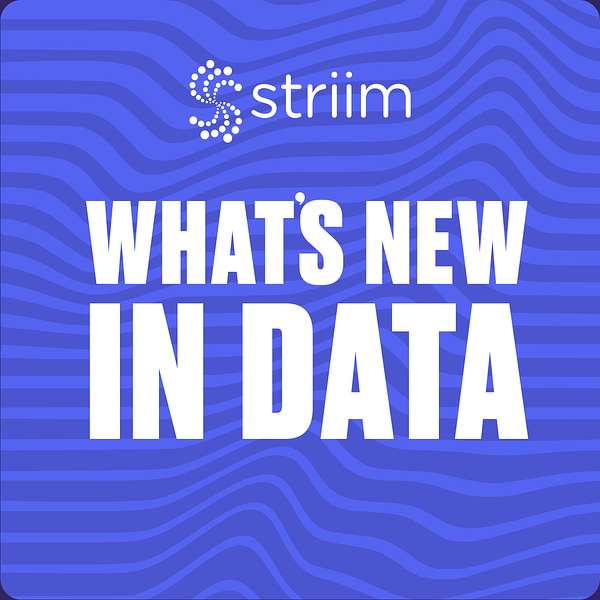
What's New In Data
A podcast by Striim (pronounced 'Stream') that covers the latest trends and news in data, cloud computing, data streaming, and analytics.
What's New In Data
Causal Artificial Intelligence, potential AI pitfalls, getting executive buy-in
John K Thompson is co-author of "Causal Artificial Intelligence: The Next Step in Effective Business AI" and Global Head of Artificial Intelligence (AI) at EY.
John's career path went from being an assembler programmer, to creating the first neural network utility at IBM, and now running the AI group at Ernst & Young. We'll unfold the pages of his acclaimed book, Causal Artificial Intelligence, and gain insights into his fascinating writing process. A relentless seeker of the 'why' behind data and analytics, John's insights are sure to fuel your curiosity.
Fasten your seat belts as we navigate through the multifaceted world of artificial intelligence. With the rise of AI, we are looking at a portfolio approach, focusing on several types such as generative and causal AI. Understand how these AI types generate context-specific responses, and the role of retrieval augmented generation in enhancing AI models. We'll also uncover how John masterly built a production generative AI infrastructure for UI, and some smart ways to sidestep pitfalls while implementing AI.
We examine how AI can be a game changer for businesses. John delivers invaluable advice on team collaboration, secure data management, and the crucial link between data, analytics, and measurable business outcomes. In an era where AI is revolutionizing industries, John's practical insights are the compass you need to chart a successful course.
Check out John's book on Amazon: Causal Artificial Intelligence: The Next Step in Effective Business AI
Follow John K Thompson on LinkedIn
What's New In Data is a data thought leadership series hosted by John Kutay who leads data and products at Striim. What's New In Data hosts industry practitioners to discuss latest trends, common patterns for real world data patterns, and analytics success stories.
Hello, thank you to everyone tuning in today on today's episode of what's New in Data. Very excited about our guest today we have John K Thompson. John, how are you doing today? I'm?
Speaker 2:awesome man. I'm really having a great day. How are you, john?
Speaker 1:Likewise, likewise. This is my second pod of the day. I was just on appearing on someone else's pod today Lauren Ballek and Mary's show, tech Bros, which is fun. But now I get to be on the other side and have you on the what's New in Data podcast. I'm super excited about that. So, john, you're the author of Cosal Artificial Intelligence super popular book. It's back ordered on Amazon. It's just been a huge craze. But first tell the listeners a bit about yourself.
Speaker 2:Sure, Absolutely. I just absolutely love data and analytics. I've been doing this for 37 years now. When I talk to people, they're like hey, what are you thinking about? The next phase? I'm like another book, more innovation, data analytics, just going for it and a lot of people are like wow, dude, you're way over the top on this, but it's what I really enjoy doing.
Speaker 2:I've been doing it for decades. I started out as an assembler programmer way back when, building systems for large corporations, and I kind of thought what's the point? I really felt even way back when the data was the source of the center of everything and analyzing data and understanding data was the place to be. So I spent my career doing that. I worked at IBM when we wrote the first neural network utility. I ran the advanced analytics division of Dell. In the last four and a half years, I ran the AI group of CSL Bering, which is the second largest biopharmaceutical company in the world.
