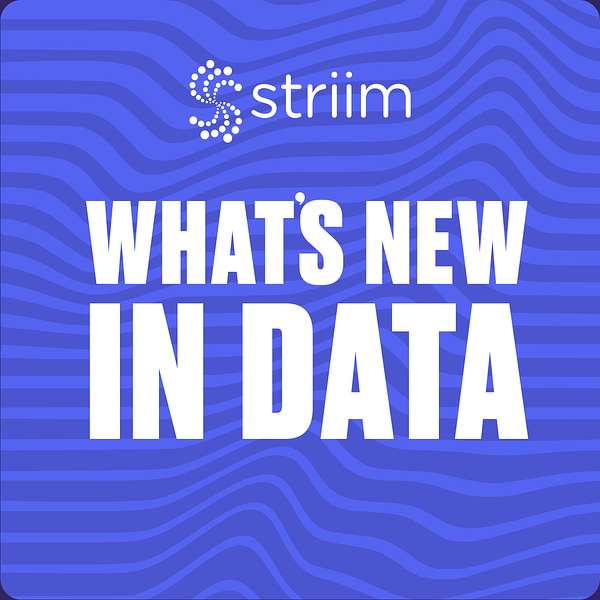
What's New In Data
A podcast by Striim (pronounced 'Stream') that covers the latest trends and news in data, cloud computing, data streaming, and analytics.
What's New In Data
Blending Philosophical Insights with Cutting-Edge Data Techniques with Sawyer Nyquist
Embark on a transformative journey with Sawyer Nyquist of the Data Shop, as he reveals how his liberal arts and theology background fuels innovative approaches to data solutions. Our dialogue with Sawyer spans from the intriguing intricacies of Microsoft Fabric to the development of his educational Udemy class, equipping you with the expertise to navigate the often-mystical realm of data. Get ready to unravel the tapestry of service models with his delightful dining analogy and witness his dedication to empowering data aficionados through practical knowledge and application.
This session also casts a spotlight on the human elements in the age of AI, dissecting the evolving challenges of ensuring security and the nuanced skills data practitioners must refine to thrive alongside technologies like LLMs and GPT. We don't just stop at the technicalities; we traverse the heartwarming narratives within the nonprofit sector, uncovering how data plays a pivotal role in magnifying their societal impact. It's a rich exploration of how nonprofits utilize data to navigate their unique challenges and the gratifying experience of leveraging data to drive meaningful change. Tune in for an episode that promises to elevate your understanding of the data universe and its extraordinary potential in both business and the broader community.
What's New In Data is a data thought leadership series hosted by John Kutay who leads data and products at Striim. What's New In Data hosts industry practitioners to discuss latest trends, common patterns for real world data patterns, and analytics success stories.
Hello everyone. Thank you for tuning into today's episode of What's New in Data. I'm really excited about our guests today. We have Sawyer Nyquist. Sawyer, how are you doing today? I am doing well, John. Pleasure to be here. Excited to have some data conversations this afternoon. Oh yeah. Always a great time to talk about data. And, you're doing some really cool work, both, the cloud side of data with Microsoft and Azure, but also helping nonprofits with their mission, great work across the board. Sawyer, tell the listeners a bit about yourself and the work you're doing. Thank you. Yeah, my name is Sawyer Nyquist. I'm based in the beautiful woods of West Michigan, and it's winter here, hoping for some snowfall very soon. I run a shop called The Data Shop, which is a small boutique data consulting firm. It's a solo practice. It's just me, always will be me. And the main heartbeat of what I'm doing at the data shop is helping data teams define, identify, create and deliver value for their business and communities. And as you mentioned, I have a particular focus and interest on nonprofits and helping them further their mission and the impact that they have in their organizations. So that's, that looks like strategy and vision work as well as kind of technical infrastructure, data team processes and delivery. Helping data teams execute better is kind of my, my passion and what I've been doing at the data shop. Yeah, that's amazing. And you have the perspective of both the technical side of it implementing great data pipelines and reports and analytics, but also tying it to the mission. And in making sure that it's bringing value to the business. So it's great to have, you know, a glue guy like yourself kind of bring it all together. Yeah, my background is not technical. I didn't come from a computer science background or software engineering. I have, you know, religion and theology and liberal arts humanities degrees. And so I grew up in the business world and then the liberal arts space and that kind of gives me a different perspective or approach to the data world than somebody who maybe came from the technical side, and that's kind of the fun parts about data as it ends up merging us and people from lots of different domains and backgrounds into, into data. Absolutely. You've been working in data analytics and data engineering for a few years now. What drew you to data from, your background of theology? Mm-Hmm. Well, started my career for the first several years working in kind of business and logistics and operations at a travel company. Kind of loved the strategy and the marketing and the sales side of things and all that world. And then I found myself stumbling into a technical support role at a software company where I was still focused on the business side of the house, but somebody told me I needed to learn SQL to do better, do better at my job. And so I still brought with me kind of the love and skills from the business world and then started dabbling in technical skills and a switch kind of flipped in my head. I could all of a sudden SQL made sense to me. Data became a real important draw for me and the space between those two worlds of like the technical and data teams and then the business world realize that there's a gap that exists in a lot of organizations between those two spaces. The data team doesn't always talk to the business. The technical world struggles to speak business language. And I figured out I could talk to both teams well, and kind of the merging of those two worlds is kind of like that business intelligence data analytics space. And as soon as I figured out that that was a domain and a, and a career path, potentially, I dove in as deep as I could and having a fun time just chasing that Venn diagram, that overlap between the business world and the technical data world. Excellent. And you recently came out with something really cool, a Udemy class, certification
