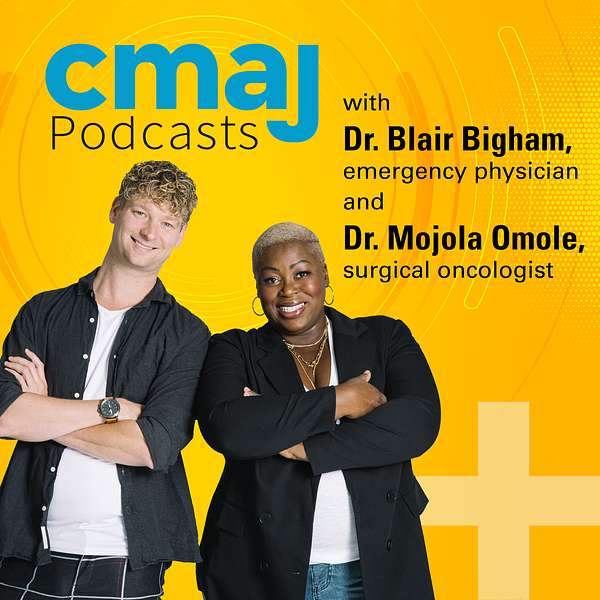
CMAJ Podcasts
CMAJ Podcasts
AI versus physicians: who’s better at spotting high-risk patients?
On this episode of the CMAJ Podcast, Dr. Blair Bigham and Dr. Mojola Omole discuss how artificial intelligence (AI) significantly improves the identification of hospital patients at risk of clinical deterioration compared to physician assessments alone. They are joined by Dr. Amol Verma, a general internist at St. Michael’s Hospital in Toronto, an associate professor at the University of Toronto, and the holder of the Temerty Professorship in AI Research and Education, who shares findings from his recent CMAJ article, “Clinical evaluation of a machine learning-based early warning system for patient deterioration”.
Dr. Verma explains how the AI system, ChartWatch, analyzes over 100 variables from a patient’s electronic medical record to predict deterioration more accurately than traditional early warning scores like the NEWS score. He discusses how the integration of AI into clinical workflows improves patient outcomes by complementing human decision-making, leading to better results than relying on physicians or AI alone.
The episode also looks at the potential future of AI in medicine, with Dr. Verma sharing insights on how AI tools should be thoughtfully integrated to support clinicians without overwhelming them. He stresses the need for AI systems to fit seamlessly into clinical workflows, ensuring patient care remains the priority. While AI is currently a tool to assist clinicians, Dr. Verma argues that the full extent of AI's role in healthcare—and its impact on the physician's place within it—remains ultimately unknowable.
For more information from our sponsor, go to medicuspensionplan.com
Join us as we explore medical solutions that address the urgent need to change healthcare. Reach out to us about this or any episode you hear. Or tell us about something you'd like to hear on the leading Canadian medical podcast.
You can find Blair and Mojola on X @BlairBigham and @Drmojolaomole
X (in English): @CMAJ
X (en français): @JAMC
Facebook
Instagram: @CMAJ.ca
The CMAJ Podcast is produced by PodCraft Productions
Dr. Mojola Omole:
I’m Mojola Omole
Dr. Blair Bigham:
I'm Blair Bigham. This is the CMAJ podcast.
Dr. Mojola Omole:
So today, Blair, we are going to be talking about AI in medicine.
Dr. Blair Bigham:
Yes. A very hot topic. And let's talk a little bit more generally before we get into the CMAJ article.
How does AI affect your job Jola?
Dr. Mojola Omole:
It doesn't,
Dr. Blair Bigham:
Right? Mine neither. But isn't that crazy? Everyone's talking about AI, but on the ground level, I
don't know that AI is doing a lot for me, but I think we'll sort of give a prelude here that AI might
be doing more than we think in the background.
Dr. Mojola Omole:
For sure. And I would say that similar to what we're going to talk about ChartWatch, you also
use Epic. We have, what's it called? The new score or the…
Dr. Blair Bigham:
Yeah, there's a lot of scores and sort of computations that happen within Epic that are meant to
sort of alert us to help us do our job. I've heard a lot of it referred to as augmented intelligence
where it's sort of just like it's a computer just flagging something reminding you of maybe the
best practice or what you ought to do. But today we're getting into something a little bit more, I
guess technological or advanced. We're going to talk about real artificial intelligence.
