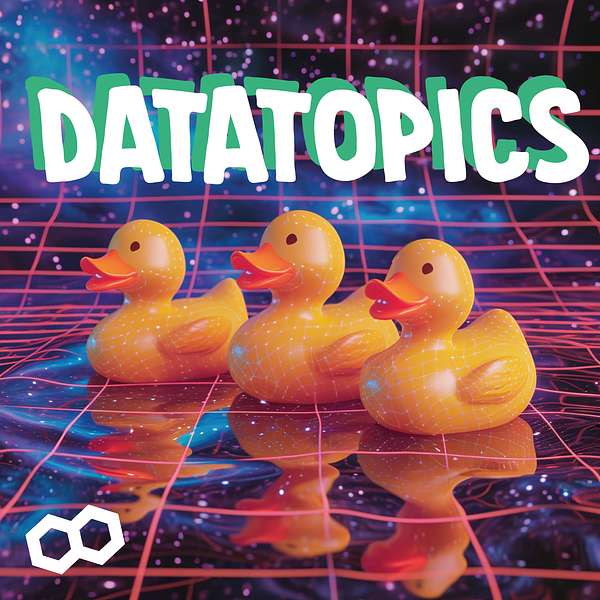
DataTopics Unplugged: All Things Data, AI & Tech
Welcome to the cozy corner of the tech world where ones and zeros mingle with casual chit-chat. Datatopics Unplugged is your go-to spot for relaxed discussions around tech, news, data, and society.
Dive into conversations that should flow as smoothly as your morning coffee (but don't), where industry insights meet laid-back banter. Whether you're a data aficionado or just someone curious about the digital age, pull up a chair, relax, and let's get into the heart of data, unplugged style!
DataTopics Unplugged: All Things Data, AI & Tech
#39 Microsoft, Musk, and Extremely Fast Image Gen using SDXL Lightning
Welcome to the cozy corner of the tech world where ones and zeros mingle with casual chit-chat. Datatopics Unplugged is your go-to spot for relaxed discussions around tech, news, data, and society.
Dive into conversations that should flow as smoothly as your morning coffee (but don't), where industry insights meet laid-back banter. Whether you're a data aficionado or just someone curious about the digital age, pull up a chair, relax, and let's get into the heart of data, unplugged style!
In episode #39, titled "Microsoft, Musk, and Extremely Fast Image Gen using SDXL Lightning," we take a spin through the latest tech tremors and thought nuggets. Oh, and don't forget to give a warm welcome to Alex, our enthusiastic intern stepping into the podcast spotlight!
- Elon Musk's Legal Drama: Musk takes on OpenAI and Sam Altman over a philosophical fallout.
- Microsoft's Smart Bet: Investing a cool $16M in Mistral AI sparks curiosity.
- SDXL Lightning Unveiled: A breakthrough in image generation that might just redefine speed.
- GPT-Script Evolution: The rise of GPT-Script signals a coding renaissance.
- pkgx Drama: Unwrapping the GenAI layer over OSS packages stirs the pot.
- Database Dilemma: Questioning the database abundance echoes a common tech gripe.
- Sticky Table Headings: A plea to make web table headings sticky finds its voice.
Intro music courtesy of fesliyanstudios.com.
Hello everyone, welcome to Data Topics, unplugged, casual corner of the web where we discuss what's doing data every week, from GPT to lawsuits, anything goes. Today is the 1st of March of 2024. My name is Morillo and I'll be your host for today, and I'm joined by Bart. Hi and in the back scenes we have another special guest. First tidbit here today we have Alex Alex.
Speaker 2:Ah, there we go.
Speaker 1:Is she muted or no?
Speaker 2:There we go. Hi, I'm Alex. I'm the new intern at Data Topics.
Speaker 1:Yes, she'll be helping us here, so we'll be seeing her.
Speaker 2:She's hopeful If the quality skyrockets you know that it's all because of Alex, the Alex effect, the Alex effect. Yeah, we'll call it the Alex effect, the Alex effect indeed Cool, very happy that you're joining us.
Speaker 1:Indeed, indeed indeed.
Speaker 2:I'm happy to join.
Speaker 1:All right, and we have also some other tidbits right, we have some other exciting news. Yes, do you want to share that part?
Speaker 2:I assume that you're talking about the introduction. Yes, yes, I am that's why we didn't start the intro jingle.
Speaker 1:The intro jingle. Yeah, we didn't start the podcast in the usual way because we have this little surprise for the listeners, viewers, maybe as you play it, then you can talk about it a bit. I'm a bit shy about it. I'll do it. I'll do it. Oh, you want to do it together? Let's do it together.
Speaker 2:You're going to play it. This is awkward.
Speaker 1:You have taste in a way that's meaningful to software people.
Speaker 2:Hello, I'm Bill Gates. I would recommend TypeScript. Yeah, it writes a lot of code for me and usually it's slightly wrong. I'm reminded that there's a rust here. What rust, congressman? Iphone is made by a different company and so you know you will not learn rust while you're trying to do it. Well, I'm sorry, guys, I don't know what's going on.
Speaker 1:Thank you for the opportunity to speak to you today about large neural networks. It's really an honor to be here Rust Data topic.
Speaker 2:Welcome to the data topic. Welcome to the data topics podcast.
Speaker 1:Hello, hello. So that's how it's going to be from now on. Yeah, cool.
Speaker 2:I like it.
Speaker 1:I like it. So what? Maybe? Alex? What do you think about it?
Speaker 2:Yeah, I really like it, oh wait, we need to think that we unmute you. Yeah, go for it. Yeah, I really like it. I think it sounds cool.
Speaker 1:All right, yeah, that was the.
Speaker 2:This is the completely unbiased answer. Yes, yes, first day answer. I love it actually.
Speaker 1:I would have done better myself. Yeah, no, it's cool, but maybe the backstory there is. I was on holidays last week, as I mentioned, and Bart apparently felt really lonely. He had too much time on his hands.
Speaker 2:The backstory is that on Friday evening I had a bit of time and this crawled into my head, so the concept is basically like there is a jingle, but all of the people that you hear talking are famous people. Yes, In the software and or data world.
Speaker 1:So you heard, so maybe some Bill Gates, bill Gates yeah.
Speaker 2:Or.
Speaker 1:There are some highlights there. No, Like Steve Jobs with I don't know what's happening.
Speaker 2:This is not working. He's like he's doing a demo somewhere.
Speaker 1:Yeah. And then like on the stage right. Yeah, it feels. Yeah, a demo effect, this became, I think, very popular.
Speaker 2:No, because I think he got really, really pissed off with the engineers, because yeah, not sure if it's that one, but Indeed, yeah, and the one with, I would recommend, typescript. Do you know who it is?
Speaker 1:I think, you mentioned to me, was it a Linus. No.
Speaker 2:He's also in there who, gito van Rossem.
Speaker 1:And that, by the way, the people that don't know who that is. That's the creator of Python. Yes, so interesting that you took that.
Speaker 2:I really purposefully took it out of context.
Speaker 1:Okay, okay, Cool. And Linus, the guy from Linux who.
Speaker 2:Well, he's actually not him. But the first thing, what is this one Like you?
Speaker 1:have taste. Yeah, yeah, yeah.
Speaker 2:In a sense it's meaningful to software people. So it's a bit Like you have to and that's an interview starting a question. Too light a struggle, yeah, yeah.
Speaker 1:It's like you look really blind. No, you look really good for a blind person. He's like, okay, Cool, Cool, cool, cool. To be fully transparent with whoever is listening to, whoever is watching Today would be a bit of a bar show. I was on holidays last week. I was skiing on the slopes of Romania Really cool as well. How was your this week? This past week part? Only missed you. Huh, you only missed me.
