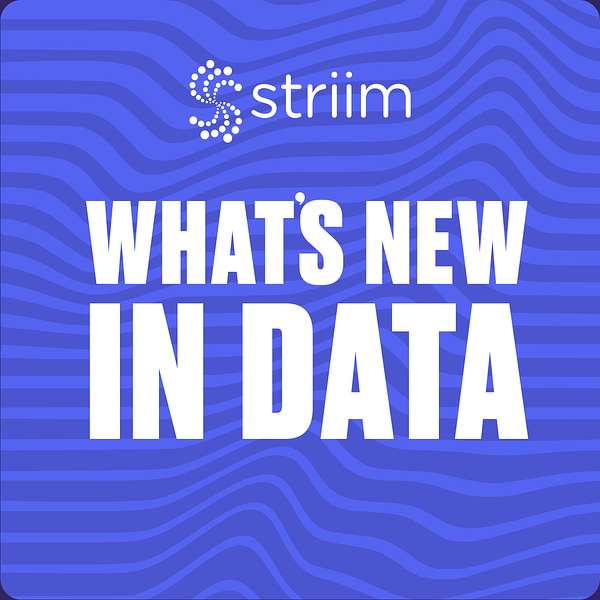
What's New In Data
A podcast by Striim (pronounced 'Stream') that covers the latest trends and news in data, cloud computing, data streaming, and analytics.
What's New In Data
Live from Snowflake Summit: Transforming Data Management Insights with Sanjeev Mohan
What's New in Data's Live Recording from the Salesforce Tower during Snowflake Summit
Imagine a world where real-time data processing is the norm, not the exception. In this episode, we bring you a fascinating conversation with Sanjeev Mohan, former VP at Gartner, who unpacks the seismic shifts in the data processing landscape. You'll learn about the convergence of structured and unstructured data, driven by Generative AI, and why streaming is becoming the default method for data processing. Sanjeev highlights the significance of innovations like Iceberg, which create a common table format essential for decision-making across a variety of applications.
We then traverse the cutting-edge realm of real-time data streaming platforms, spotlighting technologies and companies such as Materialize and Apache Grid Gain. Sanjeev explains the essential design criteria for these platforms, including scalability, cost performance, and fault tolerance. He also discusses the pivotal role of Kafka and its implementations across major cloud providers. This episode is a treasure trove of insights into how platforms like Snowflake are being utilized beyond their traditional roles to act as streaming databases, redefining the boundaries of data management.
In our final segments, we accelerate into the future, examining the rapid advancements in streaming technology and its interplay with AI. Sanjeev reflects on how applications like Tesla and Uber are driving innovation and demonstrates the complexities of handling real-time data replication with tools like Snowpipe Streaming. We also explore the potential for real-time training of Large Language Models (LLMs) and the ever-evolving landscape of data management. Packed with expert analysis and future-forward thinking, this episode is your guide to understanding the groundbreaking technologies shaping the world of data.
What's New In Data is a data thought leadership series hosted by John Kutay who leads data and products at Striim. What's New In Data hosts industry practitioners to discuss latest trends, common patterns for real world data patterns, and analytics success stories.
Okay, we are getting started with our event here. Our San Francisco edition of What's New in Data at Snowflake Summit. What's New in Data is a thought leadership series we've been doing a long time. We've had some great enterprises including UPS, CIBC, folks like the head of enterprise data at Google have joined us. And we really just like telling the stories of great thought leaders and what they're thinking. And today we have, to my left, we have Sanjeev Industry analyst, uh, ex VP of, uh, who covered Gart, uh, data and analytics at Gartner. Without further ado, I'm going to hand it over to Sanjeev to kick it off. All right. Thank you everyone for coming to this evening. Uh, amazing views. We were here last year at the same venue and we did a fireside chat. We had this guy, uh, a good friend of ours from Google, Bruno Aziza, and he and I were literally just chatting. Fighting with each other on stage. But what I'd like to do is I'd like to make my sessions very interactive. You know, it's a small group and it's evening. We've all had some drinks. I had some frozen margaritas before I walked over. So let's make it a fun evening. So if you have any questions, are you have any, uh, like, you know, your air your thoughts because I tend to be an insufferable optimist. Sometimes my wife just says insufferable, but I you know, that's where I see myself. So So let me get started. So I'm talking about, uh, since I'm an analyst, so I talk about the market landscape and I cover, uh, data and analytics from a very, very deep technology space. And then I try to bubble it up into what makes sense. And, um, I see there are three major, uh, major pillars in the data space that are really important these days. One is a convergence of structured and unstructured data, obviously driven by Gen AI. Because so far we've only spent all our time doing structured, now we have to think about unstructured data as well. The second piece is a convergence of batch and streaming. Because it used to be when I first started looking at streaming, it was like, how many of you remember Lambda architecture? So a few of you remember where you had a separate stack for batch, a separate stack for for streaming. That is just just crazy. So that's a second major pillar where we are focused on. And the third one is on iceberg or a common table format. So those are the three areas that are very, very important in my coverage these days. So I'm gonna get started. And, uh, just a brief introduction. I was in Gartner five years as part of the data and analytics team there. And after five years, I decided it's time to branch out and do something on my own. So I started a company with a very strange name. It's called Sanjmo. And, uh, and I had no idea what I was doing. I was just like, let's give it a shot. And it's been literally nonstop. It'll be three years next month. So So I and I wrote a book called data product for dummies. Actually, I'm doing book signing tomorrow at 4 30 PM. If you are around in the expo hall, I'll be signing the book. I also I'm a very prolific blogger on medium. If you get a chance, please follow it. It's Sanchmo dot medium. And I'm also a host of my own podcast. It's called. It depends. So, so with that, there's some links here. So let me let me get started with. So I spent a lot of time trying to figure out, like, connect the dots. In fact, my website is trying to connect the dots. So I had this epiphany that what is how do we traditionally do data processing? So I put it together and very simple. We take the current data, we ETL it, nightly batch jobs or micro batch. We make it past data and then we predict the future. That's just crazy. What we're actually doing is that we're literally predicting the present. When we apply a model that was trained in the past, you can predict the present. You already know what's happening. So this is the reason why we need to rethink and think about how do we, you know, how do we act upon live current fresh data rather than, you know, so it used to be, why did we go to batch? That's because we were limited by the data processing systems of that time, like 20, 30 years ago, it was too expensive to do streaming data. Today, streaming data is becoming the default batch is actually becoming a type of streaming. rather than the other way around. So our entire mentality is shifting towards streaming being the default. So, but the question begs, why do we need, uh, streaming? So I'm going to talk about, uh, actually two major topics, the market landscape, and then we will dive into, into how stream is enabling real time snowflake. So why do we need stream processing? We want to be able to do patent recognition in real time. Uh, fraud, anomaly detection is probably the number one use case. Whenever I talk to, to a customer, I say, why are you doing real time streaming? It's almost always for, for fraud detection, like credit card transactions. Obviously, you cannot do it once a transaction has started. So we need to make sure that that's committed. So the second thing is, uh, sometimes we want to enrich the data in real time to do preventive maintenance. For example, I have Iot devices. I'm getting some information or, uh, or it's a factory on the floor, but I need to look up some manuals and enrich the data that I'm, uh, that, that I'm collecting. and then Uh, also to aggregate data in real