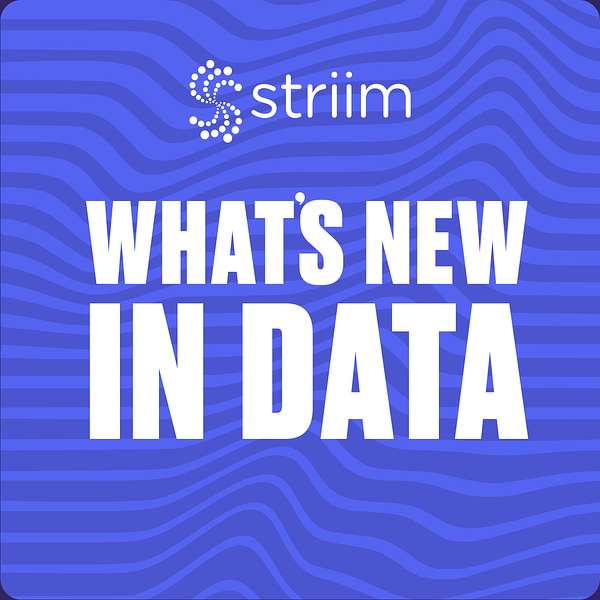
What's New In Data
A podcast by Striim (pronounced 'Stream') that covers the latest trends and news in data, cloud computing, data streaming, and analytics.
What's New In Data
Is Text-to-SQL Ready for Prime Time? Insights from Ethan Ding, CEO of TextQL
Can AI really make your data analysis as easy as talking to a friend? Join us for an enlightening conversation with Ethan Ding, the co-founder and CEO of TextQL, as he shares his journey from Berkeley graduate to pioneering the text-to-SQL technology that's transforming how businesses interact with their data. Discover how natural language queries are breaking down barriers, making data analysis accessible to everyone, regardless of technical skill. Ethan delves into the historical hurdles and the game-changing advancements that are pushing the boundaries of AI and large language models in data querying.
Ever wondered how the quest for full autonomy in self-driving cars relates to data? We draw fascinating parallels between these two cutting-edge fields, emphasizing the importance of structured systems over chaotic, AI-driven approaches. This chapter reveals the often-overlooked limitations of current data management practices and underscores the critical need for high-quality data and robust modeling. Through a comparison of traditional business intelligence tools and advanced AI-driven solutions, we explore what truly makes data querying effective and insightful.
Hear from Ethan Deng, co-founder and CEO of TextQL, as he explains how their innovative tool integrates seamlessly with existing BI infrastructures, boosting productivity without the need for disruptive overhauls. Tune in to find out how TextQL is making data-driven decisions faster and smarter, paving the way for a future where data is everyone's best friend.
Follow Ethan Ding and TextQL at:
- Ethan's LinkedIn: @TheEthanDing
- Ethan's Twitter: @TheEthanDing
- TextQL's LinkedIn: @TextQL
- TextQL's Twitter: @TextQL
- TextQL.com
What's New In Data is a data thought leadership series hosted by John Kutay who leads data and products at Striim. What's New In Data hosts industry practitioners to discuss latest trends, common patterns for real world data patterns, and analytics success stories.
