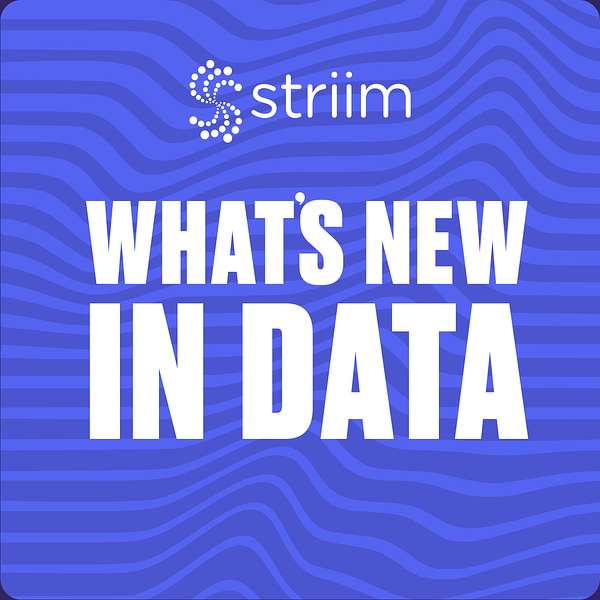
What's New In Data
A podcast by Striim (pronounced 'Stream') that covers the latest trends and news in data, cloud computing, data streaming, and analytics.
What's New In Data
How UPS battles porch pirates with AI (Pinaki Mitra from UPS, Bruno Aziza from CapitalG, Alok Pareek from Striim)
Unlock the secrets of AI's transformative power with latest episode live from Google Next, where UPS's story takes center stage. Joined by AI trailblazers like Bruno Aziza of CapitalG and Pinaki Mitra from UPS, we delve into how UPS is tackling package theft and reshaping package delivery. This isn't just another discussion; it's a firsthand look at how AI and data analytics converge to solve real-world challenges, improving security and efficiency in the e-commerce landscape.
Ever wonder how AI can streamline your business operations? Our panelists, including Sanjeev Mohan of Sanjmo and Alok Pareek from Striim, reveal the nuts and bolts of integrating AI into supply chain processes and the pivotal role of data lifecycle management. From enhancing address validation to offering insights for small and medium enterprises, we uncover the practical benefits of AI and the importance of a meticulous approach to data management. Get ready to be inspired by the parallels drawn between packet delivery and data event observability, and the critical steps for aligning AI with your business strategy.
We wrap up by exploring the broad implications of generative AI across industries, with case studies that will alter your perspective on AI’s potential. Whether it's summarizing legal documents or mining data for pharmaceutical insights, the versatility of AI is showcased in its full glory.
We extend our heartfelt thanks to our live audience and listeners, encouraging you to engage with the innovative ideas shared at Google Cloud Next and reminding you of the importance of robust data foundations in harnessing AI’s full potential.
Join us for a conversation that promises not just to inform but to transform the way you view the intersection of AI and business.
“UPS AI Battle Porch Pirates.” ABC News, Good Morning America. Accessed April 10th, 2024. https://abcnews.go.com/GMA/News/video/ups-ai-battle-porch-pirates-103459177.
What's New In Data is a data thought leadership series hosted by John Kutay who leads data and products at Striim. What's New In Data hosts industry practitioners to discuss latest trends, common patterns for real world data patterns, and analytics success stories.
Hey everybody, Thank you for joining today's live fireside chat here at Google Cloud. Next, this edition of what's New in Data. Super excited about our panel. Today we have a real-world implementation of AI that we're going to discuss here with UPS. First of all, I'll ask the panel to introduce themselves. I'll start here with Bruno.
Speaker 2:Thank you. Thanks for having me. Hi everyone, I'm Bruno Aziza. I'm an operating partner at CapitalG. Capitalg is Alphabet's independent investment fund. Before that, I was running outbound product management at Google, so products like BigQuery, dataflow and Dataplex and so forth and I've been in the data space my entire life. I'm also French, so that's why you hear a little bit of an accent. So, but don't hold it against me, I'm nice. I'm a nice French person. I can see by the laughs that that's not very credible. All right, move on.
Speaker 3:Sanjeev. Hi everyone, I'm Sanjeev Mohan. I am a founder and principal analyst at my own company called Sanjmo. Before this, I was at Gartner. I ran the data and analytics practice for about five years and then I decided it's time to hang out with people like the wonderful people from Stream and UPS and Bruno, so I left NS Drive, my own company, two and a half years ago. Now I do a lot of research, blogs, podcasts, webinars and just get deep into AI and anything to do with data management.
Speaker 1:Thank you.
Speaker 4:Sanjeev Alok. Great Welcome guys. Delighted to be here. I'm Alok. I'm one of the founders in Stream and I run product and engineering. I've been in the data space for a long time. Prior to this, I used to run Oracle's data integration product portfolio and I was CTO of a company called GoldenGate, which Oracle acquired, and originally I spent my first 10 years in the Oracle database as well. So thanks, john, for putting this together and excited to be on this panel, and thank you guys for attending. Hopefully it'll be a great fun session.
Speaker 1:Pinaki.
Speaker 5:My name is Pinaki Mitra. Thank you for inviting me in this panel. Great to be here. I work for UPS Capital. It's a subsidiary of UPS and I've been working in the data fields for almost 25 years, so currently I have a team. I manage data engineering, data science and analytics for UPS Capital.
Speaker 1:Excellent, thanks so much. So we're going to start the panel by watching a video of a real world implementation of AI. Prolific mathematician Andrew Ng says AI is the new electricity. We really have to reimagine the core vital services that we have in our economy with AI, and there's no better example than this. I'm going to start up this video demo with the thank you to the team at Good Morning America for putting this together. All credit goes to them.
Speaker 6:UPS now using artificial intelligence to stop them from stealing your home deliveries. Eva Pilgrim is here with that story for us. Good morning Eva.
