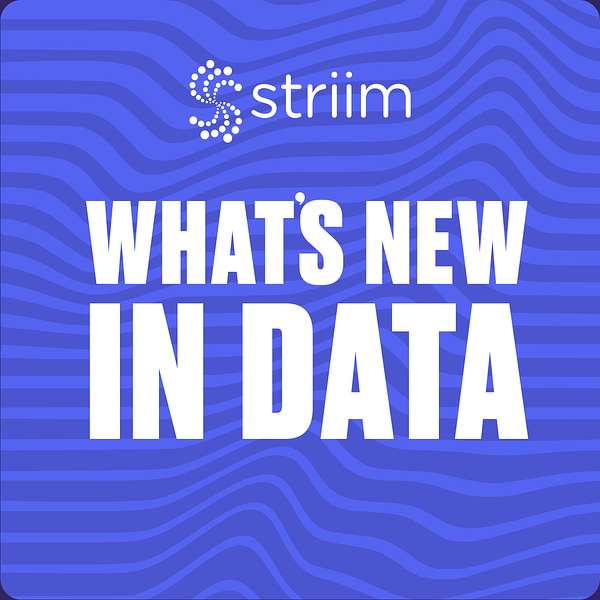
What's New In Data
A podcast by Striim (pronounced 'Stream') that covers the latest trends and news in data, cloud computing, data streaming, and analytics.
What's New In Data
Microsoft Fabric vs Databricks. Should Databricks be worried? Fabric vs Databricks pricing differences.
Ever ask yourself how to choose between Microsoft Fabric and Databricks for your enterprise data workloads on Azure? Join this discussion with cloud pricing and cost optimization expert Everett Berry from Vantage.sh as he illuminates the differences between these two powerful data lake technologies. We delve into the depths of their unique features, pricing models, and deep integration with Azure.
Our conversation ventures into the world of AI and its transformative impact on the modern data stack. Everett offers brilliant insights into how data teams are redefining their strategies to prioritize AI in their roadmaps.
About Everett:
Everett is Head of Growth at Vantage.sh. He is known for creating one of the most widely used indexes of cloud infrastructure costs at Vantage Instances.
Follow Everett Berry on X (formerly known as Twitter)
Everett's original article on this topic: Microsoft Fabric: Should Databricks be Worried?
What's New In Data is a data thought leadership series hosted by John Kutay who leads data and products at Striim. What's New In Data hosts industry practitioners to discuss latest trends, common patterns for real world data patterns, and analytics success stories.
Hi everybody. Thank you for tuning into today's episode of what's New in Data. Super excited about our guests today we have Everett Berry from Vantagesh Everett how are you doing today?
Speaker 2:Doing great. John, Thanks for having me on the show.
Speaker 1:Absolutely, absolutely Everett. Tell the listeners a bit about yourself.
Speaker 2:Sure, so I'm the head of growth advantage, started out as an engineer about a decade ago working on AI and actually computer written products, and from there I've sort of transitioned more into the growth side and developer relations side and the sort of one of the main things I do for Vantage is maintain this site called EC2 instancesinfo, which is a sort of widely used Amazon pricing comparison site spree and open source. And then when I'm not digging into Amazon pricing weirdness, I am doing often analyses of kind of interesting things happening in the data infrastructure world and so sort of the genesis of the show, which is Databricks and some interesting stuff that Microsoft has been pushing out, is one of those items.
Speaker 1:Excellent and just a testament to your work. I mean I first discovered your work on EC2 pricing when I was just individually searching for the price of the instance and equivalent Intel instance on AWS and your work was the first search result that actually gave me the answer like very quickly, right, If I went through AWS' website there's a little bit extra clicking, you have to go through a little bit of marketing on it on top. But I thought that your work there was just super easy to sift through and do comparisons and very discoverable as well. So definitely props to you and I'm sure everyone's come across your EC2 pricing page as well.