I'm You Hello, everybody. Thank you for tuning in to today's episode of What's New in Data. We have an awesome guest today. A lot of people told me I had to talk to him specifically about this topic of text to SQL. Ethan Ding, Co founder and CEO of TextQL. Ethan, how are you doing today? Doing good. Thanks for having me, John. Absolutely. Absolutely. We last crossed paths at a Snowflake Summit. We were able to catch up there. Always love hearing your perspectives on. The things that are going on right now in the market, especially with respect to AI and how it's converging with data and analytics, but first tell the listeners a bit about yourself. Yeah, I, graduated from Berkeley a fall ago and I, right out of college, I started working at a VC, he's called Bessemer. They, introduced me to one of their portfolio companies. I started running their 10 person data team there, and then. The I guess I just entrepreneurship bug bit me, or I think that's the thing you're supposed to say. And I started working on on this 35 year unsolved Texas Eagle problem. And so in the past two, two ish years that's what we've been working on. We're about 10 people. And the we've raised 4 million in work with places like the NBA and Anheuser Busch. But the, technically that's the thing you're supposed to say to the, very to to the, press. I think the way closer to the truth is that I. Entered in a VC, started a company, worked at a startup, and then was probably a little bit too hyperactive and aggressive and had the, all the humorous of a 22 year old who like doesn't understand that people have been working on data problems for the past 50 years. And after I got fired from that job after a year and a half and I was like 30 days from being deported I just met a guy on the internet who turned out to be my co founder. We exchanged like 10 messages for like, all right let's just see. We can solve together. We knew that we didn't have the connections to get like a bunch of companies to become our customers right away. So we're like, okay The only thing we have going for us is that we're fairly young so we have, we have 12 hour days, no kids to look after dedicate to the, craft. And so we're like, let's just pick the hardest possible problem and the data space that we've, that everyone's like kind of tried to fail and solve. And there's like a 3%, 5 percent chance that we can solve it. The expected value on that might actually be pretty high. But yeah that's the background. Yeah. Definitely appreciate you for being a direct, a lot of people do respect your opinion here. And I think it is. Directly related to the fact that you're so obsessed with solving this problem, really end to end in a way that's actually resilient and scalable. And that's this problem of Text to SQL. And of course, this is all tied to the fact that AI is becoming very popular generative AI specifically the, just today on the day that this podcast is being recorded on September 12th, all the AI leaders in the U S are at the White House. Briefing the president and the executive staff on. What we're actually going to do as a country with respect to what are our needs and what are our goals to actually make sure we're the leader in AI now, bringing this back down to this discussion, which is okay, Text to SQL, maybe quickly, like if you could define Text to SQL and the value of it for the listeners. Yeah, at the, at the most naive level, it's this idea that it rhymes with just users not having to write code. This idea that everyone's now a software engineer. If you spend any time on Twitter or LinkedIn, you might have seen a ton of people posting about that. And it's that you shouldn't have to know how to write code. You should just be able to ask a sentence how many Okay. How many customers of ours from the Midwest region engaged with one of our pieces of marketing and then give me a list of all give me the list of all those customers. And then let me like do a bunch of stuff with it, right? Sequel is. The probably the most, most similar to natural language in terms of the coding languages. And so I think a lot of people felt like this was going to be like a really easy problem to solve with large language models coming up. I think it was the second most common like company idea in the YC application batch for the three, for three batches in a row, right after chat GPT came up. And yeah that's the, Seems to be the, very much it was front of mind for a couple of years, but it has a super long history. 35 years ago, like SAP business objects was the first company. That tried to say, Oh, your business operators won't have to learn SQL. They can just type things. And at its simplest, it could just be typing sentences like, get me top seven, like assets by by week, by month, by state and. Basically, you're just teaching someone to write slightly more compressed SQL in, English, something that looks like English. And at the ultimate end state, right? The dream for text to SQL or for like true self service analytics is you don't have to do a thousand hours of cleaning. You don't have to do a thousand hours of documenting. You don't have to like teach someone exactly what kind of syntax is they should be able to say something meaningfully like nebulous, like how we make more money. And that should return like a intelligible result. It won't be an algorithm for you to make more money reliably but it should be able to explore your data for you. It should be able to answer questions for you and along the way, it'll generate a lot of SQL to explore that data for you. Absolutely. This all comes back to this like very like fundamental question. Okay. We have chat GPT now. Can we have chat GPT basically for our internal data? Can someone from marketing or sales just go log in and say, Hey, show me my top 10 customers right now so that I can put them into this email