Speaker 2:So my career has been split into two halves. I've either been building the technology that we've used for analytics and day mining and artificial intelligence, or I've been on the other side of being a practitioner. So a lot of people are like why did you do that? Why didn't you just stay on one side or the other? And I really love innovation, I love building software, but I really felt that if I couldn't actually do it myself, then I was kind of a poser. So I really wanted to be able to make a difference in using the technology and the data and making businesses more efficient and effective.
Speaker 1:Excellent, and I think having the practitioner's perspective on top of everything just adds so much knowledge, and being in the weeds of things is so much different than simply talking about stuff, which is why your book is so popular and has a real boots on the ground perspective, along with the market facing futuristic lens on it, so that's a super exciting frontier for you.
Speaker 2:Yeah, it really is. It was one of those things that I've always been looking forward. I've always been trying to think of what is the next thing, when can we get to? And causal AI just really seemed to be a topic that was coming, that it's going to arrive. And when we started talking to the publisher Wiley published this book and we were talking to the acquisition editor and bringing up causal AI, there was a lot of skepticism in the dialogue. They were taking the position that, hey, this is a research thing, this is an academic thing. People are just dabbling in it. And I said, look, by the time we write the book and we get it edited, we get it published and printed, we're going to be on the cusp of this thing. So luckily they agreed and went with it. So here we are with a brand new, exciting book in the market.
Speaker 1:Absolutely Tell the listeners the story behind writing this book.
Speaker 2:Absolutely. It's one of those things that I always go back. We always think of ourselves as earlier phases in our career, different personas. I think of myself as a lapsed product manager, so I always look at things through the lens of what a product looks like. But even earlier than that, my father had an auto repair shop and he always had me go to work early in the morning, really early in the morning, before school, and then I go home and clean up and I go to school and then I come home and work more, so that kind of set me on a certain path in my life.
Speaker 2:So I always get up very early in the morning, sometimes three, four in the morning. I don't write early in the morning before I start my day job. So it sounds kind of airy-fairy and a little odd, but I think about what I want to write just before I go to bed. It's the last thing I think of. So I think that I'm writing while I'm sleeping. So what I do is I get up in the morning and I channel what I've written. So that's the writing process. That's how I do it.
Speaker 2:But the idea for causal AI came to me because I really felt that there was more to AI than what we were seeing. We started writing this book before gender to AI exploded, and I've been doing traditional AI for multiple decades, so I really felt the why, understanding why things happened, why people did what they do and why processes unfold the way they do and what were the real causal activities that led to the results that we see. So it was a product of my curiosity and my co-author, judith Hurwitz, and I were talking and she said we really should write this book and I said, god, this is funny. I've been thinking about this for a while. So we just jumped into it and did it.
Speaker 1:That's amazing and it's so interesting to hear how your life and your upbringing waking up very early played a part in you having this time to yourself in the mornings where you could sort of meditate and just write about things you see at a very high level, cause I noticed as well in my day job once that nine to five starts, those slacks are firing, the emails are firing, you got everything on your calendar.
Speaker 1:You can't think clearly, right, you're just selling from thing to thing, right? So having that extra time to just sit, meditate, write and you really were able to look into the future, right, as you were mentioning, even your publisher was saying this causal artificial intelligence seems so pie in the sky. Does anyone do AI? But now look at us here in 2023, where you have to have AI on your short-term roadmap, not just your three-year, five-year roadmap. We recently had a panel what's New in Data Live in San Francisco with Bruno Ziza from Capital G and Sanji Mohan, a Gartner background, and that was the question like, should people be looking at AI in the future or now? And the consensus is data teams have to do it now or someone else is gonna be brought in to do it and that person who comes in, where they have to get the data to do.
Speaker 1:Ai you the data team. So yeah, absolutely so. It's extremely critical, which is why your book is almost perfect timing, and I wanted to ask you another question. So, in your words, there's three pillars of artificial intelligence. Can you walk us through that?
Speaker 2:Sure, yeah, we really are coming to almost a portfolio approach to artificial intelligence, the traditional, classical artificial intelligence neural networks, clustering, classification, all those things that we've done for many, many years that we're able to predict what will happen, how many people will come to the store, what is the right price these kind of things. Generative AI is now giving us the ability to generate really quickly, different ways to how to respond to people. All right, we wanna respond to a teenage girl or we wanna respond to a senior citizen. Generative AI is very good at generating narratives that are appropriate for those kind of responses. The how of it We've never really had the why, and that's what we have with causal artificial intelligence is the understand, the ability to gather data, analyze it, put it into certain shapes, run it through this new form of calculus and get the why of what's gonna happen.
