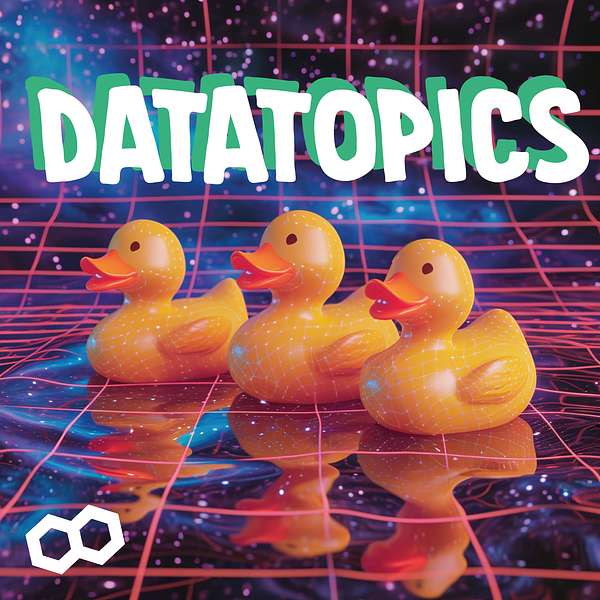
DataTopics Unplugged: All Things Data, AI & Tech
Welcome to the cozy corner of the tech world where ones and zeros mingle with casual chit-chat. Datatopics Unplugged is your go-to spot for relaxed discussions around tech, news, data, and society.
Dive into conversations that should flow as smoothly as your morning coffee (but don't), where industry insights meet laid-back banter. Whether you're a data aficionado or just someone curious about the digital age, pull up a chair, relax, and let's get into the heart of data, unplugged style!
DataTopics Unplugged: All Things Data, AI & Tech
#51 Is Data Science a Lonely Profession?
Welcome to the cozy corner of the tech world where ones and zeros mingle with casual chit-chat. Datatopics Unplugged is your go-to spot for relaxed discussions around tech, news, data, and society.
Dive into conversations that should flow as smoothly as your morning coffee (but don't), where industry insights meet laid-back banter. Whether you're a data aficionado or just someone curious about the digital age, pull up a chair, relax, and let's get into the heart of data, unplugged style!
In this episode:
- Slack's Data Practices: Discussing Slack's use of customer data to build models, the risks of global data leakage, and the impact of GDPR and AI regulations.
- ChatGPT's Data Analysis Improvements: Discussing new features in ChatGPT that let you interrogate your data like a pro.
- The Loneliness of Data Scientists: Why being a lone data wolf is tough, and how collaboration is the key to success.
- Rustworkx for Graph Computation: Evaluating Rustworkx as a robust tool for graphs compared to Networkx.
- Dolt - Git for Data: Comparing Dolt and DVC as tools for data version control. Check it out.
- Veo by Google DeepMind: An overview of Google's Veo technology and its potential applications.
- Ilya Sutskever’s Departure from OpenAI: What does Ilya Sutskever’s exit mean for OpenAI with Jakub Pachocki stepping in?
- Hot Takes - No Data Engineering Roadmap? Debating the necessity of a data engineering roadmap and the prominence of SQL skills.
you have taste in a way that's meaningful to software people.
Speaker 2:Hello I'm bill gates. I'm using pence today. I would, I would recommend. Yeah, it writes a lot of code for me and usually it's slightly wrong.
Speaker 1:I'm reminded it's a bust. Rust, rust, rust Rust.
Speaker 2:This almost makes me happy that I didn't become a supermodel.
Speaker 1:Oh, Cooper and Netties Boy. I'm sorry guys, I don't know what's going on.
Speaker 2:Thank you for the opportunity to speak to you today about large neural networks. It's really an honor to be here.
Speaker 1:Rust, rust, rust Data Topics.
Speaker 2:Welcome to the Data Topics. Welcome to not LinkedIn, just YouTube. Check us out there. If you leave a question or comment on the video, we'll try to address it as well. No promises there. Today is the 17th of May. My name is Murillo. I'm going to be hosting you today. I'm together. I name is Murillo. I'm going to be hosting you today. I'm together. I'm together with me, my partner in crime, my sidekick, question mark, Bart Yellow, and the sound engineer behind the scenes. Keep the lights on Alex. Hi, Actually, maybe Alex will have you on the spot here. Today's a very special day for Alex. No, what's today, Alex?
Speaker 1:What is today?
Speaker 2:What is today?
Speaker 1:It's the end of my internship.
Speaker 2:The last day of the internship.
Speaker 1:Can we get an applause?
Speaker 2:Yes.
Speaker 1:Applause for a great internship. Many thanks to Alex. Indeed indeed, indeed, For getting us professional. Indeed indeed, indeed, For getting us professional.
Speaker 2:Indeed, indeed. Yeah, I think there was one day that Alex was not here, and I think we were like oh yeah we need to do this. Oh no. Oh yeah, alex did that too. Oh no, this was a bit all over the place. So very happy that we had you here this whole time, and I couldn't help but noticing that the intro song is slightly tweaked slightly tweaked, so do you want to share anything about the soundbites there that you added?
Speaker 1:there's a soundbite about Cuber and Neddy, and there's a soundbite about Cuber and Neddy's and there's a soundbite about being happy not becoming a supermodel. So all these soundbites in the intro, they always in some way come from interviews or speeches that have something to do with data or ai and uh, being happy, not have to have become a supermodel yes comes from uh conan o'brien discussing uh deep fakes okay where, where he has been stating like uh, the supermodel career is a bit under pressure.
Speaker 1:Now it's become easy to create fake supermodels that is true so he was happy that he didn't became one. It's a bit like, uh, like your life my life. Yeah, like, like at some points, like you were at a crossroads, like am I gonna be like ai tech lead or become a supermodel? Oh yeah, you chose the left part, right yes, yes, it's true, and with all the development, it's probably the good one.
Speaker 2:Yeah, you know I was this close to making a big mistake, but it's fine for the people that I feel like I need to explain this a bit, you know, because I think for you and me that we know the content. This is is not true. Bart made a deep fake of me and he actually spread the news, the rumors within Data Roots that I took a modeling.
Speaker 1:It was a good picture that I generated. People actually bought it. Do you still?
Speaker 2:have it actually, I don't know. I want to see it, I don't know if you can see it.
Speaker 1:I'll look it up.
Speaker 2:in the meantime, yeah, I'll look it up. In the meantime, yeah, and while you're looking that up, one thing we've just shown this before supermodel. We saw this on Twitter. One of the colleagues at Data Roots shared this and I thought it was pretty funny. It also relates to what we mentioned last time, so for the people that are not watching the video, it's a side-by-side picture. On on the left there is a dicaprio and gisele bunchin, uh, and it's a bit weird, like, as I'm saying, her name, because she's brazilian but she has a german last name yeah, so it's like should I say with the?
Speaker 2:if I say with the brazilian accent, is this okay?
Speaker 1:I think you said it correctly. It's just the first name. Say with the, if I say with the Brazilian accent. Is this okay? I think you said it correctly. It's just the first name. I would have said Giselle.
Speaker 2:Giselle, no, but that, that one I'm most confident because, like Giselle, yeah, exactly, giselle Bündchen. So that's so. Then it says on the top it says dating a model in 2004,. Right, so then you have the's the name of it a joking, joking, uh sky, something like that, the guy that the actor that played the joker, and uh, it's a scene of the movie her. And then he says stating a model in 2024 pretty close, when, actually the first time, when I heard the supermodel on the intro, I actually thought of, uh, like a super ai model, like a open ai chat gpt kind of model. So you know, it made me giggle. So hopefully it made someone giggle at home there as well.
