding the possible risks of your AI application, but it doesn't even give tolerance levels for the risk because it's so unique to the agency or the corporation.
So, this is a new thing. And for somebody like me, a data person and a data governance person, it is a natural extension. Data governance is going to change at a cellular level because of AI. Because of AI governance, and I welcome it. So, for any data professionals in your audience, your job's about to change and expand, and it's a fun time.
Paul: Yes. And I actually, I picked up on a few little things you said. One is I hear the word risk and the reason I kind of couch it there is because when, the way I've always seen data governance, data management, it's really a risk-based analysis. And you look at, you have this data, which is gold, it's the new gold. How do you leverage it, how do you use it, how do you work with it?
And we both know, David, and I guess because I've done the same thing I've done roll up your sleeves, build it out type of AI efforts, you have to first look at what's the benefit, why are you doing it at all? And then you have to look at what the costs are, is the data available to you, which is, I think one of the biggest problems you have with AI.
But what this does, it seems to me, is it takes the risk, the benefit that you're looking at, adding in the benefit of the AI and then looking at the risks that are involved and then working down from there. Exactly. Go ahead.
David: I was just going to say, just to your point, especially with how many AI applications are being used, where they're going out to the public, they're going to a broader audience. It's one thing if we have a company, Paul and David's company, and we screw something up internally and it messes you up or it messes me up, that is one thing.
But when you have an AI application that may be facing your customer base, maybe going to the general public, the risk is so much higher. And you can ask companies like Uber and others Chat GPT what happens once some of these problems happen? It happened in the Dutch tax authority. They had thousands of people accused of tax fraud, who didn't commit any fraud, right? They were using AI and it just cranked it out and people didn't understand how the AI was built. And that is one of the foundational components of AI governance.
If you cannot explain your AI application, and I don't mean to an AI professional, I mean to a businessperson within your organization, you already have your first problem. It should be explainable, and it should be transparent.
Paul: You know, I couldn't agree more. I actually think that is the foundation of governance in AI, is explainability. If you don't know what your model is doing, you don't know how it's doing something wrong or correctly. So, you don't know if it's violating regulations, you don't know if it's going to measure up to what you expected from it. Is it giving you the benefit? Because not meeting up, not measuring up, is a risk unto its own, especially for public companies.
So, I think that's a key thing. The other thing, so think that that's perfect. One of the reasons I mentioned risk is that data governance. Data governance, excuse me, legal, professional compliance, however you want to couch that, typically been very risk averse. And now that we've seen this meteoric value come through efficiency, it provides how it enables people to do things. A quick example is the transcription that I'm using to record what we're saying. If I had to transcribe this by hand, it would take me hours.
Now it's just a matter of just doing nothing. Maybe, you know, so the value to that is enormous. That same type of ascension is happening in all other kinds of various client facing areas that you mentioned, threat detection, and we could go on forever. But I think….
David: I love the example you gave of transposing our discussion. A lot of are required to be something called section 508 compliant, and that is a section of US law that if you are going to build a training module or build a presentation that's going to be used within the federal space. It is 508 compliance. So what about somebody who can't see? What about someone who, the color spectrum.
So, if your image is dependent on color and one of the other topics we – they have to be able to read. They have to be able to read or have the option of reading in case they can't hear, because what if, what if they can hear? There has to be a transcription, closed captioning, if you will. And it's hard, especially somebody like me. Paul, I talk fast sometimes I badly for the person who has to transcribe what I'm saying. So the technology makes it so much better.
Paul: It really does. And you actually bring in a new facet, frankly, that, you know, we want data and communications to be accessible. It's a fairness thing. It's, yeah, we want to have that as part of what we do, just as good people and good practitioners. So, I think that's because there's a lot of public policy involved in the governance and I'm sure you could, you've gotten more than I'll ever know, but.
David: I love seeing it! I've given over 300 talks on data management, analytics, AI governance, all these topics. My lowest attended talk ever happened about two decades ago and it was on data ethics. And I was so ethics, I would normally draw about 200, 250 people. Yeah. And it drew flies. It was so poorly.
