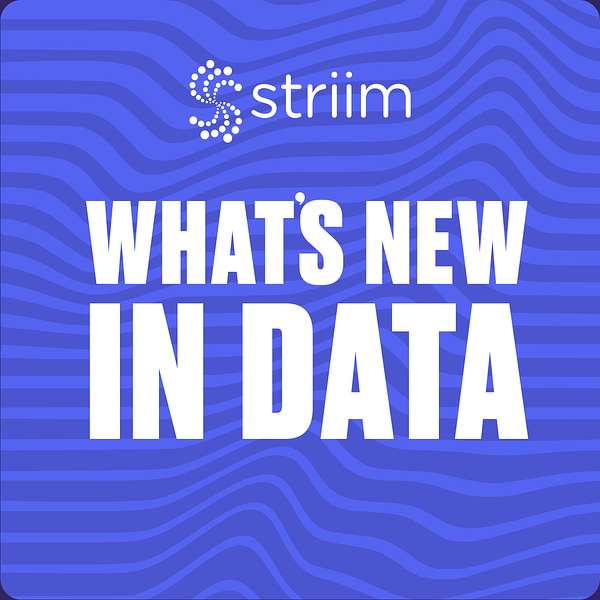
What's New In Data
A podcast by Striim (pronounced 'Stream') that covers the latest trends and news in data, cloud computing, data streaming, and analytics.
What's New In Data
Philosophical Reflections on Data, The dbt Conference, Streaming Workloads, DuckDB with Santona Tuli, Ph.D.
Ever wondered why the philosophical and cultural considerations of data work are essential? You're in for a treat as Santona Tuli, Ph.D. , Upsolver's Head of Data, joins us for an enlightening discussion. Santona shares her takeaways from Coalesce 2023 and the importance of understanding the 'why' behind data work. We traverse the nitty-gritty of grappling with streaming and batch data workloads, shedding light on the imperativeness of balancing data freshness with resource efficiency. Plus, we don't leave out the current happenings in the data scene like the rise of data streaming, portable compute options like DuckDB and the potential hiccups in bringing it back into the cloud for collaboration.
Reliving our experiences at the recently concluded DBT Conference, we muse over the irreplaceable value of face-to-face conferences in fostering connections with fellow data enthusiasts. The conference wasn't just about serious talks; there were also fun-filled booths with puppies and headshots! The mystery surrounding the new product, MotherDuck, doesn't escape our discussion as we speculate about its potential role in the modern data stack. Reflect with us on the philosophical implications of our work and its impact on our mental health and overall job satisfaction. Get ready for an episode packed with insights, reflections, and a healthy dash of speculation.
Follow Santona Tuli on LinkedIn
What's New In Data is a data thought leadership series hosted by John Kutay who leads data and products at Striim. What's New In Data hosts industry practitioners to discuss latest trends, common patterns for real world data patterns, and analytics success stories.
Hello everyone, thank you for tuning in to today's episode of what's New in Data. I'm super excited about our guest today. We have Shantona Tuli, head of data at Upsolver. Shantona, how are you doing today?
Speaker 2:I'm doing really well. Thank you, John. How are you?
Speaker 1:Great, it was really fun catching up with you at Coales 2023 down in San Diego. Really fun events and running into familiar faces and good friends. Glad I got to connect with you there. How did you feel about Coales 2023?
Speaker 2:I loved it. I really enjoyed it. I've been going a lot of conferences this year, which is like the volume is new. I've always given talks at conferences and stuff and I really loved them, but this year was a lot, lot. But Coales stood out because I think there are a few reasons. It was a big conference, but not unmanageably big. It felt like I could go to talks and I could actually engage with people and have meaningful conversations. The second reason is there were lots of data professionals. It was less focused on vendors, it was less focused on specific agendas and just folks wanting to learn how to better use DBT or just how to better do analytics or data work. I really enjoyed that.
Speaker 1:Absolutely. I was also impressed by the amount of data practitioners there. Sometimes there are practitioner heavy conferences, executive heavy conferences and sometimes it's literally just vendor heavy. I think DBT Coales down in San Diego both this year and last year were a pretty healthy representation from the real practitioners and you got all their perspectives. Shantona, tell the listeners a bit about yourself and your background.