Speaker 2:Yeah, I appreciate you saying that, and actually the page has been around for almost 12 years at this point, so it was really developed by the community of AWS developers back in the day, because there's not too many places where you can get the specs of the instance alongside the pricing of it. But I've been it's really been a great privilege to take that over and maintain that for folks and hopefully improve it as well. So, yeah, I appreciate both. A shout out.
Speaker 1:Yeah, definitely, definitely. So everyone appreciates that page. On the AWS instance, it's great to hear it's open source as well and the community is helping maintain it. The reason you're on the pod today is you have a super awesome blog that's making the rounds. It's titled Microsoft Fabric Should Databricks Be Worried, and it's essentially diving into a comparison between Microsoft and the Databricks and wanted you to break down that topic a bit more, but at a high level. Can you just describe Databricks and Microsoft Fabric for the listeners?
Speaker 2:Yeah, absolutely so.
Speaker 2:Databricks really got its start as a managed Apache Spark provider and there's sort of very many layers to this.
Speaker 2:But Apache Spark is a modern version of like a Hadoop workflow, where you have a ton of data that you're trying to process and you distribute the processing which, let's say, it's like a complicated SQL query.
Speaker 2:You distribute that out over a cluster of compute notes and Apache Spark orchestrates which nodes to do what were, how the results should get combined and so forth, and that way of doing things was pioneered, kind of, you might say, like 2008, 2010 area, and was made open source as an Apache project. But actually managing the infrastructure in order to do that was pretty challenging, and so Databricks is a commercial version or really started as a commercial version of Apache Spark, and now it does an enormous number of things. It has notebook features, it has BI reporting, it is increasingly known for its machine learning capabilities and I think OpenAI recently talked about their massive Spark cluster. So I don't know if they're Databricks customer or not, but Databricks has kind of become a go-to place for people to run very large data workloads and has a lot of enterprise penetration and it's often compared to although not always correctly. It's often compared to Snowflake as being a place where enterprises can run very large queries and data tasks.
Speaker 1:Absolutely. That's a great overview. Certainly a lot of enterprise representation of Databricks users. I did present a data and AI summit with American Airlines, for instance, and they power a lot of core operations through it and there's so many examples of that. And one other interesting thing is that Databricks is an Azure first-party service, so there's Azure Databricks which runs in Azure, but it's also sold by Microsoft, which is interesting, and you break down that part in your blog as well. So, now that we know a bit about Databricks, what is Microsoft Fabric?
Speaker 2:Yeah, and just to hit on the relationship between Databricks and Microsoft, because it powers a little bit of the entry of the post, databricks is and Microsoft have one of the deepest integrations that I have seen between a cloud infrastructure provider and a cloud service. So when you are on the Databricks doc page and you click over to Azure pricing, it actually redirects you to the Microsoft docs page for Databricks and, similarly, the company at Work for Advantage. We have a Databricks cost integration and in order to access Databricks costs, you have to connect Azure cost management APIs and so this is very unique. In AWS, for example, to access Databricks costs, you kind of access, say, s3 bucket that is provisioned by Databricks. So Databricks and Azure have this very deep integration and very long history and some of the intrigue of the post is that Microsoft has this, in my view, launched what amounts to a direct competitor now to Databricks, so basically offering that Azure users could consider if they were trying to do basically Apache, spark or kind of Databricks style workloads, and it works very similarly to Databricks.
Speaker 2:That has some of the Lakehouse features that we can talk about that Databricks has. It provides a unified computer runtime across notebooks, across SQL queries, and it integrates Power BI and Synapse, which, for folks that have been in the Microsoft ecosystem for a long time, most likely those are the tools they're using for a lot of their data needs, and the gist of the post is that Microsoft has enabled their users to have a Databricks style experience. There's a lot of technicality there. In many ways, the experience is degraded from what Databricks offers. It doesn't have a lot of the same machine learning features and it has a much different end. Could be better or worse depending on your point of view pricing model, but the other long and short of it is. I Do believe there's a real choice now between Azure Databricks and Microsoft Fabric and and it's Rising in some ways to see that just because that partnership has been so deep for so long.