Speaker 2:Yeah, I had a countdown like so many more days, really, totally me looking back. I'm sorry, I'm sorry. Every day you're like. I hope he's thinking about me.
Speaker 1:I wasn't, but sorry, okay, but yeah, so actually quite a lot of stuff Happen. If you, maybe we can start with very fresh, fresh news, feel like, actually, do we have what's this? No, no, no, no, no. Very fresh news Elon Musk sues OpenAI and sell Meltman over Betrayal of nonprofit AI mission. So actually this is very see, march 1st, that's today's date 1130 AM. So very, very, I think it's three-hours old news.
Speaker 2:Yeah, very, very hot out of the Out of the Out of the oven.
Speaker 1:so you heard here first, or maybe or second, or on TechRange.
Speaker 2:Or on TechRange. Yeah, but well, I glanced over it.
Speaker 1:Bart, I don't know if you have more info, but I think it's the Betrayal here that they're referring is because OpenAI. Actually Elon Musk was one of the founders of OpenAI. I'm not mistaken.
Speaker 2:Elon Musk was in the founding team and I think what it is about but again, like I also glanced over, this is that and the statue that I'm talking about, the statutory documents of the founding of OpenAI. It basically says that it's a nonprofit organization, but what it comes down to today, that's like it's a complex setup. There's a non-profit component but, like the main mission is a for-profit one.
Speaker 1:Yeah, actually I heard that on the whole OpenAI drama. Part of it was because there was the You're referring to the board.
Speaker 2:Yeah, firing yeah.
Speaker 1:Yeah, so for a refresher for people that were not in the know, sam Altman, he's the CEO. He was the face of OpenAI. He was very vocal about it. He was like going to Well, he was basically the face of OpenAI and the board fired him. Yeah, still is right, but the board fired him on Friday and then a lot of people from OpenAI were starting to resign. In how do you say?
Speaker 1:And he was fired by the board, by the board yeah, and then basically a lot of people turned to leave in solidarity I guess that's the word I'm looking for and then by Monday he already had his job back and a lot of people from the board were fired or replaced or I don't know. Promoted Promoted to other companies, new hobbies, but yeah, I remember like there was a bit of a discussion like how OpenAI there's the for-profit and not-for-profit, and one was taken over the other a bit, because, well, profits are always in people's minds and it's a bit of speculation why this whole thing happened, why they fired him. But yeah, and I think Elon Musk has been very vocal for a long time, that OpenAI I think there are even tweets from him or X's, or actually, how do you say tweets now that it's X?
Speaker 2:X's Cheats.
Speaker 1:Cheats. You just made that up right now. X for the eat behind it Cheat, cheat. Okay, cheats, I guess.
Speaker 2:That's what we're confused with.
Speaker 1:Yeah, with other stuff. The OpenAI should be open and when he founded it it was with the openness like open source and stuff, and now it's fully for-profit. It's part of Microsoft, right? So I think this is a bit of the document there. So let's see. Let's see how it plays out, maybe. What do you think is going to happen, bart? Do you think there's any? I mean, this is all the news, because it's Elon Musk, right?
Speaker 2:Well, yeah, exactly.
Speaker 1:Do you think this is going to go anywhere, or do you think?
Speaker 2:I don't know enough about US legal system to really reason about this specific thing.
Speaker 1:Yeah, I'm a bit. I mean, what can you do?
Speaker 2:Well, I think what a factor is that Elon Musk probably put capital in it and that means that at the very beginning and I think he still is a shareholder, that's maybe it was a we're meaning that you partially owned the company. Maybe it was not involved enough for them. So it's going to be the important part in the story.
Speaker 1:Yeah, let's see. Let's see it still very early, like we mentioned, but I think it's caught my attention as well. And talking about Microsoft, right, because OpenAI is part of Microsoft, microsoft has been, it's not. No, my God.
Speaker 2:My God, my God, my God. Openai is not part of Microsoft. But there was Microsoft has an exclusive license to OpenAI, but they have influence too. No, I think through that. They also have influence in the era.
Speaker 1:Yeah, because I remember there was something, I think, maybe what I'm also confusing, because during the whole drama, sam Altman, he was going to work for Microsoft, like he moved, like he was going to get a role in Microsoft or something, because I also saw here somewhere the Microsoft Satya. I don't know, I'll just stop talking.
Speaker 2:In any case, he's too much here and he actually says that Musk donated. I'm not sure if he is a shareholder. It says he donated to the nonprofit.
Speaker 1:But, In any case, Microsoft Microsoft has also well, I think, ever since they have this partnership with OpenAI. Azure has the OpenAI exclusivity thing Right. I think they've been taking leaps forward on the AI race, and they actually made another leap. I saw you added this not that long ago as well that Microsoft made a 16 million investment in Mistro AI. Yeah, so maybe Mistro AI refresher. What is Mistro AI Mistro?
Speaker 2:AI is a European competitor to OpenAI. It's the easiest way to explain it. Based in Paris, they are very similar offering models behind an API. Different Zeta models are also open sourced. We've discussed this earlier. They very recently also launched La Platform.
Speaker 1:Where is Kevin La Platform Having a minute?
Speaker 2:It's actually the API thing, but they also launched it in the upper right. What does it say? How do you pronounce it? La La-cha Voila. It's functionality very similar to what OpenAI has with Chatchapiti, so it's very comparable, right.
Speaker 1:Yeah, but it's interesting, right, Like well, Microsoft is just eating the.
Speaker 2:I think it's interesting that Microsoft now actually invests in them because they have this exclusive license with OpenAI and I think OpenAI is still very much at the forefront of this. I don't think Mistro AI don't have. Performance is a competitor.
Speaker 1:Is there a competitor to OpenAI today?
Speaker 2:If we just talk purely about performance. I don't truly think so. Yeah right, like every time we think something comes close, they announce a new version.
Speaker 1:Yeah, I mean, sometimes I feel like there's a lot of hype and people talk about it, but then you try and then you're like, yeah, yeah.
Speaker 2:But the interesting thing is, like, what is the rationale of Microsoft to do this? Because towards their OpenAI partnership license that they have for the OpenAI, like this also comes can come across a bit weird, right, so there must be a strategy behind it and I I think potentially like it's hatching batch what if? Ms Roy is indeed the next big thing, but I think also a very important one is that there's already some antitrust rumors going about around the Microsoft OpenAI collaboration.
Speaker 1:So antitrust rumors meaning like?
Speaker 2:that they basically have a monopoly position on this. Because they have so strict eyes, they're exclusive. Then at the same time, they're not really competitors out there that make it a fair market.
Speaker 1:I see. So basically you're saying that there is. I'm going to try to repeat what you said. If I understand, openai is so ahead that, like Mr, is not really a competitor.
Speaker 2:so Microsoft investing them is not really a conflict, because no, no, I think the trust agencies, I think in Europe, maybe also in the States, they have their visor, settle Microsoft and open AI, saying that the position that they're taking and the exclusive partnership that they have is not conducive to a fair market. And you can, one can make the argument that Microsoft investing in Mr AI, that that puts Microsoft in a position, we're saying, but it's not only exclusive with OpenAI, see, we also invested in another.
Speaker 1:But you guys say we play nice, we give money to everyone, so there's competitors.
Speaker 2:But potentially I'm very much, but like if they have an influence on all the big AI players.
Speaker 1:Is that also not you know? Like is this? Because I guess in my head is like, if Microsoft has influence over all of them, that's more damaging to the market and you know.