Speaker 1:And and we have uh for the listeners. Marilo is opening uh his own um deep fake about him as a supermodel. If he would have chosen the right part well now right is correct, no you took the left part to become a AI tech lead. If you would have chosen the right part, there we go.
Speaker 2:This is Bart doing. If you're wondering what bart and I we talk about, well, I'll link to the show now and you know, and it's like I thought it was pretty obvious, uh, that it was a fake. You know, and then I even put here you see the comments like I put when you order robert peddinson and wish that was actually my good.
Speaker 2:It's very good remark and then and then, but actually like afterwards, we had a ski trip at date roots and a lot of people were like oh wow, he's real. And I was like no, it's not really. And I had to really explain this. So I thought it was pretty obvious I don't, I'm not, I'm not modeling material by any by, not in close right. But uh, quite a lot of people uh believed you. So I think I need to make sure that I explain it here so there is potential.
Speaker 1:That's the conclusion I'm not sure.
Speaker 2:I'm not sure. I think it's like when you say something about people that don't know you that well, they're like, oh yeah, no, he's the you know founder of dataverse. It's like he's a serious guy, yeah, but um, that, but that is that. Would you have believed him, alex, if you saw this? When was it made? When was it made? This was made A month ago, two months ago.
Speaker 1:Two months ago.
Speaker 2:Wow, ouch, okay, whoops, yeah indeed.
Speaker 1:What do we have on the agenda marula?
Speaker 2:well, why did you fill the, the picture that you put on slack? Maybe a good segue to talk about privacy principle slack? Uh, I think it's not a blog, but a privacy principle search learning and ai. Uh, what is this about? Bart?
Speaker 1:yeah, so Slack has a page on, basically a sub page on their data management info page on their website. That is about privacy principles privacy principles specifically about search learning and artificial intelligence. Why I put it on there and I'm not 100% sure if it is actually a new page, but there was a bit of chatter on this on Hacker News because what they're basically saying and they're not very specific on what they're doing and how they're doing it is that they use customer data to build AI models and that you can also, like your data will be used for global models, so meaning that models that other other slack workspaces can also benefit from, and it's not an opt-in principle, it's an opt-out principle and it's not even an opt-out like. You go to settings and you click opt-out. Now you really need to send an email to feedback at slackcom to opt out of this.
Speaker 1:It feels a bit weird, right? They also give some examples on what type of models. So they do channels recommendations, they do search results, autocompletes, emoji suggestions and emoji suggestions feels very low risk, right, but autocomplete. What if there is some data leakage in these type of things? Like? They state also in the same document that there is no possibility for data leakage, but well yeah.
Speaker 2:Yeah, yeah, there's a lot of trust. Yeah, they mentioned here that data would not leak across workspaces, but at the same time, yeah, like you said, like if you.
Speaker 1:They don't give any transparency on like. What type of models are these like? Are these models that like more, let's say, a more traditional machine learning model, like classification model, these type of things, a recommendation model for channels? Or are these really like LLMs that they're training to do auto-completion? Like based on these models? Like? There are different risks linked to this as well when it comes to global data leakage, but they're not very transparent about it and it feels a bit weird.
Speaker 2:Also, not 100% sure whether or not this will, uh, survive gdpr yeah, I was thinking gdpr or the ai act uh, or both, both probably, but even gdpr um but like but you're saying, it will survive gdpr, but gdpr is already there, right?
Speaker 1:I'm not 100 sure, like how new that this document is. To be honest, I just saw the chatter on Hacker News today, but it feels weird that you have an opt-out principle instead of an opt-in principle as a customer, given that there is a lot of PII data, typically on Slack.
Speaker 2:Yeah.
Speaker 1:Without any other transparency on what is the type of data that they're actually using, right? Is this actually like private conversation, uh data that they're using, or is this just metadata on channels like that's a big difference, of course yeah, no, I see your point and I also think, indeed, they're saying ai as a umbrella term, almost right.
Speaker 2:and I think ai can mean a lot of things. Right, like yeah, it's very general, so it's like search engines, like maybe you can even say it's AI or something like that. Right, so yeah, does this make you want to use Slack less? I know you're a fan of Slack, so maybe that's how.
Speaker 1:that's why I'm also asking I'm a fan of Slack-esque communication. You have more of these type of things like Mattermost. There are a few others. Migrating to something else is a whole lot of work, right? Yeah, um, I think this is also like like that slack is doing these things is also a sign of their success. I think what they need to now do is a few iterate a few times and then create the needed transparency around this yeah, I think that is uh I prefer more transparency versus the whole effort of migrating to something else.
Speaker 2:Yeah indeed, and also it feels like they're not being very transparent either right, which I think that's a bit of a flag.
Speaker 1:Well, I think that is why there is a bit of kerfuffle.
Speaker 2:Yeah, not only that, but also they make it a bit harder to opt out.
Speaker 1:Well, yeah, exactly that doesn't feel.
Speaker 2:It raises some flags right, raises some flags. More on AI, then why are we talking about AI? Maybe for people that are not super uh familiar, but the differences between what chad gpt is and just ai like, if I say chad gpt, I guess I'm talking about lms right and ai. How would you uh describe the differences between the two or the nuances between the two for someone that never, that is not in the field?
Speaker 1:when it comes to the risk of uh data leakage or yeah, we're also like the yeah, data leakage.
Speaker 2:I think he's like yeah, because, for example, when I was studying ai, they talked about pathfinding. You know, like, what google maps does, probably. You know from path a to path b when you have a heuristic or like the old school chess systems, right, that's technically AI. But I think when you talk with like yeah, if they use something like that to train a model, to build heuristics, whatever, it's very different from machine learning. It's very different result from LLMs, right? I think that the levels of risk and why we're thinking about that is if there was more transparency, maybe the question wouldn't be so much in our heads.
Speaker 1:Yeah, that's a good question. It's hard to give a holistic answer to that, but maybe in the context of Slack right, like where they're saying where now there's this discussion, like there's customer data being used for globally, for models that will be used globally across workspaces, for models that will be used globally across workspaces, like if we have a bit of a sound issue, yeah, sorry, so the difference between LLMs and and AI in general, right, like I guess AI is more what I'm trying to get at more, yeah, sorry.
Speaker 1:I was a bit distracted with the sound issues. So if we look at the risk when it comes to using customer data within a Slack workspace with traditional models versus LLM, is that with traditional models? What you do is that you look basically at historical patterns and see whether these patterns are occurring today. Like, for example, if you look at channel recommendations in Slack, we saw that everybody that joined in the past also joined channel X. So based on that, when a new pattern occurs like a new joiner, it probably makes sense to recommend channel X to this new joiner. That's a bit like these traditional machine learning models.
Speaker 1:We recognize a pattern and we see that there's a big potential that this pattern is also at the state of emerging again and we quote-unquote forecast that and I think the big difference with LLMs, which is the underlying technology, with ChatGPT if we ignore how it's built and the architecture and stuff. What it basically tries to do is it learns on text and what it tries to do is based on a question or a word or a phrase. It tries to predict the next most, uh, likely word.
Speaker 1:Yeah, the quote-unquote most correct word that comes next and it iterates over that until the sentence is complete. And from the moment that you do that's based on actual text that was used in your channels they they were in your private channels then you have this risk that from the moment someone asks this model something that it could be that you use this a lot in your Slack space and that the model thinks it is very likely that I need to respond in this way, but that this way actually is something that was very specific to your current Slack space and that it is actually sensitive data, and I think that is the risk here that we're trying to understand what is text and what are responses to text versus what are patterns that you can express in numbers.