campaign for loyalty that we're doing or just make all this data very accessible and simple. Is it actually possible with the technology we have now? For teams actually build like a chat GPT style interface for their internal data. I think everyone's in the past two years, has noticed that despite all the companies that all the enterprises that have said we're going to bring you like chat GPT for your, specific data within their system. They don't feel that every day. You're still submitting JIRA tickets to your data team. That takes two weeks to two months to turn around. And you're still like, yeah, if you're on the data team, you're still getting people like tapping you on the shoulder and going Hey, please, can you pull me this report that asked you for last week, like seven times over again? And it's that I think most companies today are, I've seen them get trapped in this, in this like local optimal called like the, I would call it like next token prediction based text SQL. And it's, it looks like you type in a sentence, you just ask the language model, here's the schema. Can you generate like the next tokens? Maybe you do some fine tuning on the side of the large like cloud providers. They have a very high incentive to tell your data leaders to, So I keep just putting more money and more money into like fine tuning it, which really just means like tuning it to your hard coded schema, because as we all know, our data schemas definitely never change on a regular basis. And then because, and because you can always say that it is like a black box and so it'll nebulously approach something that's good enough to accurate. It siphons up a lot of energy. The spider benchmark for a Text to SQL is something that I think a lot of people like try to use as like a benchmark for performance. And the reality is it seems like, we've built, we knew we didn't know very much going in. We'd rebuilt the platform like nine times over at this point. And I think we gave up on that. Style of like black box next token language model generation based like Texas equal a long time ago. I think we have a path forward to like really, good. Texas evil like across the board for like for most use cases that we can conceive of. Chat GBD for your data is definitely not just a pipe dream. And it's something that is well in a way. But you just forecast how long it took. How long for us to get like personal computers out of the early computing paradigms language models very much feels like a new class of computing and I would expect it to take maybe two ish more years before we get, where we feel every single day that like all the questions that we want to ask are just at our fingertips, we're able to get this number, we're able to get this list of customers, we're able to get this like set of criteria or forecast this like direction or forecast this metric forward by three months with some confidence intervals. Yes, but we've been stuck in somewhat of a local minimum for about two years now. Absolutely. And you, mentioned Spyder, which was a benchmark that a team from Yale ran on Text to SQL. And they mainly focus on trying to make it real by having a very complex cross domain data set. And it seems like nothing is really performing at the level where it's actually bulletproof and always going to give you the right response. So it's not there yet is what you're saying, but we're, getting close. So with that being said what is the right way to do it? If you're a data team tasked with delivering something like this? When we first started, we like Spyder was like our everyday, like we used every day, like we dug into the research papers or like methodology to the past I think we, we actually spent a lot of time with the guys over at ServiceNow who produced the who wrote the paper Before language models and transformers of a different class of model for doing who like nailed up to 85 percent was the leading like accuracy. What he established with us was like the problem with text to SQL was never like, like natural language, like interpretation. It was that, The last 20 percent of those questions are so ambiguously phrased that there is no, there's no reasonable way to conclude that this answer that they have, like in this benchmark is like the correct answer when they're like three or four other potential answers that are equally valid. And so at the end of the day, if you like, if you're hitting over like 85 to 90 percent accuracy on that benchmark you're probably just hard coding it to that. Like you've probably over tuned the test set. And. What that takes us to it, it takes us to the fact that like self driving cars have five years ago, everybody thought autonomous driving was going to be a very competitive field. There were so many companies raising like multiple billions of dollars to go after it. And today, I like. Waymo is alive, kept alive by, Waymo is working and getting very close, but still not quite there, only in a few cities and it's because the long tail of these, human, human error, or human interpretation, or schema error type things are basically impossible for us to get 100 percent out of a black box. And. Yet, if we look at places like if we look at places like Tokyo or Shanghai there are already very large pieces of transportation and infrastructure that are autonomously driven their cars, they have wheels but they're not they're not, they don't drive on roads. They drive on train tracks. And it's because they built they built systems around not human drivers but around like getting people from point A to point B. And that's, I think the lens that we should take for like language models. And Doing Text to SQL, which is that the goal is not to generate SQL, like willy nilly on anything in like a way that we're not like worried about. The goal is to get insights. The goal is to get your users to be able to