called Microsoft Fabric:The Complete Guide Future of data with Fabric. And it's really a Fabric master class. First off, it would be great for you to tell the listeners all about, Microsoft Fabric and how people can use it and why it's valuable. Yeah. Fabric is just a really exciting space right now. And I mean, I worked on filming that course for you to me because I wanted to learn the tool, it was new and exciting for me too. And I was like, what better way to, really get in and to try to teach somebody about the tool. And so I spent three years at Microsoft, I was kind of a little bit behind the scenes watching as this thing was getting legs internally. And and then when it came out in May, 2023 under private preview, I was really excited to get started with it. Well, just to back up for a second to think about how Fabric fits into the landscape of data. I like to think about it from an IaaS, PaaS and SaaS perspective and kind of just that progression between the three. And so I explain kind of those three different paradigms with an analogy around like eating dinner. And there's three different ways you can choose to eat dinner primarily, right? You can cook dinner at home, you can get takeout or have Uber Eats deliver, or you can eat in at a restaurant. And, and that's how I think about kind of the, the differences between some different models of how a data platform could develop. There's three different ways to eat dinner. There's three different ways to kind of build a data cloud data platform and all of them have different levels of responsibility and cost and expectations on you. So if you're cooking dinner at home, you've got the expectation of having groceries and having a kitchen and the skill and know how not to burn the food. You're responsible the food is all the way cooked and it tastes good. If you order takeout, a lot of those responsibilities about the food get outsourced to somebody else. And then you're just kind of responsible for having a place to eat it, silverware, and maybe a little bit of cleanup. And then the last model is the, I can eat at a restaurant where they take care of all the food. They take care of all the cleanup. I just get to show up, eat and leave. And that's kind of, that's what the difference is between IaaS infrastructure as a service, PaaS platform or SaaS solutions. And we've been seeing really a progression across those 3 models from IaaS to PaaS to SaaS and the, you know, in the data world, we certainly had a lot of I have solutions where you'd have a VM and you'd be SQL server or your Oracle database living in a in a VM or or on prem server. And then past solutions have been the dominant place for a long time. In Azure, you have your Azure database or other past cloud tools around data factory. And then SaaS is the last kind of progression along that cycle. In terms of ease of use, in terms of speed of onboarding, in terms of the amount of expectations placed on the user to manage. And that's what Fabric is. Fabric is the SaaS. That's what Fabric is all in one data solution it's that you go eat out of out out at a restaurant and everything gets taken care of for you. You're just responsible to show up and like the maintenance, the scaling, the config most of the configuration infrastructure gets taken care of place behind the background. So that's kind of how I like to position Fabric. If you're used to IaaS solutions of spinning up a VM and having SQL server on there or even past solutions in Azure. Fabric is just like a different look and feel as a SaaS product, you're familiar with this, John, just from like stream being like a SaaS platform, but it's just a different mindset than what we've had in a lot of the data platform world for a while, and it's kind of an exciting proposition there. Yeah, it's, it's incredible. And just the amount of value it brings. And I work with teams across the board from, on premise to PA to cloud pass to IaaS finally SaaS. And you just see that teams that use SaaS are just able to move so fast, they're able to do more with less. And the way Fabric is, it's becoming super popular, and helping people move faster and unify more of their existing data and infrastructure. It unlocks a lot of innovation within companies for sure. Yeah. And there's a number of things inside of Fabric to that are really exciting. So 1 of the things that Fabric brings to the table is a different pricing model that I'm excited about where if you pay for a specific compute capacity, that covers across all the different workloads within Fabric. Fabric is really quite, broad in its scope. You've got data warehousing, data engineering, analytical workloads, data work, like data integration workloads custo workloads, all sorts of different workloads across Fabric. And typically those were all like separate past services you'd spin up, you pay for separately. They all kind of get bundled into a single pricing model so when you talk about the simplicity of SaaS services, that's one way that like Fabric is really is unique and easy to get. Involved as soon as you buy a capacity, all of a sudden you get access to all the different workloads. So I think the pricing model is kind of an interesting framework and really became kind of a and how it's positioned as inside the power bi world directly. So, there's, you know, I don't know the