Speaker 7:Good morning Robin. It's a real problem. The number of stolen packages is up and an expert telling us after the pandemic, we got so used to getting deliveries that we're now ordering more items and more valuable items, making your front porch a prime target for thieves. This morning, thieves hoping to steal packages from your front door. Better beware. There's a new tool to keep an eye on would-be porch pirates artificial intelligence, ups Capital introducing delivery defense machine learning algorithms that assign a confidence score to potential delivery locations to help determine how likely a package is to get where it needs to be without being lost or stolen.
Speaker 8:UPS has one of the largest delivery databases in the world, and we're using machine learning and AI tools to look at all this delivery information. We have the address confidence score from a one to a thousand, so a thousand would be absolute highest confidence that a package will be delivered successfully, and one would be our lowest confidence. And so there's other choices that we can help merchants use that can increase the chance of a successful delivery.
Speaker 1:Great and with that presentation I want to pass it over to Pinaki, one of the people behind Delivery Defense. We're going to talk about the implementation here. We will switch over to his presentation. I'll pass it off to Pinaki to talk about the implementation.
Speaker 5:Thank you, john. I'm just going to start with just the motivation behind this delivery defense and the address confidence score. So as you can see through this data that e-commerce is steadily growing. For the last I guess, probably a decade it's a steady growth of e-commerce and then during the time of pandemic the e-commerce has really surged, right. So that's. And then when we surveyed with some of our customers the most of the customers that they're pretty confident that e-commerce should be steadily increasing in coming years. So as the e-commerce is growing, at the same time your fraud related to e-commerce are exponentially growing.
Speaker 5:So if you think of the package lifecycle, so the order gets processed, level gets created and then packets start moving through the network, then it gets delivered to the consignee and then if the package needs to be returned, then the return gets initiated. So that's kind of very high level. That's the journey of the package, right, and we call it package lifecycle. So at the same time we observe the losses are also increasing at the same time as the package volumes are growing, right. So the way we see these losses and then when we categorize it, there is this in-transit loss. So you know, when a carrier carries a package, there are things happen in the network. Sometimes packages get damaged, packages get lost, but then there's a big component on this whole loss category is your post-delivery loss and then the return losses, right. So when you talk to the customers, these are two big pinpoints that our customers have. And then post-delivery loss, a big part is the porch privacy, right? So you saw that example that the package get delivered and then these porch pirates they just come and then grab all these packages. Sometimes they target a specific geography and if you look through the data and one given day all these packages for a particular neighborhood is gone, right. So it's a big problem for our consignees. Which is a recipient of the package, for the customer. So nobody wins with this, right.
Speaker 5:And the returns also are getting a big problem for customers because there's all kinds of frauds happening with the returns. So this is you know these things we have been hearing like we talk to all customers, especially the enterprise customers. These are huge pain points for them. So the customers, you know of course they are not staying idle. The customers, they have to solve this problem. So some customers they have implemented you know these different rule-based algorithms right, what they can see through the data. Then some customers, like the high value customers, they have implemented this private security stuff right, so that they can control these losses. Then there are different authentication software and then so there are all different technology tools that different types of customers have implemented.
Speaker 5:But in spite of all these, as we saw through the data that you know, there is still the fraud is a big problem, right? So we have been thinking that you know the data we have from our customers, from the movement of our package to the network and all the receivers of our packages, right, if you think, probably every one of you at some point of time received the UPS package, and then UPS has these millions of customers, right? And then so UPS picks up about, on an average, like 20 million packages a day, and then, you know, in the US it's about 135 to 137 million addresses where packages get delivered. So we have this data and also, from UPS Capital, we provide insurance coverage for the transactional cargo and transactional packages. So what we could do with all this data that we have, right, and build a solution for our customers to solve this problem, at least to help customers, you know, to mitigate this risk, right? That's where we came up with this delivery defense address confidence score, so what we're really.
Speaker 5:I mean, this slide kind of summarizes this, the essence behind it. So all these. So I gave you the package figure. So we took historical package information. So, of course, when this slide was made, it's like 11 billion packages we took and there's 130 million, so now it's about 135, 137 million addresses that we could identify where package got delivered. And then we have all these claims information that we see through the data. Then we have all these returns issues through the data.
Speaker 5:Then you have delivery attempts or multiple delivery attempts, and then there are different features from the package. These are like the big elements in the model. And then, once we have this, then everything we're doing in Google Cloud right, the BigQuery is really the workhorse behind it. And then and this is where we are pulling data from all these different UPS sources and also we're using Strim to pull all this UPS capital information. So I mean, we know, right, if you don't have the data into one place, there is really no ML, there is no AI. So that was the biggest thing for us to keep that build that foundation, the data foundation, where we could have all this data that we need and that's where we're using BigQuery and then from there we're using Vertex AI and then this is where we built our own proprietary ML models and then so we're taking all these and we're running through Vertex AI.
Speaker 5:So these are all the features that are going into model and then we build this address confidence score and this thing has been in kind of keeping that credit score keeping in mind, because people kind of relate to this. So it's in the scale of 100 to 1,000. So 1,000 means that there is absolutely no issue. What we're seeing through the data, we can confidently say that these addresses are purely no issues at all. But the lower you go in the score, then where we see most of the problems. And then one interesting fact if you see on the graph to the right that actually 2% of the addresses that's where most of the problems we're seeing, so 35% of, like, the losses that originate from 2%. So that tells us actually most of the problems we're seeing. So 35% of the losses that originate from 2%. So that tells us actually most of the addresses are pretty safe. But then again we're talking about 2% of $130 million, which is a big number. So that was the first version that we deployed. And then we are implementing more features to the model, right. So we are introducing, like, the latest loss frequency, so earlier we're looking at just the historical data. So now we are taking the recent events that we're seeing and then so it just the models are getting even better and better, right. And then we are taking all these different volume characteristics of each of the addresses and then we're overlaying on the score.