Speaker 2:Yeah, indeed, and I'm also, for example, for the text. Completion is a good example, right? For example, let's imagine that I'm always asking people to transfer me money on my bank account and then somehow this data gets through and then when someone else is typing, hey, can you transfer money too? And then my bank account is there, right, which is very sensitive. And that would be different from if the actual AI is just trying to see what's the. They have all the possible available words in english and they just see what's the shortest path to the next word, like some heuristic or something like that, which technically is also ai, right? I even seen, like, if you really push it far. I remember I was even in a talk that he said there was a ai powered coffee machine, but the only thing that the coffee machine would do is that the coffee you order the most would be the first one on the list, and they consider that AI. So I feel like AI is a bit more fluffy word.
Speaker 2:Definitely true, and people play more with it and I feel like it's also safer for people to say AI because it's an umbrella term, so for sure it's going to be inside there. But I think the actual nitty-gritty is very different and I think the concerns are very different different and the complexity is very different, and I think that is also a bit of our.
Speaker 2:Why people feel like there's a lack of transparency when slack says is because like yeah, indeed, like it's become this umbrella term, like it is just some smart heuristic exactly and like that indeed goes from a coffee machine to chat gpt exactly and talking about chat gpt, there was some improvements for data analysis, and it's a bit meta, right, because chat gpt there was some improvements, uh, for data analysis, and it's a bit meta, right, because chat gpt is a system that is for text, but the text, the content of the text, can actually be about data analysis, right, and um, the chat gpt, or open ai, actually released that. Uh, there's some more things. Actually, that was a pretty well documented use case, right for chat gpt. Like you can actually send a csv there and say, hey, what's the highest paying customer? Or hey, what's this in natural language, and then chat gpt would actually do a pretty good job at looking at the data and answering with yeah so what is this about, bart?
Speaker 2:what are the improvements here?
Speaker 1:um, so what uh open? I recently uh launched may 16th, which is uh, which was yesterday, and I haven't tried it out yet yet, which is a bit of a pity. Um, but I think it's already available and it allows you to basically take this, what you were explaining like upload a CSV, ask some questions about it to the next level.
Speaker 2:What is the next level?
Speaker 1:Where before what I did with CSVs, for example. I uploaded them to chat GPT and I asked it to generate a plot, and it used Python and matplotlets to actually generate plots. What you can do now, how I understand it again, I haven't tried it yet is that you can directly connect Google Drive or Microsoft OneDrive to give JGPD access to your data and that you can then query this data using natural language.
Speaker 1:You can say, okay, I point to this file, that data set in my drive and then interrogate that, and that it also has built-in native visualizations, where before it used Matplotlib and, if I understand correctly, now it has like native charts and graphs.
Speaker 2:Yeah, for the people following the video, we actually have the announcement page here and if we show some of the graphs it looks pretty nice. It looks more polished than metplalib exactly, exactly.
Speaker 1:It looks more native, um, to a web application and uh. But I think here, like we'll, we'll, uh, we'll test it out and come back with some feedback next time, right, I think this is is like again we had the discussion, uh, last episode a little bit on like open ai, putting companies out of business, that we're building on top of open ai because, like every time that they have like a stable foundation, they take the next step. And I think this is again like a good example, like where you saw a lot of these initiative. A lot of startups like to use llms to, for example, ease building of sql queries, these type of things to to ease more the, the citizen data science process, to to interrogate data, where they now have it more or less built in, and today it's just google drive, it's just microsoft one drive, but from here it's very easy to see like it's going to be very easy to immediately connect to a database, for example, like the step to that becomes very, very low-hanging fruit yeah, yeah, you know.
Speaker 2:But yeah, I fully agree. But I also want, like, if I had built a tool like this on top of ChatGPT I also would always be a bit anxious that because it does feel like a very good use case, right, and I do feel like with time, openai is getting bigger and more popular, like it is very natural for them to take this next step. I do remember this alluding a bit to to very, very early podcast days when it was still 2D tools. That was, I think, a tool that you covered that did something. It's very similar.
Speaker 1:No, there was like natural language querying, but I don't even I think it was before chat GPT still even no yeah, we once had in another podcast the author on yeah, I think it was actually Brazilian, even that's how I podcast the author on yeah, um, I think it was actually brazilian, even that's how I remember the ceo was yeah, uh, the company was swiss, but I'm uh, but putting me on the spot here now to come up with the name of the product. That's what I do. I want to say viso, but it's not that. But it's indeed like what they do. Like that it's like a dashboarding application, but their value proposition is not necessarily the dashboards, but that it's indeed like what they do. Like that it's like a dashboarding application, but their value proposition is not necessarily the dashboards, but that it's very easy for users to interrogate data using natural language, to not say, oh, I'm going to build a SQL query to understand, like, well, how were my sales last month? I'm just going to type in what were the sales last month.
Speaker 2:Yeah.
Speaker 1:And this was before Chachd. You know, I think they started before chpd before the before exactly before it was this big, yeah, yeah so I think, with this if you today have your and probably no one has like in a structured manner and up-to-date their data on google drive or microsoft one drive. But I think if you would have, you could already do that today with what they now released, yeah. So I think that step to go from Google Drive or OneDrive to actual databases is really like a small step at this stage.
Speaker 2:Yeah, that one is very, very I mean or integrating with other like Dropbox or whatever right. It feels like it's just an API call. Yeah, yeah, do you feel like now, with these, uh, with these things, do you do you fear for the data analysts of the world, even maybe data scientists? Do you think? Uh?
Speaker 1:I think that is always a very uh, hard question. I like there will always be changes. Um, I think, even even though it's closer to natural, like there will be evolutions in role descriptions and I think a lot of these roles will become maybe a slightly less technical and maybe a bit more experts in the business domain, which probably makes sense, like when the tools become more easy to use, like your priority becomes a business.
Speaker 2:Yeah, so there will probably be an evolution there, but I don't think yeah I think it could even be that, like the, the focus of the, the role changes. But I also feel like for data science or ai or machine learning. I also feel like they expect you to be able to do more, so it it's not like the focus shifts, but it spreads. So dashboarding is easier today, so now we also expect data science to build dashboards or doing the data exploration is easy, so now we also expect you to deploy models as well. I feel like we expect more and more for one data scientist, a machine learning engineer, to cover a broader area.
Speaker 1:see what I'm saying I see what you're saying. I see what you're saying. I I think that is uh, maybe, uh, I think it depends very much on the, on the context that you're in as well. Like I think the advances we see with stuff like OpenAI, with Anthropic, like, with perplexity, they are also going moving much, much, much faster than your typical enterprise environment. True, so I think there, I think it will, that will become true, but I don't think that is the fact of the truth today.
Speaker 1:I think there is still very much dedicated like these are your tasks, this is how you isolate it. But, of course, like we're very much, especially when we talk about LLMs, like we're going towards an era where a managed model becomes a default. Like you don't build an LLM yourself, you use a managed model becomes a default. Like you don't build an lm yourself, you use a managed model and it takes different set of skills and, from an engineering point of view, maybe a bit more software engineering skills, um, and maybe less theoretical skills about how is this model built, how, what is the architecture of this model? But, more than, how do you use it for business? Business purposes, yeah, how do you use it for business?
Speaker 1:purposes and how do you make sure it's robust for these business purposes? And it takes a bit of a different mindset. And also there we will see role evolutions, especially when we talk about LLMs.
Speaker 2:And also feel like the time expectation for you to do these things also changes.