number, but it's in the millions of power bi users out there and and hundreds, hundreds of thousands of companies already have power bi stood up and they just like Fabric just. You know, under the cover of night, basically snuck in there and like, took over the Power BI portal. And now it's like, all these Fabric tools are at the hands of Power BI users. So if they're Power BI users are just so comfortable in the portal, all of a sudden, they've got a SaaS spark runtime, which has never existed before. Like, there's been pass and there's, there's certainly been like, Iaas, SaaS workloads, but that was a pass or a SaaS spark. Runtime, which is exciting. So you can spin up a SaaSs spark cluster don't really even have to spin it up, it just kind of starts in like three or four seconds and you're typing spark code. Yeah. Oh, go ahead. And then there's also like co pilots that are getting baked into these things too. So if you're writing code in a spark notebook, if you're writing a power BI report, There's a co pilot, if you're building data integration pipelines, there's a co pilot. And so Fabric's like really like touching all these different spaces and then empowering co pilot into each of them to make, to make it just super accessible and really quick to value and quick to action for, for users there. Yeah. And I, I love how it ties all these things together. Being able to go from loading the data to, transforming it for analytics purposes. And then, of course, Power BI is a huge component. And then you have this general purpose compute layer for whatever you're doing. Like, hey, I have a bunch of JSON that I want to flatten into tables but it's nested. That's a great use case for like Spark or doing machine learning for it. And having one pane of glass just simplifies it so much for data teams. So that part's definitely great, I'm seeing teams getting that value for sure. And and like you said, the pricing model sort of just simplifies it a lot. You don't have all these moving parts. I had Everett Barry from Vantage come on to talk about specifically the pricing advantages of Fabric versus other Spark platforms and he even pointed out, yeah, there's like big advantages that just simplifies it. When you talk to enterprise teams that do a lot of work in the cloud at massive scale, it's really the kind of the simplicity that they value the most. Obviously the bottom line price is very important, but the simplicity, and not having too many moving pieces to manage and monitor all with their own usage consumption based pricing, right? That can, that can kind of deter things and, you know finance and procurement kind of gets in the way, but now it's really simple, right? You have the SKUs for the different compute engines and it's all kind of bundled together, right? Yeah, yeah, and that's going to be a hard kind of mental shift for a lot of people who are familiar with provisioning resources and managing those through Azure. And I think you'll find a lot of small organizations can quickly get up to speed and find value with Fabric and the ease of use of the tools. I think it's going to be a little bit of a slower game for large enterprises to figure out how to adapt a lot of their Azure administration and security and networking requirements, fitting those inside like the Fabric as a very new SaaS solution. So there's not all the same feature rich networking and security and frameworks that you would have in like a really robust platform like Azure. So it'll be it'll be interesting to see how that develops as as it goes and Fabric knows it's not a full product yet. It's not a feature parity with everything in Azure, but it's going to hit a lot of quick wins for smaller companies. There's gonna be a lot of use cases within large organizations, but that enterprise kind of data platform at a large organization is going to be a little bit of a different challenge for companies to think through how does my azure admin fit into this? How do my cost considerations fit into this? And how do we figure out governance and managing these different data assets and these lake houses and warehouses and workloads across dozens of teams and tens of departments. Yeah. The governance is obviously a critical piece of this as well because especially at the business level, if you have tons of data and dozens of people and dozens of teams interfacing with it. You really want to make sure that all the data is cataloged properly and accessible and otherwise you're kind of building the dreaded data swamp, so to speak of, just a lot of unusable data that you're constantly paying for. So how does Fabric help in that respect? Well, I think that's a push and pull when you're designing something around ease of use and SaaSs like if we make it so easy then you get a proliferation of people building things in the platform and it spreads like spaghetti as opposed to like a centralized and really unified governance and constrained model there's always a push and pull between those 2 and the easier you make the platform to, to spin up a cluster and to spin up data warehouses and lake houses and pipelines, the harder it is to kind of reign that in and keep good governance over it. So that's that's a battle we're still learning and trying to figure out. Purview is kind of Azure's governance platform that's still coming into Fabric, and we're figuring out how that's going to play into the picture in terms of giving visibility and lineage and cataloging to data assets. As I mentioned, some of the security things aren't there at the level we would want them to be yet in Fabric