Speaker 1:Yeah, and just to dive into that point, for those of you who are Watching this on YouTube, you'll see the feature comparison, but those of you listening on a podcast, we're pulling up ever its blog here and Essentially it's a feature matrix. Here where you know, there's a list of about what is it? One, two, three, four, about ten or so features, and fabric has all but two of them. Right, if you go down SQL, python, data science, notebooks, managed Spark, data engineering, serverless, sql, ml, flow at a high level, databricks and fabric both support both the ones that Databricks has that fabric does not have at this point, or just dealt the live tables and models serving. So this just really illustrates what you're describing here and this is why you're saying that fabric can be a competitor to Databricks.
Speaker 2:Yeah, that's right I there was some discussion on LinkedIn about the actual spark implementation and this is kind of an interesting side topic where Databricks has all sorts of of optimizations on top of spark, including this thing called botan, which is sort of an optimized runtime that specifically executes on like vectorized processors, like Intel AVX, that that Microsoft does not currently offer. But I do think, like at a surface level, that the two services are are directly similar and what it may come down to is really just like quality of product offering. I think there are a lot of doubts that Microsoft, in the way that they kind of cobbled together power BI and Synapse and An Azure one Lake, they're calling it into this one platform whether they can actually deliver that kind of same. You know unified powerful experience that Databricks users currently have. But Our experience at at build, which is Microsoft's one of their main developer conferences where fabric was announced, was that within the Azure ecosystem those folks may not, may not care as much that some of the workloads, some of the more advanced stuff like Delta live tables, are supported or not if they get access to this Databricks style way of doing things, and so it.
Speaker 2:To me it's. It's a real question, and you might even compared to and I don't want to go too far, but when Microsoft launched teams, that really did eat into a lot of slacks, market share and, and so it's Possible that a similar thing could happen here. That said, the conclusion of the post is that when you look under the hood a little bit to each implementation and what features are available, databricks, I think, is a pretty clear winner, but just the fact that the the data but style way of doing things is now available in a native Microsoft fashion Is a big step forward and and maybe enough to win customers. Otherwise, databricks would kind of have to themselves Fully, and there's always a nuance and enterprise software.
Speaker 1:I mean, I could take two products that seem like a total Apple to Apple's. Comparison turns out there like for different use cases, and you know, I think companies always have to directly evaluate which one is best for them. But another part that you dive into in your blog posts, and which is actually a pretty Big topic, is the pricing. So how does the pricing of Databricks compare to Microsoft Fabric? Yeah, so in both cases.
Speaker 2:There's really not a great way to estimate the costs ahead of time. Apache Spark, just by nature of its execution, is it's sort of up to the runtime, how long things will take, what gets executed and so forth. What Microsoft has done is combined all the pricing related to Infrastructure needed with one exception, which is storage. But they combined all this into a SAS model where there's tiers of capacity that you can reserve, so you can reserve a certain number of what they what they call fabric SKUs, which is basically an amount of compute that can be used for your job. So let's say, you know you're, you have a SQL query, it runs for an hour, maybe it consumes, like you know, 48 fabrics use or something, and that's and that's charged In a.
Speaker 2:In a SAS consumption based model, databricks has a extremely different pricing model, which is Databricks deploys within your own cloud infrastructure. So as a user, I pay for the instances or the bn's that I'm running, I pay for the Networking costs and other infrastructure associated with that, and then I have a dvu cost, which is a a Databricks Management fee, if you will their their fee for kind of orchestrating this, the SQL query that I that I pay directly to Databricks. And so Microsoft's argument is that their pricing is Simpler. It's it's one tier. You kind of scale up and down. What we're going to do is we're going to scale up and down what.