Speaker 2:So soon we're gonna have composite applications that will actually predict why things are gonna happen, what we should do and how we should respond, and I believe and if no one else does it, I'm going to do it we're gonna be building applications that just run all three of those at the same time and come out with okay, here's your entire pipeline of what you should be doing for this set of customers, or for this product, or for this geography or whatever phenomenon or group you're trying to address.
Speaker 1:Absolutely, and so many people take this for granted, the power of Generative AI. Like you mentioned, generative AI can have that context on how to speak to, in your words, like either a high school or a teenage girl or a senior citizen or a young professional adult in their career with a background in economics and finance, something along those lines. So how does Generative AI get all that context?
Speaker 2:It's really a great question, john, and my day job is on the global head of AI for EY and we've been banging on this for eight months now and we've made all sorts of errors, of course, but we've made great progress in being able to bring together different kinds of unstructured information, embed it, index it, structure it, store it and have it work around the large language models to help ground it so you can put in any kind of context that you want. I was working with a group of people the other day and they were asking questions of a large language model and the questions were almost the same things you'd ask of a search engine what's the capital of Georgia, what's the capital of Azerbaijan and who was the president in 1862? And I was thinking, how do I get past this? How do I get past this? Just view that I'm working with a search engine on steroids. So I said, okay, I'm gonna do a scenario.
Speaker 2:So I typed into the chat window. I said all subsequent responses I want you to respond as if you were Vito Corleone's character in the Godfather, so I didn't say Marlon Brando. It had to deduce what those speech patterns were and what that cadence was and how the speech, the didactic speech, would go, and then I started asking it all sorts of questions what is the best way to penetrate a market in fruit or olive oil? I tried to keep with the Godfather theme there what is the best way to eradicate competition in a certain market? And it answered just as if it were Marlon Brando. So what I was trying to get to people is that you can do things that are simple, you can do things that are interesting, you can put context, you can make these models do all sorts of interesting things. The real question is how creative do you want to be? How specific do you want to program that model with your language? And you can see the light bulbs going on all over the room and people were doing all sorts of interesting things with it.
Speaker 1:After that, yeah, it's very powerful and I even love how precise it can be. Like to know that when you say talk like Don Corleone and the Godfather, it knows that you probably mean like Marlon Brando's interpretation and delivery of that role, right? Not the one in the book.
Speaker 1:So it's so powerful and so aware of that, the human intention of the prompts. Now, at the same time, I've heard the term AI can hallucinate. If it was wrong information, it can go down a rabbit hole of hallucination, just completely bs-ing you. How do you prevent that from happening?
Speaker 2:Yeah, john, that's a real issue and we found through some of our research that models we were working with if they couldn't get out to the internet to reach the link that we were providing, it would take the components of the link and just hallucinate a story from it. So we started creating totally fictitious URLs that were really long, that had all sorts of stuff in them and it would take the parts of that and write a story and it was really funny to watch. It's like okay, this is a real problem. So there's a number of different approaches that are out there today and we've been working with a wide range of them. So retrieval augmented generation is becoming very popular. People are understanding that the whole.
Speaker 2:One of the main purposes of RAG, as it's referred to often, is to ground the model, to ground the responses, to bring back greater relevancy, better accuracy, less hallucination. So retrieval augmented generation is one way to do it Programmatically augmented, larger language models. We've done this and it works out very well in grounding the model and then function calling where you can actually take a model and run it maybe a RAG environment run it, bring the response back, use that response to stimulate a different system, maybe an SAP ERP system or a CRM system, get a response back, so, and then you can use libraries and all sorts of other things as well, but what you're doing is you're building a constellation of functions around that model that ground it, that bring it back to reality and, as I said, increase accuracy, relevancy and reduce hallucinations. So it's really nothing more than what we historically done in information management. It's just a different architecture.
Speaker 1:Very cool, very cool. And we've heard the term RAG and the benefit and the purpose of it, as you mentioned, but can you tell us a bit about how it works?
