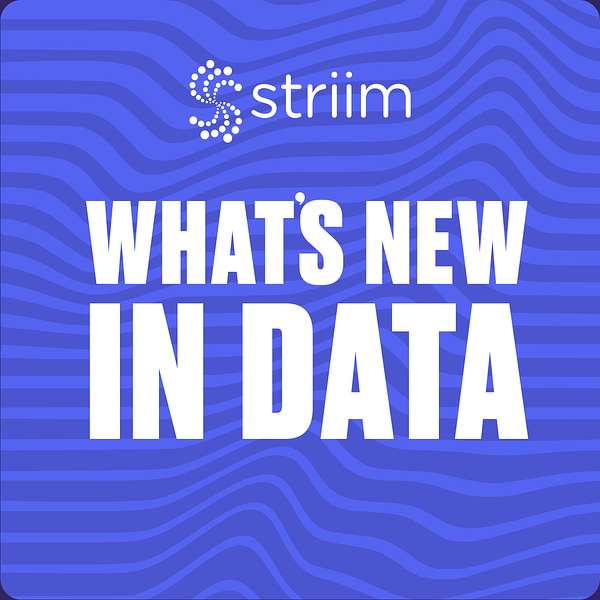
What's New In Data
A podcast by Striim (pronounced 'Stream') that covers the latest trends and news in data, cloud computing, data streaming, and analytics.
What's New In Data
Crafting Intuitive AI Experiences for Everyday Life with Abi Aryan
Embark on a captivating voyage into the intricacies of AI with Abi Aryan, the financial wizard turned tech trailblazer, who unveils the transformative power of machine learning in our latest episode. Witness the metamorphosis of data pipelines and video classification as Abi elucidates her groundbreaking research at UCLA and her influential work within the entertainment industry. Her commitment to social impact and democratizing AI is palpable as she offers a glimpse into her mission of miniaturizing large language models to fit into the palm of your hand, ensuring the future of tech is not only responsive but delightfully anticipatory.
As we unravel the complexities of operationalizing Large Language Models, Abi's insights illuminate the shifting landscape of product experiences, where AI is not just a component but the orchestrator. She expertly navigates the technical finesse required to tailor AI for IoT devices, merging the realms of luxury and practicality for the ultimate smart living experience. Tune in to discover how Abi's pioneering work is crafting a future where technology doesn't just blend into our lives; it enhances them with an intuitive touch, anticipating our every need with intelligence and grace.
What's New In Data is a data thought leadership series hosted by John Kutay who leads data and products at Striim. What's New In Data hosts industry practitioners to discuss latest trends, common patterns for real world data patterns, and analytics success stories.
Hello everyone. Thank you for tuning into today's episode of What's New in Data. I'm really excited about our guest today. We have an expert in AI and machine learning. We have Abi Aryan. Abi, how are you doing today? Hey John, what's up? I'm doing good. How about yourself? Great. Great. You know, we've been talking about doing this episode for such a long time and we, we finally found time in our schedules where, you know, we were able to make it work. So I know the, the stuff you're working on is you know, incredible interest to to, to our listeners around LLMs and LLM ops, and you're, you're also working on additional collateral, including a book on that topic. So, yeah, just it's, it's awesome that we're, we're able to dive into these topics. So Abi, you know, tell the listeners about yourself. So, well I started studying maths in college, enjoyed it, I did minors in computer science, but eventually was planning to get into finance, hated finance people, didn't want to make money for the sake of making money. Ended up in technology industry, so business intelligence was where I sort of landed first. Eventually I naturally transitioned into data science and implementing other people's models. I was like, you know, this is all fun. Yes. I can implement all of these models that are already out there, but can I build these models? So after working in the industry for like about three, four years, I was like, I'm going to go to UCLA and be a visiting research scholar. So I raised I did research stuff for about two years. I was working at the intersection of causality and deep learning, trying to translate intelligent agents and break them down and figure out what intelligent agents really are from like three different perspectives. One was basically how does auto ML work? Second was basically; how does emotional recognition work? And the third was multi agent systems. But eventually got bored with the hyper competitiveness or zero one games of academia, if I can call it so, came back to the industry. And one of the quick things I realized was the data pipelines were broken, as in there is not enough. When I came back to the industry, it was more so like around 2019. Most of the companies were still already on board with, yes, we want to invest in deep learning, but there wasn't enough labor at the companies that I was working with. So that was more so where I specifically started working on, which is building automated data pipelines. Initially worked with some insurance companies and then entertainment companies. Entertainment was fun because what I was working on was more so like video streaming company. And video streaming is really fun because you have three different modalities of detail. You have the image, which is going through, you have the text, which is basically the subtext of the image, and you have the video as such, and you want to classify, the video is basically what is harmful, what's not harmful, what should be classified under which category. And doing it manually for a video company like TikTok where a lot of data gets uploaded at the same time it's really hard to put people manually to do all of that gets incredibly complex so that was where I was working on eventually I had fun, but I kept on doing consulting but shifted a little bit more towards projects, which were AI for good projects, because I wanted my work to carry value. So sort of saving life kind of projects. And right now I'm very much, like, focused on my own style. We're building, basically, large language models to be able to run them on very small devices. You could say tiny ML devices. As such, there are some specific things I'm focusing on, which is basically, like, the evaluation pipelines. And yes, as, as John