time. So I can optimize spend, like, for example, ad revenues, like how much ads are coming in. How should I start to, uh, to leverage that data? So filter data to detect trends again, really important. In fact, um, Uh, I see even LLMs in future will be trained in real time because LLMs are really good at detecting trends, except the data is very old in LLM because they're trained very rarely. So windowing is a very important, uh, piece, uh, like tumbling window, sliding window. So I'm trying to calculate what is a moving average. For example, if my, The time it is taking for me to take a call is going up steadily. That means something is wrong. So every 10 minutes, I'm sampling it. Uh, or I have a 10 minute window and I'm sampling it every 30 seconds. So I'm doing a moving, uh, average, uh, also for trading data to see if the stock prices are going up and down. And then load balance, uh, and paralyzed distributed processing. If I know in real time how my, my, my data is coming in, then I can intelligently load balance it across different servers. So, so those are some of the reasons. And then, uh, finally, uh, Uh, if I want to do stateful processing off historical data, uh, along with my real time data. So for that, we need it. Okay, so one side of the coin is processing. The other side of the coin is analytics. So, so think of it this way. What I just shared is what we like people like me on technology side like to think about. Why do I need real time processing? But then on the business side, this is what business people want. They want to do user facing analytics to improve customer experience. If I'm on a website and, uh, you know, and I'm browsing, I need instant, uh, user, uh, user experience, you know? So, uh, also business people want to make operational decisions. And the only way you can make operational decisions is if you have live data, otherwise it's too late for you to, to, uh, make these decisions. Reduce anomalies like fraud, sensor defect. recommendations leading to high yields. If I'm on a website and I'm browsing, I go to one page and I go to the second page. I want that, that, that website traffic data to recommend what should I look at in real time? Otherwise it's too late. Also, if I have a real time data, then I can allocate my resources efficiently, uh, in real time and, um, optimize supply chain, um, in real time. And then finally, regulatory compliance. So this is also becoming a very important topic. And we've heard quite a bit today about some of the new snowflake. I don't even know how much of stuff I can talk about that's even been announced. But compliance came up all day today. So, so, um, you know, so if you heard it, it's probably, you know, out by now. Trust center is, I don't know, is that, Yeah. Okay. So trust center has like compliance is a very important pillar of some security and privacy pieces. So that gets much better if you have live data, otherwise, uh, it's just too late. Okay. So let's go to what are the key, like what, what does a business want? So the first thing I put here is, Cost performance is really important. This is why streaming has taken so long to get established because the cost of doing real time streaming stream processing and analytics was way too high. So businesses tell us it has has to be cost effective. It must be easy, integrated. In fact, I was just talking to somebody today and I was like, You should be happy with iceberg. You can do multiple engines now, like businesses have so much choice. And actually, he's here. I even mentioned it on the cube today. And he's Yeah, I see you. And he says, Yeah, but they're too many choices now. So so that you know, complexity. In fact, the V. I. Snowflake Summit Snowflakes, uh, two big reasons why people use Snowflake are It's unified and it's simple. So, what is simple, gets adopted. What is not simple, I'll give you a very simple example. When World Wide Web came out, the markup language it used, HTML, was something that just took on, like wildfire. But markup languages, I was using Unix. We had markup languages for years. But it was so difficult to use. So the moment HTML came out, like we were like, Oh, my God, everybody jumped onto one right back. So So this is what businesses tell us. And then finally, that data has to be high quality. It has to be governed. It has to be secure. All the privacy regulations must be maintained. So this These are the three most important things that customers are saying they want. So once I analyze this, then my mind started thinking about how do I create a sort of a taxonomy of how do you do real time analytics? So there are multiple options. I'm going to talk about four different options. So the first option, how do people do real time analytics? The easiest is BI tool. Power BI has Direct Connect. You can connect Power BI to your data source and you can do it, uh, do real time analytics. But most of the time, how do you use Click or Tableau? You actually download the data onto your desktop, right? I mean, how many of you do real time analytics and Power bi? Anyone here? Okay. So I don't see any hands at all. So this is, so I put this here, but this is actually the poor man's way of doing it. You can do real time analytics a hundred percent, but then it has to be on small data. If you are going to consume a lot of data, then you will saturate the capabilities of your BI tool, the network. And so most people will actually download the data onto their desktop. Uh, and that, that's where they do their, uh, analysis. So although, so in this case, what happens is ABI stands for analytics and BI platform. And so I've got streaming data that comes in and then I've got my dashboards. I can just directly, uh, look at streaming data. Most of the time, stream data gets stored in a, into a database and then it, uh, it gets analyzed. So, so this is not really a valid option, but it is definitely a, one way to get started. Okay. Let's look at option number two. This is probably by far the most common way of doing it, which is event stream processing. In event stream processing, you are basically the bringing all the processing of streams into a platform. This platform, uh, is, uh, a lot of times the most popular platform, uh, is called anyone, anyone. What's the most popular event stream processing? Not Kafka. What is the most common event stream processing platform? The de facto standard is Flink. So, uh, so Kafka, and we'll talk about Kafka, Kafka, the message bus. So Kafka sits here. So, so Kafka connect, uh, connects to the sources and it loads it into Flink. In Flink, I can do all the things I showed, like why, like aggregation, filtering, processing. Uh, enriching the data. All that I do in an event stream processing. And by the way, there are a lot of stream processors. So, uh, one of them is actually, uh, stream stream is a change data capture. But behind the scenes it has it has its own stream processor. So, uh, so that's that's what it is. And then you use your either your, uh, Uh, bi tool. So basically instead of doing your your streaming in the bi tool, you now have a separate, uh, tool to do it. So very common. In fact, one way to think of it is that event stream processing. You know how we separated compute and store in the in the cloud for databases. This is a compute. So then the question goes, What is the storage? Well, I'm glad you asked. Because that's option number three. So option number three is where you are taking the streaming data into a database. And then, uh, and this is very hard to do because databases are sometimes built for because the persistence. So how do you get very low latency access? To data. But this is is one of the hottest topics right now. In fact, this is what we are talking about today. How do you make snowflake into a streaming