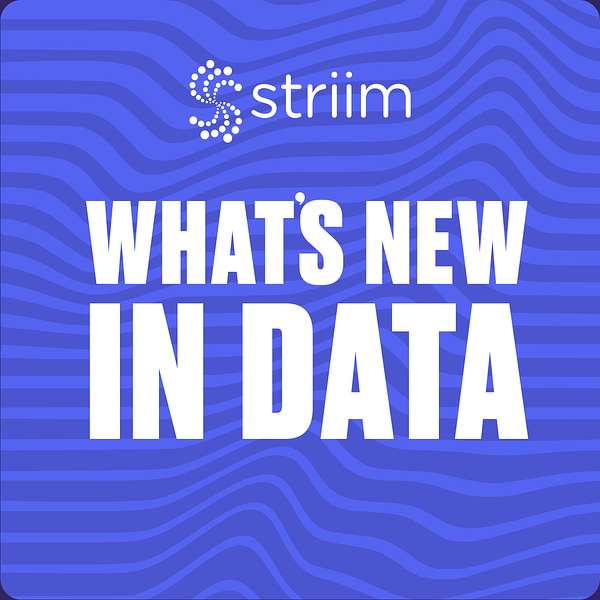
What's New In Data
A podcast by Striim (pronounced 'Stream') that covers the latest trends and news in data, cloud computing, data streaming, and analytics.
What's New In Data
Shifting Data Quality Left, New O'Reilly Book, and Data Contracts with Chad Sanderson and Mark Freeman from Gable
Join us as we catch up with Chad Sanderson and Mark Freeman from Gable, live from Big Data London. Discover Chad's insights from his well-attended talk and why the data scene in London has everyone buzzing. We're diving deep into the concept of shifting data quality left, ensuring upstream data producers are as invested in data governance, privacy, and quality as their downstream counterparts. Chad and Mark also give us a sneak peek into their upcoming O'Reilly book on Data Contracts, complete with the charming Algerian racer lizard as its symbolic mascot.
In this engaging conversation, Chad and Mark offer practical advice for data operators ready to embark on the journey of data contracts. They emphasize the importance of starting small and nurturing a strong cultural initiative to ensure success. Listen as they share strategies on engaging leadership and fostering a collaborative environment, providing a framework not just for implementation but also for securing leadership buy-in. This episode is packed with expert advice and real-world experiences that are a must-listen for anyone in the data field.
John Kutay chimes in with examples of innovative data operators such as George Tedstone deploying Data Contracts at National Grid. Data Contracts and shifting data quality left will certainly be an area that many data teams prioritize as their workloads become increasingly operational.
Download a preview of 'Data Contracts' here.
Learn more about Gable.
Follow Chad Sanderson on LinkedIn.
Follow Mark Freeman on LinkedIn.
What's New In Data is a data thought leadership series hosted by John Kutay who leads data and products at Striim. What's New In Data hosts industry practitioners to discuss latest trends, common patterns for real world data patterns, and analytics success stories.
Hey everybody, we're live here at Big Data London. Super excited that I have Chad Sanderson and Mark Freeman, the team from Gable. Chad, you gave a great talk yesterday that was super well attended. Just line going back to the entrance doors. How are you guys doing? We're doing great. Uh, it's awesome to be in London. I was here last year. I'm for some reason, I think I'm going to be in London more frequently than I usually am. Seems like the data scene here has been pretty awesome. But like you said, talk yesterday was great. Conference is awesome too. And weather's not bad. Oh yeah, amazing weather we're getting in London right now and yeah, I can see why you're going to be back here in London because people are even coming to me and asking, Hey, do you know Chad? Can I can I talk to him? A lot of the data operators here are very interested in the work you're doing, so would love to hear about that. Yeah, yeah, absolutely. So, uh, what Mark and I have been working on for a pretty long time now at Gable is, uh, this idea of shifting data left, and what that means is taking all the principles of data management, which is data governance and, uh, data privacy and data quality and so on and so forth, And starting to share that responsibility, not just having the sole onus of ownership on the downstream teams, but pushing it to the upstream data producers as well. And that's what Gable is all about doing. Um, and that's what we've been really excited to work on and talk about for the past few months. Absolutely. And you're working on an O'Reilly book. I know you just announced the early release there. Tell us about that. So Chad and I are writing the O'Reilly book on data contracts, and it's been, it's been a great journey. I mean, I think any data person kind of see those animal books and to be able to kind of do that ourselves has been kind of an honor for that. But for us, when we're thinking about like, hey, We're talking about data contracts a lot. Chad's talked about it extensively. One of the big questions that all these people ask is, well, that sounds great. Theoretically, how do you do this? Right? And so with the book, our goal is really providing a framework of like, why does this need it? And then what are the components of it? And then through open source tools, how do you actually build this for yourself? And then finally, the next question is like, cool. I know how to build them very bought in. How do I get leadership to get bought in? And the final section of the book is basically how do you build in that leadership buy in? And so really focused on being a very practical book. Beyond just theory. That's excellent. And like you mentioned, the animal books, every single O'Reilly book is have famously has a gray stencil of a animal. So what animal is the data contracts book? So we are the Algerian racer lizard, uh, which is now my favorite animal. Uh, I love that. Yeah, exactly. So unfortunately we didn't get to choose our animal. And that was like, when we first said, Hey, we're writing O'Reilly book. Everyone asks what animal do you, are you going to choose? And we're like, we don't get to choose. We're just, we're just going to say, we don't want that animal, but that's, that's about it. So we're excited. I actually just found out a couple of weeks ago, uh, what animal it was. an exciting moment. Love to hear that the book is represented by the Algerian racing lizard and now it's my favorite