Speaker 2:Yeah, absolutely. My background is in physics. I'm one of those physicists that went into data In particular. I got my PhD in nuclear physics. When I say that, I think sometimes people think about nuclear reactors. It's a very different kind of nuclear physics that I did. I was studying the atomic nucleus and fundamental particles, trying to explain why the universe is the way it is. Then came back down to Earth, worked as a machine learning engineer in customer relationship management space. We were building essentially artificial intelligence that would understand what folks were trying to ask for help with within a product and then how best we could resolve their question. We can talk more about that if you want to. Then I had a traditional data scientist title, worked at Astronomer, which is managed airflow. Now I'm at Upsolver, as you said, as head of data. I also head of, essentially, product strategy. A lot of my role is actually focused on how do we make Upsolver the best version of itself for practitioners like ourselves and how do we make data workflows easier.
Speaker 1:Excellent. You went from the causal forces in the universe down to the causal levels of a business, which is the data. That's a very cool transition, but I love the way you went about tying those two together. You recently gave a talk at COLS. I tried to attend. There was literally a line out the door. I said, okay, I know this is a great talk with Shantona, the best place to learn. Catch up with her on it. We'll be here on the pod. I would love to hear a bit about your presentation there.
Speaker 2:Yeah, happy to chat about it. I was really happy. I enjoyed giving the talk and enjoyed the audience, the engagement. Not all conferences are the same. Not all talks are the same. I did something new this time, which was I was talking more about sort of philosophical and cultural aspects of scaling up data workloads and scaling up individual developer productivity. A lot of my talks previously have been directly on like the pipeline that I built or the work that I did and like going to some technical details or, you know, trying to define what is ML ops. But this was very much like how do you, how do you scale, how do you scale the business, how do you scale data pipelines and how do you scale yourself? So, yeah, I had a lot of fun.
Speaker 1:Absolutely, and it was a very well, well attended talk, very popular, a lot of people. You know there were a lot of murmurs in the hallways about your talk. So I think when the links go live, everyone should definitely check it out in depth. And I do want to drill into one part, which is you know, like you mentioned, you know the philosophical side of you know developing yourself and why. You know you're working on certain pipelines and, in general, you know approaching data. You know why is it important for data practitioners to think about questions like the kind of the kind of the philosophical raison d'etre behind data.
Speaker 2:That's a deep question. To keep us grounded, I think is a short answer. It's it's. It's interesting because I could frame it in terms of a metaphor, where you're going high level or I could talk about, you know, like getting grounded, and I think it does both, but in the same way. So here's what I mean A as someone who is, like as an engineer or a scientist, like doing, you know, executing on something.
Speaker 2:It's easy to get lost in the weeds. So, thinking about the why am I doing this? Why am I here? What is the you know, what is the purpose, all of these things kind of helps you snap out of that and also, like, say, a bit more grounded, like I think it's healthy from a mental health perspective to to sort of, you know, be able to come out of the weeds, because sometimes it's just not that big of a deal. Yes, I mean you should absolutely feel fulfilled from your work and get you know like work is great and it's it can be a lot of fun sometimes, but coming out of it can also make you, make you realize that, okay, maybe I don't need to push myself and pull a 70 hour week just because of this one project, because there's like it's at the end of the day, it's not that impactful overall. So yeah, that wasn't exactly what my talk was about, but I think it's like it's important to be able to introspect certainly.
Speaker 1:Absolutely. And with the growth of AI, it's clear that our definition of self is going to change. Because what's the difference between, you know, shantona or John going in and writing code and a pipeline, versus chat, GPT or co pilot generating that same code? There's a human element and this is kind of one of the the open questions of the universe of like, what does it actually mean to be, to be human? And this is going to be tested very thoroughly over and over again.
Speaker 1:As you know, you can have conversations with with AI now and you know it's it's going to start freaking out more and more people as they do it. So it's very important, as you said, to ground yourself and introspect and understand what it means for the, for the person to build the pipeline Right. So I love that you look at it from that perspective. Maybe we're both nerding out over this too much and, but I think it's such a fun way to look at the world and really makes you think about the meaning behind it, getting more into you know certain technical topics. A lot of teams are are building their pipelines for scale. You know what are some of the challenges of large scale workloads.