Speaker 1:Yeah, and I think the difficult thing there is that. So it looks a bit like magic, like ChatGPT is very tangible. I can type something in and I can get well since yesterday, like a full report of my sales of last month, and then if you're a data scientist or a data engineer or data analyst, then you have to explain.
Speaker 1:Yeah, but we need to make sure that we have the correct connections and we need to make sure that there is good data quality and that we need to set up these pipelines. I need to get the infrastructure in place and then we're going to have these dashboards, and all of this will will cost us at least a number of months yeah then you will have this reaction here. But I just queried chat gpt yesterday and it came to me immediately like and there is this this disconnect that we didn't have before yeah, right, yeah, yeah, I hear you, I hear you.
Speaker 2:I agree, I think it takes some more people. I mean, I think people that are already used to managing teams, like data science teams, understand the whole thing behind it behind the curtains. Quote, unquote right, I think the message is more easily adopted. But indeed, if I have to explain to my partner this, she's like what you have to do all this?
Speaker 2:This is going gonna take that much, but it's just there, you know, it's like it's just here, you know. So agree, fully agree. But but maybe all of that will also become easier. I mean, I was even uh for even the software part, right uh for model deployment. I think it was even before I started working at data roots.
Speaker 2:But I know that there was the MLMonkey thing, that was to keep track of the models and have some documentation on these things, and today it also evolved a lot right, like when I used it, I think once the MLMonkey thing, like the framework, I guess, and it was a lot more work compared to what we have today.
Speaker 1:Of course, yeah.
Speaker 2:Indeed, like it's not just the AI part, it's not just this or that. Everything kind of evolves, everything matures, the community, the whole, everyone kind of converges to certain standards, right, which also make it easier to switch tools and yeah, so things tend to be easier. But I think, yeah, the difference with that is that ChagPT feels like a bigger step, yeah, and it feels like much more in your face, bigger and faster, bigger and faster, yeah, and everyone can relate to it, right, like you said, anyone can go to ChagPT and be like, oh yeah, I did this. Or like go to the data analyst, like why is it taking so long? I asked ChagP it's a bit misleading as well, which I think is a challenge is a quote-unquote problem of the data science world. I think it's a no. And why am I saying problem? Because another thing that I read is that an article that claims that data scientists work alone, and that's bad. So maybe do you agree with the statement, bart, that data scientists work alone in general. I know it's a bit like a statement.
Speaker 1:I've seen it happen a lot yeah.
Speaker 2:Yeah, and do you agree that it's bad?
Speaker 1:I agree that it's bad, yeah.
Speaker 2:So in this article the author here, the name escapes me right now he goes through an anecdote that he thought he was pretty good at English but that's just because he didn't get a lot of feedback on it. And then he went to the sophomore year of, maybe, high school and then he got a lot of notes and then he kept working on it and he got better. There were less notes, right, not not so surprisingly. And he says that in data science it's similar a lot of times, these projects, there's one person, one data scientist that kind of does everything you know, the exploration, the modeling and all these things. Um, and he, when he started working, he was the only data scientist of this company. And then he started working, he was the only data scientist of this company, and then they hired a boss or someone to look over his work. And then it was kind of similar to his experience in English class that he would submit a pull request and then he would come back with a lot of comments. And then, of course, you get better. You address the comments and gradually, slowly but surely, you get less and less. So you get better. You address the comments and gradually, slowly but surely, you get less and less, so you get better at it. Um, not surprising nothing like even. He says like nothing. Yeah, crazy, right, mind blown. Everything is pretty much. And you start learning more about like config files, start learning about like patterns, you start learning about encapsulation, about abstractions and all these things.
Speaker 2:Uh, he compares data science with software engineering. He says software engineering is a team sport, and I think it's more, because usually a software engineer usually adds a feature to something that kind of exists, whereas data scientists they kind of build a use case from scratch. It's also easier to add something and to look around the code or allow drew or even ask questions, so it's much easier to learn something and to look around the code or allow Drew or even ask questions, so it's much easier to learn. The learning curve for software engineering is steeper. Let's say that's kind of it high level. And he also. He finishes off by saying that the analytics world has analytics engineering. So now with DBTbt, right, he says that people tend to review more the code. And he said data analyst was also more of a solo job, like people were like doing the queries and replying and these things. And now you have dbt, so people are reviewing code, etc. And then he here's to hoping we soon have data science engineering. What do you think of that comparison and that statement?
Speaker 1:I think, if you look at software engineering to make that parallel, like what you typically have is like a product and features get assigned to people, but you're together working on this product.
Speaker 1:Yeah, there's a foundation, you know, like what is expected, like what are the best practices of this product, and you have a bit of a frame of reference and you're very close to the other person because they're also building a feature towards this product. Yeah, I think what we see in data science typically is that and it's becoming better and better but definitely, if we look three, four years back, is that the things that get assigned to data scientists is not a small feature, but it's like a use case. Let's test out this use case and while, at the same time, there is very limited maturity in the underlying framework, like there's very limited maturity in the platforms that they're working on. Like what is the best practices do we? What do we do for experiment tracking? What? How do we do cicd? Um, so there is this. It's at a much earlier stage, I have the feeling, than software engineering is today yeah and I think it's also a bit bit.
Speaker 1:I think think working on it, hands-on on this case is a bit hard with multiple people, because it is very much a specific use case. But what I do think is and I'll leave that a bit in the middle whether or not for a small use case, you should be able to work on it with multiple data science. But what I do think is always bad is if there is not someone involved from business to say, like, what is it that we're actually building? Because that's something that you also see a lot. Yeah, okay, let's just try this out. We really believe in it, and then we can convince the business to use it. I think that's that's not a good idea. Yeah, uh, and also to do this really in isolation of the more established IT slash software team that there already is.
Speaker 1:To say ah yeah, what we do is really experimental, it's really something new, it's a state of the art. So we need a bit of freedom. Sure, you need a bit of freedom, but we also need decent software engineering practices. And what it makes me think about a little bit is the I think that's where we're going is a bit the, the data mullet concept data mullet are you familiar with this?
Speaker 1:not sure I am um, so data mullet is a bit of an extension of the data mesh and I'm not the best at explaining this, but like the a mullet. You know what a mullet is right? Like a hairdress? Okay, yeah, that's what I thought, but I was like I'm not sure, like a mullet, like business, in the front party, in the back.
Speaker 2:Okay, yes, I know.
Speaker 1:And that is like a bit of a frame, to like a bit of a next step on the data mesh to also make sure that how do you operate, operationalize these, these more ai type of processes into a company, not just have the whole data infrastructure in place the right way, but also like say you only do cases, you only build something if there is ownership from the business. Like you can't say we don't care about data quality that's that's for the it.
Speaker 2:No, you need to own it Disconnected, yeah.
Speaker 1:Proof that you can do something with this data, that you can't say that as a business department, like you say, let's build this use case together. So you need to have someone involved. Like you don't do it if you're not someone involved in business. Two more. And I think this type of evolution that we see going on with these type of skills, these type of technologies maturing will also mean that people working as a data scientist will work less in isolation.
Speaker 2:Yeah.
Speaker 1:Because they will be close to the business. They will be close to the software engineering team, but I do maybe also.
Speaker 2:I did some quick Googling here, data mullet. This is the architecture. It's a bit convoluted, Not sure if it adds any clarity here.
Speaker 1:Not sure if it adds any clarity here.
Speaker 2:I'm not sure if this this uh maybe we need to link some, uh, something that data will link it in that are interested, um, and you mentioned working in isolation, and I agree. I also agree with the. There are some use cases that people start working on and then when they deliver it, they're like, yeah, but no one is asking about this, no one cares.