And so I love, for the first time in my professional career, people are asking me about ethics. And it's all because of AI, because of what you just said, fairness, ethical, because these are things that don't exist, like, like they're a harder thing to program. It's something I think we as humans intrinsically understand a little bit better. I'll give you an example.
When people, when a layman, someone who doesn't exist in our insane world, they think artificial intelligence is a mirror of how humans think, because we saw great movies like the Terminator, Arnold Schwarzenegger cries in the second one because it gets human emotion. That's not how it works.
Paul: Yes.
David: No, it's not AI. I mean, I love those movies, don't get me wrong, but AI really, it's mimicking human behavior. And of course, we use terms from the medical field like synapse and things like that. But really it's not human intelligence because it works differently. And that's why when you brought up fairness and ethical, that's one of the reasons struggled with it.
We stand as humans kind of that treat others the way you want others to treat you. You want to be treated fair, you want to be treated ethically, you want to be transparent. And AI just isn't – It's not a human being. So we go through some steps called AI governance to help make that happen.
Paul: Yes. And we're actually starting to touch the guardrails of other things. Guardrails, I don't mean we have just, I should say, a borderline, because you get into a thing called AGI, which is essentially automated general intelligence, which means it's fully, ostensibly, fully humanized or what have you.
I think until people, we're getting a little philosophical here, and then we'll bring it back to center here is until we understand the experience of pain and pleasure and identity and all those things that are integral to how our brain works, we're really not going to be able to mimic the brain like we think we can. But that's, I'm digressing. I think you're welcome to pick up that a little bit, if you like.
David: I have to, Paul. This is – I love that. I love these kind of conversations. So I would love to say that I learned best by somebody smarter than me, giving me really good advice, and I follow that advice and I never make the mistake, but that is not how I learn best. In fact, I learned best by putting my hand into fire, burning it, and saying, wow, I never want to experience that again, and I'm never going to experience that again.
And I think most of us, if we look at those seminal moments in our lives, the ones that got us going, maybe from a wrong direction to a better direction. Talk about philosophical. I'm going to take your lead and run down the path. It's really those times of trauma, those times of difficulty that led you to those. And how do you create that? In a lisp program or with Python?
Paul: Yes, good.
David: Good luck! That harder one.
Paul: That's precisely it. And again, this is a whole other topic on its own, and I'm sure a fascinating one that we could probably carry on for a while. But I do think that there was a professor, I'll just mention very briefly, who's out of University of California, Santa Cruz, which is not far from where I am. He wrote a book. And in there he says, well, what if I could take the brain and replace each brain cell with a silicon version? And over time, I would eventually replace all of those cells.
Now I have a fully silicon kind of based brain. Is it the same thing? So anyway, you get these heady kind of, you know, I don't know, you want to go smoke pot or something to think about it. But yeah, I think that, you know, the experience of pain and pleasure that plays such a millisecond role in everything that we do is really a whole other area of research and philosophy.
Frankly, I think what you mentioned is a great segue into my second question, which really has to do with how does a company or an enterprise or project get their hands around this? Let me just say at the outset, set it up, is that if I recently put a podcast out on mystarrettlaw.com pod or, excuse me, blog post, that generative AI is kind of like a pinball machine, whereas standard AI is more like golf. You have a ball, you hit the ball, it goes down and it goes in the hole. It's very straightforward. You got the wind, you get the player, you got the golf club.
However, generative AI is much more like a pinball machine. It just goes in and bounces around. So, it's a very different, it's a very different place. So you have that. It's very difficult to explain the millions of decisions that makes on any given prompt. The other thing is AI generally just adds dimension. You have training data, you have SAS platforms that you have to use, and I'll finish up here in a second.
But an important point is that what I've seen is that most AI or machine learning projects or engagements, what have you involve many different people that are from different backgrounds coming together to marshal the quest of the AI project. And they all. Yes, and you have to keep it simple, stupid. You know, the explainability comes right back to your point earlier. If each of the doesn't understand it, they can't have their expertise accommodated.