database? So so, uh, snowflake. And how many of you think snowflake is a streaming database? Okay. One hand, but it's rigged. You guys are not. So, so we always think of Snowflake as an analytical database. In fact, in a few minutes, I'm going to break this into two parts, OLTP and OLAP. So, so hold that thought for now, because those are the two databases, but most of the time, Uh, the database is not considered to be fit for streaming, but there are a lot of examples. There is a I'll give you some. There's one company called materialize. Anyone heard of materialize? So that is a streaming database. There are a lot of small companies in this space. There are a lot of examples, but I'm gonna hold that thought for a few minutes. Okay, so this is option number three. And then there is a new option that And I've invented, it's called Unified Real time Platform, which is actually a combination of, uh, of streams. Uh, so it's got, uh, the database, it's got stream processing, and it's got applications. So URP is not that well known, but there are a number of companies in this space. Uh, there's one, I think Ali, you, you connected with, uh, Hazelcast, right? You're on the boat. So Hazelcast actually falls in a URP, a Unified Real Time Platform, because in Hazelcast you can build your applications also on top. So you're not only just streaming the data in, you're processing it like an ESP, and you're building an application. There's also, there's something called Apache Grid Gain, so that also falls under URP. If you want to learn more about URP, This is sort of groundbreaking research. Uh, if you go to my medium, sanjmo. medium, you might have noticed I'm pitching. Um, so, uh, I have a couple of documents on this, uh, on this topic. Okay. So, so with this, um, I want to summarize it. So here I'm going, I'm doing something different. So So first of all, uh, message bus. So all this data that's coming into either the database or, or event stream processing or into a BI tool, it's coming through some protocol and that protocol a lot of time happens to be Kafka. So this is where Kafka sits. Kafka is a message bus. It's a transport layer. And Kafka is actually a commodity. In fact, there's Kafka and then there's Kafka like. Kafka like. Why do I say Kafka Lite? Because there are probably 20, 30 different Kafka types. There's one called Red Panda. Has anyone heard of Red Panda? Okay, so a few of you. There are, uh, Amazon managed, uh, managed service for Kafka, MSK. That's, that's Kafka. So there are open source versions. There are, uh, uh, commercial versions. So there are a lot of different, so there are about 20, 30 of these. But it's not just message bus, uh, event broker, change data capture. So the second half of our talk, we'll talk more about change data capture. What is change data capture? But for now, it just says CDC here, WebSockets, Webhooks. But then there are other products. Every hyperscaler has their own product. Azure has Event Hubs, Amazon has Kinesis, and then Google has PubSub. So these are the transport layer. But when the data comes into that, then, uh, here I've got these four options. One, what I did here is I broke up the stream enabled database into two types. So why did I do it? Because remember I said databases usually come in OLTP and OLAP? So OLTP, streaming database, I gave you an example that's materialized, for example. But on the analytical side, there are lots and lots of choices that you may have heard of. Clickhouse? Has anyone heard of Clickhouse? Yep. Yeah, Clickhouse is very popular. That's, you know, a rock set. Uh, Droid, Pino, StarTree, Imply. So there are all these databases. So this is a very popular category of, uh, and this is where Snowflake would fall because Snowflake is an analytical database. So now I'm going to finish with one more slide, and then we're going to go to the second part. So there's a lot of stuff here. But what are some of the most essential design criteria that I need for a streaming real time streaming platform? First of all, it has to scale because the problem with with real time streaming data is that it's unbounded. Unbounded means unlike a batch which has a beginning and there is no beginning and the data is just coming. These are like the web logs that are coming, IoT sensor data is coming. So scale becomes extremely important. And then of course, how cost performance includes throughput and latency. How quickly can you process that data? A lot of event stream processing Products are in memory because that's how you get speed. So, uh, so there's a lot of, uh, performance and then it must be fault tolerant because, uh, because you are scaling and you've got a cluster with. In fact, some companies like Walmart, for instance, has 1400 clusters of Kafka. Every department has, you know, its own cluster. So fault tolerant, having an ecosystem, this includes things like connectors because I'm going to get data from different sources. So I need all kinds of connectors. We talked about governance and then where is it deployed? Is it deployed on premises? Is it deployed in the cloud? If it's in the cloud, is it one cloud? Is it hybrid cloud? Is it in my VPC? Is it in vendor VPC, multi tenant? So, so these are some technical considerations. So anyway, um, so just a quick, uh, conclusion, um, first of all, why do all these options exist is because, um, Different use cases call for different requirements, so it's not like I cannot have like bi is old. I should do event stream processing. I should do streaming database. A lot of people I talked to like large customers like like DTC DTCC probably has all the four options, at least three of them. So because different use cases will require different different choices. URP is new because I'm trying to to connect the event stream processing, real time database and application development. Also, I'm trying to to handle both async and sync process and also data in motion and data addressed. So that is where URP is a new thing. Uh, Jenny, I, uh, is, uh, is raising the profile of streaming data because we are trying to get to a point where, uh, when you ask a question to a chat bot, your question needs to be embedded. Uh, sorry. It needs to have a vector embedding created. That vector embedding will be created in real time. So then you can send it to an LLM. So, so this is one of the reasons why streaming is now so hard. Uh, and, uh, even text to video. Like if you ask a question to perplexity or to some other model, then your answer must be generated right away. Have you noticed? Like if you ask chat GPT to summarize a long document, it starts showing you the results without completing it because it's streaming. It's, you know, because if you wait for two minutes, it's just too long. So, and then stream and snowflake, uh, I have now collaborated because they can provide under one minute latency for, for data that has all the governance around it. And so that is a topic for the next, uh, remember I mentioned about change data capture in my, uh, input to all, all these, uh, options. So what is chain data capture? Uh, this is this is the bridge between OLTP transaction and analytical databases. What we are trying to do is, uh, operational databases are constantly being updated. So if so, obviously, I want to run my reports on the latest data, but if I run it on my operational database, I will slow it down that that as a result, I won't be able to take any new orders. So I cannot overburden my operational database by doing analytics on it. So then what is my option? My option is to do chain data capture. What that, what this is showing is, this is my operational database. Inserts, updates, deletes are happening here. And as the data is written into the database and the transaction is committed, I'm going to take that