Speaker 2:Well, exactly that's what I mean, which I also feel like is a bit of a tangent. I think it's a bit interesting because I have the feeling that we, as people, when doing work, we feel like we need to do something always, and then I think that's a bit of a consequence of that. You know, like we have a data science team, we have four people and it's like, oh yeah, no, we need to have a use case, so let's just work on this and let's spend four months working on this, but no one is asking. You know and I think this is something that I think on my role as well uh, that you can be busy but not bring anything right.
Speaker 1:like just because you're busy doesn't mean you're making progress I think we also come, when we talk about ai, very much from a bit of an R&D type of setting, like we're very interested in this problem, okay, there is this new type of methodology to solve this. Okay, let's find some data. Okay, maybe we can predict this and then try to match that to an actual business case.
Speaker 1:Yeah, yeah, indeed, which is also very atypical for software engineering. Software engineering is like let's create a button here, why it first? And software engineering like, yeah, so for me it's like let's, let's, let's create a button here why? It's not because we wanted like because we need it, right.
Speaker 2:Yeah, yeah, like. But also even from the business sometimes I hear like oh yeah, we need to use ai where I don't care, but we need to say we're doing ai. It's also a bit like backwards sometimes that's the hype.
Speaker 1:That's the hype, indeed.
Speaker 2:So but there's.
Speaker 1:There's so many things that play, but also what I want and I think it's a fair one to be honest, like I think it's fair to say, okay, we need to invest in it. We need to understand, like, how it improves our competitive advantage yeah, that's true, but just saying like, just do something, it's not a smart way to go about it. I agree. I feel like you need to strategize a bit like how are we going to make sure that what we're going to test is actually going to create bits of value?
Speaker 2:exactly what does business value look like to us, right? Does it mean more visibility? Is it marketing? Does it mean more customers? I also think there should be some work like to think about exactly to me that is.
Speaker 1:That is part of defining a uh, a good term. I fully project I fully agree.
Speaker 2:But to go back so you did mention um data scientists will probably work, not alone, which I agree. But to go back so you did mention um data scientists will probably work, not alone, which I agree, but still not technical persons, right, there's. It's not like they're working alongside other data scientists or engineers or something right, they're still just a business person I don't.
Speaker 1:Yeah, well, I think you need the business business side of this, but I think also, like what we see happening around us in small tech startups also large enterprises that companies are becoming more mature in this and that the default is no longer like there is a separate data science team that works in isolation on individual use cases, but that is already a bit more like.
Speaker 2:This is part of what we, of our technical capabilities, and we need to make sure that we have the right skills at the right moment yeah, I think that's where we're going I think also, I guess for me, when I read this as well, like it's bad, and you're working alone, I was also thinking of the whole conundrum of data scientists quote unquote don't know how to code, yeah, and I think and again, if you work in isolation, like the other tricky thing about data science is that you don't necessarily want to uphold the highest standards throughout the whole life cycle of a use case, right? If you're in the beginning you're exploring, you don't even know if there is data, enough data. You don't know if the models can actually learn these things. You don't want to spend time with linting, necessarily, right? So I feel like you should be strict at some point, but not always. And I think in software the value is pretty much guaranteed.
Speaker 2:You know, you invest four days building this button and then this button is going to bring this much value. It's like it's there and in data science you can spend three months.
Speaker 1:I mean, if you really want to, you can spend two years and then you go back it's like, yeah, the model is not doing that great, like that is possible as well. That is possible, yeah, which I think it makes. That is the major difference with software engineering to me.
Speaker 2:Like, like with software engineering, when you plan for something you typically know this is feasible or not exactly with uh ai, data science, whatever you want to call it, machine learning you plan for something in your very first phase is let's see if this is feasible or not indeed, and a lot of the times like, even if it's not feasible, like even like you train a model, it's not good enough, or like it's not as good as you thought with the metrics and whatnot, there's always a thing of oh, maybe I can try some other things and maybe it would be good enough yeah, I think there, as well as a team, you need a strategy around it.
Speaker 1:Completely. Don't leave that up to the individual because, some people will say, okay, I'm gonna try this. I have a poor performance of, uh, let's, let's, let's give a percentage 70 of 100. I'm gonna try version two. It's gonna be 72. I'm gonna try version four gonna be 74. And some individuals say yeah, I'm not gonna get much better than this.
Speaker 2:And some individuals will say let's build on this for the next year because I see, still see incremental movements and I think you need a bit of a strategy and I think also the business under the side is like you've been working on this for for two years now, how good are you? And then they're gonna be like whoa, that's it. Like I would expect more, which I also think it creates a bit of friction, but yeah, but at the same time. So on the data scientists working alone, I do feel like there is all those challenges, right, like the ai is a bit different from software. Also, like you should know when to uphold the standards. But I also wonder if you can actually have a truly collaborative experience for data science use cases, like if we're doing exploration.
Speaker 2:It's not like you can say, oh, you explore the data from this country, this country, I explore from this country, this country, and then we come together. You know, I feel like the nature of the job, it is a bit more isolated in a way, and I feel like there's a lot of context. Like even if I do a whole, the tooling doesn't help either. Like Jupyter notebooks, I don't think is the best thing for review collaboration because of the metadata, and I think it's like I can do an exploration like ADA, right, the exploratory data analysis thing and I can give it to you to review. But then there's also a lot of context. Switching to you right is also very challenging, so I feel like there's a lot of stuff that goes into it, which I think makes data science challenging, and I think it would be nice to see a data science engineering like he, like the the author mentions here, but I also feel like it's very difficult.
Speaker 1:I'm not sure if it's something feasible really let's see where we're at a few years from now let's see.
Speaker 2:And one of the things data science do, bart, is like graph analysis. You like that?
Speaker 2:uh, segue like the segue uh, graph is tricky because it's very compute, intensive, right. A lot of the times you have to really compare all the data points. A lot of times you have to combine all the edges and all these things. All in all, without making too big of a deal about this. It's very tricky to computationally have something that scales. You know databases there are specific databases for graphs, like graph, databases like Neo4j, but all in all, it's a tricky thing thing and the reason why I'm mentioning this is because recently I learned about a new library.
Speaker 1:I guess ah, it's library time it's library time.
Speaker 2:Do we have a soundbite for that or not yet?
Speaker 1:not yet not yet, not yet you can now say something and we can reuse oh, I'll say it.
Speaker 2:maybe say it, or we can say it together. A library a week keeps the mind at peak. There we go.
Speaker 1:I'm not sure if that was really a sound snippet or a snippet.
Speaker 2:I don't think so, but you know, we try. If anything, we're agile. So what is this about? This is RustWorkX.
Speaker 1:This is a graph library. Okay, what about?
Speaker 2:what does a graph library do? Well, different things, but, for example, you can plot. So, basically, like, usually the way you describe a graph is through the edges. So, for example, they can just to be very concrete here, you can think of your Facebook friends, right? In that case it would be not directed, right, because if I'm friends with you, bart, you're friends with me as well. What are edges? So edges like, if you have, okay, imagine Facebook, me and you are friends. Are we friends on Facebook? Maybe not. We'll change that.
Speaker 1:I'm not a Facebook user. Oh no, okay, maybe not, we'll change that.
Speaker 2:Not a Facebook user, oh no, okay, if we're both friends, that means that I'll be. How do you say it Actually? The edge would be the friendship. So the connection between two points.