So that adds complexity, does it not? You have the programmers, you have the data people, you have the lawyers, you have the DevOps, the people who are writing the programs, all converging around this one thing. You have the data lineage. Where did it come from? Is it IP issues? Is it the model governance, the training itself?
I'm sorry, I'm throwing this all out there. What you did, what you've done is you've taken data governance, even from the standpoint of AI governance, before genetic AI, and you've really blown it up. It's like a grenade. Your thoughts on, how does somebody take that beehive and run with it?
David: Oh, heavens!
Paul: Sorry.
David: You gave me ten threads that would have loved to pull on, but I'm going to go to the last question. I'm going to kind of go slowly and hit several things because it's such a big question, it may take up the rest. That's okay. So.
Paul: Sure.
David: Because I want to get to where does the company start and what do they do? So let's take one step backwards. When we talk about AI governance, what are we talking about? It's a framework of policies, regulations, principles, capabilities and practices that are going to what are we doing with that? We want to guide the development, deployment and use of AI technologies. Now, the key word I want you to, so with your background, I'm sure when you hear regulations that wow. Become so crystal clear in your head.
But one of the things I want to dive into more is capabilities. And this is something, even when you look at GDPR and NIST and IEEE, you see this to me, it's glaring in its omission. And I will get into that about now. So let me kind of get into when you are talking about building any AI application, Gen AI, I loved your example. People who know me privately know I'm quite the sports person. I've played or competed in a ton of them, except for golf.
Paul: Me too.
David: Golf is, although I played it a couple times, but that's about it.
Paul: I'm not good at it.
David: Boy, that is a hard game. That is a really, really, really hard game.
Paul: Yes.
David: Anyway, but I still, I loved your analogy because I could really, I could, I like your pinball analogy. So regardless of if it's Gen AI or something new that happens tomorrow, it doesn't matter. All of those applications use what data?
And at the typical global 2000 company, and who's ever listening to this, I am talking about your company right now. Any of you. The data in these companies, why? First off, wildly redundant. Needlessly so, so in my professional experience, the average company has four-fold needless data redundancy. What do I mean by that? If they have a pet, if they have four petabytes of data, you can remove three petabytes, have the same backup, same recovery, same, same reports that are being used, same everything. It's just needlessly redundant.
Number two, very few companies have an understanding of their data. Let me give you an example. I will not name the company, but there's a company I worked with that our company worked with, and they create beautiful, very high-end accessories. Everybody here would know this company and they load their customer facing website from eight excel spreadsheets. Let me let that thing. And for your knowledge of risk, feel free to wake up. You probably fainted hearing that. I asked three of their key people. Their chief marketing officer, their chief merchandising officer, and a third person whose name I don't remember.
How do you – What is the definition of product number? I got three different definitions of what a product number is for a company that sells high end merchandise. How the heck are you going to build an AI application when you don't even have an understanding of what the heck product number means?
Paul: Absolutely.
David: So, understanding terrible, inaccurate data. That's the third and the last. Just death stake, if you will. There are four horsemen in the apocalypse, but there are three on data, so let me give you the third. I am stunned at the things I see in the data sets that are existing out there, how wildly inaccurate the data is. So, when data is either misleading or misunderstood, good luck getting your AI application to work correctly.
Paul: Yes.
David: And it's unfair to the developers and their team, so we got to get that right. So, I'm going to pause, let you ask some follow up questions, and I'll get into how do we do it? Which is that capabilities component.
Paul: When you say that the data, I know it to be ROT, redundant, obsolete or trivial. And I think that there's risk there. There's enormous risk with data that is, that you keep, that you don't need.
The other thing, though, is I think you're absolutely on point that they don't understand the data. The data quality is not good. That is to say, a product number, if in fact, has different meanings. You don't want to conflate machine learning training set or supervise data on data that's not properly resolved.