Speaker 5:And then one thing our customers like when we first get this solution, a lot of customers say well, when you have a low score or a high score, what does it really mean? Right, because they want to know that customers really don't care how you come up with this, but what it really means, right. So we kind of came up with some description. When I say the score is 350, is it because a lot of losses, because of the delivery issues or because of the returns? So we kind of implemented that. So that really helps the customer and they know that. Why this score is, you know, a low score versus a high score. So this one we have a little video. So one of our customers, texas Precious Metals, yeah, so they are using it. So it's a good way to see how easy to implement for our customers this solution, and then, once it's done, then I'll explain it further.
Speaker 6:This is Nicholas Berger. I'm a part of our product development team here at Texas Precious Metals and I wanted to showcase some of the features that we've been building with Delivery Defense and to showcase that work for you so you can see how we're using it today. Okay, so let me jump into the demo. We're going to primarily be using Delivery Defense, of course, for for shipping. So here's our ERP system where we're using it. Now Imagine that you're about to ship out a package and you need to check to make sure that the address is a deliverable address. So here we are. We're going to be generating some labels, so I'll first check the item that I'm going to be generating the label for and then go ahead and begin that process and we just have delivery events run immediately as we begin doing this process of selecting a shipping provider, shipping method and editing the details for that before we generate the label.
Speaker 6:So this is awesome it gives immediate feedback to our shipping team and we're able to make decisions pretty quickly. Here's another one.
Speaker 6:I'll just do this one, and the address is different, obviously, and it just gives you a slightly lower rating but based off the information you guys were able to supply, we're just using that information right here as like helper text to explain hey, this is less likely to likely to arrive based on your information, and then, as the scores get lower, we add additional ones. Here's one test where actually it's an invalid address. So we will get that response right back. So this really helps us cut down on oh, this was not the correct address to be sending to. So, yeah, that's, I mean, really basic Down the road.
Speaker 6:We also like to add in the ability to compare addresses. We know that we get a response back from the API that says, hey, this is the address, and just to compare and contrast what we have typed versus what DeliveredDefense sends back. So we'll get that added at some point, we believe. Another way that we're going to be using this at this time is we have another page specific to just testing addresses. So here our team can enter in an address, pretty, pretty straightforward, and then it's a bit, and delivery defense just runs right here for us. So that's also a great little tool that we've added here. All right, uh, thank you so much for coming along with me on this journey and, uh, letting me show off what we've been able to do. Uh, thank you so much for your help and looking forward to what we do in the future.
Speaker 5:So you know we, so you see that video there are actually two solutions we're providing to the customer. So when the customer enters an address, we're validating that address, right, whether it's the right address or not. So that's part of the solution. And then it is returning a score. So actually two solutions kind of embedded into this one API.
Speaker 5:So I think the reason that customers like it is very simple right, if you incorporate this API and you can take it in your entire supply chain, you can embed it anywhere you want, right. You can do it when you're processing your order or however way your supply chain works. You can just take this API, incorporate and then you get this information. And then for our SMBs also, we have a web portal. So you know, sometimes when you ship very few packages, the API really is not the solution and people don't have the technology staff to do these API integrations. In that case, let's say they have 30 addresses that they're going to ship today, they can take and file, upload it to a website and they will get that score. So we have both these solutions available for the customers.
Speaker 1:All right. Well, thank you so much, Panaki Mitra. A great real-world implementation of AI that's generating revenue, that's making customers happy, really providing a vital service in our economy through supply chains. So quick question for Panaki, which is how did this business problem present itself to you?
Speaker 5:It's actually started with a news case, right. So one of our enterprise customers they were having a lot of losses, right. So they came to us. They're trying to identify what happened to this. So we took all this data that we have with the customers and then we looked through where all these claims come from, right, so, there are, you know, like we saw in one slide, right, If you look at the package journey, then claims can happen through the network, like you know, with the carrier's network, Then also after you deliver the package, that's where claims can origin and also from the returns, right.
Speaker 5:So when we're going through this, then when we started looking at this address level because earlier, always, we used to look at from the customer's view, right, we're never looking at from the other side of it, which is the recipient's view and then so we tried, and then we found that there are certain addresses that's where most of the claims are kind of started originating from there, right. So, so, so this, actually this actually, you know, created that need for us. Then we were asked that, okay, can we look at all customers that we have? And then we started moving everything on Google Cloud and because now we have the infrastructure, have the data right. So the work that we have done, we could do it because of, again, the infrastructure, data and then, of course, this business problem we had.
Speaker 1:Great. Thank you, panaki. So we're going to talk a bit about what we want the audience to come away with here is being inspired to implement AI within your organizations and go through not only the technical process of building out a solution, but also building internal stakeholders, budget champions, knowing what that's all about? First, what we're going to do, I'm going to open up to Alok Parikh, the co-founder of Stream, to give a presentation on one of the products you can use to help you get there, one of the products that's used in the UPS Capital pipeline. Alok, I'll switch it over for you.
Speaker 4:Thank you, john. All right, I think I have about 10 minutes and I'm notorious for running late, although making it interesting, though, so I'll try to go quickly. There's a lot of content here. So, first of all, I mean, you know what's new in data is the appropriate topic. There is a lot of new stuff going on. So you know from Pinaki, obviously there's a huge parallel with folks that actually are moving around packets, and we're also moving around and stream a lot of data, and the lifecycle management of events and packets and data and so forth is very dear to us, and one of the things that, when I met the UPS team, was they started talking about how it's not just about delivering the package but also the observability of what's going on in the entire lifecycle, which is again no different than what's going on in the entire lifecycle, which is again no different than what's happening to data today. So, with that at the onset today, I want to talk about a couple of. I'm going to actually show you two inspired use cases after our discussions, not just with UPS Capital, but also with other customers that are sort of trying to make sense of. You know, if there are data pipelines, then how do you actually take advantage of some of the new capabilities in Gen AI, so hopefully you'll get a flavor of that. These are inspired cases. There's a lot more sophistication and complexity behind them, but hopefully it'll get you inspired.