Speaker 2:That's another way to look at it, but I think the anti-trust discussions that are going on now is really about the exclusive collaboration between Microsoft and OpenAI being something that is very hard for anyone else to replicate, making it unfair, and simply by now investing in Mr AI, they can say it's not exclusive.
Speaker 1:Yeah, I mean in 16 million the amount there. Can we put this in perspective somehow, like how much money they invest on other things?
Speaker 2:is this I think it's for Microsoft. It's like they forgot to put the lights out at the end of the day.
Speaker 1:They just say oh, whoops, put a little bit more, yeah, so maybe it could just be like in Brazil we have a saying like Prangles V, just for just to show.
Speaker 2:And so maybe another important factor. So we have, I think, over the years, a lot of legislation in Europe that's under which and I don't think there's ever a very clear prohibition that to use, let's say, us based services, there's never a clear prohibition, but it is often easier to go for a European provider. So that might also be in Microsoft's consideration, given that they have a very big footprint in the cloud world in Europe. Hmm, potentially when they can maybe more easily position Mistral as a service behind their Microsoft cloud or Azure cloud than OpenAI.
Speaker 1:Hmm, interesting, yeah, but yeah, to be seen, to be seen, to be seen. It feels like a movie right, like the character does something. We're not pretty sure why, but it's like everyone's kind of like. Is it because of this, is it because of that?
Speaker 2:Clearly, I have to feel that this has been the last year with OpenAI. And then every other week, elon pops his head up.
Speaker 1:So make sure, like, have you forgotten about me? Cool, and so far we're talking about OpenAI. Well, actually, mistral AI is just text, right? There's no image generation at all.
Speaker 2:It's a good question. I want to say yes, the only thing I tested was totally text based.
Speaker 1:I don't think so.
Speaker 2:Maybe they're doing some R&D around it.
Speaker 1:Yeah, but there's nothing available right Like models, mistral, small, large, embedded, yeah, text Additionally. I think it's just text or state of the art technology. It's a bit weird to say state of the art technology, but you know, like you still see GPT-4 up ahead, but yeah, okay. And why I ask? Is because actually GPT-4. Well, gpt-4 is has is multimodal as well. Has also we can generate images actually just lays over to another model.
Speaker 2:Yeah.
Speaker 1:Right, so maybe I guess it's not really GPT-4, but it's through the same. You still on the chat, the same chat platform. Chat GPT I think you can call multimodal. And why am I asking this? And maybe from your experience generating images, does it take a long time?
Speaker 2:So I tend to use to default to mid-journey and I want to say it takes roughly 30-ish seconds, but I'm really a finger in the air, something like that, yeah.
Speaker 1:Okay. Okay, because I'm saying I hear, I mean I hear you heard and I just put it there and I'm bringing it up about lightning fast image generation. Blazing fast blazing the fast, which nowadays means Rust. You know maybe a site story. I was doing a talk at the university and then like all my interest light, there is always a Python, rust and stuff. And then I was like yeah, I'm also curious about Rust. And then just hear someone go Like at the university and I was like man, the Rust fanboys started early.
Speaker 2:How many people were there that stood up and cheered?
Speaker 1:Yeah, I think there was like two or three. I mean one was more emphatic but there was like 300 students, 320 students or something. So it's like, yeah, 1%, but still like the university. I was surprised too.
Speaker 2:Did they ask you?
Speaker 1:autograph. You know, I was like man embarrassing, I was like I'm just just a regular guy, you know. No, but I thought it was funny that they start. They start early, they start young. But what is this lightning?
Speaker 2:fast image generation it is like you say, it is very fast. Maybe it's more interesting if you use the other link. It's like really a demo.
Speaker 1:This one. Yes, this is a cute yeah.
Speaker 2:You try to generate yourself, put a problem in there. So we're for the people that are listening.
Speaker 1:We're looking at that image that has a Brazilian, half Brazilian, half Japanese guy. It's a be really good Enjoy ice cream.
Speaker 2:Let's go for that Half Brazilian bearded guy.
Speaker 1:Japanese bearded. But you see, like actually this thing is so fast.
Speaker 2:It generates it while you type.
Speaker 1:Yeah, we see the image building actually. So I'm not really, I don't want to be held liable for that likes ice cream and is in his end of his twenties. Why you just didn't be that specific. You just said in his twenties.
Speaker 2:Okay, it's getting young, getting young, but it's crazy how fast this is no-transcript.
Speaker 1:You think?
Speaker 2:doesn't wear glasses and has perfect.
Speaker 1:I said.
Speaker 2:It's interesting that a lot of it stays stable what the but?
Speaker 1:you see, I feel like they put the glasses because I said Japanese and has perfect, but remove and just be more specific and does not have, does not work off, I think that could very well be here Is it.
Speaker 2:is that a thing in Japan?
Speaker 1:I don't know, but I feel like you don't be great. I don't know, but I actually like these two have glasses, huh. Even if I write it, oh well, but if I remove it, it's the same, huh.
Speaker 2:Yeah, I think this is the only guy that comes close to you.
Speaker 2:Still, I think, is the do a minus, maybe it's a some I Really a minus sign. Anyway, what is happening? So Marilla is typing the prompt and, like as he writes it, new versions are being generated, which is crazy, crazy, crazy fast. Actually. The inference time says it says there are 223 milliseconds for the last image coming from, like I said, a major, like half a minute. So there's a minute. It's also, and it's still quite good, even though we can't get a glassless guy.
Speaker 1:But yeah, yeah, it's still quite good. It's not realistic, right.
Speaker 2:It's not really skin. Can you try it? A photo, realistic image of a cute bunny, and it's actually quite good. It's actually pretty good. Yeah, it's cute bunny.
Speaker 1:Let's see. Yeah, it's nice, it's a cute Okay.
Speaker 2:So, and this is super, super, super fast, and this is based on SDX as the X this one, sdx lightning, as the. Xl lightning, which is, as I understand so, stability AI. It's based on stability model, stability I. I launched a very fast model already in December, somewhere it was called stable the future now as the XL, not.
Speaker 2:indeed, this is based on the default base model, but it's stability. I released very fast model already in the in November, which was called as the XL turbo, I'm not mistaken, and this is like again one step further and this was released, I think, somewhere last week. Yeah, maybe we'll link the paper and show notes. Also, the demo app demo app you can very easily fork it wonder where you're showing now is hosted on for sale.
Speaker 1:Yeah, indeed, indeed, indeed, so Really cool. Actually we're cells like they have a free tier.
Speaker 2:No, different sellers of free tier. So for people that like looking for a side hobby project, for people that are cheap and they can just we're my Dutch people.
Speaker 1:I can say it, because I can say it but you should, you should always say that after you know, after you make a joke. Yeah that's fine. Yeah, I was gonna make the comments like maybe, maybe a shit.
Speaker 2:Yeah yeah, it's not cool to see cool to see this getting in that that fast, like a really built up, like it creates another Realm of possibilities, right.
Speaker 1:And I think actually makes sense, right like up until now We've seen this JNA eyes, whether it's text or image, that they really just trying to push to see how, how realistic, how good it can be, but now it's like taking forever. So now they're pushing to see how fast we can make you right, which I guess is it's almost like a research cycle, right, like you have first trying to make something Valuable and then you try to make it useful. Right, like usable, I guess not useful, but it's really cool, very, really cool, but, like I mentioned, it's turbo, but I also saw distillation.