Speaker 2:I think the biggest challenges though there was there was almost this, you know, movement away from a scale, I feel, in the last few years, because folks realize that, like a lot of the solutions that were initially built came out from the massive scale workloads that folks had to deal with, the massive companies right. All of a sudden, you had all this social media data and pictures and videos and all of these things, and there was tooling built sort of quickly, I would say, although, like the engineering was certainly certainly there again because they were being built at the from these like our house companies, but it was. There was a hype almost of, oh, it's all like big data, everything's at big scale, and then there was a pushback, or against it. There has been, I feel, in the last you know, three, four or five years that not everybody has skill right, not everybody has to think about or worry about large scale workloads, so let's talk about medium scale or small scale, and I think we have the best iteration right now where we're building tools that are scalable rather than focus on scale. It's like just high scale, right. So I think scalability is like a much more interesting thing to build for so that you can work at. You know low scales to high scales and you appropriately adjust. So this is this is actually from my top scale to me is robustness, being able to be able to adjust to whatever your scale demands.
Speaker 2:But the reason that that one of the reasons that scale has been hard, I think, is because of the tooling you traditionally and I mean this is still true in part right. Right, there's more self serve kind of tools that you can do, like low code, no code work in, but it's usually not going to go too far past, like a proof of concept kind of work, or you know again, low scale working, or you have to be that like really deep into Spark or really deep into Map Review, something that like helps you do distributed workloads and that's the only way to do scale. So, again, I think we're coming to a point now where we're bringing those two things together. But that's one of the reasons it's been hard. And then the other reason, which I alluded to earlier, is like just this confusion around well, what is my scale? What tooling do I need? How do I approach this? Because everyone's saying something different around. You know how to deal with scale.
Speaker 1:That's such a good point that scale is synonymous or can be compared to robustness. Everyone likes the idea of having this pipeline where it's low code, no code, super easy to deploy and get started with and maybe you turn a knob and it'll magically scale. Do you see any solution like that?
Speaker 2:Yeah, so that's what we're currently building. Actually, I mean, I don't wanna talk too much yeah, so you can build your pipelines with Upsolver really straightforwardly, like we have a wizard driven no code experience, where you sort of say, okay, this is my source and this is my target, because our engine is robust and because it scales up, it matters much less what you're doing as a user, like what you're doing on the front end, so you can just write a pipeline using the UI, or you can write it in simple declarative SQL and again the engine is abstracted. Or, if you want to incorporate it into your CI CD pipelines using DBT or the CLI or Python SDK, we have those options as well. So this has actually been very important to me because I used to be sorry, slight tangent.
Speaker 2:I used to be a little bit I think also not uncommon a little bit foo foo about low code options, like can they really do what they're claiming to do? What? Why is how can they be easy when everything else is hard? And so it was important to me to not be, not for us not to be classified as just as low code. I think what's important is to give the developer or the user the experience that they want and they need. So that's why it's, like you know, choose your own code adventure kind of scenario that we have going. But yeah, to come back to the, you know your question. If you have a powerful engine that can adequately like partition, the data process, the data can like you know, you have state and memory, or you have the robustness built around what happens. If you know, if the engine goes down, how do I recover state, all of these things that you have solved for in the backend, then of course you can have a easy to use or UI driven experience and still get the same things.
Speaker 1:Yeah, absolutely, and it's great to have, you know, performance meets simplicity in a single pane of glass, for sure, and you know that's. You know what I, what we work on at at stream as well. So it's good to see so many, so much option in the market for data practitioners to really adopt this. And I love the work you're doing as well. And it's one of those items where, you know, people don't really understand you know why can't it be as simple as turning a knob? I'm borrowing that term from Wang and Siketa from dataworld.
Speaker 1:He asked me that question like, specifically, from going from batch to real time, why can't I just, you know, have like a knob that says, okay, I'm at 60 minutes syncs right now, and now I wanna just crank it to real time with the same pipeline without doing any other work, which is, yes, I think that's what people want and I think that's what we'll eventually get to. When you actually think about the infrastructure required behind the scenes, the way you're even polling for changes, like whether you're doing, like you know, queries against a prod database. Maybe that's okay if you're doing really small queries on like a very infrequent basis, but as you turn up, the, the, the, the sync frequencies. You'll have to do CDC, log-based CDC, and then you'll have to switch from a batch to a streaming pipeline. That has other implications. How should people think about really combining batches and streams?
Speaker 2:Yeah, I really like that knob analogy. I think I think Juan said has a right idea there. The reason it hasn't been a knob right, I feel is because we've just regarded batch and stream workloads as two completely different beasts. Right, and you are doing batch work, so you're familiar with the batch tools and you've got your, you know, your orchestrator and your cron schedules or whatever it may be, and you've certain tools that you're using. And then there's this other side, or oh, okay, I have to work with streaming workloads because my data is streaming right.