data from a log that the database maintains, and I'm going to write it to my, uh, my data warehouse. And, uh, there are different types of logs. If you use Oracle, then you have redo logs. If you use, uh, MySQL, it's bin Log. Uh, Postgres has something called write ahead log. MongoDB has called something called MongoDB Streams. So, so these databases, operation databases have a, have a, uh, a way for, for target to, to accept this data. Okay, so here is an example using Stream. So stream, uh, is, uh, one of the, uh, one of the long established choices here. So you can see, I've got data coming into Oracle, MongoDB, Salesforce, which is CRM, Stripe, where my payments are coming in. So I've got all kinds of data that's coming in. Uh, it's very common these days. For even a small organization to have more than a hundred SAS tools. And if you're a large organization, it's easily four or five hundred. So you got all these, all this data coming in. Now you need the ability to capture it in real time, or near real time, and then write it into Snowflake. So for that, we are using something called Snowpipe Streaming. So when I first started looking into, uh, into Snowflake, it used to be that Snowflake, just a year or two ago, would, uh, would let Stream write the data onto S3, or, or GCS, or Azure Blob, and then Snowpipe would actually read that data from S3 and write it into Snowflake. Today is different. Today, the data comes on Kafka and, and, uh, Snowflake can read it directly. Uh, you know, so, so now it has this ability to read the data directly as it is being generated. In fact, it's actually very interesting. You see here schema metadata lineage. Uh, I may I may not even have a table. I may have a new data that is being, uh, streamed, uh, through chain data capture. I haven't even created a table and I can set up snowflake so it can look at the schema off the data and boom, create a table for me on the fly. So it's super interesting how far snowflake has come. In, in, uh, in handling this, then, uh, what stream, uh, stream gives you this whole, uh, sort of a, uh, governed platform? Because schema change. What if somebody deleted, uh, a column or renamed the column? What do you do then? So, so you need this ability to, uh, to, uh, to handle schema changes. In fact, one of the very interesting thing is, if somebody updated, let's say, you know, my salary, I got a bump and my salary got, went up. So great. You, the update happens in the source. I can, uh, send that chain data capture into my target and I can update my salary. But what happens if something is deleted? Because now that that causes a lot of issues. If you delete it in the target, it can cause, uh, you know, acid, uh, issues. And if there's dependencies, you know, so, so, so behind the scenes, what stream is doing is actually very complicated. It just looks like, you know, it's quite simple. You're just connecting source and target. Okay. So, here is an example of, uh, something that, uh, that is called dynamic tables. So you've already seen, you've got source, stream does, uh, chain data capture, and then it comes to Snowflake, which has this concept of dynamic tables, which is also something very new. In a dynamic table, it is actually like it's it's like a materialized view. It's actually kind of refreshing because the data is coming in constantly. So it's keeping track of data as it's coming in and and then and then you can build your apps on it. Now we've got the expert here. then. So if you have any question, I'm going to direct it to him. We also have Pura, who's an engineering manager. He's also here. I'm just putting him on spot. So, okay, so, um, so, uh, this is basically, uh, an example of how, uh, same thing. You know, here is an example of shipment and customer experience. And, um, Yeah, I can take over this part. Yeah, you added all these things. I do want to open it up at this point. Thank you Sanjeev, by the way. Give Sanjeev a round of applause. Very quickly. Thank you for providing a high level landscape of real time analytics. It's really interesting to see Snowflake become part of that through Snowpipe streaming and dynamic tables. A, through the real time ingestion. Right? We benchmarked this with the help of, uh, Xin's team doing high sp high speed Oracle replication into Snowflake, which is a three second latency from upstream Oracle. So what does that mean? Snowflake is a consistent source of truth with your upstream operational systems. How how is that possible, right? It's with Snowpipe streaming, it's with dynamic tables. So at this point, I want to open it up. You know, we have a lot of, uh, very intelligent, experienced people in the audience here. You know, Do you have any questions or, you know, ways you want to adopt Snowpipe streaming? And I'll open it up to, uh, uh, first I'll, I'll bring up the, uh, the benchmark that we built, right, which was showing just on an 8 core stream server. Uh, I'll jump ahead to that. Right. Just on an 8 core stream server, actually showing the replication performance to Snowflake and having just 3 second latency from Oracle to Snowflake. So, how, how many people show of hands here have heard of, uh, Lambda architecture? Okay, great. Oh. Well, it should be everybody now. Yeah. Because I, I already mentioned it, so now, Yeah, yeah. Do you want, do you want to define Lambda architecture for that? We, about half the people raised their hands. Yeah. So Lambda is the idea of having, uh, separate batch processing and stream processing as parallel pipelines. Now, how many of you have heard of cap architecture? Show of hands. A little less, but you know, some very knowledgeable people in the audience, so we still had some hands up. Cap architecture is the idea of, Having stream processing upstream to batch processing. So having the idea of, you know, unifying streaming and batch processing through stream to Snowpipe streaming dynamic tables is a really powerful concept that, you know, a lot of enterprises are already adopting and getting value from because it essentially unifies everything. So the next question I want to, well, first I want to see any show of hands, any questions for Xin on Snowpipe streaming. Okay, we'll save some for the post networking event. But is anyone from Boston Children's Hospital here? They did a great webinar, uh, using a snow pipe streaming, uh, with Snowflake and Stream and Dynamic Tables, and we're able to get some great use out of that and see the value. Uh, so it's really interesting to see Snowflake and the real time landscape come together. Uh, Sanjeev, was that something that you were gonna expect to see happen so fast? No, actually, for the longest time, we thought streaming is something that's so difficult to do. It's so expensive to do. Why should we do it? But if you think about it, like Tesla is actually a streaming device on the road, you know, and uh, and what happens is, so Tesla is like sending out all these like, you know, coordinates, uh, actually Uber, when we order Uber, that's all streaming data. And then the car goes through a tunnel. And you lose signal and then it starts transmitting again. So to your point about, you know, data getting out of order and then having to backtrack and missing data, it's a, it's, it's complicated. But now, you know, because of all these applications, uh, streaming just got accelerated. Yeah, it's, it's really amazing to see the pace of innovation. Uh, on data management on a I. How do you see a I and data streaming related to each other going forward? So, you know, I give one example of, uh, doing vector embeddings in real time. So that to me is a streaming example. I, uh, although today, uh, this year we are not training or fine tuning our models, uh, to any extent. We are very sort of, you know, Very