Speaker 2:So the stability. I released turbo, which is similar Each model, the fast image generation in November, in December of last year, and this is a new version, lightning version, but it's like you said you were looking at the description. I think it's not bill, not Based on turbo, but they rebuilt it. Yeah, it's actually a paper on how they are rebuild it.
Speaker 1:Yeah, but then base so and how they make it fast. As I'm looking here like he's like distillation or something which I think, if I remember correctly, is like you have a model and you have. You're trying to train another model. Okay, to mimic the bigger one, it's like a smaller one, right?
Speaker 1:based on that and I think from what I remember I may be butchering this, I'm just going from memory here the downside is that the smaller model can only be as good as the big model, so the performance is kept One way, but I think it's a pretty, pretty, pretty cool.
Speaker 2:Maybe, when we're on the topic of you butchering stuff, we have a correction to make as well. Go for it.
Speaker 2:One of the One of our previous episodes we were talking about warp and I was actually kind of bashing warp for sinking data Across your devices, and that's how I understood the functionality, because you like warp is so warp is a terminal, a relatively new terminal built in Rust and If you have a button says rust with, like some you know, yeah, you can log in and I assumed it was to share your history and I was bashing like, but this is sensitive history, like I don't want this to be someone, a server, but apparently it's not to to save your history. So I think it only like uses your preference. You can think you're I've really looked at what I've done, what it does think them while you need to log in, but it's probably like you're you're setting Sure. Preference is like the House called. My course I think they call it like many scripts that you can easily run.
Speaker 1:I actually haven't used that. I know they have extensions and whatnot and you can so the corrections made yes, we actually got this correction from Friend of the show. Sam actually will be here in a couple of weeks, yeah, talking about you know.
Speaker 1:I think it's fabric, fabric, so he's actually the. Since the world won't spoil it, I'll wait for the episode. Very knowledgeable guy, really excited to have him here next week and he did. Correction made. What do we have next? I maybe also related to the last topic Fast image generation. Let's see if I can find that. Actually I didn't put this, so Allow me a few moments to open this up again. Turbo art really fast image generation.
Speaker 2:Hey, I think that is what that one is actually built on the SDXL turbo from stability. I itself that's what. It's a bit older. Well, the model they're using is a bit older.
Speaker 1:Yes. So what is turbo art? Before we go further, I Saw that means an app, very simple app. Yeah, actually that basically you have original image and then you can put like there's the transformed image. So maybe just to Try to paint a picture with words here, this one is like a landscape, looks something some could be in Switzerland, I guess, like mountains, green, and then you can actually put a prompt here and, for example, for loving. You see it transforms the image on the right and then you have see some, some like Does it also keep?
Speaker 2:does it keep generating?
Speaker 1:Yeah.
Speaker 2:Try the half Brazilian, half Japanese. It's even faster, like if you see it like this.
Speaker 1:It's really really fast, but it doesn't time right because there's also the time from the UI as small or as well, I do what this is. We are here.
Speaker 2:It's a smaller image.
Speaker 1:I guess, I guess, but it's really cool. So if I see, yeah, yeah, image generation is powered by stability SD XL turbo, yeah, so we can actually go here and see the blog post that they mentioned.
Speaker 2:but really really cool and actually, but can we try it if it's also biased? What's what is it half Brazilian, half Japanese. Guy In his 20s that likes ice cream.
Speaker 1:Oh, it really like takes a combination of the image and the prompts.
Speaker 2:It's harder to Like how they put like a nice cream thing, yeah, but maybe we can change this image. This is let's take the phone because I think this is difficult to follow for the listeners. Yeah, but we'll link it as well.
Speaker 1:This, this application, really cool, but I still feel like every like I have this back and forth because, like you have these, it's a probabilistic like Mono right, and a lot of the times it kind of plays into stereotypes, yeah and, and it's like, oh, it's racist. But then it's like, if there's you discuss? This right. It's not like it happened last week, gemini exactly.
Speaker 2:But then I I go kind of back forth a bit, you know like yeah, it's it's corn cold racist.
Speaker 1:But if there is a statistical Correlation there, yeah, you know, I think it's a little bit more Statistical correlation there.
Speaker 2:Yeah, you know like we need to explain a bit what happened with Gemini.
Speaker 1:Go for it.
Speaker 2:So I want to say last week Right, it was be week before even Gemini's image generations Features were a bit it's a bit of a bust around because it didn't really represent history very well, right, and to such an extent that, and basically didn't show any white males, more or less came out of it like that is a summary right, like if you, if you generated something like a historic scene Soldier in World War two, it would be Probably be a very Asian looking and there was a lot of flak because of this, like not correctly representing history. I think the flak was a bit a bit, a bit exaggerated, to be honest.
Speaker 2:I think the flak was also what I'm really Was. Also on X yeah, x is maybe also a bit a bit extreme on these things, but if we Go back one year, two years, maybe we had it the other way around. I've heard very, very often like if you generate an image of a CEO, it's always a white male, right and I think this is this is what happened.
Speaker 2:I like the that Google tried to in their prompt processing or wherever like, add in some guardrails so that you remove a bit of the Inherent bias that is there in society else to some extent. Yeah and that you try to be more aligned with the norms and values that they, that they, hold high. That that's. This was. Took it one step further too much. But I don't. I think it was very much with good intentions.
Speaker 1:Yeah, it's like a walk and it's just a bug.
Speaker 2:in the end it's a bug that is woke to an AI. It's a walk, yeah.
Speaker 1:Yeah, indeed, I think it's like it's a line as well. Right, but To be seen. But every. I was also thinking about that now, because every time I put Brazilian on the other one, it was like women and this one is like sureless guy, which is like you know, is this who I am? You know, like, is this this, is this Brazilians?
Speaker 2:Well, I did go on a screen skater of magazine last year.
Speaker 1:But just wanted to give some love to this, this project here. Also another thing I heard, so actually haven't checked this Life. If you look at the actual repo that created this, it's very simple. So there's the front end right, which is not the actual code that generates the image. There's one file here, turbo art, not pie, and you see here that it's 139, 139 lines of code. That's it. That's the whole app, the image generation. So really, really, really cool. It actually uses modal, which is a really cool framework that has GPUs and you can actually Don't need no credit card required, you know, just sign up. This is the back end, right.
Speaker 2:What you're talking about, the lines of code.
Speaker 1:So this is actually the, the back end, the image generation part, right? So actually they use the modal stuff here. So I just wanted to give some love as well to modal and modal labs. So basically you can in the code for people that are listening you can kind of specify a Docker image with Python code, kind of like kind of Python SDK. You can specify the image, the dependencies, the Docker image, etc. Etc. So you have to import some things in the function and then they send it over to GPUs that you have. You sign up using github and you don't have to put credit card to give you $30 for free every month. So for students, actually I can. This week, when I was in the university as well, people were asking me what can you do to prepare better for the workforce, and I said I'll doing these projects. It's really cool and you have a lot of, there's a lot of things free, right. Then you can.
Speaker 2:You can give it a try and modal is basically like a remote execution of your GPU accelerated.
Speaker 1:Yes, yes. So if you know, like if you're familiar with lab the functions on AWS, right? So, it's like serverless functions, and for people that don't know what I'm saying when I say these things, you can think of like there's a computer with a GPU, so very powerful computer, running somewhere else that you don't know where it is, and then what you can do is basically tell that computer to run your code and then you only get charged for the time that that computer is running your code. So really really cool things.
Speaker 2:So just again, just yeah, and I'm love here. I think Slightly more well-known alternative is replicate More or less is the same thing, I think, the position themselves a little bit differently, as as Putting the model very visible behind an API. Here they're putting the model this one, yeah, but you're in the docs now, yeah. So here they put the model really behind a very transparent API. In modal they also do that, but they wrap it in a Python decorator so it's more easy for Python also to go.