Speaker 2:So, like, again going back to the social media, is the sort of user interaction type data or finance, financial transactions, right, and ad tech. And then, as you said, no matter what your product is supporting, it has some sort of you know prod or transactional database that is inherently like it's you know a transaction base. So you know that's another way to think about streaming and you know if you have to work with that, then you figure out that stack. If you don't have to work, if you're doing like sort of analytics that you know you have 24 hour SLAs on dashboards or something, then you're used to the batch world and we've just never really thought about the two as being same or having a meeting point in the middle. So I really like this knob analogy, or thinking of it as a spectrum, because I think you absolutely can. You can go from you know, like especially if you quantify it with what is my data freshness level or SLA, then you absolutely can think of it as an again like a good I don't want to call it an orchestration engine a good like data workload engine. Let's be super like high level should be able to like again, scale from low freshness to high freshness, or like long intervals to short intervals, and again, that's what we're building as well.
Speaker 2:But the trick is to like think of it as a continuum, one and then two, again, be robust, because when you have two disparate infrastructures, right then if you're trying to push batch to go as fast as possible, then you're, it's suboptimal, you're gonna run into inefficiencies. And similarly, if you're trying to like, if you're in a streaming workflow or ecosystem and you're trying to like make it batch for whatever reason, maybe you want to do more transformations or something, again you're introducing like a different paradigm to this system and that introduces inefficiency. So, like, fundamentally, we need that actually unification of the workloads and the infrastructure such that when you do travel across that spectrum, then what you're doing is you are balancing your freshness with the resources that you're spending. Going back to our scalars robustness argument, as you scale up your data freshness, let's say you got to make sure that you're not wasting resources or you're not causing some other inefficiency.
Speaker 1:Absolutely. When I think about the knob analogy again, which I love and we keep coming back to because it's so simple, but you're raising some good technical points of what it would take I always like to reference Michael Stonebreaker's quote Michael Stonebreaker, of course, one of the founders of data management in its current context, with decades of experience there, both theoretical and applied in enterprise software. He says one size does not fit all. What he's really coming back to is this idea of there's all these trade-offs and distributed systems. The most popular one is CAP. You're choosing between consistency, availability, partition, tolerance pick two. That's just a very popular one that a lot of people know. There's actually thousands of trade-offs and distributed systems that you have to make Fundamentally.
Speaker 1:That's why we see batch and streaming as two different technical paradigms. When you actually think about what it takes to do streaming, what it takes to do batch being able to switch seamlessly can probably be done, but it would be a lot more expensive than people would think it is or it would be open to all these corner cases that are getting give you sloppy and accurate data or downtime, things along those lines. This is why we're in this work. I think there's going to be innovation here. It's great to hear nuclear physicists like yourself working on this as well. I think the data practitioners are going to get some cool stuff coming to them pretty soon. We talked about scale and robustness. What do you think of the opposite end of the spectrum that we're seeing gain popularity, such as duck DB like small portable compute?
Speaker 2:It's super interesting. I'm going to measure myself a little bit. First of all, I think duck DB is great. It's true, you can do a lot on your laptop. Being able to tap into that is absolutely fantastic. The part that I am that I find really interesting is I was recently talking with Jordan at the DuckDB booth at Coalesc and MotherDuck. Of course, they've raised a bunch of money and they're going to do great stuff, but one of the things that I heard was now they want to bring that back up into the cloud that like DuckDB experience back up into the cloud and enable collaboration. So it feels like maybe you have more information than I do or maybe you can help me understand this idea, but there's all this push to do work on your laptop and leverage DuckDB. But if you're going back into the cloud, is there something that was up?
Speaker 1:Yeah, that's a good point. That's a good point. So DuckDB's big advantage is this portable compute that you can run in your laptop. It can be embedded in a browser, it can be embedded in a Lambda function and it gives you sort of this, this, this super mini OLAP database. And you're bringing up a point which is MotherDuck. Great team there Jordan Tagani, tina's working there as well, awesome peoples.
Speaker 1:But there, if you look at it, they came up with a graphic that looks scarily similar to the whole modern data stack graphic where, yeah, you got your connectors on the left, you got MotherDuck in the middle, which is like your warehouse, and then you have your analytics on the right.