mentioned, there's a book which is going on on the side, just, just finished the first deadline for that one. So two chapters are finished. I'll have, the second deadline come in, in end of January, sometime around that, and then we'll have the early release out and the full book comes out, I think between August and September next year. Excellent. Excellent. You have a lot of incredible stuff going on. And, you know, I know a lot of people, your time is incredibly valuable. A lot of people want to work with you on these specific topics. If we were to dive into one. So you know, you mentioned you were doing research at UCLA before doing industry work you worked on intelligent agents and auto ML. Can you dive into, you know, First of all, like, what is an intelligent agent? It was hard to define because again, the first thing is defining what is an agent. And you know, what can we say to have agency, which is basically the ability to think for itself and what my research work concluded was there are different levels of agency that any organism does have any living organism, and obviously they're non living organisms as well. But when we go into intelligent agents we can sort of break it down by, yes, it needs to have some sort of agency, which is to be able to plan and to be able to execute tasks. Again, there's, there's a common perception that, you know, humans do have their own agency and, you know, they are, they are somehow this self content. I'm not sure what's the exact word for it. But basically, we do have some sort of conscience, which we believe that machines don't have. But I think it's more like an ecosystem which does drive our decisions. So in terms of machines as well, as long as they have an input output. So for us, the goal is to be able to optimize for happiness. The goal is to be able to reduce the pain that we have at all times. And that's, that's the goal that we're working towards. It can be, you know, optimizing for short term happiness, or it can be optimizing for long term happiness. When it comes to pain, it can be optimizing for long term pain, or it can be optimizing for short term pain. So those, those were pretty much the principles that I was working towards, which is what would be those kind of goals that any agent would have. So for for an agent, it could be, it would very much be defined by the creator of the agent itself, which is, you know, what is the task that it's trying to do, because again, they're in their planning agents and their execution agents, the planning agents are able to break a problem down into very simple tasks itself, whereas an execution agent, it works more like a connector and Orchestrator of some kind. Very interesting. And I, this seems like something that a lot of people that work in data and AI will need to familiarize themselves with and what you're writing about now, the work you're doing through your startup. And you know, like you mentioned, you're working on an O'Reilly book. I'd like to zero in on that as well, but it's, it's, it's certainly, you know, a category of problem solving that people will need to familiarize themselves if they work in data and are interested in future problems in the industry. So that being said, you know, tell us about the book you're writing. You're writing a book on LLM Ops. So after working in the industry for a while I quickly realized a lot of stuff that we were building, we were incurring technical debt as machine learning engineers as data scientists and within the company itself, I started transitioning more towards MLOps itself. When LLMs came around, most of the challenges that were already there in machine learning are now 10x'd. And as we go further, as we see more complex models, I feel like these challenges are going to increase experiment. Exponentially. So some of these challenges are around for for LLM Ops specifically, which is, I say, you know, the first challenge is more so around security, which at this point, a lot of people are talking, but companies are considering, but it's, it's still sort of like swept off the rug. If we could say so, which is yes. Oh yeah. We don't really know if the predictions are reliable. But you know what, we'll take it as an afterthought. So that's, that's one thing. The second challenge that there is, is basically around scalability, which is how are we scaling the resources to be able to make sure that we're able to stay cost efficient and, you know, cost efficiency is one of those things, which I feel like, you know, is one of the stopping factors for a lot of companies that are investing, because as we go further, fantastic right now, the models are not super expensive and the costs are coming down for OpenAI and all of these API based companies. But as you're integrating more and more models within your data infrastructure, as well as you're storing more detail within your data warehouses. That cost will increase and, you know, there, there was that one question which was there with Mother Duck TV that I was really fascinated by which is how much data do we need to store and how much can we get rid of eventually? And when do we decide is that point when we can get rid of that data? Because it, it stops being relevant or it's, it's more of, it becomes more of a debt than it is an asset. It becomes a liability. So that's one thing. Second goal. And the third is basically draw business. Building LLMs in such a way that they are able to be reliable. Because again I don't feel like there's enough discussion on