Speaker 1:But they have also like in the modal documentation. So modal, I have tried in the past.
Speaker 2:Yeah, you have multiple approaches, but like, if you like, this is very clearly like they focus on the API approach. If you go to a model's website. Like they focus on Python users.
Speaker 1:Yeah, they also have a lot of tutorials, really cool, Like for people that know Discord, you know how to build a Discord bot. They can do this. So really really cool, Really cool, Nice things. And while we're on the GPT, GPT, JNAI, maybe we can segue into GPT script. Tell me GPT script basically it's from. Their tagline here is develop LLM apps in natural language. So from what I understand, maybe for people that are not super familiar, when I say natural language, why you know the ideas that you have programming languages like Python, Rust type script, and then you have natural languages like Portuguese, English, Dutch, French, etc. So natural, when I say develop LLM apps in natural language is basically develop apps just talking to it as you would talk to a person. That's the idea. So I don't know. So GPT script, it's kind of like a programming language, I guess. Yeah, GPT script is a new scripting language to automate interaction with large language models, namely OpenAI. So I guess maybe they'll expand it later.
Speaker 1:So you have a dot, I think, a dot GPT extension, and then there is like I thought GPT filed basically right that GPT filed indeed, and then there I guess you have a you can run like GPT script with this, and then in that file you have it kind of feels like YAML-ish. You know, you have some keys and some stuff, and I think that's what they're trying to standardize.
Speaker 2:Yeah, you can. You can specify in that file you like what kind of tools do you want to use. And tools are basically also mini prompts that you can and you can probably also alter that programmatically, but by default they're, I think they're mini prompts and in your natural language you can refer to these tools so that the model, probably also this context, gets injected and then yes, Actually I'm seeing here some things.
Speaker 1:Some tools can be improved by invoking a program instead of a natural language prompt. So I guess here they have an example that part of it is just natural language and part of it is Python. Actually, this looks really cool.
Speaker 2:What do you think of this? You find it very cool.
Speaker 1:Well, I think it's. I think it's cool in the sense that, like how you can mix and match things. Yeah Right, so it's natural language, but then it's a programming, scripting language.
Speaker 2:I think this is a good example that explains why developers will still be around a few years from now. Like everybody's saying, like oh, we don't, we don't need developers anymore. But like I generate code with open AI, with chat, gpt, with co-pilot, and like generating code through natural language, like it's a bit of a, like it's a lot of text you need to write, like I read a prompt probably not going to be fine, rewrite it a little bit. Rewrite it a little bit and you tend to then go a bit back to more keywords.
Speaker 2:You know these keywords work to make it shorter, to not write a whole page of instructions.
Speaker 1:It's kind of like Google search right, Like you just put Brazilian restaurant.
Speaker 2:Yeah, yeah, exactly, exactly. So you have keywords and short descriptions and tools like you have. You make it agpt script and you're programming again, right yeah?
Speaker 1:that's true.
Speaker 2:But a little bit more with natural language.
Speaker 1:True, that's very true, but like and also I thought it was like funny, kind of funny, I guess is like we used to have Python gets compiled to barcode and then gets interpreted, you know. Or you have program languages that go to machine code, you know, and now you have natural language that may be transposed to like programming and then programming goes to machine language.
Speaker 1:You know, because I mean, I don't know exactly how it works in the back right, but that's my intuition, right they probably have. They say they send this to somehow to chagpt with prompts and then that will create some code.
Speaker 2:And that code will run on the machine.
Speaker 1:Exactly.
Speaker 2:What will be the next phase? Like we will, we just use a camera. We just like we will use gestures that get translated to natural language, that natural language to code Exactly.
Speaker 1:Yeah, that's the new, that's the new thing. No, the program will run before you think of it. Oh well, that's the that's. That's what we walk, that's what we're going towards. It will be cool. Yeah, Actually has 1.6, 1.6 thousand stars, Pretty, pretty popular. The only thing I was a bit disappointed is that if you go on the repo they have a link to a, to a webpage, but if you open it, it just it goes back to the repo. So they redirected back. So there's no real documentation.
Speaker 2:But it's interesting to see these tools popping up and I think everybody can from people that that uses a lot in their coding workflow, like everybody will say, like it's would be handy to have a bit more structure and I think this is the first iteration too.
Speaker 1:But I'm wondering like again, I think it's cool, I look at it and it's like, oh, that's, that's entertaining. But then I I feel like I'll have a hard time looking at a problem and be like, oh yeah, I should use GPT script for this.
Speaker 2:Yeah, I've definitely will always use it for very small things, right.
Speaker 1:Not like, like. Give me an example. Like what would you use GPT script for today? Cause I cannot think of an example yet.
Speaker 2:Um, I can. So maybe as an alternative to uh, what's it called your own custom GPTs and chat GPT Like the the store. So I use custom GPT to generate the description. So we have, we have notes. Based on those notes, we generated the description of the podcast. Using this I could make like a very minimal CLI and just drop in the notes in my terminal terminal, for example.
Speaker 1:True, but I guess. But like would you just use chat GPT too?
Speaker 2:But the terminal is cooler.
Speaker 1:Yeah, we had the whole twoies episode right. Yeah, yeah, yeah, I think uh again. Maybe I'm just liking creativity here, but uh, to me is like, if I'm going for natural language, I probably don't want structure, I probably just want to blob some vomit, some words there and then just like do something.
Speaker 2:Oh yeah, that's also true. Yeah, the nice thing is that you don't need to think about natural language Like I can like. Instead of a sentence, I use like five of the words and it still knows what I want.
Speaker 1:But that you can do that too Well, yeah, that's what I mean I, I, I, I, I, I, I, I, I, I, I, I, I, I, I, I, I, I, I, I.
Speaker 2:I, I, I, I, I, I, I, I, I, I, I, I, I hate it.
Speaker 1:And I never really let myself get to just think about it on my own.
Speaker 2:It's also Video Game in, and, of course, użyji is a hell of a place东ay, let's we're free to lay in.
Speaker 1:Fun bullshit is like breeze and running to work around not last week, and then maybe Actually we had some promises before now. I forgot about it, yeah it's been over a week, right, all right, all right. What else we got? What else we got? Oh, I see here. Maybe we should go for this. One More on the G no PKGX.
Speaker 2:Oh yeah, that was a bit of drama.
Speaker 1:GNAI sauce on top of OSS package descriptions. Tell me about this drama part. You like drama? Huh. It's like drama in open source. It's like a great weekend for you.
Speaker 2:I think the only right, the only fun news is drama news.
Speaker 1:Okay.
Speaker 2:All right.
Speaker 1:Well, I think, yeah, sure, Package X is. I actually didn't know that. I confess.
Speaker 2:I feel a bit ashamed, but I didn't know it. So package X is NPX for everything else. So NPX comes from the Node stuff yeah, from NPM but NP executable typically to run Node stuff as a command line. So it's like kind of like pipX for Node, exactly. And package X is for everything else. So you can run Python stuff, you can run whatever stuff. And there's a long list of packages that are available for you. You can very easily install it with brew. There's, for example look at the rough CLI's available to it.
Speaker 2:You can install it, these type of things.
Speaker 1:So basically, it's like they're basically trying to put an end to the Should I use NPX, should I use pipX, should I use RubyX, whatever? Yeah, exactly.