careful. We're doing drag. We're doing prompting. We're doing in context learning. We're doing through a few short prompting, but not training models. But as the prices of hardware go down, like CPU, GPU become cheaper to train. We're going to start seeing this attempt to have our models stay up to date. And again, it's just like, you know, right now, it's like, maybe that's like five years from now, but technology has accelerated so fast that maybe next year, if we are doing this event here, that may already be happening. Yeah, yeah, absolutely. The rate of innovation since we started doing what's new in data has been incredible. I'm not going to say it's a coincidence, but you know, in in I've been in data for 35 years. I have never ever seen this rate of innovation. There's literally you go to bed, you wake up and there are five new announcements and you're basically just trying to absorb that. And before you know, there's something else comes up. So yeah, absolutely. Every week you log into your Snowflake UI. There's some, some amazing new feature. And yeah, thank you Sanjeev Mohan from Sanjmo. I'm John Coutet from Stream. Thank you to everyone who joined us. Feel free to, uh, help yourself to more drinks, refreshments. Thank you. Okay, we are getting started with our event here. Our San Francisco edition of What's New in Data at Snowflake Summit. What's New in Data is a thought leadership series we've been doing a long time. We've had some great enterprises including UPS, CIBC, folks like the head of enterprise data at Google have joined us. And we really just like telling the stories of great thought leaders and what they're thinking. And today we have, to my left, we have Sanjeev Industry analyst, uh, ex VP of, uh, who covered Gart, uh, data and analytics at Gartner. Without further ado, I'm going to hand it over to Sanjeev to kick it off. All right. Thank you everyone for coming to this evening. Uh, amazing views. We were here last year at the same venue and we did a fireside chat. We had this guy, uh, a good friend of ours from Google, Bruno Aziza, and he and I were literally just chatting. Fighting with each other on stage. But what I'd like to do is I'd like to make my sessions very interactive. You know, it's a small group and it's evening. We've all had some drinks. I had some frozen margaritas before I walked over. So let's make it a fun evening. So if you have any questions, are you have any, uh, like, you know, your air your thoughts because I tend to be an insufferable optimist. Sometimes my wife just says insufferable, but I you know, that's where I see myself. So So let me get started. So I'm talking about, uh, since I'm an analyst, so I talk about the market landscape and I cover, uh, data and analytics from a very, very deep technology space. And then I try to bubble it up into what makes sense. And, um, I see there are three major, uh, major pillars in the data space that are really important these days. One is a convergence of structured and unstructured data, obviously driven by Gen AI. Because so far we've only spent all our time doing structured, now we have to think about unstructured data as well. The second piece is a convergence of batch and streaming. Because it used to be when I first started looking at streaming, it was like, how many of you remember Lambda architecture? So a few of you remember where you had a separate stack for batch, a separate stack for for streaming. That is just just crazy. So that's a second major pillar where we are focused on. And the third one is on iceberg or a common table format. So those are the three areas that are very, very important in my coverage these days. So I'm gonna get started. And, uh, just a brief introduction. I was in Gartner five years as part of the data and analytics team there. And after five years, I decided it's time to branch out and do something on my own. So I started a company with a very strange name. It's called Sanjmo. And, uh, and I had no idea what I was doing. I was just like, let's give it a shot. And it's been literally nonstop. It'll be three years next month. So So I and I wrote a book called data product for dummies. Actually, I'm doing book signing tomorrow at 4 30 PM. If you are around in the expo hall, I'll be signing the book. I also I'm a very prolific blogger on medium. If you get a chance, please follow it. It's Sanchmo dot medium. And I'm also a host of my own podcast. It's called. It depends. So, so with that, there's some links here. So let me let me get started with. So I spent a lot of time trying to figure out, like, connect the dots. In fact, my website is trying to connect the dots. So I had this epiphany that what is how do we traditionally do data processing? So I put it together and very simple. We take the current data, we ETL it, nightly batch jobs or micro batch. We make it past data and then we predict the future. That's just crazy. What we're actually doing is that we're literally predicting the present. When we apply a model that was trained in the past, you can predict the present. You already know what's happening. So this is the reason why we need to rethink and think about how do we, you know, how do we act upon live current fresh data rather than, you know, so it used to be, why did we go to batch? That's because we were limited by the data processing systems of that time, like 20, 30 years ago, it was too expensive to do streaming data. Today, streaming data is becoming the default batch is actually becoming a type of streaming. rather than the other way around. So our entire mentality is shifting towards streaming being the default. So, but the question begs, why do we need, uh, streaming? So I'm going to talk about, uh, actually two major topics, the market landscape, and then we will dive into, into how stream is enabling real time snowflake. So why do we need stream processing? We want to be able to do patent recognition in real time. Uh, fraud, anomaly detection is probably the number one use case. Whenever I talk to, to a customer, I say, why are you doing real time streaming? It's almost always for, for fraud detection, like credit card transactions. Obviously, you cannot do it once a transaction has started. So the second thing is, uh, sometimes we want to enrich the data in real time to do preventive maintenance. For example, I have IOT devices, I'm getting some information or, uh, or it's a factory on the floor. But I need to look up some manuals and enrich the data that I'm, uh, that I'm collecting. And then Uh, also to aggregate data in real time. So I can optimize spend, like, for example, ad revenues, like how much ads are coming in. How should I start to, uh, to leverage that data? So filter data to detect trends again, really important. In fact, um, Uh, I see even LLMs in future will be trained in real time because LLMs are really good at detecting trends, except the data is very old in LLM because they're trained very rarely. So windowing is a very important, uh, piece, uh, like tumbling window, sliding window. So I'm trying to calculate what is a moving average. For example, if my, The time it is taking for me to take a call is going up steadily. That means something is wrong. So every 10 minutes, I'm sampling it. Uh, or I have a 10 minute window and I'm sampling it every 30 seconds. So I'm doing a moving, uh, average, uh, also for trading data to see if the stock prices are going up and down. And then load balance, uh, and paralyzed distributed processing. If I know in real time how my, my, my data is coming in, then I can intelligently load balance it across different servers. So, so those are some of the reasons. And then, uh, finally, uh, Uh, if I want to do stateful processing off historical data, uh, along with my real time data. So for that, we need it. Okay, so