I'm probably getting a little bit under the glue here, but I'm merely just, I guess, acknowledging what you're saying and that your machine learning is no more intelligent than the data you give it. Period. Yes. Your machine learning AI, whatever it is, generative or not, is no better than the data you give it. It is no more intelligent. It's a silicon chip that is learning from what you tell it. To your point, given what sounds like a fairly unimpressive state of data management, that we really have a hill to climb here.
David: 100%. And that's why, in my opinion, we better get the data management correct. We better be able to understand what our PII is able to track it. I'll give you an example of that R1 world scenario, a massive manufacturing company. They tell me, here are the people in charge of our PII, personally identifiable information, things like Social Security, number, gender, street address, just to make sure our audience. I know you know what all this stuff is. Sure. Keep everybody with us. They tell me. We have, Dr. Marco, we have very, very strict guidelines and regulations and guardrails for all this. I go, wonderful. And I'd been working there long enough to know that wasn't true.
But I asked, I go, oh, can you give me a list of what you consider PII? Because I will, you know, and I didn't add this part, but I'm sure you understand this, the way GDPR defines PII is different than CCPA. CCPA, much broader.
Paul: Yes.
David: And both of them are plenty open for interpretation, are they not, Paul?
Paul: Yes. Well, this is what lawyers. Ambiguity and adjectives.
David: This is, I mean, they're. I wasn't going to say that. But Paul, you are absolutely right. You can argue about all this stuff. So I say to him, give me a list of the PII that you are managing. And he goes, I don't have that. Okay, so you don't even have – So you've not even gone as far as to say name, first name, last name is PII, so you don't even have that. So if you don't know what you're managing, you're certainly not doing it.
Long story short, we brought in data management software that actually uses machine learning. Pointed it at hundreds of their databases. We found PII replicated probably fivefold in just one off database. Just Social Security numbers sitting in a table somewhere. Yeah, all over the. Everywhere. I mean, including executive pay, we had that, too. And the pay of everybody. It was everywhere. And they did not even want us to run those algorithms because they're like, well, that's very invasive. I said, well, first off, we are identifying where it's at. You now can go and clean it.
But in truth, they didn't want the disaster to become known. And so this is a reality within organizations. It's why we see data breaches. They're almost comical at this stage. The ball. Yeah. So that is the – And so to me, as a data management professional who has been doing this forever, I, you know, you mentioned my background. There's a couple halls of fame for this. Somehow I'm in all of them.
Paul: So, it doesn't surprise me.
David: Somehow people think I understand this, and I do. I've done this long enough. You clearly do, Paul. When you're doing data management, it always comes down to the basics. Do I have the metadata management? Programmatically. So we need solutions to do this. Where I understand the who, what, when, where, how and why of my data. If I don't have that, I have nothing. We're not even in a ballgame.
Paul: Let me finish your sentence. Amen. [Crosstalk]
David: Amen. I love it!
Paul: Yes.
David: Second thing is, so we need that. You had stated it early when you said the word data quality. I wanted to circle back to that for every business term. So let me, this is probably grainy and in the weeds, but I. Let me go here anyway. Cause I'm an implementer, I build this stuff. It's what I love to do. It's my passion. When we talk about a business term, think of something like customer type. We could have hundreds, if not thousands of data elements. Data elements, think column on a table field in a file. We could have thousands of data elements that represent customer type.
You know, if we're in some fortune hundred, 100 companies, that business term must have a precise definition. So, a good definition is not a tautology, meaning the definition for cheeseburger should not be a burger with cheese. That definition told me, now I'm getting hungry. You know what, I could use the burger, which she's about now. That's okay.
So, it needs to be a precise definition, but to your data quality portion, we need to have a valid value set. So, if we have ten types of customers, 1, 2, 3, 4, 5, 6, 7, 8, 9, 10, we need to define those. Because what companies try to do is they say, we have a data quality issue, let's start cleaning tables. But they never go through the exercise of defining what does their data mean? What are the valid values from an enterprise perspective?
So, without the valid values, you have no rubric of analyzing data quality. There are eight dimensions of data quality. I won't bore everybody with all of them, but one of them is accuracy. If we have defined what it's supposed to, what it's supposed to be, that there are ten. Number one means this, number two means this. If we haven't done that, then we cannot fix the data quality.