Dr. Mojola Omole:
And I think what I loved about it is that as we talked about with Epic, it just pops up when you
log in onto a patient chart, but with ChartWatch, it actually sends the clinician a text message
saying, “Hey, you should be aware of this.”
Dr. Blair Bigham:
Yeah, it's a nice blend of both automation and artificial intelligence. So we're really, really lucky
today that we have one of Canada's experts in artificial intelligence in healthcare. Dr. Amal
Verma. He's the author of an article in CMAJ this month titled, “Clinical evaluation of a machine
learning based early warning system for patient deterioration.” He's a general internist at St.
Michael's in Toronto, an associate professor at the University of Toronto, and he holds a Temerty
Professorship in AI Research and Education at U of T.
Dr. Mojola Omole:
You're probably tired of hearing from us. So let's talk to Dr. Verma.
Dr. Blair Bigham:
He's up next on the CMAJ podcast.
Dr. Mojola Omole:
Amol, thank you so much for joining us today.
Dr. Amol Verma:
Oh, thanks for having me.
Dr. Mojola Omole:
So the focus of this study was deterioration of patients in hospital on the general internal
medicine floor. How big of an issue is this?
Dr. Amol Verma:
So we know that roughly five to 10% of adults who are admitted to an internal medicine unit will
end up either needing ICU or dying in hospital. So it's a fairly substantial problem. And we also
know that from a patient safety perspective, unrecognized patient deterioration leading to
unplanned transfer to ICU is one of the sort of leading causes of those unexpected ICU
transfers. So this is a really important patient safety issue.
Dr. Mojola Omole:
How will Blair make money if you don't transfer them to ICU?
Dr. Amol Verma:
Well, my goal is to transfer them a bit earlier, sometimes even so then they can rescue and do
their work in a more controlled fashion.
Dr. Blair Bigham:
So how does the AI work that you've done, and we're going to get more into the details in a
second, but how does that differ from some of those traditional early warning scores?
Dr. Amol Verma:
Yeah, so we know that actually early warning scores have been used in clinical care for even
going back as far as decades now is something like three quarters of UK hospitals use
something called the National Early Warning score or the NEWS score, which is probably one of
the more common ones. Those traditional early scores actually have real utility. So I'm not a
huge naysayer about them. They were largely developed to take vital signs and convert them
into a numeric value, like a score that you could add together and calculate risk. And so they
were done with simpler statistical tools like they're based on logistic regression models and they
create these sort of points-based scores for patients. One of the things about those tools is that
they tend to be first a little bit over calling. So they tend to have a lot of false positives. You get a
lot of false alarms with them, right? You're in the hospitals,
Dr. Mojola Omole:
A lot of me saying aware, aware, aware, defer to primary team, which is GIM.
Dr. Amol Verma:
Yeah. So if you work in a hospital with one of those, either a sepsis or a deterioration alert in the
EMR, we just get used to tuning it out and that's a real problem. The other problem is, it's
Related, one of the reasons that they have a lot of these false alerts is those scores don't take
into account patient context. It's just like a high heart rate is the same score for every patient.
And so a machine learning tool ideally would have more inputs and it would consider how a
a patient's trajectory changes over time. So the tool that we developed takes about a hundred
inputs from a hospital's electronic medical record, some basic patient demographics. We took
vital signs of course. We took all of the lab tests and from the vital signs perspective, it was a
little bit broader.
So it wasn't just your heart rate and your blood pressure, but also your pain score and things
like that might be charted in the medical record. And so those hundred inputs are modeled to
predict the outcome of death or ICU transfer. But the additionally intelligent part about this is
one, we didn't pre-select out of those hundreds of variables, we didn't pre-select what the
computer should pay attention to when, and we asked the model to learn not just based on
the first instance of those things being measured, but as they change over time during a
patient's hospital stay. So it starts to take into account the trajectory of those things. So if a
patient has a chronically elevated heart rate, maybe it stops paying attention to it as much, for
example. So it is a dynamic model that updates its predictions based on the time that a patient
has been in hospital based on the trajectory of their previous scores, et cetera.
Dr. Mojola Omole:
And so how is it integrated into your typical workflow as a physician. We just talked about we get
the deterioration score and then we press ‘aware.’ How is this different? How does it go on my
dAIly basis when I'm rounding on my patients?