one side of the coin is processing. The other side of the coin is analytics. So, so think of it this way. What I just shared is what we like people like me on technology side like to think about. Why do I need real time processing? But then on the business side, this is what business people want. They want to do user facing analytics to improve customer experience. If I'm on a website and, uh, you know, and I'm browsing, I need instant, uh, user, uh, user experience, you know? So, uh, also business people want to make operational decisions. And the only way you can make operational decisions is if you have live data, otherwise it's too late for you to, to, uh, make these decisions. Reduce anomalies like fraud, sensor defect. recommendations leading to high yields. If I'm on a website and I'm browsing, I go to one page and I go to the second page. I want that, that, that website traffic data to recommend what should I look at in real time? Otherwise it's too late. Also, if I have a real time data, then I can allocate my resources efficiently, uh, in real time and, um, optimize supply chain, um, in real time. And then finally, regulatory compliance. So this is also becoming a very important topic. And we've heard quite a bit today about some of the new snowflake. I don't even know how much of stuff I can talk about that's even been announced. But compliance came up all day today. So, so, um, you know, so if you heard it, it's probably, you know, out by now. Trust center is, I don't know, is that, Yeah. Okay. So trust center has like compliance is a very important pillar of some security and privacy pieces. So that gets much better if you have live data, otherwise, uh, it's just too late. Okay. So let's go to what are the key, like what, what does a business want? So the first thing I put here is, Cost performance is really important. This is why streaming has taken so long to get established because the cost of doing real time streaming stream processing and analytics was way too high. So businesses tell us it has has to be cost effective. It must be easy, integrated. In fact, I was just talking to somebody today and I was like, You should be happy with iceberg. You can do multiple engines now, like businesses have so much choice. And actually, he's here. I even mentioned it on the cube today. And he's Yeah, I see you. And he says, Yeah, but they're too many choices now. So so that you know, complexity. In fact, the V. I. Snowflake Summit Snowflakes, uh, two big reasons why people use Snowflake are It's unified and it's simple. So, what is simple, gets adopted. What is not simple, I'll give you a very simple example. When World Wide Web came out, the markup language it used, HTML, was something that just took on, like wildfire. But markup languages, I was using Unix. We had markup languages for years. But it was so difficult to use. So the moment HTML came out, like we were like, Oh, my God, everybody jumped onto one right back. So So this is what businesses tell us. And then finally, that data has to be high quality. It has to be governed. It has to be secure. All the privacy regulations must be maintained. So this These are the three most important things that customers are saying they want. So once I analyze this, then my mind started thinking about how do I create a sort of a taxonomy of how do you do real time analytics? So there are multiple options. I'm going to talk about four different options. So the first option, how do people do real time analytics? The easiest is BI tool. Power BI has Direct Connect. You can connect Power BI to your data source and you can do it, uh, do real time analytics. But most of the time, how do you use Click or Tableau? You actually download the data onto your desktop, right? I mean, how many of you do real time analytics and Power bi? Anyone here? Okay. So I don't see any hands at all. So this is, so I put this here, but this is actually the poor man's way of doing it. You can do real time analytics a hundred percent, but then it has to be on small data. If you are going to consume a lot of data, then you will saturate the capabilities of your BI tool, the network. And so most people will actually download the data onto their desktop. Uh, and that, that's where they do their, uh, analysis. So although, so in this case, what happens is ABI stands for analytics and BI platform. And so I've got streaming data that comes in and then I've got my dashboards. I can just directly, uh, look at streaming data. Most of the time, stream data gets stored in a, into a database and then it, uh, it gets analyzed. So, so this is not really a valid option, but it is definitely a, one way to get started. Okay. Let's look at option number two. This is probably by far the most common way of doing it, which is event stream processing. In event stream processing, you are basically the bringing all the processing of streams into a platform. This platform, uh, is, uh, a lot of times the most popular platform, uh, is called anyone, anyone. What's the most popular event stream processing? Not Kafka. What is the most common event stream processing platform? The de facto standard is Flink. So, uh, so Kafka, and we'll talk about Kafka, Kafka, the message bus. So Kafka sits here. So, so Kafka connect, uh, connects to the sources and it loads it into Flink. In Flink, I can do all the things I showed, like why, like aggregation, filtering, processing. Uh, enriching the data. All that I do in an event stream processing. And by the way, there are a lot of stream processors. So, uh, one of them is actually, uh, stream stream is a change data capture. But behind the scenes it has it has its own stream processor. So, uh, so that's that's what it is. And then you use your either your, uh, Uh, bi tool. So basically instead of doing your your streaming in the bi tool, you now have a separate, uh, tool to do it. So very common. In fact, one way to think of it is that event stream processing. You know how we separated compute and store in the in the cloud for databases. This is a compute. So then the question goes, What is the storage? Well, I'm glad you asked. Because that's option number three. So option number three is where you are taking the streaming data into a database. And then, uh, and this is very hard to do because databases are sometimes built for because the persistence. So how do you get very low latency access? To data. But this is is one of the hottest topics right now. In fact, this is what we are talking about today. How do you make snowflake into a streaming database? So so, uh, snowflake. And how many of you think snowflake is a streaming database? Okay. One hand, but it's rigged. You guys are not. So, so we always think of Snowflake as an analytical database. In fact, in a few minutes, I'm going to break this into two parts, OLTP and OLAP. So, so hold that thought for now, because those are the two databases, but most of the time, Uh, the database is not considered to be fit for streaming, but there are a lot of examples. There is a I'll give you some. There's one company called materialize. Anyone heard of materialize? So that is a streaming database. There are a lot of small companies in this space. There are a lot of examples, but I'm gonna hold that thought for a few minutes. Okay, so this is option number three. And then there is a new option that And I've invented, it's called Unified Real time Platform, which is actually a combination of, uh, of streams. Uh, so it's got, uh, the database, it's got stream processing, and it's got applications. So URP is not that well known, but there are a number of companies in this space. Uh, there's one, I think Ali, you, you connected with, uh, Hazelcast, right? You're on the boat. So Hazelcast actually falls in a URP, a Unified Real Time