Speaker 1:So if you visualize a graph with dots, right Dots are typically called nodes Nodes, that's the word I was looking for, and the nodes in Facebook are people, yes, and the notes in Facebook are people, yes, and the connections are represented with the lines between the notes, which are called edges.
Speaker 2:Exactly so if you and me are friends, you are dot B for Bart and I'm dot A because I'm first, and then there's a line between us. That would be very simple, and also this is undirected. But, for example, if you're talking about payments right, if I give you money, then there is a direction to it, right. So it gets a bit more complicated and usually when you're describing these graphs, you just have a whole bunch of edges. So he says murillo, bart, that's one real, alex, that's another alex part, that's another one, right. And then you just kind of have like a, basically a long list of two points uh, point a, point b, whatever and then from that you can build a graph. Yeah, right.
Speaker 2:And then from that graph you can do a lot of different things. So, for example, you can see, um, well, one metric is like, well, how many triangles you have on your network, for example. So if you, me and alex were friends, then, uh, this would be a triangle, right. And then basically the the amount of triangles represents how connected your graph is, right. Another thing that you can do if you have you want to cluster the Facebook people into different profiles. You can say if I cut a few edges. How many edges do I need to cut to make these two completely separate graphs, right? And then you can kind of see okay, maybe this group is someone that is really into sports, this group is someone that's really into art, whatever, right, like there are a lot of different use cases, but doing these things is actually very compute, intensive, right.
Speaker 1:So also and that's where these types of libraries come in.
Speaker 2:Exactly right. So traditionally in Python there is one called NetworkX, yeah, which is very well known. I think it's the standard I would say for Python, no, for stuff in general. But there are other ones called iGraph or Graph2. And stuff in general, but there are other ones called iGraph or Graph2. Graph2 I never used the iGraph, I think it's in C++. I want to say and actually this is a new one written in Rust, you like that part?
Speaker 1:Rustworks, RustworkX.
Speaker 2:Yes, I think it's a play word in NetworkX.
Speaker 1:And you've put a benchmark here on the screen where it's a benchmarks rustwork x with others, where it is basically the fastest of them all and network x is the slowest right, yeah, and rustwork x. Can you use it from python?
Speaker 2:yes, that's what I was looking here. Actually I think it is. So you see here graph, classes, pygraph and all the things. But maybe it's easier even to look at the GitHub page. But yeah, I looked here briefly and there is like a Python API. So it is pretty much like Polar, like a lot of other libraries, that actually it's written in Rust but then it's bound into Python so you can actually interact this on the Python layer. So, very cool.
Speaker 2:The reason why I actually came across this so it wasn't me that came across this it was put on our internal slack channel that dbt actually uses network x. Um, so dbt is like you have the different queries and how the the tables map out right, and they were suggesting using rustwork x instead of network x for these things. So haven't checked it out, but I do know that this is. I have had my pains with, uh, graph stuff, like submit a job and then just wait hours for it to finish running, so curious to see how this would work out and then the main premise here is it's faster on rust yeah, which I do think.
Speaker 2:Actually, I think it's a good use case for Rust.
Speaker 1:Yeah yeah, this is really about. Well, if we look about the computational part of this, it's really about speed.
Speaker 2:Yeah, but I think there's also the memory thing, right, like if you're loading everything to do the computations and all these things, memory efficient also plays a. There's a. There's a benefit there, right. So haven't tried it, but very cool. You know the thing that data scientists usually care for data data um, and then that's where dolt comes in. I guess git for data dolt is git for data.
Speaker 1:Yeah, something I came across a week or two ago. It's been around for a while. Apparently. It's quite a bit of stars 17,000 stars.
Speaker 2:That's a lot. How many stars does she have?
Speaker 1:Not that much, and Dold is hit for data, and what they try to do more or less, is that every change to your database gets sort of like a commit hash.
Speaker 1:So that also means that you can revert changes and that is also very transparent, like what what causes change. And that also means basically that you can traverse back in time. And how they and I didn't try it myself, um, but how they basically did it is to, uh, they built on top of a database I think it's MySQL and MariaDB compatible and what they basically do is they add a lot of metadata to that. So I think you can actually connect to it with any MySQL or MariaDB connector, but they have more information than you would find in MySQL. So what you will have is that, for example, if you would query all the rows in a table, you would see extra columns to these rows. That said, I'm not sure how exactly they call it, but I think it says something like what was the commit to that row, what was the message, these type of things, and it really gives you, it really allows you to think about your database like you would do about your hit repository interesting, ah here.
Speaker 2:So I think this is a good example, Bart, you literally have a, so for people following the live stream here. There's in the documentation, so in the readme there's a select star from dot log, so it's a different table for this.
Speaker 1:Yeah, but that already gives more information. So what you would have is that you, let's say, if you do a select star from employees there's like an example there like that where you have to get your employees table you have your last day, your first name, these type of things. But what you also get is like you have a column called from commits, from commit date, uh, the diff type like what's the audit, was the updated? So you get all of this audit to it. Uh, but from the commit ID you can get more information about the commit from another table. So you can you actually have a commit message and stuff like this.
Speaker 2:Very interesting.
Speaker 1:Yeah, I'm not sure how, like, like I said, never tested, don't know how it scales, like if it's uh but I guess everything is like insert only, I guess what do you mean? It's insert only like uh yeah, I think you can also do updates on the table and deletion.
Speaker 2:Yeah, but then how do you keep the history? Because you need to have all the git hashes.
Speaker 1:No, uh, well, I think that it does the updates. Uh, like you mean, how can you revert, yeah, to the original data?
Speaker 2:good question because my thinking is like, if you everything is insert, then you're always just putting stuff there and you have the git hash and you have the git history, so you can always trace back what's the status here.
Speaker 1:Yeah, and if you would want to revert a delete, you need to save that data somewhere, right exactly yeah, that's.
Speaker 2:That's what I would, either they do it or they don't support.
Speaker 1:It's a good question I don't know um, but they do allow for the the modification type, so like if you've added but also modified, and so I would assume that is also revertable, but I don't know how to implement it yeah, I've seen.
Speaker 2:So this is a way of versioning your data, right? Uh, I've seen other ways, like, I guess very simple, if you have a database, is is just adding a timestamp. So here I think it's actually nice because you do have a timestamp, but you have something more rich which is a commit hash, so you also link it with with a git history right.
Speaker 2:I wonder how this feels like something could be very nicely integrated with dbt, because dbt is already on git, right, and every transformation you do. And then it would be very easy to say, okay, this row was created by this version of my dbt project. Right, because I think today dbt have the, the timestamps and whatnot. But if I want to see, okay, this row was created by this version of my gil repo, I kind of have to match my timestamps with my dbt timestamps, I guess I don't think you get that out of this, naturally, because, like the commit hashes that you get in bolt are really like they're scoped to dolt.
Speaker 1:It's like a different it's like a different thing, like you, would have to add extra metadata to these rows to do this there's a, there's an idea there for the people abt, then linked to the kid looking yeah, yeah, um, another thing that I've seen for data versioning and when I when I'm familiar with data versioning, there's a dvc data version control.
Speaker 2:so actually I put that on the notes here, but it's actually very different now that you explain it. What is DVC? Dvc is also for data versioning, but I think it shines more when you have unstructured data. Dvc is way more tied to. Are you familiar with DVC Bart?
Speaker 1:I use it, but it's been a while. Yeah, DVC is way more tied to. Are you familiar with DVC, bart? I use it, but it's been a while.