Dr. Amol Verma:
We tried to be really thoughtful about how does this tool actually enable and support clinical
workflow on our internal medicine unit. And I think that's a huge difference between, if you begin
with a clinical care unit and you begin with the problem of patient deterioration, you say, how
can an AI tool solve a problem and integrate with the workflow to improve the workflow versus,
we bought an electronic medical record, it has a prediction tool in it. Let's turn it on. There's
two totally different starting points and ways of conceptualizing how to develop and use a tool.
So our tool was very customized for the clinical workflow on internal medicine at our hospital.
For example, the tool runs off of the electronic medical record using the data that the physicians
and nurses are already entering into the system. No new data entry. In terms of how to
communicate those alerts, we thought about a few different ways that it could inform clinical
care. So the first and most obvious way is that when a patient becomes high risk, it sends a text page actually to the clinician. So we have on our internal medicine unit, the physician teams carry a mobile device and the charge nurses carry mobile devices that can be paged. So the charge nurse and the relevant physician team gets a page saying this person is high risk. And then they go and communicate with the bedside nurse and there's a care pathway for actually caring for high risk patients. Importantly, those alerts have a bunch of silencing rules on them. So if you have one alert, it won't repeat for the next 48 hours. So we don't keep dinging you. If you just came out of the ICU, it won't push you an alert again. So there's a variety of silencing rules.
If a patient has had more than five alerts, we start to silence the pages that come from it. So we
created a number of silencing rules for those alerts. But then there are other ways you can see
the patient's risk status. So we have a sign out tool and on our sign out tool, which is how we
assign patients on our clinical team for each day. So you have 20 patients that you're caring for
on our unit, which physician is going to care for which patient and where a teaching hospital, so
which resident or student responsible. So that tool gives the patients updated status every hour
so that when our teams print out the paper form of that tool in the morning to set their workflow,
they can look and see who's high risk today and they can assign more senior trainees. So the
senior resident or the staff physician would go see that patient themselves. In an analogous
way, we know that our nurses make bedside nursing assignments twice a day. It was actually
very humbling for me to hear that at three o'clock in the morning there's the charge nurse is
awake making nurse shift assignments for the next day. That is the heroic work that our nursing
colleagues are doing at three o'clock in the morning. So we designed an email that goes out at
3:00 AM and 3:00 PM to line up with when they're making their shift assignments. And that
email goes to the charge nurses so they can see the patient's risk status so that when they're
assigning bedside nurses, they can proactively make sure that one nurse doesn't have more
than one high risk patient. And so you can kind of balance the load. And then the last way that
we had it designed was, every 24 hours there's an email that goes to the palliative care team
and says, here were all the high risk patients in the last 24 hours, why don't you check in?
And so what happens is the palliative team sees that list and they contact the attending
physician and say, would a palliative consult be helpful? Would you like us to get involved early?
And so then the care team can decide whether to involve palliative care. And I'll say one of the
things we talked about was whether to send automatic escalation alerts to the ICU and we
actually decided not to because we have a pathway for escalating to the ICU. We have a critical
care response team that already exists. Nurses and physicians can call and involve them. And
there still are false alerts. So in our system, for every three alerted patients, one will experience
an episode of deterioration, meaning they will die or need ICU in hospital. So we talk to our ICU
colleagues and they're like, you know what? We would rather not get three alerts for every one
event. We just don't have the resources to manage all of that. But the work teams were actually
three to one, we can handle that. I want to know for three patients, I'd be willing to work up
three patients to address one of those events. And so with the ICU escalation, it's more just
when the clinical teams feel it's necessary.
Dr. Blair Bigham:
What are the clinicians on the front lines saying about this?
Dr. Amol Verma:
I'm always a bit cautious to say what clinicians are saying about it in the one sense because
they know that I helped design and implement it. So I'm pretty sure I don't get a totally
unvarnished set of opinions. But I will say no one complains too loudly to me or even loudly at
all. So we haven't had a lot of complaints. We do hear of course from that, of course the tool's
not always accurate. And I've heard anecdotally like 50-50% of the time it's useful, which
personally, I think, is a pretty good ratio.
Dr. Blair Bigham:
That’s way higher than in hospitals that I've worked in either where we've implemented it and
that's always a struggle or where it was already in place for a couple of years before I showed
up, the complaints were high. People said, we don't really see the value in this. There's a lot of
false alerts going on.
Dr. Mojola Omole:
How early though, is it identifying a deterioration?