So, these are the capabilities we need to build. It is what we've been doing for almost 30 years at EW solutions. And it can be done. It can absolutely be done, but it means it takes sustained effort. Let me take a breath, because that hit that capabilities point when I gave that definition of AI governance.
Paul: Yes, and I'll make sure I wasn't cutting you off or interfering with what you're going to say.
David: Oh, no. Jump in or I'll start going through the basic. Because again, I want to keep kind of going back to. Your main question is, where do we start? How do we begin this?
Paul: Well, yeah, no, I should let you finish. I did want to throw out a few more cliches. Garbage and garbage out. There is one thing I want to drop, and this is I have another blog post on my website,starrettlaw.com, that talks about this thing called the CRISP-DM – C-R-I-S-P DM. Don't ask me what it stands for. It weird. You Google that. It basically talks about the data management soup to nuts sequential process where your business value data cleaning, data analysis, training, production, and then monitoring. It kind of walks you. Any hiccup or failure upstream affects what's downstream.
When I got my master's in data science, all of my assignments had to be done. I had to walk through those processes and specifically name that standard. And so it's kind of like a recipe. And if you don't follow it to your point, one of the issues is the accuracy and the quality. In fact, GDPR, the General data Protection Regulation, talks about data quality as a core concept of what it expects companies to pay attention to. So anyway, I don't want to get too far off field there, but I thought those were important to mention. Please continue.
David:
Yeah, and actually, just to kind of, again, pull a little thread you left out there where you talked about how when defects in data enter into an environment, it becomes more and more problematic as it moves downstream. There's actually a rule in data quality known as the 1-10-100 rule.
And basically what it says is that if we resolve the defect before it comes into our systems, it'll probably cost, you know, it would cost $1 if, let's say that's what it would cost if we try to fix and clean the defect, resolve it after it's gotten into our systems and gone all over the place, it'll cost $10. The $1 becomes – [crosstalk]
Absolutely. And I forget. So if the people who came up the 1-10-100 rule listening, I apologize. I'm forgetting your name, but I didn't come up with it. But it's great stuff I quoted. However, if that same defective data leaves your walls and goes to partners, customers and the like, now your cost is 100. So it's orders of magnitude 110-100. That's why data quality needs to be right immediately, and we have to stop it before it ever gets into our system problems.
Paul: Absolutely. And I, you know, I'm going to throw another cliche, an ounce of preventions with a pound of cure, but these things need to be known. And that's wonderful, by the way. Thank you for sharing that. That really puts some quantitative, you know, realization on this. But I think that. Can I segue to the next question or did you want to flesh out? Feel free.
David: Paul, bring it on.
Paul: Okay. And I know that we've talked before this, the gloves are off. So it's just really kind of bringing us back to your background. And the purpose of this is, it's my feeling that in many ways it's just as easy to do it the right way as it is the wrong way. You build into the front end, you build into the front end of this planning at the outset, understand that data quality 110-100 thing, that's like, holy mackerel, we better spend on this, right? This, we're saving money.
But given when we started, say, data governance, AI governance are really part of the same ecosystem because you're pulling data from repositories and places that are already there which are already governing. So what you're really doing is building out an upfront from where you already are. I think in many ways when you get into the actual building of the model with program or something, you have application security, you have the CRISP DM sequence which they follow and track for auditing purposes. But beyond that everything else is pretty much the same.
So what you do is you start with that and you can build up and out into the AI governance. And what I'm looking, what I'm saying is I'm looking for an encouraging thought here, that this does not have to be something that's not understandable or something that is manageable, that everyone kind of just kind of relaxes, say, okay, just have to do it right. Is that, take it beyond rhetorical.
David: I completely agree with you. If you cannot explain what your application does in a language that a non AI person can understand, then you don't understand it yourself. And your point? Do it right the first time. I've been consulting a long time, so I've seen a lot and a lot of companies and there was a period of time, let's call it the time of insanity, when people would say, you know what, just build a system, get it out there, we'll go fix the problems later.