Speaker 2:And I'm filling in a little blank here and what I'm going to say, but I think they were challenged because you see it on the website. So now, if you go to the package list and you open one, there's very little information about what the package does. Let's see that's right rough. Yeah, rough is a good one, very little information All right.
Speaker 1:So basically, for people listening, there's a. I guess it should be the logo, but there's no logo. Then there is just like how to install it, I guess, so just the shell. Then it says programs. Rough, I guess, is the name. So I should know this no companions, no dependencies, and then just versions, and there's no more descriptions.
Speaker 2:Which is challenging. The only thing, what they want to do is also challenging to do this in a uniform way, because it's not just Python stuff that they do Like. If you could say this is only an overview of Python packages, I can say I'm going to get everything off of PyPI. I'm going to scrape PyPI and use an icon there. I don't know if you're sure. Are there icons? Probably there are icons in PyPI, I think so Well well.
Speaker 2:There's a description in PyPI. But even if you would say let's use a description in PyPI, it can be very extensive, right.
Speaker 2:Like it's basically they get the pre-me that the PyPI often uses, but that's just the icon Python they use. They pull in stuff a lot of different ecosystems. It's very hard to come up with a unified approach. So what they did is that they have had an LLM writing the description of the package and also generated image. Ah, generated image as well, yeah, and for a lot of things it was like completely wrong. Oh, really Like completely wrong. It was very clear like they use some very cheap local LLM.
Speaker 1:Like they wanted to use it but didn't have money. I think that is it, yeah.
Speaker 2:I think if it would have done everything via the chat. Gpt-4 preview like there would have been like there would still be errors, but like it would be out of date. But how much do you think that would cost too?
Speaker 1:Is it worth it? Because what's the trade-off? Right, like you're paying more money for better qualities, but how much money you pay, I don't know. It's a good question, but not going to be hundreds.
Speaker 2:I don't know how many packages they have. Okay, I don't know if it's not that big Like it's. I ran some translations through it the other day. It was easily seven euros, but like doing the extra translation would be way more expensive, but like seven euros is easy to get.
Speaker 1:Yeah.
Speaker 2:And the preview of chat GPT-4 is actually cheaper than chat GPT-4. So they want to use the latest one.
Speaker 1:Did you just want people like users to test?
Speaker 2:this stuff Okay.
Speaker 2:Okay, I feel about like for me, but it's an interesting. The drama, of course, was because there were package owners that said, like what the fuck are you doing? How are you describing a package? But it's interesting to see like I think it was very much from good intentions, like you, our users need to understand something about this package, but also like the lack of correctness introduces in this case, and here it was very, very visible, probably because we use a very cheap LM. But I think the challenge is that it's often the lack of correctness is not really visible because it sounds very believable, right.
Speaker 1:Yeah, there we go. There's. Here's an example on the screen RabbitMQ. So putting the generated AI generated images was also a bold move.
Speaker 2:That's a bold move.
Speaker 1:Yeah, Like even we saw on the turbo thing you put one word and easily becomes a.
Speaker 2:Exactly.
Speaker 1:Cool. And are this a company? Or is this like just an open source project?
Speaker 2:I think, I don't know. I don't know, to be honest.
Speaker 1:It's quite a lot of. I thought it was a bit amusing that it's like Gen AI, the drama and then so they started talking here for example, is a twin, I don't know.
Speaker 2:It's like it's actually built in Python. It's a tool for your terminal Like that lets you do very cool stuff with history in your terminal, and then described as a virtual reality mapping software.
Speaker 1:What would that even mean Virtual reality mapping.
Speaker 2:I don't know, but it sounds very cool. Sounds cool, right.
Speaker 1:Yeah, it's a chat. Gpt for you, right, like very fancy, means nothing and actually I think it was interesting. Interesting, I guess it speaks to the dangers, I guess Exactly, or the downfalls, I don't know. All right, let's go. I think we have quite a lot of topics still. Bart was very busy.
Speaker 2:Well, we don't need to cover them all.
Speaker 1:Indeed, but I always find amusing the hot takes. Maybe we can cover those.
Speaker 2:Always dangerous.
Speaker 1:I know, but that's I mean. I didn't put them there, so it's not dangerous for me.
Speaker 2:Next week you're going to bring the hot takes, okay.
Speaker 1:I'll see if I can find some, but I'll do my best.
Speaker 2:So I'm going to. So I have an article here on how to choose the right type of database. It came into my feet one way or another From Tiny Bird, which is yet another database. It focuses on real time data, but it was more the article that triggered me a little bit. I'm going to make a statement. I'm going to let you handle it. We have too many databases.
Speaker 1:Yeah, I think I agree. I mean so I'm not the most in the know when it comes to databases, but I also think it's a reflection that we do have too many, right, I feel like if we didn't have too many, I feel like I will have a grip on what's out there, but that's not the case at all. But even like there are different types as well, right, so maybe we should start there. Yeah, there's like relational, like sequel, then there is no sequel, then there is a graph, there is a Vector, databases, what else?
Speaker 2:And I think for the. So if you key value store so often used for caching of stuff. Yeah like Redis, these types of things. I think that is we need this.
Speaker 1:We need the red is in our life but I feel like, do we need different types, or like if we had one for each, that's, that would be enough, or no?
Speaker 2:new bit of competition, right, but I think if Tomorrow the world ends, so okay okay, normal life ends. Yeah, you need to rebuild. Two databases left, okay, one is not enough. I'm gonna explain you why. I'm gonna tell you what the only things that we need. One is Redis. Okay like good key values.
Speaker 1:Well, I think I know the next one. I think I know the next one pause. Grace for that man. I knew, but like you're a pause, chris fanboy but pause credit.
Speaker 2:So yeah you go. You go into the article. There's key vice first. Redis is there, of course, like it's Graph databases. Okay, it's interesting but don't see it taken off. I like every five years is a new hype and they still don't see it's really taken off.
Speaker 1:Yeah, and I feel like Neo4j is the one. I didn't even know there were others. To be honest, I only hear the graph is cool.
Speaker 2:I think the most, most modern ones are angle DB, the graph is. D graph. I Use some of the tooling, if some cool, in memory caching libraries that they separated from the from the DB.
Speaker 1:But I guess Neo4j still the standard.
Speaker 2:When it comes to corporate world, I think this is standard. Yeah, okay, they're a document databases. So I think these are your most people would think of as no sequel databases, which we do not, do not at all need in our lives. Okay, and this is very no, but it's really, this is, and I I've fallen into this myself a few times in my life and it is too easy in life to say I need a no sequel database, okay, and you're always wrong. I think what we need is, like, if you're a developer and you're often make the choice like what kind of database I'm gonna use, you need to have like a monkey sitting on your shoulder with like a I know, like a like a branch or a wooden ruler in the hand just to catch you, and from the moment that you think about, ah, maybe like this is what you could use to make Cosmos, to make and like it hits you on the, on the, on the fingers, whatever wooden ruler.
Speaker 2:Okay, I think that's what we need in life.
Speaker 1:That will solve the world problems.
Speaker 2:I think it would solve a lot of inefficiencies. Okay, maybe uh.
Speaker 1:What is no sequel, and why do you hate it so?
Speaker 2:much so no sequel is Uh well, this is no see. There are a lot of things. No sequel to what we're talking about here is document databases. Yes, um, where you basically have and I'm gonna Simplified a lot is that there is no schema and you more or less Just drop in adjacent alike structure.