Platform, because in Hazelcast you can build your applications also on top. So you're not only just streaming the data in, you're processing it like an ESP, and you're building an application. There's also, there's something called Apache Grid Gain, so that also falls under URP. If you want to learn more about URP, This is sort of groundbreaking research. Uh, if you go to my medium, sanjmo. medium, you might have noticed I'm pitching. Um, so, uh, I have a couple of documents on this, uh, on this topic. Okay. So, so with this, um, I want to summarize it. So here I'm going, I'm doing something different. So So first of all, uh, message bus. So all this data that's coming into either the database or, or event stream processing or into a BI tool, it's coming through some protocol and that protocol a lot of time happens to be Kafka. So this is where Kafka sits. Kafka is a message bus. It's a transport layer. And Kafka is actually a commodity. In fact, there's Kafka and then there's Kafka like. Kafka like. Why do I say Kafka Lite? Because there are probably 20, 30 different Kafka types. There's one called Red Panda. Has anyone heard of Red Panda? Okay, so a few of you. There are, uh, Amazon managed, uh, managed service for Kafka, MSK. That's, that's Kafka. So there are open source versions. There are, uh, uh, commercial versions. So there are a lot of different, so there are about 20, 30 of these. But it's not just message bus, uh, event broker, change data capture. So the second half of our talk, we'll talk more about change data capture. What is change data capture? But for now, it just says CDC here, WebSockets, Webhooks. But then there are other products. Every hyperscaler has their own product. Azure has Event Hubs, Amazon has Kinesis, and then Google has PubSub. So these are the transport layer. But when the data comes into that, then, uh, here I've got these four options. One, what I did here is I broke up the stream enabled database into two types. So why did I do it? Because remember I said databases usually come in OLTP and OLAP? So OLTP, streaming database, I gave you an example that's materialized, for example. But on the analytical side, there are lots and lots of choices that you may have heard of. Clickhouse? Has anyone heard of Clickhouse? Yep. Yeah, Clickhouse is very popular. That's, you know, a rock set. Uh, Droid, Pino, StarTree, Imply. So there are all these databases. So this is a very popular category of, uh, and this is where Snowflake would fall because Snowflake is an analytical database. So now I'm going to finish with one more slide, and then we're going to go to the second part. So there's a lot of stuff here. But what are some of the most essential design criteria that I need for a streaming real time streaming platform? First of all, it has to scale because the problem with with real time streaming data is that it's unbounded. Unbounded means unlike a batch which has a beginning and there is no beginning and the data is just coming. These are like the web logs that are coming, IoT sensor data is coming. So scale becomes extremely important. And then of course, how cost performance includes throughput and latency. How quickly can you process that data? A lot of event stream processing Products are in memory because that's how you get speed. So, uh, so there's a lot of, uh, performance and then it must be fault tolerant because, uh, because you are scaling and you've got a cluster with. In fact, some companies like Walmart, for instance, has 1400 clusters of Kafka. Every department has, you know, its own cluster. So fault tolerant, having an ecosystem, this includes things like connectors because I'm going to get data from different sources. So I need all kinds of connectors. We talked about governance and then where is it deployed? Is it deployed on premises? Is it deployed in the cloud? If it's in the cloud, is it one cloud? Is it hybrid cloud? Is it in my VPC? Is it in vendor VPC, multi tenant? So, so these are some technical considerations. So anyway, um, so just a quick, uh, conclusion, um, first of all, why do all these options exist is because, um, Different use cases call for different requirements, so it's not like I cannot have like bi is old. I should do event stream processing. I should do streaming database. A lot of people I talked to like large customers like like DTC DTCC probably has all the four options, at least three of them. So because different use cases will require different different choices. URP is new because I'm trying to to connect the event stream processing, real time database and application development. Also, I'm trying to to handle both async and sync process and also data in motion and data addressed. So that is where URP is a new thing. Uh, Jenny, I, uh, is, uh, is raising the profile of streaming data because we are trying to get to a point where, uh, when you ask a question to a chat bot, your question needs to be embedded. Uh, sorry. It needs to have a vector embedding created. That vector embedding will be created in real time. So then you can send it to an LLM. So, so this is one of the reasons why streaming is now so hard. Uh, and, uh, even text to video. Like if you ask a question to perplexity or to some other model, then your answer must be generated right away. Have you noticed? Like if you ask chat GPT to summarize a long document, it starts showing you the results without completing it because it's streaming. It's, you know, because if you wait for two minutes, it's just too long. So, and then stream and snowflake, uh, I have now collaborated because they can provide under one minute latency for, for data that has all the governance around it. And so that is a topic for the next, uh, remember I mentioned about change data capture in my, uh, input to all, all these, uh, options. So what is chain data capture? Uh, this is this is the bridge between OLTP transaction and analytical databases. What we are trying to do is, uh, operational databases are constantly being updated. So if so, obviously, I want to run my reports on the latest data, but if I run it on my operational database, I will slow it down that that as a result, I won't be able to take any new orders. So I cannot overburden my operational database by doing analytics on it. So then what is my option? My option is to do chain data capture. What that, what this is showing is, this is my operational database. Inserts, updates, deletes are happening here. And as the data is written into the database and the transaction is committed, I'm going to take that data from a log that the database maintains, and I'm going to write it to my, uh, my data warehouse. And, uh, there are different types of logs. If you use Oracle, then you have redo logs. If you use, uh, MySQL, it's bin Log. Uh, Postgres has something called write ahead log. MongoDB has called something called MongoDB Streams. So, so these databases, operation databases have a, have a, uh, a way for, for target to, to accept this data. Okay, so here is an example using Stream. So stream, uh, is, uh, one of the, uh, one of the long established choices here. So you can see, I've got data coming into Oracle, MongoDB, Salesforce, which is CRM, Stripe, where my payments are coming in. So I've got all kinds of data that's coming in. Uh, it's very common these days. For even a small organization to have more than a hundred SAS tools. And if you're a large organization, it's easily four or five hundred. So you got all these, all this data coming in. Now you need the ability to capture it in real time, or near real time, and then write it into Snowflake. So for that, we are using something called Snowpipe Streaming. So when I first started looking into, uh, into Snowflake, it used to be that Snowflake, just a year or two ago, would, uh, would