Speaker 4:So the problem statement here is that these claims, so this is not a UPS case. However, it's an inspired case because when we've had some discussions, there was some thought given to what would happen to data that's, for example, not structured. What would happen to image data? Especially when folks are filing claims, oftentimes they may send images of things that they didn't receive or they did receive, that were broken or damaged or tampered with or deliberately tampered with, for example. So these insurance claims are filed against logistic companies for damage in transit. So users can upload these images of damaged parcels along with receipts and invoices.
Speaker 4:And what we want to do in this thing, in the actual example, is identify duplicates. Okay, and the duplicates are going to be identified using particular integrations with GenAI, with large language models, and our goal is to try and detect these duplicate and tampered images in near real time. And that's also an intense. I don't have time to get into that, but me and Pinaki have been talking about you know the urgency of this as well because of the time that you're filing the claim. It is important that if you want to, at that specific time, do similarity searches on images and so forth, that the necessary data representation is available at the claims processing time. So there's some urgency around why you want to do this in real time.
Speaker 4:So our solution today is twofold. So I'm going to talk about two scenarios. One of them just takes an image and we're just going to run the image through some models and we're going to create some vector embeddings and use similarity searches. And the second one is actually slightly different, where invoices are actually uploaded. So in this case now this is just an image, but we're going to actually leverage some Google services to do OCR on it, grab a bunch of unstructured text and then pass that to a language model to actually go ahead and leverage some of the reasoning and the inference and the actual generation capabilities to push that into BigQuery so that we can actually take advantages of other distance approximations there to identify the similarity.
Speaker 4:Okay, so with that let me get into the first one, so just to kind of set it up. Hopefully you can see Pinaki. So I'm going to try and simplify this, there are two flows going on here. In this picture. Flow number one is at the bottom. So this is imagine if you have an operational tier and in the operational tier, along with a lot of your structured data, you could also have image data, file data and so forth. So that's actually being picked up continuously. So the middle box where it says continuous Oracle CDC embedding generator, real-time parallel delivery, that's the stream layer and so that's the actual data pipeline. So the idea is that as images are coming in, we are picking them up in real time. We are pushing them with a component in stream called an embedding generator, which actually talks to Vertex AI in this case and leverages their multi-model embeddings model to create vector embeddings, and then we grab them and then push them into a post-test vector database. It could be a LOADB, post-test vector database, any data store that can really represent vector types. So that is flow number one in the blue dashed box.
Speaker 4:Okay, now let me get to flow number two. So flow number two is at the top, the orange one. So that's how the actual Gen AI application is unfolding, right? So I'm going to. So this one was going from left to right. Let's start reading that from right to left, so right to left, there's a front end, so there's a user that actually goes in and uploads an image into an application that actually does the image analysis service. That actually then, you know whatever when the user uploads the image, generates the, again invokes the embedding model to Vertex AI, gets the vectors and then it actually checks the vector database to do a similarity search. Okay, and that's actually the end-to-end scenario here.
Speaker 4:So there are two pipelines going on. One is forward, going as real-world data is coming in. We are making sure there's a data store which not only has the image but also the embeddings, and the second one is the invocation of the similarity search on top of those embeddings. Okay, let me show that in action now. So I tend to talk ahead of my slides, so I've already explained all this stuff. So let's actually get to the demo. So, john, if you could just go, okay, so we're going to just play this thing and I'll talk through it. So in this case, on the left side is where we're going to drop the actual images and the right part is where the similarity search is going to get executed. So this is what's going on behind the scenes now.
Speaker 4:So in the pipeline forward, going in the blue box that I showed you, this is the stream pipeline, where we are getting the data from the Oracle database, generating the embeddings with one of our components and pushing that into the Postgres database. This is the design of that specific pipeline, okay, and then let me go ahead and deploy this so it validates all your connections. Let's start this thing, and that's when data actually starts flowing through the pipeline. So we're going to go ahead and insert like about 30 claims here, okay, into the table. So originally there's nothing, the table's empty, and I'm going to go ahead and insert a bunch of data into it, okay, commit that workload, and now you can see that I have about, in this case, about 30 odd records there, okay, and then that gets processed in the pipeline. It just takes a second to initialize the first components and now you can see that, okay, 19 have already been processed and it's going to catch up in a couple of seconds. Here, there you go, 30, okay. And so now I've actually processed all of that. Now we're going to go to the big query, to the Postgres side, and take a look at the table there and you can see that all of the embeddings are actually available now in real time for you, okay, of all of the images.
Speaker 4:Now this is the second part of the pipeline, right? This is the actual invocation. So here's a package, so I'm going to submit this and you can see that it's kind of a delivered package that's a little bit not looking so pretty. And now the similarity searches are against the same package. It is the same package, but it's just oriented a different way or doesn't have the tape on it. Okay, so that's a real-world example of how you can actually do the similarity search to identify duplicates based on the same image. So hopefully, that was my first demonstration.
Speaker 4:So this ties together ideas of a lot of us are trying to talk about the rag pattern, for example, which fundamentally is no different than just a truth pattern to me, because once you have the model, share its results, you do want to fine-tune the results. You do want to make sure that if I know something better, then that context gets incorporated with the findings of the model to either better my response or give me the right appropriate response. Response or give me the right appropriate response. Okay. So there's one more thing here now where we are adding a new package which wasn't earlier available in the search, and now I'm going to, in real world, add the 31st image here and once I add that in the data store, that image will be available. So earlier, remember, we saw that there was no image that matched this box. Now I updated one which does match this box and when I submit it you can see that and also notice it's not the same exact image, it is a different orientation, but it can actually tell you that, hey, I think these images are in fact, these boxes are in fact duplicates of each other. Okay, so that's the first one. These boxes are in fact duplicates of each other. Okay, so that's the first one. So let's actually now go to the next guy. So this is what's going on here.