ask a question and get the answers they want right away. And that is. Like for the most part, like you, you have history, you have footprints that you've left your analysts every time they write a query every time they run that against the warehouse. Every time they get a data set back because somebody asked the question, that's a footprint that they're leaving behind. That is an indicator that like these joint paths are the kind of joint paths that need to be run. And from there. You can, almost compress the problem of like text to SQL down to, that's a series of checkboxes. And language models much more. I would trust them much more to like transparently take a series of checkboxes to find a series of attributes to answer the question then to figure out every possible possible join that you're brute forcing with like fuzzy matches or all of the, data munching that I think, anyone who's worked in data is like super familiar with. Yeah, and I, that's a great description of where we, are now and the way you have to abstract it to be successful in the future, which is essentially, yeah, you're, building roads for your agents to navigate. Between your, internal data sets, your core data models that are, surface of the business. You don't want it going through too much raw or uncurated data. Something that could be it happens all the time, like where, data teams are ELTing all their internal data into the warehouse. A lot of fields might be something from sales force that the sales team doesn't, know. Even use anymore as a deprecated field, but no one tag is deprecated. So to your LLM and very well, it could be like a source of truth. And so there are all these challenges that can be solved with good internal data quality and good internal practices for data modeling as well. Which again, creates more overhead, comes back to your problem out. Yeah, I need a report. I still need someone to go in and spend. Two weeks to actually pull the right data. Cause they're actually going to do this nasty stuff that I mentioned, which means like going and asking the data producer is this data is this field sold accurate, or is it deprecated? And doing all this other stuff, like pulling in. Third party data sets, et cetera, right? So none of that stuff is exactly solved here now, that will get solved, right? Data teams are continuously getting better. Thanks to all the technology coming in the market that accelerates things. But coming back to the last question, which is what's the right way for data teams actually? Deliver something that's like chat and QPT for their data. Maybe we'll step back and say, okay, should we even care? Why not just keep the status quo with current business intelligence tools? Yeah, that's fair. It's, it is a, it's one of those pains where You're just used to it. Like this is you've you were able to, your business was fine last year. It was fine the year before necessarily because it was not fine. You wouldn't be around anymore. Therefore you probably had a good amount of time where you've you have gone without with, having just like brute force, your SQL on brute force, your like your questions and waiting like two months, two weeks to two months just isn't, doesn't take that long. And. And like at the day, your BI tools, like most of the questions that you're asking are going through the same paths, the, I think, actually something really quickly to touch on something you said about the fact that all these problems are going to get solved, it actually feels like right now, if I gave you. Like a billion dollars and an army of a thousand engineers. And I told you like for a large enterprise like Goldman Sachs or capital one, like clean and organized and catalog their S3 that might actually be an intractable problem because even with a thousand people, just the communication bank, there's a maximum amount of throughput of work you could do. And, the end of the day, there's so much stuff getting dumped in there that this idea, there is more data being produced than we can catalog. And I think anyone who's used any of the AI generated, like data descriptions and stuff in the past year has found out that language models without the context of where the data came from and not all data comes in the context of where it came from can't really be trusted to catalog that data. And that problem actually looks a lot like, it actually looks a lot more Google apps where, you know if, Google maps relied on every single. Like some like data governance committee to manually update like the like open and close time of every single restaurant on the planet and updated as the restaurant closed or all these things, they would have a really, hard time. And that's and the way Google Maps saves up data is they make that a capability of updating that system of record a a stochastic system. Where you have a bunch of users they like note when something is wrong and vote with their actions, vote with their decisions to actually go to a restaurant. You can see them like physically go to the restaurant from the geolocation. In the same way, we have those footprints that your analysts are leaving across your org, where if somebody goes back to a dataset over and over and over and over again even if they didn't clear this with the data governance committee, even if the dataset is in like Google sheets or something cause we've all seen that. Pain. That's probably an important data set. It's probably a genuinely valuable source of truth. And Whether or not it's been stamped source of truth doesn't matter if like your entire customer success team uses this as like the canonical like data catalog. And so as you build out as, other solutions are built out that are able to get your users numbers faster, you see something that's similar. That takes place with hedge funds, or if you actually look at the airline industry, how