things like, you know, prompt drift is one of the things that people are talking about. But what about data drift? What about concept drift? What about the dependencies on which we are sort of building stuff? A lot of them happen to be unreliable. Yes, there are too many packages, but how many of them will end up being managed over a period of time those, those are still questions, which I feel like are not really answered that well. So it's like, you know, fantastic. This is a good time for somebody to talk about operationalizing these large language models like fantastic. You can build one last language model and press everybody else. But can you put an infrastructure and product around it, which tends to add a value because at the end of the day, sure, there's a lot of it was meant going into the field, but there will come a time when people will say, what is the revenue that you're driving? Are these models? The fancy, this isn't really kind of fascinating people for a very long time. Yeah. Very cool. And I, I want to zero in on one of those points. So your, your book, LLM Ops, Managing Large Language Models and Production. You, you had a great point, which is, you know, yeah, you can build a lot of technology around this, but I want to ask you, what does it truly means to operationalize LLMs? So I think there was this one big discussion, which I'll reference, which is what's the difference between LLM ops and ML ops. And I think that would be a really good point to start. So I I'll say to that, which is why a lot of competence throughout the model pipeline remain the same. Yes, we're doing the air collection. Yes, we're choosing a base model. We're doing some sort of fine tuning. We're doing some sort of evaluation, deployment, monitoring or reliability engineering on top of these models. Yes, that's all there. But the big difference is These models are no longer just models. What I look at them is basically more like connectors and orchestrators in themselves, and that's, that's where the big difference is, which is they're, they've stopped being a cog in the wheel, if you could say so, and they've become the entire view where you're interacting with the models directly and they've become integrated in the product in such a way that you're not really thinking about just the model performance. As a standalone feature, you're thinking about the entire product experience as a whole, where things like latency suddenly come into picture, things like security, or how are the people interacting, what's the PII, which is personally identifiable information that's going into the model in real time, or, you know, what's the language for the model looking like? So those are those are all the things that make a big difference. And that's one of the reasons why I wanted to write this book, because I feel like, you know, while the tools may, remain same, which is yes, we're still using Bento, we'll still use Sheldon, we'll still use Shrin most likely. But there's a very good chance the way they become integrated into our entire infrastructure becomes very different in the stages at which they get integrated will be quite different. Excellent. And describe the day to day of someone who's doing LLM ops. That's something I'm super curious about and I like how it differs from working as a ML engineer or a data scientist. So one of the big questions, which are one of the things which I've been trying to do, which is taking the focus away from picking up the new recent model and going more towards can we build an end to end pipeline for this? And what it means by that is now the pipelines look different for so very simple thing, which is let's talk about like the data collection or the data management stage. So. The first thing is basically how are we dealing with noise management, which is identifying and removing all the irrelevant information? How are we dealing with data augmentation? How are we, obviously, tokenization is quite easy, I would say, because you can use tokenizers available from HuggingFace. How are we dealing with data deduplication or data sanitization? Those all things sort of need to be integrated within the pipelines itself. And then there's evaluation that gets integrated, right? Over at that stage, then moving on to the base model, how are we picking up the base model itself, which is how are we controlling the customizability? How are we controlling? How are we making sure that we remain cost optimal? Or at least we do have some sort of parallel deployment run again so that we can sort of focus on yes, this is a feature that we want to release, but you know, what's the performance of the existing model itself? And how much does that differ? So it's, it's very much like it right now. For me, it's very much around pipeline design. It seems like it's, it's, it's a, yes, you're working with data, but it's, it's a different class of problems where it is, you know, maybe a, a data engineer might be more focused on, Hey, let's just move data between various pieces of our infrastructure, like our database SAS apps into data warehouses and data lakes. And once data's in the data lake, you know, we can kind of figure out what to do with there. But with LLMs and machine learning, there's this, you know, making sure the data's clean and precise. Right. You have like, accuracy is, is, is so much more important because ultimately these LLM Ops, like you said, they're agents, right? They're, they're gonna Yeah. You know, automate decisions and do this type