so, in terms of individual asset security and who can do what and who can create what, where some of those pieces are still coming to fulfillment, in Fabric, so this is why I think some large enterprises are looking at Fabric they're like we want to do some stuff there. We're trying to figure out whether we can yet. And smaller companies are like, we don't need those same requirements. We can go now. And that's a little bit where we're at. So, you know, I, I saw some of this from the inside at Microsoft and as a little bit of an external viewer now that, you know, it's not a hundred percent there and it's going to be growing a lot and evolving a lot. I will say they are moving very fast with Fabric and I'm struggling to keep up with the changes they're deploying because there's so many different parts to it. Every month, lots of new features are hitting the hitting the market, and so it's a fun space to watch as it develops quickly. Yeah. Speaking of fun spaces to watch with fast development let's get into some of the trends in, in data and AI. What are you seeing in terms of things changing very quickly in the data and AI stack? A few pieces have come to mind, even out of this conversation from Fabric is LLMs and GPTs and copilots and how those are changing the data practitioners life. And this isn't a new conversation we've been talking about this a lot over the last year, but the tools are integrating much more heavily now. And they're showing up in all of our different places. Like, GitHub copilots been out for a little while. But Fabrics now introducing it into the native domain of the coder inside Fabric. It's going to continue to pop up and I think that the skills that data practitioners are going to have are going to be continually adapting and changing. If you had built your career around being a very skilled coder, that's going to become a more commoditized skill as GPT gets better and better at writing code, or as you get more specialized LLMs that can write code in specific contexts and know where you're at. So I think, or if you were You know, very skilled at the things that GPT and LLMs can do. You have to figure out what is your skill set look like now? What is your career path look like now? And so that's that's what area I'm trying to spend time thinking about. Like, what does my career path look like? What is what kind of services and offerings do I need to position myself? Well, at to continue to deliver. To be unique and distinguishable from what GPTs are doing now. That's been an interesting place. I think data, data teams and data professionals need to think about a lot. How am I different than GPT? Not just how can I use it, but how am I developing unique skills that GPT can't replace? Because it's going to move faster than you are. How can I develop skills that GPT can't replace in the In the next 5 years so that I still have career stability. Yeah. And when you look at a lot of the real world implementations of AI in more of a enterprise or consumer lens, especially when there's risk to it. There's still heavy use of human in the loop, to sort of oversee things. Let's say you're an insurance company, giving out quotes and you can have ai part of the process there, but you don't want AI to just like autonomously go do all the work for you 'cause there's legal implementation implications and all types of issues that could arise. And I think at the same time from a business standpoint, yeah, it always makes sense to have that human in the loop. Are you generally seeing the same thing with AI rollouts? Yeah, there's, there's certainly risk elements and required like that human element attached to what you're doing. I think the interesting pieces is, is around what, what is going to be replaceable and what will GPT continue to get better at and how do I position myself well alongside that? There's certain things that GPT won't be able to solve in the near future. Team culture, human dynamics, business stakeholders, interacting with data teams, and overall thinking about patterns and frameworks for solving business problems. GPT can do some of that stuff, but a lot of that is still very human style work. And then there's the larger architectural patterns and larger problem solving that it's hard for GPT to get its head wrapped around right now how do we solve organizational challenges around implementing data solutions and data products effectively, like those are still very human problems that need to be solved. And as opposed to, like, I need to get faster writing Python faster writing, Python is not going to be the best thing for your buck right now because GPT is going to be faster than you and will only get faster than you. And so I think it's much more like, how do we think about frameworks and patterns and people challenges and organizational challenges? Those are the things I don't see getting solved by LLMs. Yeah, anytime soon. And so I'm trying to invest my time in that space. And, and how do I understand the problems well enough to to be that human oversight of what, what LLMs are doing. You need to know enough Python to know when LLMs are crazy and not giving you something useful. And there's all sorts of fun stories about that that have come out of people writing, you know, prompting GPT and getting weird stuff and knowing how to fix it. So it isn't. You're not completely out of the game, but your role is shifting from, from writing code to thinking about frameworks and patterns and overall principles that drive what you're trying to do. Absolutely. I always say that the growth of AI over the