they first adopted like dynamic pricing. I think it was either American Airlines or United that first discovered that if you could get numbers faster, and you're able to drive analytics faster to drive up the price of a seat between Texas and between Austin and like New York, that Like it helps you like capture some amount of value. And for about like 18 months after the first airline adopted it that airline just dominated every single, like every single quarter, their bookings were higher, the revenues were higher, the profit margins were higher because they were like crowding every, they could just make decisions faster. They can make data driven decisions 10 to a hundred times faster than every other airline on the market. And they didn't have to pay a margin to the human operators that had to stand there in the way. And then because of that first one which only came about because I think the CEO was like sitting in the. Sitting on a plane in first class with the IBM CEO by accident every other airline had to keep up and they had to build out their own system. And the dynamic pricing had to keep up. And so in this world, like you, You don't have to be like right now, like those airlines are fine. But those like 18 months where the first early adopters were able to move and they were able to dominate the market share for a very short period of time with an overwhelming advantage. That's what data driven is supposed to mean. That's what it's supposed to mean when you can move faster with more confidence, with more numbers with 10 to 100 times more numbers at 10 to 100 times the speed. And so existing B. I. Tools are great for visualizing things. They're critical on every basis. Your analytics is a commodity. And if you're Nike competing with Adidas, if you're Kroger competing with like another grocery chain, if you're Walmart competing with Target, your ability to win is a function of how much more quickly can you get information about your business than your competitors? And if you both have the same B. I. Tools, which, for the most part, you I guess companies that large tend to have every B.I tool. There's not a lot of competitive pressure for you to need to like do something about it. So you're saying that, they're all using again this original question was like, why not just keep the status quo at the current business intelligence tools that are popular in the market? Your point is that analytics is an arms race of getting numbers faster and better than the competition, essentially uncovering alpha, that means that you have this kind of more creative thinking and not be limited by the status quo, typical tooling that's in the market. You need to think about how you can, you as a enterprise can innovate and beat your own competition. And I think this does come back to the value of accelerating analytics by making the data more accessible to the operational teams that are actually going to directly tied to the business okay, if we're in an airline, yeah, we have the super smart pricing seat pricing system. If we're a retailer, we have dynamic pricing, surge pricing, et cetera. And then of course making customer experiences better and more streamlined can always add additional value. If, those retail experiences of contextual, right? Like you walk into. Your favorite retail store. And they're like, Oh, last time you were here, you were shopping for a shirt, but you didn't buy one here where you'd put out all the shirts that you want to could potentially own. So you don't have to linger around and wait for someone to come talk to you and get lost in the store or whatnot. So there's all these places for data to quickly add value. So now we're talking about thinking outside the box and doing, innovative things with, data. First I want to ask you a good, so you're CEO of TextQL You recently just raised 4. 1 million in a seed round, and many people are talking about what you're doing and consider you a thought leader on this particular topic of Text to SQL. So I want to ask you what, does TextQL do? We are, we help you get numbers faster. That's the tagline. I was trying to think of something clever related to high frequency trading. But ultimately like your, business people. Companies do better when your business operators know more information. And if you've no more information than your competition then you do like even better still if you Toyota early like assembly lines, like the, operators knew, like the failure rate of every single component on the field. And that just that's why they were able to outclass American manufacturers, like for those early days at high frequency trading firms, they can get like the price of a stock faster at like the With higher precision than at like other, like traditional banks. That's why they were able to edge out an edge, eke out an edge. And and for us we, plug into your business users. They don't care about your difference between a spreadsheet and a database and a data warehouse and a BI tool. They just know that you have some set of data assets across your organization. And it's stored in some place and nebulously called like the data, the cloud or the databases that you have. And we, plug into all of that. We're, building an agent. That can read from your bi tool from your tableau to your power bi to your data bricks, your snowflake across multiple clouds and pull that data into one place so that you can ask any question from, what are the which plants that are manufacturing cans of Coca Cola have the highest volatility. To like temperature because you see a cold front coming up to having which like, show me all the fans of the warriors that tuned into the first 30 minutes of the game, but then tuned out afterwards. And then ship like, so that I can send a marketing campaign, get me their emails, give me their favorite player and favorite team. So