Dr. Amol Verma:
It's a really good question. So what we know is that without the alerts, we did a little chart review
in our hospital as we were just trying to understand the problem. So we identified 20 patients
that had a death or a deterioration event, and we looked through the chart and what we found
was for the deterioration events like the ICU transfers on average there was less than three
hours notice between the alert and the actual event in terms of document someone documented
that they were worried about it. Obviously that's an imperfect way of assessing clinical
awareness. We don't always write down what we're aware of. And we know there's a term for
this, right? It's like the crashing patient is something we talk about a lot, that unexpected
deterioration. So in one of our earlier papers, we looked at the time from the first prediction to
the outcome.
And so I believe that on average in total, it was somewhere around three to four days between
the first prediction and the outcome. But that includes deaths in which patients might receive
palliative care, for example. And so in that context, it's a longer window. Someone might be
palliative in hospital. So if you just looked at the ICU transfers, I think it was somewhere around
20 or 30 hours between the first alert and an ICU transfer. And that was actually what we tried to
design the model to do. So when we first were designing the tool, we were getting a lot of
feedback from the clinicians, especially we had clinicians design the care pathway for high risk
patients. What should a physician do? What should a nurse do when they receive a high risk
alert? And implementing that protocol itself I think is really important. And in some ways, the AI
tool is just a little wedge into allow standardization of care. And I think in some ways that's one
the benefits of even why news or those other early warning scores can be useful, even if
they're annoying, which is they create a language and a pathway for high risk patients like a
care standardization that tends to happen.
But so what we found was that clinicians said, our initial models were just predicting anytime in
hospital and the clinicians were saying, you're going to tell me a patient might die at any point
during this hospital stay. What am I going to do with that information? I'm just going to watch
them for how long? So they told us 24 to 48 hours was an actionable window. If you tell me this
event might happen in the next day or two, then I know what to do for one or two days. I will
clarify goals of care. I will monitor a patient more closely.
Dr. Mojola Omole:
And how does this differ from, let's pretend that burnout doesn't exist and we just all are
diligently watching our patients all the time. How does this come close to a physician who's
doing that in terms of the outcomes?
Dr. Amol Verma:
Well, I don't know if that situation ever existed where a single physician or nurse was able to
continuously every hour monitor all their patients at any one time, even before workload
capacity. Just because if you just think about how the history of medicine and how it's all
designed, the whole idea of rounding is that you go sequentially around to see your patients and
you just assess them each one in turn. And so I think the value of a tool like this is that it can
watch all 20 of your patients at the same time every hour and say, Hey, pay attention to this
person now. You know what I mean? And I don't think humans could, not for lack of
sophistication, for lack of intelligence, for lack of good intentions, or even because we're
overworked, just because our ability to do any more than one thing at a time is relatively
constrAIned. And so we will sequentially see your patients anyway. But to have a tool that can
say, I'm monitoring all hundred patients on a medical unit, I'm now anthropomorphizing a tool,
which I hate to do, but the computer is monitoring all a hundred patients on a unit and saying,
these are the ones to pay attention to and just prioritize them, right? It's like a triaging function.
Dr. Blair Bigham:
So first of all, this might be the first time we've heard the term anthropomorphized used on the
podcast, so kudos because one of my favorite words, but second, recently in the media sort of
like this unveiling that maybe the promise of AI isn't as grand as it was originally made out to be.
A lot of things where AI maybe isn't doing as good a job as we had hoped. Have you found any
areas where AI was either very clearly beneficial and better than humans through its ability to
constantly monitor such a high volume of cases? And similarly, did you find cases where
humans were definitely better than the score or the AI model at certain aspects of picking up
sick patients?
Dr. Amol Verma:
So I started developing this tool when I was in my last year of residency and started doing a
research fellowship. And so I was kind of a newbie and eager beaver. And so I brought the tool
to my colleagues in the general internal medicine division, and I said, Hey, we've developed this
prediction model that's pretty accurate at predicting death and deterioration on the wards. And
so obviously their response, which is the same response I would have now, was like, well, I
think I'm pretty accurate at predicting this. How do I know this tool is useful. And so to answer
that question, we sent a research assistant to the ward every day for four months to ask doctors
and nurses, which of your patients are going to deteriorate? We compared their predictions to..
Dr. Mojola Omole:
None.
Dr. Amol Verma:
Yeah, because you’re such a good doctor.
Dr. Mojola Omole:
I'm a surgeon. They don't do that.