Speaker 2:What we believe to be true is that it may actually be too simple in some ways. In fact, some interesting quotes from Microsoft's documentation on fabric where they say you know that the best way to understand your costs are to just like run some workloads and estimate from there. We don't have a good calculator, we don't have a good way to tell ahead of time which which tiers are going to be in. And so it's my belief that actually many data teams would prefer to have a greater degree of control over which infrastructure is used and and kind of where the costs go, which database provides. But Again, like if I'm an azure user, I may appreciate kind of the simplicity of the fabric model, but it is one of those models where I would say surprise costs you know, surprise bills are are potentially more of a concern than than in data bricks case.
Speaker 1:Makes sense and you know we're For those of you on youtube you see that we're sharing it, but on the podcast You'll just have to listen. In Databricks, as you mentioned, ever, it does have this granular cost per dbu. On azure Across all these services right, like model serving data, brick, sequel, jobs, compute, etc. So, like you said, it's more flexible and gives you a little bit more control Around. You know what services you want to use and you can do some more fine-grained cost optimization, whereas an azure fabric they're. They're just giving you these, these skews, right, and the skews sound like they have all the features included and they should tell you how many CUs you have power, bi v cores, spark cores, all that's kind of thrown in with a flat hourly cost.
Speaker 1:But it's up to the user to try it with their workloads and see, you know, is it meeting the, the performance and data latency requirements which I've evaluated a lot of cloud software as well, and ultimately that's. I think that's pretty standard now. But you know I've done evaluations of several cloud data warehouses and everything that we did with like a self-serve compute model. You know the costs do change over time, over adoption. It's hard for teams to estimate like the one to three year usage. With these you know cloud usage based pricing models and every year you sort of have to course correct, renegotiate and see what's actually being deployed.
Speaker 1:Now, if we were to just simplify this for the, for people listening, would you say that okay, between the two, who has the simpler pricing model?
Speaker 2:So it's a harder question to answer. On the surface Fabric is simpler because there's a set of tiers and everything is combined into one. In my view that makes it actually more difficult because for example, with Databricks, if I'm running compute jobs that I know that that is separated out from the Delta Live tables jobs I'm running, or I know that it's separated out from notebooks. With Fabric you can have users. You have a data scientist who maybe has notebooks that they're running that contain these complex queries. You might have a whole separate team or set of users that is running kind of individual analytical queries or Python jobs or machine learning jobs. All that is mixed into one.
Speaker 2:And so if I'm sitting at the Fabric console then certainly I'm just seeing kind of one cost that's coming through and for some teams that might be preferred. But it's a mixture of everything and so there's less of an ability, which is in that Databricks offers, where you can say look, 60% of our costs are machine learning, 30% are data science and 10% are model serving. I can kind of go in and further optimize, I can change the instances and so forth. So Fabric's combination of everything into one set of SKUs and one kind of tiered pricing system is simpler if, at the end of the day, that is the preference of the company. But my view, kind of living in a cost optimization and cost visibility world, I tend to prefer a Databricks model where different types of workloads have different pricing and there's greater granular control over which instances and what infrastructure is used to run that stuff.
Speaker 1:It totally makes sense. The flexibility is always going to be something you can leverage to tune your costs over time. Now, so that's comparing the pricing models, but just what about the overall costs? Which one do you expect to be lower if you were to pick one or the other?
Speaker 2:So it's again hard to say. I do think that many Azure shops will find that Fabric's probably offers savings versus Databricks. There's a couple reasons this is the case. One main one is that you can use your Power BI premium capacity, which is a little bit of the previous model of running Power BI jobs. You can use that to pay for Fabric and so, and compared to those capacity reservations, fabric is actually more of a finer grained consumption based model.
Speaker 2:So for teams that maybe are ending every year with leftover Power BI capacity or have a lot of unused capacity for their existing Microsoft workloads, I actually do think they will get a lot of value out of Fabric and probably see cost efficiencies that as a result.