I can send a, like a 15 second clip from the second half of the game that they didn't watch to their emails so I can activate them And so the, like we were. We're trying to build the magic box, the magic autopilot for getting analytics and trying to drive data driven decisions at a higher frequency for, most of our, customers. Yeah. Absolutely. Powerful stuff. What were some of the core technical decisions you made in your product to enable this type of power? Because the way you describe it is business users don't have to be aware of these technical details, whether it's in a warehouse or a spreadsheet or what have you. So you've clearly abstracted that in some way. You don't have to reveal like your secret sauce, but maybe at a high level you can talk about some of the cool stuff you've built to enable this. Thanks. I don't actually believe in secret sauces. I feel like you should put all of your plans on the table because if you're like really good, you're going to build something valuable. People should come to you. And that's ultimately like how you make your, get your advantage. We, do we, we build something called an ontology. It's, you can think of it almost like a map of your, like a Google maps for all of your assets. It sees like the times that you've gone from you've gotten a ticket in Jira. It takes you in a snowflake. You write a certain kind of query that references these backing tables with these join paths. And then eventually it pushes in the Tableau and it ties all of that together. So that the next time like a user like a user asks a question to our agent, they're not generating the SQL. They are traversing the paths you've already taken through your data. Because knowing that you as a human have gone through that before. It's probably a more reliable path. And the more your people use it the more paths, like the more footprints they leave the more these the more the canonical objects that are, like, that represent your contracts, your entities, your conversions, your payments, your bills, your logs they become, crystallized. And then using that, we, traverse this graph and we are able to get you your numbers back. Yeah that's, absolutely incredible. And I think this is where a lot of data teams struggle is like the, even the concept of a, of an ontology on top of your internal data can be, quite an effort to build out and, data teams also, ultimately they don't want to be in the position where, you know, every time they get a request it's, It feels ad hoc, out of left field, and they have to do a bunch of work, which is blocking infrastructure work to go serve it. If that thing is just an out of the box insight that a business user can go access themselves, that's amazing, right? The, even the way I look at organizing our data internally is that we have to do extra work to make it. Easy for business users to use, right? We have to do a lot of ETL and modeling on the data and then think about how we're going to sync it to HubSpot or, Salesforce as like a really, easily readable field, but that's work, right? That's always engineering effort. I would never tell our, sales team, Oh go have fun with our data warehouse and figure out like what leads you should go after, right? Cause it would never work. So I, do really see the value here to data teams, because data teams ultimately their job is to just maintain the data state and the infrastructure and then making it easy for both business and technical users to get insights from that data and operationalize it. The I think one of the things that a system there's some old idiom and it's, that The purpose of a system is what it does. And now what it claims to do and if you have a ticket queue that somebody can submit or if you have like a field and like snowflake It's not like you've like finally brought in. And if somebody asks a question about it and you say oh random salesperson. Could you like learn sql and figure it out yourself? And in doing that you're not you're the claim is that you're helping them like find the data Because you've already done the work to bring it in but if the result is that they don't Go learn it. And I think we all know that they're not going to go learn it. The purpose of that action is effectively to prevent them from like answering the question. And I think there's a lot of, people are going to behave a certain way. They're going to go through a certain set of tools. You have to bring the data much closer to them for them to feel comfortable. And the longer it takes, the less that's a necessary trade off that everyone has to acknowledge. And and so if we can make it a lot faster, then hopefully there's a lot more data that's available for everybody. Absolutely. And yeah, the reality is that people focus on what they're good at, right? Salespeople have to go out and sell marketers up to do marketing. And SQL isn't a skill that everyone has. And even if you do know SQL. Learning your company's internal data state is additional overhead, right? And the best possible scenario, if we were just make this really simple a seller just going in and asking whatever the internal data system is, hey, give me the top leads for me to go after. I don't have to do select from this table, do a join across, my, my product databases and my marketing databases and my third party, lead scoring systems. And you don't have to think about that, right? So I think this is the path for a future where that is possible. People can just chat with their data and get the response that they need. That's, relevant to their operations. I think we're, I think we're close. First it's gonna be we need to build out the systems that let let relatively clean data to be analyzed very well. And then we need to build the systems that brings basically all of your data that's not super clean into a place where it's like clean and that that