Speaker 4:So there's a on the data ingestion. So in the so remember, there were two pipelines there. So again, in the first pipeline, which is a stream pipeline, there's the data ingestion layer, where we are again loading images from a source database. So you'll see, I'll show you the images to you there are a bunch of JPEG and PNG files which represent receipts in this case. The second part of this is the data processing layer in Stream, where we're extracting the data from these images using Google's Vision API. That's the one that actually does the OCR and converts everything into just free-flowing text. The third part that is getting done in Stream is now we are actually extracting structured information. So this is where the power of the language model, large language model, comes in, where you're actually doing some semantic reasoning, you're actually trying to go ahead and do some inference, because it has to figure out how to lay out the data in this case in JSON format, and also generate the JSON for me, which then ultimately, I will map into a table in BigQuery. So, effectively, what I've done is taken a receipt and taken all the contents there which are printed out and effectively just structured them into BigQuery in a table and that's available now for you to do some other searches and so forth. In the application, which was pipeline number two, right, the user uploads a new receipt image in this case, and now again we push that through the extraction layer so that we can actually get the characters format that again as JSON Now in BigQuery, is where we are actually doing a distance measure, right, and they have functions that support this In this case.
Speaker 4:Get the characters format that again as JSON. Now in BigQuery is where we are actually doing a distance measure, right, and they have functions that support this. In this case we are doing this 11-string distance. The specific parameter is set to less than three, which means you can be off by up to three characters, and then it returns a response to say whether it's the same receipt or not. So ultimately this ties into people trying to do fraud, where maybe they tampered with the receipt or maybe they are submitting duplicate claims with the same receipt and so forth.
Speaker 4:All right, okay, so this video is a little long, but I'm going to try and forward pieces of it so that the core thing is clear. So on the left side again is where the image is going to get dropped. Okay, this is the actual pipeline. So, similar to how I did it before, we're going to deploy and run this pipeline. So this you can see that. You know this pipeline is a little longer. Right Top to bottom, there's a file path reader so that actually reads the file.
Speaker 4:There's a second part which actually converts it. These are the files in storage. They can see that. You know there's a bunch of files. Jpeg. I know the files in storage. You can see that there's a bunch of files, jpeg. I know the people in the back probably can't see it. Can you pause this one for a second, john? Yeah, thank you, we just got this off the internet.
Speaker 4:So, pinaki, there are UPS package receipts that are floating around and for those of you who can't read it, I just want to zoom in and just tell you there's a store, ups store address, there's the weight of the package, there's a tracking number and all that You'll see at the end of this demo. There's a Google BigQuery table with actual fields and I've literally flattened this out and all that information is available. So that's the kind of information that's available from the receipt itself. So that processing I'm showing you on the right, those are just functions within the stream pipeline that we are doing to extract the actual JSON and map that into the BigQuery table. So one of the cool things that we can do is, as the data starts flowing through the stream pipeline, you could actually see now that the data the UPS store number 1948, drop off location 3876, it's already been processed, recognized and already we are getting that data. Next I'm going to convert this to JSON and actually stick this into the BigQuery table. So I think that's the general idea. This is the end result, where I'm showing the actual BigQuery table and you can actually see literally like all of the fields that were available in the receipt. You can actually just run select state in SQL. That is pretty cool. Okay, let's keep going. Okay, so finally getting to the demo. So now this is the actual receipt that we added to say, hey, are there any duplicates that exist? And there you go. So the idea again is it's the same receipt that we pulled up, but it is oriented. It's not the same image, exactly right, but actually it is.
Speaker 4:From a text extraction perspective, we actually did the similarity search using the distance function and it actually pulled up the exact same image, right. So this is an example. So in the first one, we were just purely taking an image, creating embeddings, right, without extracting any text. In the second one, we actually took an image, we extracted the text from it and we actually were able to then leverage both the actual multi-model layer, the Vision API, to actually go ahead and run OCR on that. And, number two, we were able to also teach the model that, hey, I have all this unstructured text. Go ahead and create a JSON document for me and then I mapped that into the BigQuery table. Okay, so you can actually then run your algorithms in the BigQuery site. So that's it. Thank you very much. Those are the two use cases that I want to share with you.
Speaker 1:Thank you, alok. So we've showed you the bits and pieces and we've showed you the enterprise implementation and ways you can go about. You know, doing this at your companies. Your mileage may vary in terms of press coverage. I don't know if you'll get Good Morning America coverage like UPS did. Maybe you'll get Today Show or SportsCenter if you're in that industry. But now we want to talk about some of the ways that we can champion AI solutions internally. I'm going to open it up to the panel and then people can go around and do some Q&A. First question is for Bruno Aziza. People can go around and do some Q&A. First question is for Bruno Aziza. So, bruno, what's your advice to data executives who want to go about building internal AI products and getting the internal alignment and identifying champions, getting budget things along those lines?
Speaker 2:Yeah, so I could tell you what we've learned from the organizations that we work with. It's a complex issue because I think there's a few questions that everybody's asking themselves right now. The first one is what should the size of my budget be? If anyone's figured out exactly the budget, let me know, because every single customer I talk to is a combination of. We are going to just experiment and see how expensive this is going to get to. We are just going to take away from the AI budget and go into Gen AI, and so there's still a lot of best practices there.
Speaker 2:There is great research from McKinsey that looks at the types of approaches that you can take, and they gradually go from half a million dollars to $200 million a year in terms of how you can organize yourself. So I'd say the first thing is probably take a look at that McKinsey research and then figure out what your model should be. Are you in the models? Are you a taker, a shaper or a maker? So a taker is somebody, an organization, that takes from what happens. A shaper is somebody that takes a model and then shapes it, and the maker is someone that will start from scratch, and the maker is the most expensive of all of them. So I think that's the first question is establishing principles around your budget, with low end and high end.