Speaker 1:So your question is like how is that different from what you were doing before? My sense and this is an interpretation, I'm sure if I, if, when we put out this pod, I feel free for someone to dunk on me and tell me I'm wrong. But my interpretation is, yes, you're going to have all these little DuckDB instances running everywhere and MotherDuck is essentially that, literally, when you think about a row of baby ducks and you're going to have this MotherDuck that they're all going to connect up to, sort of like the mother ship, what it means in the product, I don't know. I mean, we're all waiting to see, you know, how that, how that, turns out, and I'm sure it'll be some exciting stuff Ultimately. You know, the idea of having all this, you know, compute all over the place from an enterprise perspective seems risky, and you'd probably want some way to to centralize and orchestrate it. But that's my guess, though. What do you think?
Speaker 2:That makes sense. But then I want to ask like how's that different from you know, kubernetes pods right and Kubernetes engine, like orchestrating everything? I think that there is innovation here. I'm just not sure I understand it yet.
Speaker 1:Yeah, I think there's some. There's definitely some allure to the ambiguity here and we'll see what direction that goes. I think the whole data community is honestly asking the exact same question you have and having similar. You know, I wouldn't call it doubts, but you know, just wondering how it's different than you know previous iteration of modern data stack where you know, rather than having snowflake be your, your compute engine, it's going to be, you know, duck DB, like a little more portable compute. Or you know, having Kubernetes, pods that are, that are all over the place running in different regions and data centers, and on-prem and cloud as well. So we'll just have to see that's. That's what makes this industry so fun, right?
Speaker 2:Yeah, yeah, absolutely.
Speaker 1:Yeah, and you know, duck DB is super popular. I don't know if you had a chance to go by the Zenlitic booth and see that they had these data monns and this was at a colis, by the way. So so Zenlitic, a company that does self-service business intelligence. If you talk to them, they'll say they're the first company to do self-service intelligence, which you know eyebrow raising, but listen to their story on it. It's very cool. At colis, at their booth, they had this thing of data mon, which is like Pokemon, with, with, like people from the data community. Did you get a chance to check that one out?
Speaker 2:It did not, no, oh okay, Okay.
Speaker 1:Yeah, that was, that was a fun little. That was a fun little thing at at colis, for sure.
Speaker 2:Did they have your data mon?
Speaker 1:Yeah, they had me, they had, they had some other folks as well. I'm sure they they had one for you as well. I mean, we'll have to check on that offline they they had Sarah Krasnik and they had a Pedram Nabeed and a bunch of other folks. So I'm sure they'll get to everyone in due time. And you know the other thing at colis, in the words of Patrick Miller, who leads data at NAI at new front, he said it's it's the best hallway conference, because when you walk the hallways they're going to run into some amazing expert practitioners and people to catch up with. Would you say you had a similar experience?
Speaker 2:Yeah, absolutely. I mean, I didn't know that you were going to be there and you know, we'd literally ran into each other in the hallway and had a conversation and yeah, no, absolutely there's. What was interesting to me, too, is there were lots of people in the Expo hall like vendors were getting a lot of traffic and there were a lot of people in the tugs, but there were also a lot of people in the hallways, as you said, and like just having conversations. So like it almost doesn't make sense. I think, in that sense, things were planned really well and I also liked that there weren't like seven bazillion parallel tracks going is like two or three, I think, at a time, which was really great because you didn't have to choose from a lot of different options and you could, you know, try to get to the tux that you wanted to go to.
Speaker 1:Absolutely. What were some other highlights for you at DBT?
Speaker 2:Let's see Definitely a lot of really great conversations. Oh, I'm running to people. It's always nice to like actually meet in person people that I haven't met in person before. So Eric Dodds was someone that I met and chatted with there. He runs the data set show and I was recently on it. Then Jason Paul, who works at Databricks We've had many conversations on the phone and he was there. The Databricks booth is nice to chat with him as well.
Speaker 2:So I think for me at least, more recently it's really been about the people at conferences and, again, it's great to have practitioners and talk to them. It's also great to actually run into people that you can sort of consider friends. You know these people and you're interacting with them, and there are various podcasts, like your awesome one and, yeah, actually putting a face to that. So that was another highlight. Oh, the activation ideas at the different booths were fantastic. I think there was definitely a step up from other conferences that have been to like really interactive, fun activations like puppies and, you know, coconuts, and we did headshots and stuff. I really like those.
Speaker 1:There's so much fun stuff. Yeah, yeah, yeah, exactly the puppies. Oh, my gosh, who's the company that had the puppies? Dakota, dakota, had the puppies in their booth. Yeah, shout out to the Dakota people for having puppies in your booth. That's always a good time.
Speaker 2:And Motherhood had a little arcade. I had one of those claw games and I tried to play it. I could not grab anything from the claw machine, but that was also a lot of fun.