next 10, 20, 30 years really makes everyone's own definition of what it means to be themselves more important than ever. I always think back to one of the live episodes of What's New in Data that we did in San Francisco, where we had a Redema Khan, who's a VP at Dapper Labs, and they have this AI generated social media influencer and her name's Michaela. Michaela has millions of followers, millions of dollars worth of brand sponsorships, but just a completely generated not real. And that's the future of we're, we're going to be competing for attention and budget and dollars against AI in some sense. So as an individual how can you be above that? And that ties what you said very well, think about organizational frameworks and, and ways to really set yourself apart from the pack. There's probably some people who are more philosophically skilled at this than I am or thoughtful, but creativity is still something I'm leaning into as one of the core things that makes me human. And I don't see these LLMs generating the type of creativity that humans can and so I'm still investing in my own ability to create and the courage it takes to create in new and innovative ways that I think only humans can. And, you know, there's lots of things I could probably outsource to LLMs but in terms of creating and thinking as an individual in that space I'm exploring that world and interacting with humans. So, like the connection and creativity, human connection and human creativity are still the domain that I'm leaning into as what's available for me in the future in a world of AI. Yeah. Creativity is certainly part of what makes you yourself. And I, I also liked this book Strange Loops by the same author of good old Esker and Bach. It's a really good book by Douglas Hofstadter and it's really helping you define what it means to be I, and it's kind of this like recursive loop of like, okay, you know, explain what it means to just the concept of I, right? Like what makes I, you know, me, right? And to be self perceiving, self inventing ultimately there's a lot of self reference there, which is sort of a computer science concepts if you really look at it, but of course, there's a philosophical element, even theological, spiritual element to it too. So that's everyone's journey that's for when to figure out, but like you said, yeah, like becoming a little faster at writing Python you know, probably not going to get the most leverage out of that unless you're using AI for that. Yeah, sure. Yeah. Yeah. So you're also doing a lot of amazing work with nonprofits. Talk to me about that. Yeah, I found nonprofits to be a really interesting space from a data landscape and something that I've like wanted to focus the data shop around. Like, so that's kind of the specific niche and area that I'm spending time in. Nonprofits have a different overall framework than for profits so, at a high level, for profits it's about making money at the end of the day, that's how they deliver value to their stakeholders. You make the most money or create the most value for your stakeholders, financially, monetarily, share price, sales, revenue, et cetera. That's what for profits are, and then not for profits are about creating the most value and experiences for their members. And so that's like country clubs and credit unions. And then non profits are specifically designed around creating the most value and benefit for the communities that they serve. And so the metrics just get different at each level and data world, we think about metrics a lot and how are we measuring what we're producing and creating. And I found that the for profit problems are fun to solve and creating, how do we create more top line revenue, bottom line profit, reducing risk along the way. That's a that's a problem that will always be there and fun to solve. Not for profits are a niche around. Like, how do we create the most value for our members? But nonprofits are creating the most value for their communities. And so that's humanitarian things. That's educational things. That's disaster relief. That's housing. That's economic stability. Which are different sorts of things to measure, instead of measuring profit or revenue. Which nonprofits care about it some sense too they have financial matters but at the end of the day, they're measuring. Are we improving educational outputs and educational outcomes for the kids in the actual program? Are we improving the economic environment of this neighborhood or this community? Are we improving the financial viability of people in this demographic or in this region? Or are we adequately meeting the food security needs for children or for adults in in this market or this area. Like there's a lot of disaster relief that shows up. So nonprofits are solving different kinds of problems that are, that are harder to measure in some ways and that are very soft sometimes to measure. And so I enjoy thinking through those types of challenges of what kind of data do we need to measure the opportunities that are in front of us and the challenges that we're trying to meet, whether it's whether it's related to food or housing or, education or economic development or disaster relief. All those pieces are really kind of unique challenges to solve. And so do we have the data to measure that stuff? Do we know what we're trying to solve? And do we have the data to kind of measure that? And those are different quantitative metrics and qualitative metrics. And then do we have that data to tell the story to our donors? So if you're looking to donate money to a nonprofit, which is how