Dr. Amol Verma:
I would never, as an internist, I would never have anything negative to say about a surgeon.
Dr. Mojola Omole:
You shouldn’t.
Dr. Amol Verma:
So we tested, it was an earlier version of the tool, but had very similar performance. And
we compared at the same time period, like the nurse prediction, a doctor's prediction, and the
model's prediction. And before I tell you the results, so to introduce a little suspense here. What
I want to say about the way we designed that study, which I am pleased about, is, and I think
actually it speaks to the hype of AI. To your point, a Blair, a lot of the early research comparing
AI performance and clinical performance was very unfair to the clinicians. I'll give you an
example. There was a huge dermatology study and it found that AI can detect skin cancer as
well as a physician. And the comparison though was physicians looking at pictures of skin and
computers looking at pictures of skin. But that's not how doctors work.
Like dermatologists, they take a history, they talk to the patient, they're able to assess a lesion in
three dimensions over time. They're able to use tools, different light microscopes, whatever
they're using. And so if you have this fake comparison of a doctor who is not the patient's doctor,
looking at a picture and comparing that to a computer looking at a picture, that's just not what
we really want to ask. And so that first generation of AI research did that and showed repeatedly
that AI was, and I think that was part of the hype cycle to be honest. So our study didn't do that.
We said, the doctors that are caring for the patients, the nurses that are caring for their patients
in real time, they are making that decision. This is a real comparison. And so what we found
was that the model performed about as well as the doctors and nurses in predicting death or
deterioration. That in itself is a technological feat. You have an AI model that is performing as
well as doctors or nurses, but in and of itself, it's not that compelling to doctors or nurses that
you should use this model that’s just as good as you.
But what we dug deeper and found was that the model was much better than doctors and
nurses at predicting ICU transfers. So it was not as good at predicting deaths in..
Dr. Blair Bigham:
In a short period, within 24 to 48 hours.
Dr. Amol Verma:
That was on that hospital stay, I think. And within 20 it was better on both time windows.
Dr. Blair Bigham:
Oh, okay.
Dr. Amol Verma:
And those are the very patients that we're trying to rescue that the unrecognized deterioration
issue happened. But perhaps the most interesting thing out of that study was that humans and
computers make different predictions. So the ways that the doctors were wrong were different
than the ways that the computer was wrong. But when we combined the predictions, the
combined predictions were more accurate than either prediction alone. So actually there are
cases where a doctor will recognize a deterioration event that the computer won't, and cases
where the computer will recognize and the doctor won't. And so it's really the collaboration, the
combined work of the two, that is where the opportunity lies. And to put a number on it, the
combined performance was 16% better at predicting, identifying a deterioration event, then the
clinicians alone without having more false positive alarms, without having more, which is what's
really fascinating about that approach.
Dr. Blair Bigham:
Can we go beyond your study and just sort of hypothesize about the future of medicine and
where do you see AI or augmented intelligence not taking over, but really bolstering that
decision-making safeguarding patients?
Dr. Amol Verma:
The first is that the prevailing discourse about AI replacing X, whatever X is…
Dr. Blair Bigham:
Radiologists.
Dr. Amol Verma:
That's right. Famously radiologists, according to the Jeffrey Hinton quote. But even outside of
medicine, we have to recognize that all of our healthcare providers, we all live in this the
broader context of AI. And so it is true that AI is going to displace some kinds of work. Now, how
that influences workers remains to be seen. Does it totally displace certain kinds of jobs? Does
it change other kinds of jobs, et cetera? I think we're not sure yet. And when we talk about
medicine and the various professions within medicine, I would say that it's really not clear to us
what the upper ceiling on the capabilities of AI will be projecting forward. And so I think it's unfair
for us to say AI won't replace clinicians. You hear that AI won't replace doctors. Doctors using AI
will replace doctors. I've said that before, but I actually don't think that's a fair thing to say
because I don't know what AI will and won't replace five years from now, 10 years from now.