Speaker 2:Another example of a Microsoft or Azure user that would be dealing with that would be a team that's running multiple different types of Synapse workloads, and Synapse would be kind of the Azure data warehouse solution alongside Power BI.
Speaker 2:So those teams probably shouldn't see some good savings too. The folks that are going to see it cheaper, more cost efficient workloads on Databricks are people with an ability to optimize the jobs that they're running and perhaps change the instance types that they're on, perhaps really control the amount of data that's used. So teams with kind of a detailed understanding of what their workloads are and maybe perhaps less of a inclination to run a lot of experiments, are going to see cost savings on Databricks. So yeah, that point is one reason why I do see this as a little bit of a threat to Databricks, where otherwise they might be able to win some Azure workload. They're not going to be competing with teams who are saying, well, if we migrate the Synapse and Power BI things that we're doing on the Fabric, we're actually going to see cost savings and we don't have to change the existing tool set that we're employing.
Speaker 1:Do you see a case for using both Azure Databricks and Microsoft Fabric?
Speaker 2:So Microsoft says yes. They have a couple of blog posts where they are talking about using Fabric with Databricks, and there's one case in particular that they highlight, which is where you have data that's kind of siloed between your different tools. So one great feature of Fabric is that it combines the data storage from Power BI, from Azure Blob Storage, from other data lake solutions available on Azure, into one unified pool, and you can connect that pool directly to Databricks. And so the use case that Microsoft talked about, which seems very legitimate to me, is that, by unifying your existing Azure data workloads inside Fabric, you can then connect all that data to Databricks and use Databricks features to practice on it to run workloads on it.
Speaker 2:So that makes sense to me. Outside of the kind of unified one lake situation, I'm not sure that a lot of teams would use both. I'll make one exception to that, which is the machine learning side of things. It definitely appears that Databricks has a strong lead here, and I would think a team that has kind of a continuous data pipeline or maybe they're continuously training new models, they would want to employ Databricks and they may end up using Fabric for more of a traditional data warehousing solution.
Speaker 1:It definitely makes sense and data bricks itself. There's no question. It's a platform in a true sense, where it's very flexible. You can do general purpose data processing, compute, etl. Machine learning Fabric has Microsoft Fabric is also a platform. It does, with the Power BI aspect, seem like it's closer to the business intelligence side. Maybe it's business intelligence plus, where it's including ETL and simplifying a lot of the workflows. With the BI use cases in mind, would you say that's a good assessment. Yeah, absolutely.
Speaker 2:Power BI is strongly there and actually this is an area where data bricks is punching back, if you will. So their most recent major announcement, in my view, was an expanded set of BI capabilities and dashboarding capabilities, and in some ways those features make data bricks look a little bit more like a tableau, just looking at the UI for it. So yeah, short answer is yes. The people moving from a more traditional BI and reporting place into a more modern distributed processing spark place, going through Fabric to do that transition, seems very logical to me. There's. It'd be interesting to actually know from data bricks how they kind of sell against Fabric in that scenario, but that does seem to be how Microsoft has positioned.
Speaker 1:Totally makes sense. And I, who would you say? The real winner is from the fact that Microsoft launched Fabric. It's Microsoft.
Speaker 2:The thing to appreciate with even Azure data bricks is that the infrastructure to run data bricks is being run in the customer's account, and so that's generating Azure costs and revenue for Azure, even while that's happening.
Speaker 2:With Fabric, not only is the infrastructure being run in the customer's account although you don't see that in the pricing model but also the DBU that the data bricks fee is also going to Microsoft, and so there's no doubt that this is a competitive offering. It's something that I think data bricks should be a little, you know, at least look closely at. I don't necessarily expect Microsoft to win a lot of bake offs with data bricks right now, but over time, if they keep investing in the platform and they have sort of an entire microsite and, instead of developer relations, people dedicated to it, you know, I think it does pose a real, a real threat, and I also think it may help Microsoft retain users who, ultimately, were going to be pulled to data bricks, just because the data bricks way of doing things, which Fabric is mimicking, does seem to be kind of the new way of the world for these larger enterprise data stacks.