of work. So how would you say the the tooling differs? So the tooling, I would say, more or less would stay the same, which is because, again, there's, there's that difference, which is most of the ML companies, they cannot keep building tools for the the old machine learning models. They are, they want to raise money, they want to stay relevant, so most of them have already transitioned to providing support for large models. And for now, obviously, large language models, but soon large version models, and then eventually multi model models itself. So, when we talk about companies like, let's say, Kubeflow or MLflow, they've already provided tooling for large language models. So I wouldn't say that the tooling in terms of the providers would change. Sure, there will be a few new providers that will come up. But that does always happen in the space, doesn't it? So my concern is more like not really, like, the big change is not really around tooling. The big change is around goals or what we can accomplish. And for these models, what that would be? Which is what's the relevancy of the inference that you're making? And what is the latency of the inference that you're making? The third thing, if we could say, is how are you managing the scale of your entire operation, which is the model as well as the data that you're dealing with and all of the pipelines that you're running as well, because there will be induced latency if there's evaluation happening at so many stages, or if you're using a multistage microservices architecture as well to run different models. How does the complexity work when you're running each different model as a service as well? Excellent. And, you know, you have a great academic depth on this topic, along with the industry view and the scene implementations, which has inspired you to, you know, start working on your book and your company, which we'll get into that. But I also want just your high level view on the, on the current state of AI and LLM ops in the industry. So I would say there are not a lot of people come focusing on operations there's, again, like I said, there's, there's far too much focus on the next big fancy model. And one of the reasons for that was having this discussion with on query. About two, three days ago with Greg, Greg Kramat. And he was like, you know, there are, there are companies that are trying to hold back on building pipelines and building infrastructure around a particular model because they feel like the next model variation that comes in may not require all of that infrastructure may actually simplify a lot of things. So what's the point? Wouldn't that not be technical debt in the future? And. You know, the answer to that is very simple, which is there will be complexities with text. There will be complexities with vision. There will be complexities with images. Or, you know, with videos as well, there will be complexity with audio as well. Knowing what are the, what are the key factors that you want to optimize for with languages instead of focusing more so on what is the tool that we can use by focusing more so around processes would make a lot of difference for companies. So having like a process oriented mindset with any sort of model that you're using right now, is basically the key to scaling in the future and the key to successful deployments in the future. Just because there's a great model out there doesn't mean it will not degrade with time or the performance will stay the same or there will be more companies continuing to work on those. So those are things I don't feel like most people are considering right now. And while we've seen that in person with the performance decline in GPT 3 and GPT 4 models where being closed source models, again, we don't even know the updates that are going in the models itself. So yes, there, there are plenty of people who built these very complex prompt engineering pipelines and they end up being useless after like one update because there's substantial amount of prompt drift with the new model. But again, one of the big reasons for that is because they haven't really built a process around thinking, you know what? What is the key thing that we are optimizing for? How does this model actually work? What is this really optimizing for? They don't have evaluation in place where they can really very quickly just patch things up together. So that that big gap there is basically what's the big gap across the industry and in my conversations with people and, you know, there are there companies like Waits and Prices that are building really nice tracing tools, but they're very simple right now catching very basic things, which is are there any system failures within the pipeline that you're building itself? But I think we need more than that within a tracing tool. So within any sort of observability tools there are. Excellent. And tell me about, you know, what you're working on at Abide AI. So the goal is more so to work on just evaluation itself, which is why the name Abide, because you want the models to abide the rules. But again, I personally was more fascinated with you know, there's, there's this big vision I had since I was quite young about not having dependability on on other humans, but instead of machines where they sort of exist around us as more like companions taking care of us. Not, not as human companions but more so like, you know, where the technology becomes smart enough. In the sense that if you're sleeping on a bed, it should automatically