Right? It's a very dynamic field. What we can say clearly today is that AI systems do not have
the reliability to autonomously make healthcare decisions in a safe way. They're just not reliable
enough today. And so with that in mind, any kind of AI tool, it's about augmenting human
performance, augmenting human intelligence, and augmenting the way we
work. Some of that might be offsetting some tasks like making it the scribe, reducing our need to
type or whatever the case might be, right? Other cases, it's helping us with prediction. Other
cases, it might be suggesting some treatments for a patient, or it might be suggesting a
diagnosis, telling a radiologist to look in a certain part of a scan. So one, I think my reflection is
there is no singular AI. These are all different kinds of tools and technologies, and their impact
remains a bit uncertain. But for today, it is absolutely true that they're not replacing any
individual. And we just really have to think about how do we use these technologies safely. And
importantly, part of that means how do we design them so that they support our workflows, or if
they are going to disrupt a workflow, it should be deliberate. And then we have to kind of design
for that.
Dr. Blair Bigham:
We don't want to make work project or add to..
Dr. Mojola Omole:
Imagine medicine making make work projects.
Dr. Blair Bigham:
Well, the whole promise, I've heard a lot of people say that the scribe technology, for
example, originally sounds great, but then they spend so much time going back and editing it,
just as an example. Or the scribe thing is either overly verbose or then they just have to read
more. So there's a lot of sort of critiques around, well, hang on. For me, I'm wondering, is this
just because it's so nascent, it's just new, we haven't nailed it down yet, or is this, no, AI is not
going to fix the problems that we think it's going to fix, but it could help us get from, I dunno,
98% to 99% accuracy, or maybe I'm being overly generous and maybe we're only 70% accurate
in a certAIn area of medicine, but it can get us to 82%. What do you think the big obstacles are
in realizing AI as hugely transformative instead of just an incremental improvement?
Dr. Amol Verma:
I mean, maybe the first challenge is to set that as the bar for success, right? Maybe it's not fair
that AI has to be hugely transformational. And I would say that if we think about the probability
distribution of where AI will lead us, meaning if we think about all the future scenarios, there's
definitely a world in which this technology does become so good that it produces a really reliable
scribe, that it provides certain kinds of things really well. I will say one framework that I have
found helpful for thinking about this is, if we think about AI as an information processing engine,
then certain kinds of tasks in medicine, like diagnosis, is very much about processing
information.
And you process that information with the information that's currently contained in, if you could
capture enough information, you get better diagnosis, for example, of the conditions that are
known.
So for example, and I think it's why radiology is a really use case that gets pointed on as AI
should do this very well, and in fact is doing it really well. If we look at where it's made the most
contributions and has been the most effective, it is within vision-based fields. All the information
that you need, or at least that is usually made for those decisions that a radiologist makes those
decisions off of is contained in the scan and the medical record in by and large, right? It is
logical that a computer would be very good at doing that task. On the other hand, if you think
about predicting future events, there is randomness in life and there is chance. And the solid
example of that that I have always found really strikes home is the notion of, if you think about
breast cancer, the vast majority of women who have breast cancer only have it in one breast.
But if you think about the genetics are the same, the environmental exposures are the same,
everything. Why is it that it was the left breast and not the right breast or vice versa? There's a
component of chance in life, right? So if you think about that, then predictive tasks for AI, it'll
never achieve perfect performance, right? So if I think about it that way, let's assume the best
case scenario for AI in the future, then it should be able to achieve perfect accuracy compared
to humans on diagnosis. It should be able to do that as well for an image, let's say. It will not
ever achieve perfect prediction of when someone is going to die because it's just not knowable.
So I mean, I dunno for me, when I think about will, how can AI be transformative? I don't think
it's the right way to start.
I think we should say today, what can we do with this technology? Which means incremental
improvement across a wide range of fields, and we need that in healthcare. And then two, how
do we shape this technology carefully so that unlike let's say, electronic medical records, which
introduced a lot of benefits at the expense of clinician burnout and harming the patient physician
relationship because it took our eyes and ears away from our patients and onto our screens,
how do we design these new AI tools so that they orient around the needs of the healthcare
providers and of the patients receiving care? And that to me is the task for today. Sure,
someone, some futurist and philosophers should be thinking about how can AI transform our
life? Great. But for nose to the coalface clinicians like myself and researchers, let's think about
how do we get incremental benefit out of the technologies we have today? And let's think about
how do we design the further development, testing of these technologies so that they meet the
needs that we really care about.
Dr. Blair Bigham:
That is a pretty inspiring way to focus in on how maybe all of us could open our minds to
integrating more with AI technology as it incrementally changes the way we go to work.
Dr. Mojola Omole:
Thank you so much for joining us today.
Dr. Blair Bigham:
Thank you. That was awesome.
Dr. Amol Verma:
Thanks for having me.