animal too. Never knew it existed before this conversation, but just sounds really cool. And apparently it symbolizes data contracts, which is another great concept. So what are some of the recommendations you'd make to the data operators that are looking at implementing data contracts for the first time. Yeah. So the number one advice I have when data leaders or operators start thinking about data contracts is to start small. Contracts are a cultural initiative. Primarily, the technology can make that cultural initiative easier to maintain. Um, but ultimately, like you do have to get in front of people, you have to have a conversation. And what I find really works where I've seen work in other businesses. Is if you run a data platform team or your data engineering group responsible for building out your raw and silver layers in the data warehouse, you can go back to data producers and say, Hey, guys, we will no longer accept data into our data platform unless there's a contract attached to it. And that contract could be something as simple as documentation in a confluence page or an Excel spreadsheet. But you need to have the data producer signed up for ownership somewhere and express. What does this data mean? What should the schema be? What are the semantics of the data? What are the values of the fields that you expect? And so on and so forth. And now you have a record, right? That's a great starting point. And it provides an incentive for the data producer to take ownership, because of course, if they don't, then their data is not made accessible to the broader business. Absolutely. And I just gave a talk with George Tedstone, who leads data platforms at National Grid, uh, which is the largest supplier of renewable energy in the UK. And he even touched on data contracts and the part that they have so far, but it seems like, you know, trying to align it within the analytics team is like a relatively simple place to start. But then once you go to the producers, then it gets very complex. What's your recommendation there? Yeah, I think that aligning data contracts between the data platform leadership and the data engineering leadership and data consumers or analytics engineers is a pretty straightforward task. That's the equivalent of if a software engineer producer said, Hey, I want to take a data contract out with my consumers. Of course, no consumer would say no to that. And that's great. It does build a tremendous amount of trust within the organization. It makes sure that any data that is arriving to that raw layer or whatever the the analytical platform. Uh, wherever, wherever ownership of that layer happens to be is high quality before it passes that quality gate to the consumer. And that's, and that's really great. But the challenge of course, is if indeed from the producer to the platform leaders. The data has changed, there's new data that's added, the schema has updated, the data semantics have been updated, or so on and so forth, it is still up to that data platform team to now figure out where these changes came from, to understand them, so all the same work that the data engineering org was doing, that the data science org was doing, now it falls to the data platform team to do so they can provide that good data to their consumers. So that's sort of, uh, the, the relationship of, uh, uh, sort of, uh, data platform teams, analytics teams. And data producers now the reason why it's so complicated to ensure you have data contracts at the source systems themselves is really just a problem of technical complexity and heterogeneity right if you're putting data contracts within something like a snowflake or dbt that that's usually one technology or two technologies. But your, your source systems could be a wide variety of technologies. You've got many different types of code bases that produce events, many different languages. You might be using segment or amplitude or Google analytics or mixed panel. You have third party data sources. You have tools like Salesforce and HubSpot and ERP systems like SAP. You've got data coming in from FTPs and APIs and your transactional databases. And so you have to apply sort of contracts on all the sources that actually matter. And that's just a bit more of a time consuming process, which is why I recommend the platform teams to start off sort of being the bottleneck and say, look, it doesn't really matter where that data is coming from initially. If it's going to reach us, it requires a contract. Absolutely. And Chad, you're Both of you, Chad and Mark, you're, you're both amazing thought leaders on this topic. You know, on LinkedIn, every time I log in, I, you know, I see a post from you guys that is, you know, in the hundreds of likes and, and it's true that, you know, data operators, whether they're in here in London or back in the States or in Asia, you name it, they're, they're following you guys for advice on this because it is such a complex topic and there's no rules around it. Right. And when analytics was just a matter of pulling reports that the team was looking at once or twice a week, you know, it's all good and fine. If like a schema changes and you fix it before the next model run. But now what we're seeing here at big data London and at all the shows, right. It's all about using data for AI, right. And when you talk about using data for AI, it's suddenly operational. And when it's operational, it's a production system. So that means you can't just have schemas changing willy nilly within the organization. It's They're going to just completely