And in my experience, nobody ever goes back and fix the problems until it's bad that the house is burning down, the cars are on fire, and you need to build a brand and that's my experience with it. Do it right the first time.
Our client partners, the ones who've had the greatest successes, the ones that, in 30 years, when I'm retired somewhere, sipping probably a protein shake for people who know, sipping a protein shake somewhere and looking to lift the barbell, I will think of those clients which were really my most enjoyable programs ever, and they did it right the first time. They wanted to do it right the first time. They didn't want to. Just, I can name you an organization right now. I will not name them. And they approached us, wanted to talk about us doing an engagement. And this is a billions and billions and billions of dollars. And the stuff they do. Lives are on the line.
And literally, we realize we're just not ready to work with a company that really wants to do it the right way. We want to kind of just and I'm like, no problem. Whenever you're ready, let us try to do it right. Unconscionable. If you were to understand what you, especially with your background and regulations that they're asking for it and the. It is not a good thing.
Paul: Yes. And if I may, I think a lot of these people are under a lot of pressure. You know, they're supposed to turn. I mean, I know you notice I'm just, just sort of expanding the, the concept here, but so what I can glean from this, and it's an obvious answer, is that it's something that you can ultimately relax about, or at least relax more about, because it's already working with systems that you have the data you've got. They maybe do things, they're new concepts, but it can be put into common terms, and it has to be, just recognize that and lean into it and accept it, and it's okay.
And people like you can come in early and help to make sure that they're off to the races in the right ways, saving money, using preventative approaches, and being able to sleep at night knowing that you started out of the gate the right way.
David: Exactly. And when the data's right and you have good, well understood data, now you're seeing real analytic results, real things. I, you know, I could share with you clients we've had where they took mortality rates from cancer and reduced them because the data was clean, it was well understood, and we got them into professionals who could use it.
That's the kind of stuff that, again, for me, is exciting, where you can, where you have real results. You know, I could share financial institutions who, who have been far more profitable just to your point of, hey, we can reduce our risk, we can reduce our exposures, and guess what? We can save money.
And one of the biggest challenges any CDO, we work with a lot of chief data officers. One of the biggest headaches is how large the IT footprints are. They have so many applications, so much technology. A little fun with you, Paul. What is the most popular form of technology? You know, we hear about database systems and the like, you know, Microsoft. What's the most popular type of technology? I'll answer for you. Gaming. Gaming, probably shelfware. It is technology that we purchase, that companies purchase, and it doesn't do a d*** thing. It just sits on the shelf and they're paying, right? Yes. A bit of a fun joke.
Paul: Okay. I've heard that term. No, and I follow you.
David: Shelfware.
Paul: It's really crazy!
David: Exactly. So I'll give an example when, when we work again with a large company. And, you know, if you were to ask them, hey, what database technology do you have? They probably, if they really knew their systems, they'd look at you and say, well, all of them, I think they knew a car company.
Everybody here would know this company. They wanted to build a massive data warehouse, and they have hundreds and hundreds of millions into their data warehousing. And they say, hey, we want to build it with. They decided to build it. It wasn't my choice. I wasn't there at the time with DB 2 at the time, perfectly good, strong database software.
Paul: I remember that.
David: However, in this mega Fortune 50 company, how many DB 2 DBAs did they have? Zero. Not even one mega Teradata shop. Everybody knew data, so they didn't have the structure to support it. There's nothing wrong with the database. It's a good one, but they just bought it.
And most companies have, I mean, there's – How much reporting software, hundreds of analytical software that people come by. Most companies have 40, 50 of them. And it's just useless stuff that they didn't need. So, this redundancy is so difficult. We've mentioned the redundant systems to. Redundant data, redundant processes. I have one company. Again, you'd all know this. They make apparel. Very stunningly well known. They have 28 order entry systems.
Paul: Wow! Paul, talk about herding cats.