Dr. Mojola Omole:
Dr. Amol Verma is a general internist at St. Mike's Hospital in Toronto, and holds a Temerty
Professorship in AI Research and Education and Medicine at the University of Toronto. So Blair,
okay, the first takeaway is actually just admitting that I’m maybe not as smart as I think. I did not
know what CCRT stood for until literally today.
Dr. Blair Bigham:
Jola, you have called me when I'm on CCRT to come and respond to you
.
Dr. Mojola Omole:
I just called it CCRT. I didn't know it was called the critical care response team. I didn't know if
that's what it stood for.
Dr. Blair Bigham:
What do you think we do?
Dr. Mojola Omole:
I don't know. I just, just C-C-R-T, C-C-R-T, C-C-R-T. I never thought of exactly what it meant.
Dr. Blair Bigham:
I think, you know what though, probably because all these early warning scores
and your score is high, this and that. Call CCRT people go, yeah, yeah, yeah, whatever. They're
not overly accurate. And so I think it gets lost in the mix, but it sounds like this is the next wave
of early warning scores. A much more advanced way of more accurately predicting who might
need CCRT response on the wards. And maybe we can prevent an ICU admission, or at least
get to them in the ICU before they totally crash and end up being a code blue or something like
that.
Dr. Mojola Omole:
For sure. And I think what kept on coming to my mind is this concept that AI will replace us. Did
people feel this way when CT scans came out? I remember when I was in, I worked in
sub-Saharan Africa, I think it was in Rwanda. One of the older surgeons was like, this is called
the Auscultation Splash. I was like, what? And basically it's like you hear a swishing sound in
the left upper quadrant, which shows that you have a gastric outlet obstruction. I was like, okay.
Exactly. So a long time ago, before CT scans or in places where there's no CT scans, you rely
on those clinical tools. But now I just examine them and then I say, okay, you need a CT scan.
Right?
Dr. Blair Bigham:
Well, let me throw this at you though. Hang on a second though. If we're, let's just say we're
CT-ing everybody. Are we actually better physicians? Are we losing clinical skills by not being
able to do a physical exam and make a diagnosis? I guess my question is not, do you need to
CT for cholecystitis? But can AI actually take over some of those skills that we’ll then lose? And
will we become worse diagnosticians without AI? What if in the future there's a giant
electromagnetic pulse and AI disappears? Will we have lost the skill of medicine?
Dr. Mojola Omole:
I literally dunno what an electromagnetic pulse is. Sounds like something that happens in Star
Trek.
Dr. Blair Bigham:
Oh come on, you don’t watch movies?
Dr. Mojola Omole:
No, I don't watch Star Trek. I don't watch Star Wars.
Dr. Blair Bigham:
Let's just say AI disappeared. There was a copyright infringement, or the Supreme Court said it
was illegal. I dunno, let's say there's no more AI anymore, but everyone's dependent on it to
close that…
Dr. Mojola Omole:
No, for me, it's like I view it as a tool. It is just another thing to, it's another set of
information that I get to process, but at the end of the day, I'm still the one who's going to make
a clinical decision and then I offer that to the patients. What would you like to do? So I view it as
just another tool that I get to use to make a decision of what is the next course of action for this
Patient.
Dr. Blair Bigham:
Well, you bring up an interesting point about how patients will react to this type of information
and whether they will trust AI as much as we might, or less than we might. Or maybe they'll trust
it more than they trust us. But I think we are running out of time, and that'll be for another
Episode.
Dr. Mojola Omole:
So that's it for this episode or the CMAJ podcast. If you like what you heard, please give us not
a five star, at least a 10 star rating wherever you get your podcasts. It only takes a couple of
clicks, but really helps us a lot. Share it within your networks and leave a comment. I've been
told that some people listen to our voice to fall asleep. That's still okay. As long as you download
it, it counts.
Dr. Blair Bigham:
Do we get a download each time they repeat it? Well, that would be good.
Dr. Mojola Omole:
Exactly, so just listen to us as you fall asleep.
Dr. Blair Bigham:
Eight hours on rerun.
Dr. Mojola Omole:
Doesn't hurt my feelings. The CMAJ podcast is produced for CMAJ by PodCraft Productions.
Thank you so much for listening. I'm Mojola Omole.
Dr. Blair Bigham:
And I'm Blair Bigham.
Dr. Mojola Omole:
Until next time, be well.
Dr. Blair Bigham:
Be well.