Speaker 2:The second bit that we've learned is the types of employees you need to hire. Who is on the team that's actually going to allow you to be successful? And we've identified five roles that are critical to the success of Gen AI deployments, and the five roles look very much like what you'll see in the software engineering organization. You have first what is called the data product manager. It's a new role that has come out and essentially that's the person that's in charge of the data acquisition to the data activation. So you're looking for somebody that has a profile as a product manager and is kind of the CEO of your data product, if you will, to help that person. We found that the role of the program manager is important because that's the person that's going to take the product requirement document and is going to make sure it's delivered on time. Often, that's where software organizations tend to fail. We have these big ideas and we don't deliver it necessarily on time. Then we've also found that the UX manager is the person that helps the data product manager shape what the solution looks like, and then finally, you've got the data engineer, and if you have one, a chief data officer is the person that is hopefully going to give you the air cover.
Speaker 2:Now, in this last one, what we've learned and I'll stop talking because I know I can go on forever on that one is support is not enough. Like what we've found is if your CDO says they're giving you sponsorship, that's never enough, because sponsorship is basically yeah, I'll let you do it, but if you fail, you're kind of on your own. So you're looking for the mandate from the CEO down to the CDO so you can succeed. Very, very hard to accomplish. But if you don't have a good combination of these three, if you don't have these five roles, you haven't figured out your principle on budget and you don't have the CDO that has the mandate down from the CEO, it's just really, really hard to succeed. That's from an organization standpoint.
Speaker 1:There's a bunch from a tech standpoint but hopefully we'll get to talk about that. Oh yeah, yeah, We'll have plenty of time for that. Thank you, Bruno. This next question is for Sanjay Mohan. So what are the patterns and frameworks that you recommend data practitioners?
Speaker 3:look at to implement AI. So I it's all about data, it's not. There's no AI stack. You don't want to move around petabytes of data and make copies of data just to run this AI and that AI, because then you'll just create more silos. But I think there's a more fundamental problem to so. Bruno, you did an amazing job explaining. You know what it is, but I think there's there's a but coming. But. But there's a step before that and the step before that.
Speaker 2:We famously disagree on panels. That's why that's what's happening here.
Speaker 3:So the step? Yes, by the way, we did it last year and it was absolutely amazing. For one hour we were literally just going at each other. But I think the organizations have a very simple question to answer what is it that you want to do with AI? So, before you go with budget, before you go with roles, so there are only two questions what do I do with AI and how not to F it up? And if you have to read the McKinsey report, you shouldn't be doing it. There are only two questions what do I do with AI and how not to F it up? Then, and if you have to read the McKinsey report.
Speaker 4:you shouldn't be doing it. That's my opinion, yeah.
Speaker 2:You should go to Sanjumal, yes, okay, so it's difficult to disagree with that guidance, because it's true that you know there's 168 use cases inside your company, and so, before doing anything, you probably need to figure out which are the use cases you're going to get support on, particularly assuming that you're going to mess up a good part of it on the way there.
Speaker 3:So the beauty of AI is that we're all learning. Business is super excited. For the first time in our career, in our entire history, businesses on board more than IT is. It is actually holding back and going oh wait, slow down. And business is like where is my chatbot? How come I don't have agents? And this is the first time we are seeing.
Speaker 3:From my own personal experience, being a veteran of going to tons of industry conferences, I would come back to my organization and say, hey, guess what? This is a new graph database. People would be like really Get out, we don't want to talk to you. Now the business is coming back and saying this is what we can do. New use cases, we can save money. So IT get on board.
Speaker 3:So, talking about patents, what I recommend is because we are learning, so this is a place where business and IT should have hackathons and identify what are those use cases. Some use cases you will get funded and some cases you won't get use cases. But those use cases are unknown, Unless you sit together for a week and you say here are all the potential use cases, let's prioritize them and let's get started on these use cases and get funding for those. And then, because at the end of the day, you have to show ROI. If there is no ROI and your LLM is hallucinating like crazy, then that project will get shut down. So your data has to be correct, your processes have to be correct, your organization, the rules have to be in the right place. So you have to take a very structured business and IT, business-driven approach, not an IT approach.
Speaker 4:I guess maybe Sanjeev, I'll ask one question which bridges the gap maybe between the two. I mean, it seems like there are certain things that are rather obvious, right? For example, none of us want to read a 300-page document just to answer somebody's email, and people love sending attachments. That's just like a painful problem, especially if the corpus is super large. So I think you know, in your daily applications, anytime there's collaboration, anytime there's, you know, a sort of a network and you're moving around large documents trying to actually leverage, you know, particularly generative AI. I think that seems to be like an almost straightforward application which almost every business could benefit from. But think that seems to be like an almost straightforward application which almost every business could benefit from. But so that's the part about hey, the use case is kind of known.
Speaker 4:The flip side of that is the trust part that you're like we tried to do this. You know, the first thing, you know my own team was. I was like, hey, can we just? You know, because in stream you have to design a pipeline, and you know everybody's like, oh, can I just write the pipeline, which is a SQL-based pipeline using Gen AI? And they're like, okay, let's do that, let's train it on our documentation particularly, and as we started going to it, people are like well, what about our knowledge bases?
Speaker 4:Now, all of a sudden, we started seeing different areas of information Some were sanitized, some were not sanitized, some had access controls, other didn't have access controls. So I think that's where the piece. I think around quality and governance and trust. So I do think that that conversation is super critical between business and IT. But I think, if you come at it with these larger bucket categories, that, hey look, obvious use cases that nobody can disagree with are these. But the same data management things that we've applied to deliver business intelligence also need to be applied to deliver generative AI, and I think that would be a good discussion to have, I think between these teams.
Speaker 5:Anything Pinaki, yeah I think, from my perspective going through this journey, one thing I like in Bruno's point just the sponsorship is not enough. You really need to have strong support from the top. And so you know, one of the success that we have with this address confidence score, because our president strongly believed in it. It's not like, okay, here is the funding, here is the staffing, then you go with it and if you fail it's your problem. It's not like that. So he really stood behind it.