Speaker 2:Absolutely so. We talk about tuning a model or training a model, and that's what open AI and cohere and then Thropic. They train their models and we get those models and we can open them up and fine tune them. But generally not too many people are doing that right now. We'll do more of that in the future.
Speaker 2:But RAG is really the ability to bring in unstructured information, run it through an indexer like Lama index or Lang chain or something like that, build embeddings and vectors, so you have, you know, all the different unstructured information aligned and into compact representations and then take the and you can.
Speaker 2:You don't have to do this. You can take the entire corpus of documents that you brought in and indexed and put it in blob storage. Now there's a number of ways you can run these queries, but usually what happens is you run the queries in into the indexed information and the embeddings. You bring out the top three, five, ten hits that you've got. You run that through the large language model. It generates a narrative and then, if you need citations, you can run it through the blob storage and get the citations and then you bring the whole thing back. So you're using your corpus of information indexed in a way to get relevant hits, you know, in your search. You run it through the model to get the narrative, you run it through the blob storage to get the citations and then you bring the whole thing out to the user.
Speaker 1:Very cool, and I think this is. These are all the components that you know people are just starting to familiarize themselves with, you know, as they start their AI journey. Now you've actually you're an implementer right. You have another, very unique perspective on what you're building now, and I don't know how much detail you can get into, but if you can, you know, share exactly your approach to building out production, generative AI and artificial intelligence in general. That would also be great.
Speaker 2:Yeah, I am an implementer. I am a practitioner, which I'm very proud of. I really like to solve real-world problems and use this technology in a way that makes a difference for people. You know my day job is to build the infrastructure of all three pillars of AI. For UI.
Speaker 2:We have been focused most recently on generative AI and we've built a scalable, robust infrastructure for all 400,000 UI employees. So we have a private, secure chat feature that people are using on a daily basis. We have a sandbox that allows people to do experimentation and we have a proof-of-concept environment that allows people to build applications in a secure, governed way. So you know, we've built this entire infrastructure over the last few months and we have, you know, hundreds of thousands of people on it every day banging away. So you know, we went out there and did it. We thought, well, there's not really many people that can help us because we're kind of on the bleeding leading edge. So we just dove into it and, as I said, we made mistakes. We went down blind to allies, but we did it quickly, we learned fast and we stood it up and we're quite proud of what we've got.
Speaker 1:Excellent, and you know I love speaking to people who've done AI before. You know we've had some previous guests on the show who either did it at Facebook or you know places like Lyft. And you know it's not only just like hey, deploying the AI and making sure that you know, or you know it's not just about deploying the AI and talking about the technology benefits and there are so many and there's so many ROI you can get out of AI but it's also avoiding the pitfalls. So what are some of the main pitfalls of AI and how do you avoid them?
Speaker 2:Yeah, you know, almost each pillar has its own pitfalls. There's no doubt about it. You know traditional a I, you know I think some of the biggest pitfalls are not getting executive buy-in. It's not really even technology, it's not getting the organization to understand that if you take this journey and you make this investment, you have to change how you do business. You know it just doesn't work if you don't use the results. I mean, you know, if uber and when it did all this work on understanding the distance between drivers and riders and then the company said they're not going to implement it, I mean, what's the point? You know there's, there's no sense in that. So traditional a is usually the pitfall is cultural With with Generative a I, what we found the some of the biggest issues, the biggest stumbling block is is inaccurate or out of date content.
Speaker 2:You know, because we're taking a lot of content that hasn't been looked at in quite some time and some of it has been looked at recently but it just hasn't been, I guess, reviewed or, you know, quality check or whatever. So we put this, put the content into a gen a I environment. We start querying it and people come back and say, oh well, the models wrong, that answers wrong or that answers wrong, and what we found is ninety percent of the time it's the content is wrong. So we go back and say, okay, well, let's, let's go back and review the content, let's take out everything that we did, you know, get rid of that, review the content, get the quality content, put it in, ensure that the models are working, and then you go forward with a content upgrade process and then for cause a li, what we've seen so far, the pitfalls are, it's hard to understand.
Speaker 2:You know we haven't gotten to a point where there are great software packages that are easy to use. Most of the software out there is developer facing, and trying to understand how to do a directed acyclic graph that accurately represents the world you're trying to model is challenging. So you know, we've got cultural, we've got data and got technology. So those are the pitfalls I see in those very in those three pillars.