David: Yes. You're ordering sports apparel. It's not that complicated. Like, give me some shoes, give me some socks, and I'm ready to roll. Like, it's not that complicated. You got 28 of them. That's kind of what we're dealing with. So anyway, so reducing that footprint is a big deal. Data management, really big in the net and into our eye to bring it back that AI governance, we need to get those fundamental components working and in our AI governance programs we need to really make sure, and I think you touched on it a bit, is these systems, they're still systems, it's still silicon, it's still zeros and ones at the end of the day.
So we need to test them the way we do systems. We need to have integration testing, we need to have regression testing, we need to have user acceptance testing. We cannot assume. And the one area where these systems are even more demanding, and you brought it up, is because of the nature of AI and it's constantly changing. The model is learning.
In addition, most, and we've spoken about, hey, we're building a new Gen AI system, but really, and I know, you know this, rarely are we building one system. Typically we're pulling from a couple. So I call it the AI stack. You know, so it's more than one. Even if we're building something new right now, we're probably pulling from two or three existing things. We're not going to rewrite a natural language processor.
Paul: No, that's a risk. Oh, trying to rewrite your own, you know. Yes, and third party risk. And that's, that's going to be a whole other discussion. Maybe I can have you back, David, for that, but I hear everything you're saying. I think we're kind of coming up on the top of the hour here. Happy to go beyond that.
But I think, maybe one way to kind of encapsulate things is that you do it right the first time. It's something that can be done, it's risk based. You don't have to go and put a glass dome over everything. If it's a low risk, if it's a low-risk project, then you don't have to go and put locks in all the doors, figuratively speaking.
So accept that, recognize the incredible value that generative AI has brought to us. Pinball machines work. You know, if the analogy works, then degenerative AI can work. And here's where I'd like to kind of go here as we close out. So, we've got this sort of very high level, pristine kind of utopia of start early, monitor it. Of course, it's not just, well, we did it. Great, now let's go off and have lunch. No, someone's got to keep their eye, nose and bright spin.
Last question is, where are we now as an industry? I think I could tell already. But the last question we spoke about was, what's the current status of AI governance? It is, Nathan. It is Greenfield. We can state that. But is it compliant? Is the hype pushing running us path where we should be?
David: Boys, what a great question. And so I will go with. You've already hit the point where you mentioned Greenfield. I call it brave new world.
Paul: The Wild Wild west. Yeah.
David: Because it is. Right. It's a brave new world. All of these, again, you know, go right through the AU, which you probably have the EU, AIAC. Yes, this is evolving. It is changing. The United States AI Bill of Rights is the Chinese one. They're all changing and evolving, and they will continue to do so because AI is changing and evolving.
One of the dirty secrets, like people look at Chat GPT is one of the things that is a popular topic in our industry. People don't realize it is wildly expensive to run that sucker every day and they are losing half a billion dollars a year, Open AI, at least by some measurements that are out there. How are they able to do it? Microsoft gave them, I think it's about $20 billion. That'll keep the lights on for a while. We could get into why they're doing it, but that's probably best left for another time.
The point is, until we can figure out how to manage that kind of power drain and that kind of demands that these systems require, things are going to change. They're going to constantly change. And for professionals like us, we have to be able to right size our solutions. I loved the term, I forget the exact words you use, Paul, but I'll use my term right size.
If you're building a – Yeah, like, I live in Chicago and I'm quite the football. If we're building a generative AI system to manage our weekly football pool, um, we don't really need to worry about risk a whole lot. It's just that. And we don't need to spend money and time on it. However, if we have something that's going to every one of our customers, that's now a whole different ball of wax. So I'm with you about that. That right sizing we don't have to. Do not let perfect get in the way of good enough there is.
Paul: I love that!
David: Yeah. And we've been, we've been blessed. We've won basically every award you can win in data management and not a single project could I cite that was perfect where we covered every scrap of data because there's no value in that. Let's hit the 80% that we're using everywhere. Let's hit the stuff that's really relevant. You know, it's the 80-20 rule, right? Like 80% of the value of your data comes from probably 20% of the data.