Speaker 5:And in a large organization like us, there are a lot of difficulties. Right, because you know all this data, because, as I said, if you don't have data, you have nothing. Right, but all this data, you know there are data owners, there are application owners. You have to really convince everybody that why you need this data, and so that's a huge part, right? So if the people I mean especially at very top just a sponsor, it's not enough. It really needs to, you know, take down all these silos, all these barriers so that you can get there. Right?
Speaker 5:And to your point, sanjeev, so what I have seen, that you know now, like you said, right. And to your point, sanjeev, so what I have seen, that you know now, like you said right, the business is coming up with all these things. Right, I want this, I read about this, you know. But I think the prioritization is a big piece. Right, because I have so many business cases to work on. But if we don't really focus on the top one and try to deliver something that matters to the customer, we will be just all over the place. It becomes like a bunch of science projects.
Speaker 1:Excellent, and it seems like now that we're talking about generative AI here at Google Cloud Next and various conferences in the industry, we're certainly talking less about things like data fabric or data mesh or high-level data ontologies in general and thinking about the business use cases and Pinaki, what you've implemented at UPS is very powerful and it's something that a lot of people can relate to. I'll ask another question, which is to Pinaki what were some of the things you learned along the journey of building out generative AI within UPS?
Speaker 5:I think I just covered, right, this is kind of my learning Again. I think that support is a big thing, and so, before even that, right one thing, I realized that you, we have access to the data, but the data just need to be in one place, which is so. I know we talk about this all the time, but it's a reality, right, I think a significant amount of time just need to be spent so that we build a robust data pipeline, because if we don't have the good data, there is really no ML, no AI, right? So, and then we really need to have this good infrastructure. So, for us, going into Google Cloud and really leveraging this Google Managed Services, it's a game changer for us. So it's the you know, it's just this rich data behind UPS, and then this infrastructure, and also and another one is like, what we talked about is the executive support, and then also, you need to have the right people in place, right?
Speaker 3:So, yes, Do you have quantifiable? Is there a dollar amount that UPS has saved?
Speaker 5:So this one, you know it's a revenue generating product, right? So one thing we realized that even if you have these overnight, the dollars will not flow, because you know the customers need to understand what it is right. We know that we're trying to solve a customer problem, but there are also on the customer side, there are IT integration. So even if you build an API, the customer needs to be able to integrate into their system. So all these, to answer your question is there a quantifiable dollar number? It's not there. Yet we know we're solving a customer problem and then, if we really solve a customer problem, the revenue will come.
Speaker 6:And.
Speaker 1:Bruno question. I want to ask you, since you operate at a macro level from Capital G and your work at Google Cloud, working with so many companies, what are some of the other amazing implementations of generative AI that you've seen in the enterprise?
Speaker 2:Yeah, there's a lot going on, and I'd say, as what we're learning from what customers are doing, what we're learning is that every single one of us here in this room is going to be stuck between FOMO and FOMO. Have you heard of those two acronyms, fomo and FOMO? I might have come up with the FOMO one. I'll tell you what it is. Fomo is all of us here we're looking at these stories like I want this, a fear of missing out if I don't do it right, and FOMO is something kind of what you said earlier is the fear of messing it up, you know, and so the reality is somewhere between those. I'll give you three examples of companies that we've seen have kind of learned through this. The first one is professional services organization kind of relating to what you talked about earlier, this idea of lots and lots of legal documents. They kind of all contain cases and so forth, and what they realized is that summarization is one of the primary use case where you know you're going to hit 80% of what you need, and so not only were they able to get to the truth faster across these documents, but they also were able to help their junior staff get to more productive work because prior to that, they were doing a bunch of work of just reading the documents, summarizing it for somebody else, just taking action. So the idea here was that Gen AI for them was a performance leveler for the staff of facilitating the execution of tasks that are fairly basic because they were focused on summarize. But the learning there is summarize versus verify right. So you got to be careful. It's not like you can take, as we've seen, because of the hallucination. It's a solvable problem, but there are still margins of errors there. That's first example.
Speaker 2:The second example is an interesting one because it's an organization that actually first failed and then they learned from that failure. So this is an online retailer and they thought they'd create an external bot to their consumers. To just start now saying you come in and you want to create this particular dish and us as the company, we will go into our inventory and then create a recipe for it Sounds pretty basic. The issue is that they realize there's language barriers and inventory barriers. When that happens, say that I come in and I'm French and I'm typing it in French. It can translate it and maybe the translation isn't exactly accurate and it hits the inventory. Well, you don't have it as part of your inventory. So now the recipe is incomplete.
Speaker 2:So there's a lot of things where they thought, oh, we thought we had the data, it's fairly controllable and we want to create this compelling customer experience. But they discovered, or discovered, they were reminded of, that you can't do Gen AI unless you have really really good data governance, unless you have really really good data. So it's kind of like what's interesting in Gen AI is that it's kind of I don't know who's married here. I got married 25 years ago and at the time when I got married it was there's something old and something new. The something new stuff is everything we hear. That's creating the FOMO, but the something old is the rules of this business are not changing. If you have bad data, you can build a application that gives you results the wrong results really fast, and nobody wants that as an experience, and so what they did is then they turned the LLM towards internal data management problems to start now cleaning up the data that they thought was bad. And I thought that's kind of interesting, because what we're learning is maybe the best use case is actually an internal use case first, before you start going out to your external consumers, because there's a high risk there that you're actually going to get a lot of bad results potentially.
Speaker 2:And then the third example, and I promise I'll stop there. As you can see, I'm very passionate about this space. So what we've learned is that the mindset of people when they interface with LLMs or chatbots is that it's changed right. In the past, you used to go to a search engine and you get result sets, and you knew that the result sets were a component that you needed for you to make a decision as a human. Now, with chatbots and the technology the way it's advancing, it's almost like we're transferring the liability to the chatbot to actually give you the perfect answer. And I think there's a bridge that we have to get to. Where is it reasonable to expect that you're just going to get the answer right? And so, again, if you go back to the principle of like do you have strong data? Do you have governance? Maybe your first use case is going to be between the FOMO and the FOMO, right, it's like, just make sure you don't mess it up, because it might not be recoverable if you do it on bad data.