these nonprofits run, is on donations it's sometimes on grants, but on donations the nonprofit wants to have data to tell a story to that donor to say, here's the impact we're making and we measured it. And that's what a lot of donors want to understand is I want to give my money to something that's going to actually make a difference and how can you show me that you're making a difference? And so I get I get excited and passionate about those kind of stories. I have the opportunity to work with a kind of a child safety organization that's focused on like tracking children who were in foster care and in different stages of vulnerability and different stages of house insecurity and financial insecurity and food insecurity. And it's a compelling problems both the people you're working with are great. Cause they're there for a reason and they care about the nonprofit world. But then the stories that come out of the data work that you do, it's like, Hey, we've now through better data infrastructure, better data modeling, better data integration, we've now created a much clearer way of keeping track of the safety of a child throughout the course of the care in this organization, and that has a very real human impact on lives and I like to think that in all circumstances, good data makes lives better both in a for profit and not for profit as well as in a nonprofit world. And it's really fun to start to see some of that showing up and being real. Nonprofits in the space I've focused on because I enjoy solving data problems at all different levels, but I have specifically found the The metrics and the types of stories that need to be told around data really compelling in that world. Excellent. And in some ways it's comparable to kind of proving business value with data across many industries, but with nonprofits, it's so mission oriented, making sure that the nonprofits help move the needle financially for certain groups in different demographics or, you know, you're helping, you know, more people get access to education and that education is actually getting positive results. How are you measure with standardized testing or how are that works? I'm not an expert, but and it really does come back to The data and the analytics. And, I think some of the, the innovation that's going on in the cloud space is an enabler for nonprofits to also better succeed at their mission. Yeah. And you think about the I was talking about the beginning of the conversation, like the rise of SaaS platforms being a huge trend over the last decade in the data world. And how that makes it so much easier and quicker to spin up data resources and to get to value quickly without the same infrastructure and administration and customization required. And non profits tend to be under resourced and tend to not have the same skills that for profits do, it's like skilled internal resources. And so these SaaS platforms and the ease of use that these tools that are coming to market can offer organizations that may not have the same resources or the same skills that large organizations have. Yeah. It makes it that much quicker for them to also join in with what large companies have been trying to do for a while and get them hopefully the same tools and insights and metrics that large organizations have had for a long time. Excellent. Excellent. Well, Sawyer doing so much incredible work, both in the data space and the nonprofit space. And I think people across the board who work in data engineering and analytics would get a lot of value from following you. So where's some of the places that people can follow along with your work? The data shop, I focus a lot on thinking about the question around value and defining, identifying, creating value. And that's what data teams are like at their core, what they're trying to do for the business. And so I put together like a 12 day bootcamp, email bootcamp of something that I've been thinking through the thought process around, like, how do you find, identify, create value? And so I'd invite anybody who's curious about that idea and how they do that on their team. If you're a data leader, if you're a senior member of a team, or if you're a junior member of a team trying to grow into a senior member, connecting your work to value of the business or the direction of the organization is the fastest way to grow your career. So At my website, the data shop dot CEO slash value is just a free 12 day email bootcamp. You know, those emails come directly from me and it walks you kind of through the pattern of how to think about what do we mean by value? How do we, how are we currently doing that in our organization? Are we failing at it? Are we doing great? How do we continue to drive that direction forward? And so that's a, that's a fun place that I enjoy interacting with people through kind of email bootcamp course. And so if that's interesting to you, invite you to check that out. And then I'm really active on LinkedIn that's a fun place where I've made a lot of great data friends. And I've enjoyed the conversations that happen there, lots of things that I think are moving the conversation forward, both around like SaaS and the modern data stack as well as nonprofit world as, as well as like, the market as in general so love LinkedIn. Feel free to find me there and say hello. Excellent. And we'll have those links down in the description for those of you who are listening and Sawyer, it was great to have you on this episode of What's New in Data. And thank you to everyone who tuned in. Thanks so much, John. It was a pleasure to be here.