let Stream write the data onto S3, or, or GCS, or Azure Blob, and then Snowpipe would actually read that data from S3 and write it into Snowflake. Today is different. Today, the data comes on Kafka and, and, uh, Snowflake can read it directly. Uh, you know, so, so now it has this ability to read the data directly as it is being generated. In fact, it's actually very interesting. You see here schema metadata lineage. Uh, I may I may not even have a table. I may have a new data that is being, uh, streamed, uh, through chain data capture. I haven't even created a table and I can set up snowflake so it can look at the schema off the data and boom, create a table for me on the fly. So it's super interesting how far snowflake has come. In, in, uh, in handling this, then, uh, what stream, uh, stream gives you this whole, uh, sort of a, uh, governed platform? Because schema change. What if somebody deleted, uh, a column or renamed the column? What do you do then? So, so you need this ability to, uh, to, uh, to handle schema changes. In fact, one of the very interesting thing is, if somebody updated, let's say, you know, my salary, I got a bump and my salary got, went up. So great. You, the update happens in the source. I can, uh, send that chain data capture into my target and I can update my salary. But what happens if something is deleted? Because now that that causes a lot of issues. If you delete it in the target, it can cause, uh, you know, acid, uh, issues. And if there's dependencies, you know, so, so, so behind the scenes, what stream is doing is actually very complicated. It just looks like, you know, it's quite simple. You're just connecting source and target. Okay. So, here is an example of, uh, something that, uh, that is called dynamic tables. So you've already seen, you've got source, stream does, uh, chain data capture, and then it comes to Snowflake, which has this concept of dynamic tables, which is also something very new. In a dynamic table, it is actually like it's it's like a materialized view. It's actually kind of refreshing because the data is coming in constantly. So it's keeping track of data as it's coming in and and then and then you can build your apps on it. Now we've got the expert here. then. So if you have any question, I'm going to direct it to him. We also have Pura, who's an engineering manager. He's also here. I'm just putting him on spot. So, okay, so, um, so, uh, this is basically, uh, an example of how, uh, same thing. You know, here is an example of shipment and customer experience. And, um, Yeah, I can take over this part. Yeah, you added all these things. I do want to open it up at this point. Thank you Sanjeev, by the way. Give Sanjeev a round of applause. Very quickly. Thank you for providing a high level landscape of real time analytics. It's really interesting to see Snowflake become part of that through Snowpipe streaming and dynamic tables. A, through the real time ingestion. Right? We benchmarked this with the help of, uh, Xin's team doing high sp high speed Oracle replication into Snowflake, which is a three second latency from upstream Oracle. So what does that mean? Snowflake is a consistent source of truth with your upstream operational systems. How how is that possible, right? It's with Snowpipe streaming, it's with dynamic tables. So at this point, I want to open it up. You know, we have a lot of, uh, very intelligent, experienced people in the audience here. You know, Do you have any questions or, you know, ways you want to adopt Snowpipe streaming? And I'll open it up to, uh, uh, first I'll, I'll bring up the, uh, the benchmark that we built, right, which was showing just on an 8 core stream server. Uh, I'll jump ahead to that. Right. Just on an 8 core stream server, actually showing the replication performance to Snowflake and having just 3 second latency from Oracle to Snowflake. So, how, how many people show of hands here have heard of, uh, Lambda architecture? Okay, great. Oh. Well, it should be everybody now. Yeah. Because I, I already mentioned it, so now, Yeah, yeah. Do you want, do you want to define Lambda architecture for that? We, about half the people raised their hands. Yeah. So Lambda is the idea of having, uh, separate batch processing and stream processing as parallel pipelines. Now, how many of you have heard of cap architecture? Show of hands. A little less, but you know, some very knowledgeable people in the audience, so we still had some hands up. Cap architecture is the idea of, Having stream processing upstream to batch processing. So having the idea of, you know, unifying streaming and batch processing through stream to Snowpipe streaming dynamic tables is a really powerful concept that, you know, a lot of enterprises are already adopting and getting value from because it essentially unifies everything. So the next question I want to, well, first I want to see any show of hands, any questions for Xin on Snowpipe streaming. Okay, we'll save some for the post networking event. But is anyone from Boston Children's Hospital here? They did a great webinar, uh, using a snow pipe streaming, uh, with Snowflake and Stream and Dynamic Tables, and we're able to get some great use out of that and see the value. Uh, so it's really interesting to see Snowflake and the real time landscape come together. Uh, Sanjeev, was that something that you were gonna expect to see happen so fast? No, actually, for the longest time, we thought streaming is something that's so difficult to do. It's so expensive to do. Why should we do it? But if you think about it, like Tesla is actually a streaming device on the road, you know, and uh, and what happens is, so Tesla is like sending out all these like, you know, coordinates, uh, actually Uber, when we order Uber, that's all streaming data. And then the car goes through a tunnel. And you lose signal and then it starts transmitting again. So to your point about, you know, data getting out of order and then having to backtrack and missing data, it's a, it's, it's complicated. But now, you know, because of all these applications, uh, streaming just got accelerated. Yeah, it's, it's really amazing to see the pace of innovation. Uh, on data management on a I. How do you see a I and data streaming related to each other going forward? So, you know, I give one example of, uh, doing vector embeddings in real time. So that to me is a streaming example. I, uh, although today, uh, this year we are not training or fine tuning our models, uh, to any extent. We are very sort of, you know, Very careful. We're doing drag. We're doing prompting. We're doing in context learning. We're doing through a few short prompting, but not training models. But as the prices of hardware go down, like CPU, GPU become cheaper to train. We're going to start seeing this attempt to have our models stay up to date. And again, it's just like, you know, right now, it's like, maybe that's like five years from now, but technology has accelerated so fast that maybe next year, if we are doing this event here, that may already be happening. Yeah, yeah, absolutely. The rate of innovation since we started doing what's new in data has been incredible. I'm not going to say it's a coincidence, but you know, in in I've been in data for 35 years. I have never ever seen this rate of innovation. There's literally you go to bed, you wake up and there are five new announcements and you're basically just trying to absorb that. And before you know, there's something else comes up. So yeah, absolutely. Every week you log into your Snowflake UI. There's some, some amazing new feature. And yeah, thank you Sanjeev Mohan from Sanjmo. I'm John Coutet from Stream. Thank you to everyone who joined us. Feel free to, uh, help yourself to more drinks, refreshments. Thank you.