Speaker 3:Can I add something to this? You have a third acronym no, no, no, no, no acronym. Although I'm from Gartner, we are known ex-Gartner. We are known for creating acronyms, but I'm going to stop that habit. So you know to your point how maybe our first use case should be internal. So I want to tell you about this real life use case from a very major pharma company known for its COVID vaccine.
Speaker 3:The CEO has given all the business heads a directive to come up with some generative AI use cases, and it's fascinating what the CEO wants from his business heads. What he's told them is that over the last few decades, we spent millions of dollars and we've accumulated all of these documents New England Journal of Medicine, cdc documents, who, you name it. There are millions of these documents. So they want to ingest all this unstructured data. They don't want to summarize it, because summarization is great, but so what? They want their business heads to find out what is hidden in those millions of documents, ie cures for diseases.
Speaker 3:How do I reduce the time to do FDA approval from two years to six months? Maybe there's a cure for cancer that you know. Over like decades and decades, we found some commonality that humans have missed it Now maybe we automate it, we bring all this data and do similarity search. That is a goal that they're aiming for and if they summarize and they do like customer success and they manage to reduce the number of tickets, amazing there's money in there. But there's a bigger goal of what generative AI can do.
Speaker 2:Yeah, I think what we're learning is a lot of you know the. There is this idea of like, oh, all the things that I can do and create the brand new content, and I, I think verify, I think is what I would say right, and even in in another obvious, uh, use case, is code generation or code completion. You have to think that it's not like if you don't know how to code and you get, you know, you know a bunch of code, how are you going to know that it actually works? So you do have to embed this idea that, as a human being interfacing with these tools, you want to have the ability to be qualified to verify the information.
Speaker 4:And I think just to add I think that is one of the interesting research areas is to use Gen AI to precisely solve that specific problem, which is to improve the quality itself, which hopefully will take us to true intelligence at some point. I guess that's an active area where I think cross-checks and guards and safeguards, leveraging these models to at least tell me I think there was an example this morning, also in the keynote, where I think I found an interesting example was am I actually violating a certain thing based on this rule book? And I think that's a great example where it's not summarization, but at least if I'm overtly violating something, at least it can highlight it so I can go take a second look. And these are like what I would call just. I mean, they give tangible benefits and they're also very low hanging. You can actually implement it Internally and, bruno, to your point, internally, the first use case that we did was for us, right?
Speaker 4:We said, okay, well, we'll have our own strategy, no-transcript, informal settings and so forth. So that's the direction that we are moving in there as well. So just classic, great examples.
Speaker 2:The reason for why I'm suggesting internal use case is because if you look at the companies that are not succeeding with Gen-A and they're not in production, there's two patterns that quickly appear.
Speaker 2:One of them is around data governance and the legal use of the data and who's supposed to see it and who's supposed not to see it, which we've known forever for data in general. So it's not a new problem, but it is the core to this particular new ARF technology. And then the second one is when you're in the content creation business. Now you have to worry about attribution, and if you can't trace back attribution now, we open a whole can of ones here. So that's why when you're dealing with your own data, you're close to your enterprise truth. That's the data you own, and then you can go clean that up before you start just rushing to an external one without having those guardrails. I think that's probably that's what I would do is I would take a hackathon with the business team, identify the 168 use cases they're gonna throw at you, or 16,000 and a hundred they're gonna throw at you, and then determine which is the one internal one that is going to be a needle mover across all the other ones and attack that one first.
Speaker 3:So governance moves up front, and when we did data governance, it was an afterthought. We don't want to make the same mistake. So you do the hackathon, you find out what are these 160 use cases, but then 68. 168. Oh, thank you. But then starts a long process. Okay, so these are the use cases. What models do I use Today? We heard Gemini Pro 1.5, a million process. Okay, so these are the use cases. What models do I use Today? We heard Gemini Pro 1.5, a million tokens. Is that the right model? Or should I be using something from Hugging Face that is open source, like Lama 2? What model do I use? And then so a model evaluation mapping it to the use case, testing it out, seeing how much it costs. It's all governance. That AI governance needs to be baked from get-go, and that's a lesson we learned in data management. We didn't do it right and now we're trying to fix it. I don't know if you do that, yeah.
Speaker 5:But before getting to that, so I was thinking about your question on ROI. But before getting to that, so I was thinking about your question on ROI. So one thing we have found through this address confidence score that in general population, for customers, they can save up to 40% of their losses. So that's a you know, it's a huge testament from the customer. So now going back to your question about these governance. So UPS has a very strong AI governance right. Anytime there is a new project comes on, before you jump onto using this model, you have to fill out a questionnaire. It's really not the bureaucracy, but just because it is so new, we need to make sure it is really safeguarded, right. So whatever model you want to use, you just have to put the business case in place and sort of document right what you're really doing with it. So it kind of doesn't go out of hand.
Speaker 1:And that's a great example of you know having the safeguards in place. But look at the innovation that you've accomplished and look at the recognition you've received in the public. You know both. You know Good Morning America and CNBC talking about what you built, but you still have those governance and those safety measures in place around it, so it's not like having these measures actually stifles innovation in this context. So I think this is a bull case for data teams who are sophisticated and already understand the principles of processing and managing enterprise data, taking on the AI innovation initiatives as well within these companies Excellent. Well, thank you so much to the panel for joining us today. Thank you to the audience that was here live. Really appreciate you all being here. Hope you all enjoy the rest of Google Cloud Next and thank you to those of you who are tuning in to this episode of what's New in Data that's recorded. Thank you. Thank you, john.