Speaker 1:That's the thing with those claw machine games. They just they rope you in. Luckily, I'm sure, in this case Mother Duck wasn't charging for it, so it wasn't as frustrating.
Speaker 2:Yeah, apparently there's a knob speaking of knobs that you can adjust voltages so like to increase or decrease chances of grabbing. So they had to try and solve it, but I still didn't manage to grab anything.
Speaker 1:Oh no, okay, okay, well, all right. Well, we'll just have to do better next, next year, across the board, in terms of, you know, making sure the claws are more dexterous and able to grab more. What was it? Ducks, baby, baby, ducks. Is that what was in?
Speaker 2:there Mostly T-shirts, yeah.
Speaker 1:Exactly, yeah, yeah, it's always fun to see the the booth novelties at these shows, and I've coal us, of course. You know, in Centaur we ran into each other at Snowflake Summit as well. I would say coal us had a lot of flair to their booth novelties, which is really fun. Every, every vendor was really encouraged to make it, as you know, as fun and sort of silly as possible, whereas, you know, some of the other events that we've been to are very professional. I'm sorry that they're both professional, but some other events are more keen.
Speaker 2:On the enterprise focus yes.
Speaker 1:Yeah, what does it mean that we call it enterprise? Well, anyways, so someone else can read into that for us. Yeah, so we ran into each other at Snowflake Summit, which is a very enterprise audience, and you know how would you say, like Snowflake Summit differs from a DBT coal us.
Speaker 2:It's just much bigger and like I mean, you know I don't you know I don't really have anything negative to say at all about like the companies right they're. You know I use a lot of these products in production and you know I love them. But, like I, when I go to a big and I feel the same way about like AWS conferences have been to a few of those it's just too much. I mean, I'm not an introvert but I am a little bit measured in my, in my, you know, interactions and then, like you know how much I can be on. So it's for me it's just like you know, it's too big sometimes. Which I didn't feel I'd call as my favorite conference is actually, I think, day to day, texas, which is coming around again that's every year in January down in Austin, texas, and that one is like actually small but has a lot of like really smart and awesome people and you can have tons of conversations and learning.
Speaker 1:Absolutely. I'll be there as well, so it'll be fun to range it and run into each other there. Catch up on the pod. I'm sure it'll be completely viral by then. So we'll just be dealing with the. The fame of from generated from this episode. The yeah yeah, the the the nuclear physicists who made everyone existentially question their pipelines. I love it. That's the. That's. That's what what's new and data is all about.
Speaker 2:You've been doing lots of great live shows. I like watching those and it's a little bit different, right it must? It's seems like it's more enjoyable too.
Speaker 1:Yeah, yeah, thanks for bringing that that up. And yeah, we've been taking what's new and data live on the road. We did it in San Francisco with Bruno, aziza Sanjeev, mohan, radima Khan, and we did another one in Toronto with Databricks and CIBC, and Eric was the main thought leader on that panel, along with Sarah Krasnik, who also chimed in on a lot of cool topics, and next week at the time of recording this, a week from the time of recording this, we're doing one in Atlanta. So, but once we do one in your area, it would be amazing to have you on the panel as well.
Speaker 2:Yeah, I'm super excited. I'll hold you to that.
Speaker 1:Yes, please do, please do. I need people calling me on my on my bullshit and making sure that we we actually get these events coordinated because we're all so busy. Yeah, cause I mean, honestly, this is a labor of love. It's right, I run, I run products and and data stream and what's new in data and connecting with the community is such like fun stuff on the side for me to to really go out there and do these events and really, you know, read between the lines and get a pulse of you know where, what all the data people are thinking at a given time. So, yeah, absolutely we're, we're. I definitely want to do one out in your area. Have you on the panel and we'll see. You know what fun people in your area latch onto it and you can invite them as well. It'll be super fun.
Speaker 2:Perfect yeah, Slowly but surely getting a community together in DC area.
Speaker 1:Exactly, exactly, that'll be fun. Yeah, I mean DC totally makes sense to have a big data community there.
Speaker 2:Yeah.
Speaker 1:I'm sure DC stands for data center or something right.
Speaker 2:You're not wrong, because a US West one is like maybe 10 miles from my house.
Speaker 1:There we go, there we go. Well, great, well great. Shantona Tully, head of data at Upsolver. Thank you so much for joining today's episode of what's New in Data, and thank you to everyone you tuned in.
Speaker 2:Thank you so much for having me. This was a blast.