crash production systems. I think what you're talking about is really applying these best principles. that make the most sense for data teams and engineering teams. So how do you bring that all together? Yeah, I think that's a great point. And I think you're exactly right. Once data becomes a product, and it starts serving production utility and making a company money, then all of the same best practices that you see in software engineering, when it comes to ensuring that the code they deploy is high quality, Which is DevOps and unit tests and integration tests and code review. The whole reason we do all of that is to make sure we don't deploy buggy code. And in the analytics world, well, if you deploy buggy code or if you make a change. And it impacts the dashboard. Well, it doesn't really have a production impact or a business facing impact. But in the AI world, it does. And in fact, something that we've heard is that teams that have invested very heavily into generative AI now find a new struggle. And the struggle is, well, we know these LLMs hallucinate. Sometimes they say the wrong thing. But the challenge is figuring out was the data that fed into the model correct and the model was wrong, or was the data wrong and the model made the correct estimation? How do you decide whether an incorrect output was due to wrong data or due to a hallucination if you don't have something in your pipeline essentially going all the way through? Telling you what the data should look like. It's very, very hard to come to a conclusion about that. So, yeah, I absolutely do think that software engineering and data engineering when it comes to managing data products and best practices is going to start converging over time. Data is different from software, so we just need to think about all of the unique elements that data has. And something I'll add was a big shifting moment for me in thinking more about data as a core driver for these workflows. Andrew Ng's, uh, work on data centric AI. Um, back then I was a data scientist really focused on building models or analytics. And reading his work where he's basically calling out the model. Yeah. You can spend a lot of time doing that for a fraction of the time and cost. If you just fix the data, the models outperform all these other different teams. And that was a big click moment for me. I was like, I need to get out data science for me personally and move into data engineering and thinking about the governance aspect of it and all those components. And that was a few years ago. And so now seeing kind of the gen AI movement kind of come ahead and look Chad's talking about, I think that was the right bet to, to place. Absolutely. And it's a reoccurring theme of this conference. And even the talks that I did with the team from Morrison's, which is one of the top grocery retailers and Crump, which is one of the part top parts suppliers here, they all started as data science teams and to actually support the adoption of data and operational use cases, they had to become data engineering teams. And now they're evolving into software engineering teams. Cause they're There's no other way to really deploy this stuff at scale in a way that's, the data is reliable for use cases like AI or even data driven, simple data driven operations, right? So absolutely incredible work you guys are doing. I'm super excited to continue following along. Can people get access to your book now? So the book, the early release chapters of the book are available for download at gable. ai. Just visit the website. It's right there in the banner. And when are we going to be releasing? What's the? Um, so that's still TBD, but sometime next, sometime next year. Okay. Um, we're, we're, we're kind of cranking through the chapters right now, going through like final review of things. So writing a book and starting a company at the same time, not recommended. We were quite ambitious last year. I love it. And honestly, everyone who follows along with you guys loves your story and everything you're working on, because you both have operator backgrounds. You've done it before. It really seems like you're, you're building the product that, you know, you wish you had in that same environment and the high stakes operational use cases ate up. And we're excited for your book to come out. We'll have a link to gable. ai in the show notes and do keep following along with Chad and Mark for their great thought leadership on this topic. And the awesome stuff that Gable is going to release as part of this. Thanks. Chad and Mark, we're both in London here. What are you guys going to do next? Uh, well, we're, well, me and my, uh, my wife are probably going to end up going to Cambridge, I think. So we did Oxford last weekend, saw the campus. Uh, I think Cambridge is up next. She wanted to go to Oxford. I want to go to Cambridge. So we just split the difference. So probably end up doing that this weekend before we head back to the U. S. Oh, maybe you'll run into Martin Klepman and you guys can autograph each other's books. Maybe so. Yeah. Yeah. For, for me, uh, John actually took me to this amazing Indian food restaurant, Dishoom. And I must go back because it's ruined. It's ruined me. It was so good. Yeah. Dishoom ruins Indian food for a lot of people because it's just so good. It sets the bar so high. Uh, definitely recommend it. Um, that was a fun, fun lunch, Mark. Chad and Mark, thanks so much for joining. Thank you for having us. Thank you everyone for tuning in. And we'll wrap up here at Big Data London. Thanks guys. Thank you.