Speaker 2:Yeah, maybe by schema is like there's no, it's not columns with specific types, it's just like yeah, it's not that black and white like that, but but yeah, that's a bit how you can, yeah, um, which means that it is super easy to start developing because from the moment that you start developing, you typically don't know no, these are the columns I'm gonna use, these are the tables I'm gonna use. It's super hard to know when you start developing. So what people at that point wrongly do. They choose a document database Like mongolabee or hb. So xanthas, here, um, they start developing, they say, oh, here I have a user, I'm just gonna create an adjacent with properties, name whatever and the challenges. From the moment they actually deploy this, it gets used and then you're in shit.
Speaker 2:Because schema less. You know you don't define the schema. So this, these documents can be anything Right and you, as a developer that just created that, like you, know what the content is, you know what you can expect. Right, but you tomorrow, you tomorrow, but also okay, now it's like one service that talks to this, this, to this database.
Speaker 2:Tomorrow, let's say there's another team that creates another microservice that also talks to this database and they're gonna say it's a bit difficult that there's no schema in the database. Let's put a schema in our orm, in our code, and so they're gonna have their schema basically written in the code. And you're gonna think, oh shit, these people changing stuff. My expectations are not long, so you're also gonna get to put the schema in your code, so you know what you can expect. And now you have two places of of of uh, where your code, where you basically meant to have to maintain the schema.
Speaker 2:Yeah, because it's not always aligned because it's not coming from your database. And then tomorrow you said the first thing that you made was written in python. Even if you now do an extension of your first service, like in another language, I need to rewrite the scheme again, the scheme again in that. So schema list doesn't exist. Yeah it's just like do you want the schema In your database or do you want to write?
Speaker 1:writing it or when you're using it, I guess, yeah, yeah. So it's almost like you put a stack of papers Just like, oh, I'll use this later, and then just put it in a closet, you close it and then, when you actually need it, that's when you're in trouble.
Speaker 2:And then it's all like, for the moment that you grow these things and beginning, it's not very painful, but for the moment you grow these things need to maintain it, it's always becomes an extremely painful process.
Speaker 1:Yeah, maybe uh the difference between.
Speaker 2:This is very opinionated. There will be people that uh might cancel me for this, but I I stand by it.
Speaker 1:You're gonna go down proudly.
Speaker 2:I'm gonna go down proudly yeah.
Speaker 1:I will be shouting postgres, postgres. Okay, the maybe difference between document and key value is just that key value cannot have nested stuff, because you mentioned json. Json has keys and values, but I guess that what's the difference there? Maybe.
Speaker 2:Uh. So a key value is basically what the name says. You have a key, yeah, uh, which is uh, it should be unique, and you know that this key, something is linked to that can be, json can be some binary can be whatever, but like it's there's only, it's much easier to retrieve because it's a single key. Yeah it's um.
Speaker 1:Yeah, and I think when documents you can have nested stuff right like you keep going on and on. Uh, if it's truly like a json, I guess.
Speaker 2:Well, I think what we're talking about here document databases that you need to need to compare more with a, a traditional sequel, not a, not a key value store, but a traditional sequel data is where you have like an A, uh, an entry for, for example, a user and it's in a json structure or this cleanly in in columns. Yeah, I see, that's it, and you're gonna do searches, you're gonna do queries on that and you need to retrieve stuff and you want to do that on a clean column. We know what it's in there. Or you need to like unpack jason's and uh, yeah, and I think postgres, postgres, um, maybe we should touch the, because our number number of other ones as well, I think they're there before you Take postgres home. Is that what you're saying? Yeah, exactly, exactly. So vector databases um, I got really hyped this past.
Speaker 1:Uh, that really hyped.
Speaker 2:Thanks to uh, thanks to rack um retrieve look mentored generation, generation, uh, which is uh. So vector database. What are they used for?
Speaker 1:Basically you have some text and then that text gets mapped into a vector. Well, that's in rag, like I guess. But then vector is just a sequence of numbers. You can think of that Um and then basically you store that somewhere and then guess it will be in a vector database. The idea with rag is if I ask, for example, what's the capital of brazil, you can also create a vector that represents this sentence and then you can look for the vector closest or like the neighboring, like, if you so, if you think of a vector In math, you can plot it, so it's basically a point in space and then you can look for the points next to this.
Speaker 1:So the idea is that if I'm asking for the capital of brazil and you have wikipedia, the whole wikipedia downloaded as a vector database, you can see the chunks, the paragraphs, the sentences that are closer to this question and ideas that Usually that will have the information that you're looking for. You may not be perfect, but then you extract that and you put it all into chat, gpt and you base or a gpt lm model and you basically say Based on this text, can you answer this question? And that's how you have your answer there. So it became really popular because it's very efficient on storing and finding this information.
Speaker 2:And I think only very relevant when, like, if you're doing this at huge scale, right. Yeah um, and maybe and Maybe, at a huge scale, they outperform Pg vector, which is a plug-in for postgres, which is for free.
Speaker 1:Well, but even like, maybe we can get to the postgres. But postgres, uh, they also have a json type Ski column. No, yeah. So even then you kind of it's not like a black and white right like you can have a bit of unstructuredness into your structured.
Speaker 2:Yes, so you're in with with postgres. So you have a standard schema where you say this column is that type Etc can be a number, can be text, can be, but can also be A json or json b, binary json, representation, um, and that indeed allows you to still have a little bit of flexibility. Yeah, we say I don't know yet what I'm gonna, what kind of data I'm gonna capture, so I'm gonna land this in this json b column, but it will also make this transition of okay, now I know what the structure will be. Yeah, now I'm gonna create columns for it makes that transition much easier.
Speaker 1:Yeah, agreed, agreed, and now there's even, like you said, postgres can have plugins. There's a pg vector which is a vector plugin for him. Yeah, what else we have? Real-time column databases. Is that the last one old app where I mean there's a lot of?
Speaker 2:There's a lot, yeah, um, there's a lot of so, and, of course, a little little bit, tiny bit exaggerating on postgres, I think, for this is a good example. So these, these, uh, these type of databases are really focused on analytics, analytical workloads, or large aggregations, large joins over over over different Tapes, sometimes even over over different dbs. Um, and the ones that you see last year, clickhouse, patchy druid, for example, duck db is in there, um, tiny bird, the website where we're on they they are, of course, very much focused on this specific type of workload, right, um, and I think the um. The actually next category that they list here is is olab database for warehousing. They are a little bit linked To that in the sense that one is more focused on on real time. They're the more on batch, but really focused on analytical workloads.
Speaker 1:Maybe what is olab for people that Just see this first, for the first time olab is online analytical processing and it Uh so really based on analytical workloads.
Speaker 2:As I said, this you can mirror, that logger, you can put that. Compare that to oltp, online transaction processing, and online transaction processing is, uh, the, I think, the original premise of why the database was needed. You have, uh, you need to do and need to store a record somewhere, or you need to update a record To re operational usage. That's oltp, uh, a lot of databases are optimized for that. So if we talk about native postgres optimized for that, hmm, um, these type of database olab database are really optimized for the analytical workloads. So large queries, large joints, sometimes over different systems.
Speaker 2:I think that is the the main, the main difference, and it's very hard to do both. Yeah, to to optimize for both. It's extremely hard and, um, of course, like they are exaggerating a little bit for Large cases where there you need a lot of scalability, where you have a huge amount of data, these things are very good. Yeah, we use them a lot, um, but If you don't have huge amounts of data, I would also look at uh pg analytics, a recent plugin actually for postgres uh, which uh allows you to uh save, uh Uh tables in a column in our way and also use them.
Speaker 1:Okay, yeah, I don't think I could the right one.