Speaker 1:I love the comprehensive overview because even if you get pet, like you're saying, if you get past the technical hurdles, you don't have executive buy in, you don't have other business groups really using it, then it's, it's wasteful and ultimately you won't get ROI return investment out of it. Sure, yeah, and that's such a good Area to dive into on the technology side. You know how do you make sure that is making you know correct decisions like, like. How are you continue continuously testing model fit, for instance?
Speaker 2:Yeah, we've been working on. You know, the work in a white predates me. I like, I think I mentioned I've been in a year, almost a year now, and the work on responsible AI has been going on for a couple years and we're now moving into a phase of where we're taking responsible, ethical, transparent AI and turning it into code. So you know, soon we'll be able to have a rigorous, comprehensive testing regime that's linked to the laws and regulations and compliance metrics of a specific industry, a specific country, a specific state. So what we're doing is we're doing statistical testing on models to make sure that they're not drifting or breaching certain levels, either above or below where they're supposed to be, or left to right out of a box.
Speaker 1:So we're working on that and that's obviously very proprietary and confidential.
Speaker 2:And then we're working with external bodies on what they think is responsible AI universities, research organizations, universities around the world and research institutes around the world as well and we're trying to distill that down so we can not only have it in artifacts that we use in consulting and professional services, like PowerPoint decks and processes and Spreadsheets and documents and things like that, but actually reducing it to code so we can really test these things in a in a large scale model. The environment?
Speaker 1:Absolutely, and you know, I think there's there's lots of examples of, you know, companies sort of doing sat and forget with AI, which is obviously not an option. But you know, when there was one famous example where they use a forecasting model To basically dictate the prices they were going to offer some good I'm going to keep it very broad and anonymous, but let's say they were using forecasting To set the price of something they were selling in a marketplace and it turned out that they didn't take in you know, new factors such as inflation, rising interest rates, things along those lines, and this, this business generated hundreds of millions of dollars in losses. You know they ended up losing you know, 25% of their market cap as a result of this. And these are, you know, risks. But how do you, you know, mitigate the risks on just the, the culture of setting and forgetting AI?
Speaker 2:Yeah, you can't do that. Well, you can, and you just talked about the pitfalls of doing it. So you know that's not a good thing. You know he.
Speaker 2:Generally it comes down to the partnership between the modeling and the AI group, modeling AI group and the business. You know that you're working in conjunction with each other. We, as analytical professionals, know the data, we know the algorithms, we know the applications. The business people, the subject matter experts, know their business. So having those two groups working in conjunction with each other usually mitigates or eliminates, set and forget, because someone's always asking the other group a question Like hey, we just saw interest rates go up five points in two months. We really should look at that model. Or the business is saying these forecasts seem a little wonky. Let's take a look.
Speaker 2:We had a great example. We were forecasting in my previous job at the Bio Pharma Company. We were predicting how many people would come into our 350 donation centers around the United States and then, in what was at March 2020 or 2021 or whatever it was, covid snapped in and in the span of like three days, four weeks, whatever it was, our whole world changed. Our models went from an error term of about 3% to completely wacko. They were just nowhere near reality and a lot of people in the business came to us and said, hey, these models are no good. And we said, look, the models are just fine, but they were trained in a different era, which was last month, oddly, and it took us three months to recalibrate the models and get them back on track. But they were fine in the COVID area it was just a different type of velocity and volume that we were seeing. So you need a partnership is generally how I see it works best. Excellent excellent.
Speaker 1:I think you really do have that great business perspective on making sure that it's a very sure that it's viable internally and getting the right adoption, and I think just hammering that over and over again with teams that are actually deploying AI is going to be critical for success there. And now that segues into my next question who should own AI? I mean tactically, from an implementation perspective, within companies.
Speaker 2:That's a great question and there's a lot of people that like to have a parochial view of ownership I own it and nobody else owns it. It doesn't work well that way. A lot of technology should have had more of a shared ownership model than it ever did, and I think AI in all its different flavors and pillars, is bringing that dichotomy and that breakdown in partnership in greater relief. It really shows that the data teams need the analytics teams. The analytics teams need the subject matter experts and the executives need all three of those people working together to reliably move the business forward. So it's not going to be that the IT group owns it, or the CIO owns it, or the CAO owns it or the CDO, or you can do that absolutely and people do implement it that way, but you're not going to get the value out of it. You're not going to be one of the leading players in your industry or in your market. It needs to be a collaboration and shared ownership. I know I sound like a one-trick pony, but it's the only way it works.