Speaker 1:Totally makes sense. And the other thing that I'm seeing is, you know, in the year of 2023, it's all about efficiency, Whereas in 2019, 2020, we were kind of talking about the growth of the modern data stack and implementing the modern data stack. What are all the tools you should adopt? So would you say. You know, right now we're on this wave of cost reduction across.
Speaker 2:I, yes, and the quarterly numbers from the major cloud providers, which we also do some analysis on, and these cloud cost reports, seem to corroborate that. I think the modern data stack in particular is going through its own kind of mini optimization wave, and the same way that cloud infrastructure in general is going through that. I think data bricks actually may be more immune to that than a solution like Snowflake, because data bricks offers customers a lot of flexibility around how the workloads get run and which instances are chosen and so forth. But still you have the situation where Microsoft's main, one of their main selling points for fabric is it's a more cost effective way to operate data workloads on Azure. So, yeah, the short answer is yes, I don't think we're seeing as much of an expansion of the modern data stack suite of tools as we were.
Speaker 2:That said, there's one kind of counter trend to this, which is the emergence of AI. Every AI workload is powered by huge amounts of data, particularly data that needs to be trained on and changed a lot, and so if there's kind of a one maybe saving grace for the modern data stack on the next year or two, it's just the fact that clean data is almost everything with these models and data bricks seems very well positioned for that, and they acquired, of course, mosaic ML, which is part of that story. So, in general, traditional workloads optimization is king, but AI may be kind of a counter trend that lets the party continue, if you will.
Speaker 1:Absolutely. Cost reduction is one area, but AI is another big category that's getting a lot of attention, a lot of investment. I've seen this as well, where data teams are being asked to prioritize AI in their short to medium term roadmaps. There's all these questions now in enterprises who owns the AI? Is it going to be its own separate function?
Speaker 1:Data teams are saying look, whoever you bring in for AI has to rely on us to actually get the data and to actually operationalize it with capturing the embeddings, launching the vector databases, whatever it is serving the models, et cetera. A lot of data teams are viewed as adjacent to the work they're doing and adopting AI as more of an evolution rather than a revolution, and extending their functions. I think cost reduction comes into play there too, because if you're spending so much money just on ETL, just processing data, just with high price compute, where's the budget for AI going to come from? Yeah, seeing a lot of teams optimize compute on some of the more basic data transformation tasks, so you can actually invest in AI, which is its own significant area, both in terms of people and the underlying cloud infrastructure that you have to deploy.
Speaker 2:It's kind of a budget question, right?
Speaker 2:If I'm doing my 2024 budgeting, which many people are doing right at this moment, do I just have a budget category that's for AI and, as a data practitioner, maybe I can sneak in some budget for data tools into that category, even if those tools serve other functions of the business.
Speaker 2:I think it's actually a great opportunity for teams to in some ways reset and remake the arguments. There's no doubt that, especially if you believe the foundation model idea, that many companies will rely on the data they have more than their ability to invent new types of transformers. In that sense, the data teams and the work that they do with these tools is going to be the key, maybe even more so than necessarily the types of work that maybe a machine learning researcher at OpenAI would be doing, which would be a little bit more focused on the mechanics of the model versus the data that is provided to it. My sense is that a lot of companies will, in fact, just make investing in AI a priority, and that budget should trickle down into the data teams and give them that they play such a primary role.
Speaker 1:Absolutely, everett. This is an area that you work in heavily. Vantage is, of course, a leader in cloud savings and automating those savings. What's your general advice to data teams on FinOps and making sure that they're within the confines of their budget but still hitting their actual goals in terms of business and data projects?