has to come from both sides. I'm super excited to see what everyone is working through. But yeah. Yeah, absolute a little faster. Yeah. And. In a lot of these, in most of these situations, you assume that most companies have some table stakes, data infrastructure. They have a, maybe a lake or a database and then some ETL, what are, and of course a cloud provider. So what's the core infrastructure that you've prioritized to build on, top of? So for us we, connect to BI tools and data warehouses as a priority. And then we have built our ontology to be like you, any semantic layer can build into it. And so this way we have a kind of a, it is meant to be like the widest. Possible like we almost treat. We almost treat each tool as like a location for us to go to for our agent to be able to traverse. And a car would be a pretty crappy car if it could only go through two blocks. It should be able to get you to every single block. And so it's one place for you to ask those questions. And so we've definitely Redshift, Databricks, BigQuery, all the standard warehouses like Tableau, Power BI, Sigma and, Probably going to build out something for looker, like fairly soon. And so the, at the end of the day, if you like zoom out really far, like every single, SQL query in snowflake really is just table. And every single table and stuff like it's just to every single model and dbt is a table and every single, Every single metric with a couple of dimensions and a semantic layer is a table and every single dashboard is backed by a table. And so there's a, really cool way that you can unify all of these things into a standardized format. And just search through all of them. Cause it just, it's, it you have, tables all over the place. And your, business people just, they're, not going to know. The difference and you need to be able to ask questions across all of it. Yeah. And it's great that the way this solution is positioned textual specifically assumes I have BI in place as soon as I have a data warehouse in place, so you're not going in and. Replacing one or telling its name, okay you have to rip and replace your semantic layer, you have to rip and replace a BI and use our, cool thing, right? So I think, that, that, makes it almost more practical in a way, even though it has a very, grand Vision for how it can speed up productivity for your data teams. It's not it's, almost evolutionary, not like revolutionary in the way that it's building on top of what you have rather than telling you, you have to replace a bunch of what you have, which I think makes it more practical and adoptable for, data teams. I think when we first started, there's strangely like a, there was a narrative that I super bought into at the time, which that's definitely going to be the place where you store all of your data and you're going to move all of your data out of IBM dbt and SAP and all these places and operate on it and stuff like and I didn't understand why all the people older than me at the time was like, this is never going to happen. This is like a pipe dream marketing scheme and zooming out, it does seem like new technology is only ever like data doesn't move out of old systems and. Even if they try to, it usually takes 10 times longer than people expect. And if you go to r/data engineering, the top three posts, top three of five posts at any given day is always about a horrible migration gone wrong. And so it seems like it is like a smart decision to leave your data in the places that it currently already is able to do stuff with. But that means that if you're going to. Have a true self service system. It can't depend on migrations and it can't depend on moving. And because there's always that one person who is still stuck in this old BI tool, if you can't move everyone across and that person has enough gravity, you're never going to get out of power BI and you're never going to get out of Tableau. And and, that's okay because you have, these assets exist and they're important and people are used to using them and you shouldn't pull them out something that is. Going to help you move forward has to be something that has to, bring you from where you are to there, instead of just stand here and say, like, all right, now, you take on the, you cause team and users and everyone take on the risk of bringing yourself from that system to ours and that feels and that's very much like a philosophy that we've adopted from day one. Mostly because we really didn't want to build some shelfware. Yeah. And that's, that is a thoughtful way of looking at it where, yes the current BI tools, the current database, data lake, what have you, has a certain amount of gravity, meaning it's already operational. There's already data flowing through it. There's already probably internal docs on how to use it. And what you're really doing is like augmenting that which, totally makes sense. Thanks. Absolutely powerful to, to start thinking about how we can better enable the operational teams actually use data without being exposed to the complexity of the underlying data infrastructure. So Ethan Ding. Co founder and CEO of TextQL. Thanks so much for joining us today. It was super insightful and exciting to hear about your product. Where can people follow along with your work? We are, we're TextQL on I think LinkedIn and Twitter. And I think I actually probably post more than the company account because. I feel like nobody actually wants to read a post from a company account. So I'm the Ethan Ding on both LinkedIn and Twitter, and in TextQL.com is the URL . Yeah. That's great. Yeah. So text ql.com. Yep. Ethan's very active on LinkedIn. We'll have links out to all those in the show notes below if you look to the description. Ethan, thanks again for joining us and thank you to the audience for tuning in. Thanks, John for having me. Have a good one. I'm You