check for your body vitals. And the moment you wake up your day should be designed as such for your optimal performance. It should automatically give you a recommendation on how much water to drink, you should go for a walk based on your sleep and all of those things. So I've been very much fascinated by that whole IoT kind of space, and I feel like. While a lot of models will come in, but the big questions when we look at these any sort of HTML devices, if you could call them so, or you call them tiny devices is basically like you have very small sensors or you can store very limited amount of data so you have some sort of resource constraints as compared to working on very, very big devices. So those are basically the devices which I'm trying to optimize these large models for. So right now, very much in early stages where I can't say, okay, you know, we've established this thing or we have entirely worked through this thing. But it's, it's very much so optimization, making sure that we're able to run these very large models seamlessly on these very, very small devices. So, you know, my IOT dream can come to where everything is sort of personalized for your own preferences. And so how, like, what optimism, maybe this is your secret sauce and you can't share it, but I'm going to ask you anyways and you can tell me whether you can get into that topic or not. Like, how do you optimize it so that I can run on you know, these small devices like IOT devices and things that don't like have you know, massive amounts of compute, storage, memory, GPUs, et cetera. So I wouldn't say there's a secret sauce because the techniques are pretty much established in the field, which is there are a lot of people who are focusing on, let's say, quantization. Where I'm focusing more so is basically on neural architecture search, which is basically using the best strategies to be able to optimize for. What is the model that should be able to run for that inference itself? So that's, that's the key thing that I'm focusing on because not every model is built the same and you don't need a big model for everything as well. Excellent. Excellent. And you know, that's such a great point. You don't need a big foundational model for everything. You can have these smaller, precise models. Like what is it like from a technical standpoint? What does it mean to have like a you know a larger model versus like a small, precise model and you know what enables it to be, you know maybe we call it like a smaller neural network or it is, I'm very curious about that. So one of the key big differences is basically a number of parameters of the model itself. The higher the number of parameters, obviously, the more information or the more relationships it's able to store. It would come down to two simple facts, which is are we trying to build a model that stores a lot of information about a lot of different things? Because that's what these very big models are doing, which is they have, they're more generalistic various for very specific application, which is when we are working with sensors, we don't need a wide space of application itself. The second thing is basically a more focused around the operational scale of these models which is you could say do we really need to scale it to more things as well, which is, do we want to use the same model for, all the applications that we have internally, or do we want to have a model specifically for each one of those different things? So those, those are the big differences where I feel more like generalized models are fantastic, but they are fantastic when you're doing copywriting. They're fantastic when you're doing generalistic applications, but not every model needs generalistic applications. So when it comes to the selection itself, there's advantages and again, maybe one more thing I'll say over there is there are there are ways to a lot of patterns that are being stored in the model or a lot of learning that we're storing within these parameters, some of them are repeated, because again, the data that is being presented to them, we're not looking for linear independence within the features itself. So you can considerably reduce the size of the models itself by making sure that the data is unique enough. And what that also does is if the data is unique, there's less chances of an inherent bias, as well as memorization, which is the big question that people are asking with large language models, which is, are they just putting out information as it is, or you know, are they remembering circuit thing, which remains in the database itself? Yeah, it's, it's absolutely fascinating. It sounds like the problem you're solving is definitely a category that is going to require some in depth solutions like the one you're working on. So with Abide AI, you know, who is your main audience? You know, who would actually use your product? That's something we're still working out. But for me, I think the main ones would definitely be like organizations. So for like companies or large buildings that are investing in like bare architecture or, you know, more clean solutions or energy efficiency and all of those things. Because that is the one thing that we also sort of need to reduce, and there's, we need people to sort of focus on that space. So, why, yes, in a way, it's more like, I think, focused towards two markets. One, you could say luxury market in the sense, like, people who want all of that comfort and don't care, like, people who love gadgets for the sake of