Speaker 1:And that's such a great point. And I've seen this play out at companies where, hey, should this all be in the responsibility of a chief data officer or engineering or CTO, or do we need a chief AI officer? And they have their own. They have their own domain experts on that topic and ultimately, it does sound like companies will have to figure out what works best for them. However, you know there's going to be dependencies on the data teams, right, because they're the ones who are ultimately building the infrastructure to move data around. So, and more of a practical question for you so you know teams want to use AI. There's all these third-party models out there that have been trained for years with tons of data. I don't see enterprises trying to bring that function in-house of you know building the models themselves, but how does an enterprise securely deploy a model in their environment with their customer data without the model actually being trained on proprietary data used for other companies?
Speaker 2:You know it's a great question and one that I answer almost daily. You know, my job at EY is not a customer-facing job. You know I'm building the infrastructure for the whole company, but in the last two months I think we've talked to 40 customers. You know, because this area is so hot and I enjoy talking to customers, I don't have any issue being out front and center and talking to board members and C-level executives, and you know other folks that are doing their jobs. So you know it's not any different than anything we've done before. You know it sounds different but it's not.
Speaker 2:You know, at the basics, you know, if you do good information management processes and good architecture and good security practices, it's the same thing we've always done. You build systems that are clean, that work well, that are protected from the outside world. You encrypt data at rest and in motion, and it's the same thing that we've done forever. So you know it's a wild and wooly world. There's no doubt about it, and you know we've talked about hallucinations and all those different kind of things. But you know, if you build the rag architecture or the different architectures that I've talked about in the right way, they're easy to secure and they're easy to control and they actually benefit from logical and physical separation of assets. So you actually end up with an architecture that's pretty easy to defend and protect and it works really well. Excellent, excellent.
Speaker 1:So you know it does really keep. It does sound like the new technology that the knowledge of managing data securely and infrastructure securely does come back to those teams that likely have the most leverage to. You know, implement AI within the organizations, which is those kind of cloud engineering, data engineering type teams. But you know, augmenting it with the AI and data science type of work but at the same time making sure there's enough executive buy-in right vehicles internally to make sure there's adoption. So you know, when the CFO comes and says, hey, I got this big cloud bill, what was the output of it? Right? And say, well, it's tied to this critical customer-facing software that we have. Or you know this internal operation that makes sure that we're always delivering things on time on budget, you know it's gonna have to come down to that.
Speaker 2:Yeah, one of the things that I learned a few decades ago is that you know any kind of analytic or data warehouse data, lake house, a I, advanced analytics, statistics, optimization, simulation, all the different things that I've built over the years. You know I always bring them back to metrics that that non technical executives can understand. It's either acquisition of customers, or it's increasing eyeballs, or it's revenue or margin, or you know whatever they're interested in. So you know when they come and say, you know, hey, we got this bill, and I say, yeah, that's tied to the five hundred thousand you know, customers we attracted in the last quarter and they go, okay, fine, yeah, it's easy to make your life easy if you want to.
Speaker 2:You know, if you look and if you're not driving those kind of improvements in in operations, then you shouldn't be doing what you're doing. You know you should be able to tie exactly what you're doing in data and analytics to operational metrics that people care about. You know if your improvement is something people don't care about should be doing. So you know that's what I've always found is that people are having a really hard time Tying all the work we've done to any kind of measurable improvement and I say well, it's kind of your fault.
Speaker 1:You know Absolutely, absolutely well. John k thompson, author, data practitioner and leader for a I at the e? Y. Thank you for joining today's episode of what's new and data working people follow along with you.
Speaker 2:You know I, my social is linked in. You know john john thompson on linked in. That's where I post everything you know link with me there. I'm pretty Ruthless and who. I'm not a linked in open network or I'm not a lion. You know, if you're in data analytics and you're doing something in the field that's relevant and interesting, I'll connect with you.
Speaker 1:If you're trying to sell me a wealth generation scheme or franchise you know opportunity then please don't Link to the place excellent, excellent, and we'll have that link in the description for the listeners, and thank you to everyone who tuned in today. Thank you again, john k thompson, for joining us.
Speaker 2:Thanks, john, it was fun. I enjoyed it. Look forward to the next time.