Speaker 2:We have a couple of tools that look at this. One that's popular is top snowflake queries by cost On the data side. Really, the key is that we're seeing is more around visibility and cost allocation. Data teams have a lot of internal customers typically and those internal customers generate different levels of costs. You can imagine the revenue reporting team or the folks dealing with customer data requests. They may generate an outside share of costs versus other internal holders. I think the biggest thing is actually just being able to say look, we have a six-figure spend with snowflake or data bricks this year and portions of that are being allocated to these stakeholders.
Speaker 2:Generally, I've seen the argument made where if a certain stakeholder or leadership-style person is using up a lot of that data budget, they're going to be pretty okay with maintaining or expanding it, versus maybe the idea that the data team is just doing all these experiments that are generated in cost and there's no direct tieback. I think there are tools out there and we have some of them around optimizing the operation of the warehouse or of the data bricks installation. The number one thing that I've seen be successful for teams is just having greater visibility and allocation into where that spend is going. Then typically there's a clear ROI argument to make. There's a case that you really are advancing the business via this contract and your snowflake budget might be this, but the results you've generated are 10 times this. We stress more on the allocation and visibility side, but it is an interesting question. Actually, the fabrics and data bricks piece gets into this of. Maybe I do have more ability to optimize my infrastructure with data bricks versus fabric and therefore that's a decision point and which tool I go with.
Speaker 1:Is there anything exciting that you're working at advantage that you'd be willing to share with the listeners?
Speaker 2:Yeah, I appreciate the question. The major initiative we've got rolling this sort of wrap up 2023 is in the culture synopsis code. This is a set of tools and techniques to automate the process of reporting on costs and allocating costs across the organization. The brass tax tactical explanation of what it is is a set of terraform modules which allow you to provision the cost reporting in the same way that you provision your main infrastructure For example, spinning up a new data service for a new application and right next to the terraform that provisions that infrastructure. There's terraform that actually creates cost reports and vantage and creates things like tagging and filters alongside the infrastructure being deployed.
Speaker 2:In general, this is actually one of the main challenges of FinOps is just keeping the reporting up to date with the changes that are happening across the company's infrastructure. Imagine a scenario where there's a thousand or more engineers and there's things being spun up and down every day. Generally, infrastructure is code, be it terraform or Pulumi or CDK is a way that companies manage that. We're trying to bring some of the FinOps workloads that exist in vantage directly into those modules so that teams can automate the bookkeeping that's associated with keeping their cost up to date.
Speaker 2:In general, I think this is exciting for FinOps practitioners too, because it means that they can spend less time chasing down tagging and trying to figure out just what's happening in the infrastructure and more time on the actual optimization and executive level reporting tasks that they have to handle. We're very excited about that. It's also cool to me and I haven't dealt with a ton of products where you can directly provision UI elements of the product from terraform the way you can with this spin-off as code thing. I'm really excited to see that hopefully spread throughout the industry and in some ways modernize the it might be like chores and a little bit of the treasury of FinOps and automate big swaths of that so people can spend most of their time on the value generation side, which is definitely an optimization, and then allocation of costs and things like margins and showing back ROI and things like that.
Speaker 1:Excellent. Yeah, that is super exciting and sounds like it really simplifies and gives a lot of flexibility into the cost savings process there for data and ops teams. Everett Berry growth at Vantagesh Great having you on the show today. Where can people follow along with your work?
Speaker 2:Yes, I'm on Twitter, as many of us are. It's at RedX with 3Ts. I'm on Twitter. This particular post did well on LinkedIn too, so you can find me on there. In general, I spend most of my days chatting or conversing about cloud infrastructure and really get a lot of joy out of that. I would love to keep the conversation going with anyone who's interested.
Speaker 1:Excellent Everett. Thanks so much for graciously sharing your insights through your blog posts and hopping on the podcast today, and thank you to everyone who tuned in.
Speaker 2:Thanks, John.