loving gadgets, more people like me. And the second, the kind of organizations that are interested in saving costs. Excellent. Excellent. Yeah. And those are really critical problems to solve around, you know, cost reduction and organizations providing, you know, these ai powered experiences. And, you know, and, and it's so interesting 'cause, you know, the, the ai, so much of the discussion is around the technology, what it is, how it works. People, and I asked you who it's for, and I asked so many companies this, like, you know, who's going to implement AI, like who are the end users of AI going to be? And everyone's still figuring that out. Yeah. You know, whether companies will establish a chief AI officer. Or if the, the CIO will establish, you know an AI practice under their work or data engineers will sort of, you know, they're adjacent to AI because they have all the data, maybe they'll just absorb AI to, or bring on some AI domain experts, or maybe it's fully its own engineering function. It seems like so many companies are working through this. So I want to get your take, you know, what's your vision for the future of LLMs and AI? My vision? So I would say there's a lot of information that is available around us. Some of it has been extracted, some of it not been extracted. I think my big vision is that we would be able to build models that are able to make take advantage of all of that information that is available around us implicit or explicit to be able to make our lives better. And that could be different things for different people, which is one of the best things why LLMs took off, which is the ability to be able to personalize. The user experience for every single model where we've gone towards, you know, these boring models that work the same way for almost everybody towards bringing on that perspective of FCI to our work. Excellent. Excellent. And, you know, I think this is going to be you know, one of these topics that, you know, people are, you know, once you're past the, the, the foundations of knowing how enterprises can use this technology and how, you know, all types of organizations can use technology. The next is really operationalizing it. So if you're, Yeah. If you want to operate operationalize LLMs and AI, I think the work that you're doing is, is, is really incredible. And, you know, because you have both the, the, the academic depth and the industry knowledge. And, you know I think everyone appreciates your perspectives on this as well, so I'll definitely be following along with your work. I think the big question right now for almost everybody in the industry is do we build the things that we want to, or do we focus on monetization, because if you're trying to build the things that you want to, the support isn't really there because the models aren't reliable enough, or there are quite a few technical challenges in the way to getting there, so pretty much everybody is like, yeah, we want to build, we want to build, but are we sure that these are the right models? Are we sure we're ready for the regulatory and the compliant risk that comes with these models as well? Yeah, it's all very, you know, interesting and new topics. And, you know, the people who've already done it are going to have the most leverage to sort of scale it out and operationalize it. And, you know, that being said, I think, you know, to my listeners, people should follow along with with your work. Where, where can people follow along with your work? That's a question I'm still trying to figure out, which is, there's stuff on my website I try to put up but again, I'm so focused on the book deadlines right now, it's, it's very much like, sometimes you'll see me on Thread, sometimes you'll see me on Twitter, sometimes you'll see me on LinkedIn, but my website will have more of like, serious work so probably, I think if you don't want to hear jokes, it's then maybe just go to my website and sign up for the newsletter that's, that's where I'll do the serious stuff or announce things. Excellent. Excellent. And we'll have those links down in the pod description for the listeners who want to check it out. So you have your, your website you're, you're, you're on Twitter. I'm at GoAbiAryan everywhere on LinkedIn, on Threads, on Instagram, on Twitter, and I get bored with social media. I get annoyed with social media, go on a different one, then come back. But Twitter is certainly where I'm most active, but don't expect ML content from me on Twitter. It's a lot of, it's a mixed bag. It's very much a mixed bag. Well, either way, I mean, if we are privileged enough to see one of your your rare tweets, we know it'll be, it'll be great. The good thing, which will be coming out very quickly, is I finished, like, a 30 page report on LLM Ops for O'Reilly, which will be published on their website right now. They're in the editing post production phase. So most likely it should be published around January or December. I'm not entirely sure it depends how much time they take, but that would be a fun introduction to my book as well as give people the framework, that I'm sort of working. Excellent. Excellent. Well, Abhi, Arianne, thank you so much for joining today's episode of What's New in Data, very excited about your work. It's very cutting edge, both from a, you know, technical depth and, and industry application perspective. So we'll definitely be following along with you and to the listeners. Thank you for tuning in today's episode. Abhi, have a great rest of your day.