Speaker 2:Yeah, dvc is very tied to Git actually. So like all the files that are DVC tracked actually don't go on, they go into, like they Git, ignore these things, so they don't go on your Git repo. But there is like a file with basically just a hash, so basically like an identifier, and then whenever you do so, the commands are very similar to git. They really mimic git for these things. So when you do git push you can do a dvc push, you can do dvc pull and basically when you do dvc pull they will look at the id file, the id proxy file from like that would reference a version of your data set and then you pull every stuff in so that's basically meant for data on your file system, whether it's a physical or a virtual like s3 or something, but it's like yeah it's not like this data in this structured no postgres database so it's really just like five.
Speaker 2:So I mean, yeah, you could bridge that by saying you have parquet files locally right but it's.
Speaker 2:I think it shines more when you talk about um images or all these other things, right, I think. Then it's really, really, really good. The downsides of dvc is, um, if you cannot hold, you cannot have even not even one version of your data set in your laptop, then it's a bit, it goes a bit downhill from there. Okay, in my opinion, but it's a very, very, very cool tool. I think it's been there for a long time. And the other thing too, that now this, uh, the company called iterative um, they also expand this a bit, right. So, um, if you think of machine learning models, if you say that they're like pickle files or whatever, it's a file as well, so you can also version machine learning models with this. So they have um like other tools.
Speaker 1:They have dvc, studio dvc oh, damn, and I'm looking here at the you just had to hit a page on. Yes, they have less stars than dolt. Well, I think almost everybody in the data slash ai space has at some point heard of dvc.
Speaker 2:Yeah, that's true, that's true, it's crazy.
Speaker 1:Maybe there is a space that I'm not part of where DOLT is very big.
Speaker 2:Maybe, Maybe, maybe maybe.
Speaker 1:Interesting.
Speaker 2:Indeed and also DVC. They're part of Iterative I want to say but even though I cannot find it, but, like I said, they also have a version of model registry, experiment tracking. They even have pipelines and stuff. But everything is very tied to stuff, but everything is very tight to git and everything is very tightly integrated. So they're good, they're good use cases for it. But, yeah, it's true, they have less stars than, uh, than your adult thing. So curious, and while we're still, maybe, veo yes, veo was. What is it?
Speaker 1:Bayerd Was announced at Google IO earlier this week One of the cooler things in my mind to come out of it. So Google IO happened just the day after the opening ice announcement of GPT-4.0. Really day after, uh, after opening ice announcements with gpt4o really, and uh, it's interesting to like, it's too, I think, like I think, opening I scheduled it on purpose.
Speaker 1:Yeah, yeah, yeah I think it's interesting to to see a bit like different style as well, where, with the eye, when they announce something, it's more or less always like a cohesive product like this is ready to be used, yeah and like with google io, it's always like there are a huge amount of projects and they're all very cool, but it feels like like a huge amount of engineers sitting together for a year and just having like like a hackathon and like a ton of cool stuff came out, but like, what of that is like actually a cohesive product? Yeah, what would you and what will not be X'd a year from now?
Speaker 2:that's always a bit like the feeling that I have in Google IOS. Google built this reputation as well of X'ing a lot of stuff.
Speaker 1:No, build this reputation as well of xing a lot of stuff. No, yeah, yeah, but vio that you have on the screen now. Um, vio, right?
Speaker 2:is it vio? Vio, I think with the e, is it?
Speaker 1:the middle knee. Okay, vio uh they call it uh their uh most uh capable uh video model generator, video model, and it's a 1080p video model. Uh, I wanted to try it out. You can sign up to try, but it's not available in Belgium, so I assume not available in Europe, maybe only available in the US. But when you go to the page which we'll link, you see very impressive videos, but like very impressive. All of them are more or less I would say it's like they're. They are a bit of a landscape ish, like a bit or a bit of abstract but realistic.
Speaker 1:Uh, but it doesn't show like, for example, I'm walking through a city and you see tons of people where you would expect more artifacts yeah but all of the ones that they show look super, super impressive yeah but I would be interested to see how well does it perform with like a noisy environment you know, for me, I mean, I agree, it's very, it looks very nice, it's very nice this is also.
Speaker 2:we're showing the video here like it's a wheel of snippets, I guess, and if you go down they have some more. I don't know for longer examples, but they have the prompts as well. So a lone cowboy rides his horse across an open plain at beautiful sunsets of light, warm colors, and so you go. The thing for me is and I do notice now that I have a bias, because with Gemini, they released a very impressive demo and then there was a lot of kerfuffle that the demo wasn't, you know, it was highly edited. And I'm also wondering here okay, these things are very short, right.
Speaker 2:I'm wondering how much quote-unquote makeup it has. You know, to make this look very Like, did they try a million things? And now they just kind of put the top five, which they've been, you know. The other thing too is that, um, it always feels to me that they're what they're a few steps behind the opening eye, like soar was released and it was like whoa, and I feel like now we're going through this again, but it's not the same wall, because it's the second time um, yeah, yeah, I agree.
Speaker 1:At the same time, what? What they're showing here, like the short snippets? Yeah, the first, they look very impressive. I think even next to sora they're still impressive no, that I agree that, but I have the same feeling like I would like to try this out and see how wow it actually is.
Speaker 2:Yeah, that's the thing after the gemini demo. It's really like, you know, yeah, the gemini demo look amazing, and then afterwards it was like yeah, no, this is uh. It was not like that at all, because even I think on the sora announcement they also had a few examples of things that didn't work so well, or a few clunky things, you know, and here I don't see anything at all like I'm really trying to, for example, indeed, the the sora documentation on the website was also very honest, like this is here.
Speaker 1:This is where it's not good at these are artifacts that get generated, which felt a bit more like, felt a bit more transparent. Maybe transparency is a difficult word to use in the context of open ai, but it felt a bit more transparent about how performant the model actually is it feels.
Speaker 2:I mean, to me maybe transparent is not the word, but maybe believable in my opinion. You know it's like because you're highlighting a bit the the downsides. Let's say I do feel more inclined to trust. Maybe just to compare here, this is the sora video that we saw a little while ago and they had some examples at the bottom, I think yeah but, yeah, putting them next to each other.
Speaker 2:I think here, not sure what was it out here? Yeah, they had some funny stuff, like the the guy running the treadmill or the puppies that just appear, yeah, I see. So, in any like, agree, they're both very impressive, but I think, uh, I would wait a bit before losing all my marbles again around around this. Let's see, let's see. Indeed, um, let's see.
Speaker 1:indeed, should we go for the hot topics hot topics maybe before that, a lasting open AI? In the news, I think yesterday, where it was announced that Ilya subscriber resigned together with another, which I forgot the name of, with Jan Leike, who ran the Super Alignment team Sud Skiver is a bit has been named the brains of OpenAI at some point, I think. Ilja. Ilja yeah, there's also been. He's been very much involved in all the hassle with Altman being fired and then coming back on board, stuff like that.
Speaker 1:Hustle in which sense, like he was supporting Altman or Well, what I understood is that, at the beginning, uh sudskipper was uh instrumental in getting altman fired. Actually, ah, really yeah, but I think all the information that came out was still very much hush, so I don't know what exactly happened. Um, but the reality is uh that six months later, we have ilia resigning and uh wondering to see what the impact will be on the ability to innovate, if any right if it will hinder the ability to yeah, yeah, yeah let's see yeah.
Speaker 2:So right now we're just kind of like just caught our attention, but there's no necessarily confirmation that this is a crisis within OpenAI. We don't know exactly why he left.
Speaker 1:It's not a crisis. We don't know exactly. I think his statement was that he's going to work on another project, that he has a big personal connection to something like. I'm paraphrasing a bit here, but let's see if it becomes a crisis, because he is, of course, one of the one of the figureheads of opening yeah, indeed, let's see, let's see, let's see, let's see. And with that are we ready for some, uh, hot takes oh, hot, hot, hot, hot, hot, hot, hot, hot, hot, hot, hot, hot hot.