Speaker 2:But there are so many databases and the thing is that I see them popping up and when are? Where do databases get big? Is when they actually start being used in Solutions, and I think most of the ones that are listed here in this article actually Databases where there is a large team behind, where there is, or even more. I think you can for some, maybe you can still debate that, but A database we've discussed this before is like a choice you make.
Speaker 2:That is very fundamental, like you're it's very hard to change the database, so choose one that has proven itself. Yeah right, not the most fancy new tool. Yeah, I think that is super important for when you choose a database and even not only one that does, that is a tool that has proven itself or company that's proven itself, but also a paradigm that has proven itself. Paradigm meaning like. Sequel versus no sequel. Yeah no sequel document Databases. You really hate them. So yeah, they're my rent is over.
Speaker 1:no, but I agree. I feel like the fact that you see this article and so, basically to help you navigate through it, yeah, there's so many types for each type, there is a whole bunch of them for me is like I Mean, I'm, I'm in tech, I'm not really into the database game, but Even for me it's hard to to keep up right and usually, like even you say this is for OLTP or OLAP, all these things Sometimes I mean from my finder's tank, correctly is like it's not that you cannot do one thing with it, with a Database you can, it's just that it's not optimized for that. Is that? Is that a correct statement? Exactly, yeah, so it's like even then is like the terminology gets more, you know, more and more, not niche, but like it's not about capabilities, about what it's made to do, and one of the optimal things, but to me about.
Speaker 2:It's actually not on here, I think which everybody should know is equal light.
Speaker 1:Oh it is. Oh, this is the most popular one, right it's?
Speaker 2:usually popular like most most the most used, most used and often also used in development environments. So we're, where you want to test against a sequel database, because in production you are talking to sequel database, then in that case, and then your sequel light is like your local version with your current test against, and we actually since I want to say two weeks ago, we have a PG light. Really, you're really PG light is postgres Reimplemented in wasm there we go, yeah, indeed and I've seen some demos and it's really allows you also like.
Speaker 2:So to me it was always a little tiny bit of friction, but you can abstract it away. Typically, if you develop locally against sequel light, but in production you're on postgres, like most of it is similar but like you need to change a little bit of things, this allows you to have like a Very, very, very polite with postgres instance running for development purposes that you can test again. So you're actually testing against postgres, which is very cool, but you can also run very like super efficiently in the browser. Yeah, so that was online, now that you can Wasm is web assembly right?
Speaker 1:so basically means you can run on the browser. So cool. I see bun here as well. I don't know if we talked about. I think we talked about bun.
Speaker 2:But yeah, so the Sky is the limit for postgres.
Speaker 1:Yeah, so few took. One thing from this episode is Maybe you should also have a button with proscreas, you know. I think that's a good one, maybe a no, if we have time for one more quick one tell me which one just the making table head is sticky.
Speaker 2:Oh yeah, it's something super random.
Speaker 1:It's also hot take.
Speaker 2:So it's a ticket sort of mess it on. So apparently. So everybody knows any large atml, the based table, right like you scroll down the table, you have like 20 columns and you're thinking, what is this value that I'm actually looking at. You look up at the car. The header is gone. Yeah right, because you need to scroll back up for the header for the people.
Speaker 1:They have on the video. You can see a bit of Example here.
Speaker 2:Yeah, scroll it down.
Speaker 1:What is this? What is this number?
Speaker 2:for example, right and Apparently in CSS is for easy to add a little bit of CSS properties to the T hat element, which is basically a table heading element, to make sure that it sticks to the top, so it always keeps Frozen to the top. Basically, like you can also do an Excel or in Google sheets, like you can free show your first.
Speaker 1:So, basically for trying to paint a picture with words, it's like if you scroll past the heading, the heading would always show at the top, exactly right. Yeah, so then if you're in the video, you can also see another example here as well. Yeah, and Then you're, you're rent here, or you're. Well, it's not a hot take, I guess, like you just saying that everyone should do it or that should be the default everybody should it everybody should do it. I don't think that's that hot. It's like warm.
Speaker 2:Well, I, but you will get. The discussion that you will get here is that there will be people that say, yeah, but you should not mood make a table Scrollable in HTML table needs to be part of the full page, not in its own element. Okay, that's what I will assume it will happen. Anyway, maybe okay, art this, cd, these two position sticky top zero CSS properties to your T hat.
Speaker 1:Okay element maybe I'll also plug into, because I also I'm a fan of this. Actually, I'm with you if this is a hot take, if we're gonna get canceled for this, we'll do it together. I can go further. Vs code also has a sticky. What's the name sticky? Hatter sticky scroll feature so what does that mean?
Speaker 2:a sticky scroll feature.
Speaker 1:The idea is, you see, like if you go on the video, yeah, basically, if you have a function definition, I guess you say, oh, your functions are a bit too big. But if you have a function, a class, so it can be a class with functions, right? Yeah you will stick the methods.
Speaker 2:Okay, yes.
Speaker 1:Wow, look the bus. If you have a class with methods or you have a function with functions or whatever, basically you will stick the same way that the header stays on top, it will stick the.
Speaker 2:Okay, okay. Definition of the function or the class or the exact but I it's even both right.
Speaker 1:So if you're on the, on the video, you can actually see some examples here. So you see, here there's a class with some with some methods and some some methods or something, and basically, if you see, this function is like oh, what is this part of? Oh, it's part of, this is part of that, right. So if you scroll too much, so actually I quite like it. It's not a default, but you can very easily enable it on VS code and I'm also all for that. So Try for yourself, but Do it, let's do it. All right, and I see we also passed. Well, we passed the one hour mark. I'm not sure if we have more topics, but I think we can keep some from next week. Yes, anything else you want to share?
Speaker 2:What are you gonna do for the weekend?
Speaker 1:What am I gonna do for the weekend? That's a good question. I think I may need to unpack my luggage from Romania. Yeah, judge me, bart, okay.
Speaker 2:This is our disc loading that you skied in for a whole week. It's still suitcase for a week now.
Speaker 1:No, yes, thanks. Yes, indeed, I ski with some, but like you know, it's, it's fine. I actually took them out of the luggage and put it on the corner, okay.
Speaker 2:Yeah, you're a little dry.
Speaker 1:Yeah, yeah, yeah, and then I'm just gonna put it back on the closet now. No, just kidding, I'm gonna wash it, of course, but it's a bit of a mess. I feel like I came back from holidays. I didn't have time to to properly do all these things. It's too much to tidy it up. What about you?
Speaker 2:anything, nothing planned.
Speaker 1:Really so you you made me go through all this just to say oh no.
Speaker 2:I honestly don't really plan the time with the kids, but running, and you, alex.
Speaker 1:Yeah, nothing planned either. It's an easy answer yeah, maybe I'll just go last next time. You know just let everyone know.
Speaker 2:Next time I'll ask you how the laundry went.
Speaker 1:Thanks. Thanks for keeping me honest here. All right, I think that's it. We can call it a day, call it a pod. Yeah, you're pressing this thing, do it. You have taste In a way that's meaningful to suffer people.
Speaker 2:Do you not know who everybody is? Hello, I'm Bill Gates, bill Gates, I was Bill Gates. Yeah, nice fun, I Would. I would recommend. Uh, yeah, it writes a lot of code for me and then that's just a hero. I'm reminded into that the rust. It's what castle congressman? Iphone is made by a different company, and so you know we'll not learn rust. Well, I'm sorry guys, I uh, I don't know what's going on. Thank you for this. Sounds like Larry.
Speaker 1:Davis About large neural networks. It's. It's really an honor to hear.
Speaker 2:You have some outman before Congress, pretty cool, thank you.
Speaker 1:See you next week, ciao, ciao.