And same thing here. If we're going to build these solutions and we're going to govern our AI better, let's focus on the things that have a high impact, things that are seminal to our business, the stuff that's in our lab that we're playing with, the stuff that isn't going out to customers, probably, that we don't need to spend nearly as much time. So I loved your point when you hit it. I want to go back to it.
Paul: Thank you! And I do think this doesn't leave any of the precepts of data management. It's all a risk-based approach. Ultimately, it's just looking at the value and looking at the risk or the probability of harm. That's the term we use in legal, probability is how frequent is it? Harm is how mag. How much is the harm? And you can, you can get a basic equation and it's not, it's not. Doesn't make your heart, your head hurt. So I think we're - [Crosstalk] Go ahead. I'm sorry.
David: Oh, no, I was disagreeing with you, Paul. You're 100%. But what is the probability of harm and how bad could that harm be and what's realistic? It's what you do, really, in a lot of basic analyses, not just in data or in AI, but just in life and running a business, you do those analyses all the time.
Paul: Yes, exactly. No, that's. I think that's really the point, is that a lot of what we're talking about, AI governance, is really an extension of what's already there. And so that's, frankly, that was the big takeaway, I think, from our discussion. Nothing else is that it's already there, you know?
So I think we're kind of coming at the top of the hour. I would like to give you the opportunity to share your contact information. I will put that at the base of the transcript anyway, or anything else you'd like me to put there. I do want to kind of clarify something that I mentioned earlier. I have a consulting firm called stare at law. My last name,starrettlaw.com, where we are purposely separate from PrivacyLabs.
PrivacyLabs does have consulting, but we focus on the legal and regulatory piece. Separate on purpose. We can represent people. That's why it's separate privacy labs. As consulting, we have a marketplace that uses a generative AI back end. So just so people understand why the two different firms.
So David, if you want to share your information or I just put at the bottom transcript.
David: Absolutely. Feel free to reach out to me on LinkedIn and connect. I'm David Marco. It can't be any easier. You'll know it's me pretty quickly. So, I mean, how many more can there be? If you have a question on your program or if you need help, feel free. Will include my email in the bottom of the transcript. Feel free to reach out because this is what we do.
Also, we have a whole knowledge portal at EW solutions. It's free.
Paul: Nice!
David: Articles and videos. We'll include that in the transcript. Join in. I post a lot of stuff on LinkedIn and doing a series of AI governance little three-minute teachings where I'm going to take a topic, hit it, record it, and then trickle them out over time.
So follow me there and let's keep the conversation going because as you had mentioned, it's a green field. It will be evolving. And that excites me. I remember when data warehousing was like this. I remember when metadata was in data governance. I've seen these cycles before and I can't wait to experience it here. To me, it's what keeps me so motivated in our industry is that it is constantly changing. It's not the same old, same old that we did 30 years ago. So it's fun.
Paul: Well, yes, and I would like to close on that. Let's look at this in an optimistic way. Let's look at this in a way that is a hype cycle. There are going to be risks that we're going to trip over ourselves. We're going to put the iron in for our hand in the fire, as it were. But ultimately, this is something to smile about. This is something to feel good about. It is a disruptive technology, just like the cell phone and the printing press and google search engines and stuff. This is where we are. Let's be happy.
And we are thankful to have people like David who offer free services, but also have a very mature and current kind of set of solutions and services that you, that you can leverage to make the journey worthwhile. And I'll have to also give myself a shame here. I'm the same way.
But anyway, David is our guest today. Unless you have something else you'd like to close on, David, which you certainly can do. I would probably think it's a good time to close down. We go on for 8 hours if you wanted to. I can tell you back soon on a similar topic. But I do want to thank you so much. We're very honored to have someone like you who's so prolific and will place, but also who can actually come in and give us real usable advice and help us kind of encapsulate all this.
So thank you so much, David, for your time!
David: You're welcome, Paul, it was a pleasure speaking to you! I had a blast.
Paul: Wonderful! All right, sir. Thank you!
David’s information:
Email: DMarco@ewsolutions.com
Website: https://www.ewsolutions.com/