Speaker 2:Nice, I brought a hot take. This is not my hot take. I mean, let's see how hot it is actually. Yeah.
Speaker 1:I don't know what you brought.
Speaker 2:Maybe we should have like a spiciness scale, you know like, how hot is it? And then you can, you know, see, like it, and then you can uh okay you know, you can see like oh, I think this is a hot take the way by, say oh yeah. Last time actually was like that. I was like oh yeah, but it's obvious how many out of five peppers? How many are five? No, but they're like different spiciness of peppers no, isn't it?
Speaker 1:oh yeah, what's it called again?
Speaker 2:yeah, the something like the thousand and the millions yeah, I don't know, but that's the spiciest one no, no, it's like the the, the measure of the measure of spiciness okay, we'll come up with something.
Speaker 2:Skill. But so what is this about? This is an article that I came across. It's about data engineering, more specifically, data engineering roadmaps. So, first thing, the big claim here is that there is no data engineering roadmap. The author apparently he was a bit frustrated. Maybe frustrated is not the right word, even though he does have a rant here at the bottom, quick rant with peppers here, so I guess it is hot. Um, he was tired of seeing those. The roadmap to data engineering first you need to learn this, then you need to learn that, then you need to okay, like that's okay.
Speaker 1:Yeah, I think what is the roadmap?
Speaker 2:to become a data engineer? Okay, but what did you?
Speaker 1:what was your understanding before I said I taught him a roadmap within a company on how do we do data engineering.
Speaker 2:No, no it's like for someone like you want to be a data engineer, okay, what should you learn?
Speaker 2:And then he was seeing this like, okay, first you need to learn this, they need to learn that, they need to do this, then kubernetes and this. And he was a bit like no, and he also goes even as far as saying that a lot of people that do these things, they have ulterior motives because they have these courses and they try to get people to subscribe to it. So maybe do you feel, do you believe in these roadmaps, or do you think it's like you just need one or two things and then you're done. So, just to be fair as well, in this article he does say that you need a foundation. It's not like he's saying that you don't have any requirements. There are requirements and we'll get to his main requirement, or only requirement, actually. But he said it's different to say these are foundational skills and these are additional skills than to say this is a roadmap that you need to learn the A, b and then C and then D, and then is this do you agree with that?
Speaker 1:um. I agree you agree. What do you say? Yeah, I think um to me that's the danger of stepping on some toes here but that's why we have this to me data engineering is similar to software engineering, but with a focus on data. Yeah, and that's that's like. If you have a you studied software engineering you're ready to start your career as a junior data engineer. Yeah, that's that's what I feel and, like all these data specific things, you will learn along the line yeah, no, I actually agree with that.
Speaker 2:He even said, like you said uh, he I have. I have seen some say data engineering is not an entry-level role and this is nothing more than toxic gatekeeping. It's a very strong language here. But I agree as well, like you said, junior data engineers. He said that he's heard people say that there's no such thing as a junior data engineer. He even compares as well to software engineering. That before people used to say software engineering is not an entry-level role, but now people don't say this anymore because they know this is rubbish, total rubbish according to him. And he says everyone is welcoming data. So that's one thing. Not sure how hot it is. I tend to agree as well. This is a bonus hot take from reading the article. All you need is SQL.
Speaker 1:Yeah, that touches a bit on another topic.
Speaker 2:of course yes it's a bonus hot take here. Yes, it's a bonus hot take here.
Speaker 1:Well, I don't think all you need is SQL, but SQL is becoming the 80% again. Yes, SQL, SQL In terms of trends. So what did you see? It's like, if we look at 10 years back? Everything is in SQL.
Speaker 2:Yep.
Speaker 1:Talk directly to databases at some time, at some moment in time, like larger data sets, big data sets became a thing. These traditional databases could really handle the aggregates, like the analytics type of queries that we launched via sql. So we got other things to handle these analytical workloads, like Hadoop, like these type of things, and the way to build data or to build analytical queries on top of this, to build analytical products on top of this, was very much software engineering, like it wasn't Python, it wasn't Scala, it wasn't whatever. And now we see a bit of a shift back where there are very good analytical databases where you can go very, very, very far with just sequel yeah, so sequel is becoming way, way more important again yeah, I think also with dbt, right, like it's a bit sequel plus plus, let's say, uh, we can do a lot with SQL.
Speaker 2:But even DBT, right Like a lot of these DBT also have Python models, right, which you can like kind of blend in a bit. So I agree, I agree with what you're saying, yeah, but that's not what he's saying. Well, one thing also he says is when people are learning as well, and he says at some point here that's like just learn, just pick any sequel. A sequel has different dialects, it's not very standardized, but just pick one, it's fine, don't don't worry about which one. Yeah, like it says nc sequel, which is not really a standard. Uh, just pick one. If you really need to pick one and you're really stuck, just go for poschris. So, uh, I think you'll put a smile on your face, bart.
Speaker 1:Yeah, I think this calls for an applause.
Speaker 2:So now you know. If anyone wants an applause in the Data Topics Podcast, just say Postgres and Bart will.
Speaker 1:I think the description is wrong. It says it's the world's favorite free open source database. I think you should say it's the world's most robust favorite free open source database. But this is great. It's the world's most robust favorite free open source database.
Speaker 2:But this is great. You can't go wrong with starting with postgres. I agree that in most cases one case. And then he says yeah, there's some differences, and then if you want to change the database you can marry the big was, big query, blah, blah, that I'll agree. Um, if you already know sql, then like yeah, you're in a good position. But then he goes what's next? What about python, pandas, dbt, rust, airflow, spark later, all these things you can learn on the job if the job even needs them. That I'm not sure, if I fully.
Speaker 1:I mean you can say that about even sql but I think that is a bit like what kind of job are you aspiring to?
Speaker 2:as a data engineer?
Speaker 1:because I think a data engineer, because you see these evolutions like, depending on where you're gonna to as a data engineer. Because I think a data engineer, because you see these evolutions like, depending on where you're going to work as a data engineer, it can look very different where you still do a lot, for example, on daily, daily basis with uh by spark, or you don't do anything at all anymore.
Speaker 1:All the foundation is set up, csd is set up for you by another team and you just write sql like. You have these two extremes. So I think in 2024, it still makes sense to have a good understanding of more of these software engineering type of principles and language understandings. And if you need to choose a language today as a software engineer, it's Python, but I think also there, like we've seen this, evolutions at universities as well. So someone that studied software engineering would have seen Python, would have used Python, and it's perfectly suitable to start as a junior data engineer with his Python skills.
Speaker 2:Yeah, no, but I agree with that, I agree. I do think it's like if you're a data engineer and you don't know SQL, then I would raise an eyebrow, and if you're not good at Python, so you know some, I think it's fine. I do expect you to know the basics of Python. Like if you saw Python code, you would kind of, oh yeah, this Python code, I can kind of really understand that. I would expect a junior data engineer to know. But I agree, I agree with that.
Speaker 1:I would, at the risk of of of stating another hot take someone starting as a junior data engineer. If this person really shows like has a strong foundation in Python but doesn't know any SQL skills, I would have more trust in that person than someone that shows that a lot of SQL but doesn't hasn't touched any other language yet Can we press the hot hot hot again. Oh, hot, hot hot hot, Hot, hot, hot, hot, hot hot.
Speaker 2:No, I agree with you, but again, I think we discussed this in the past I feel like Python is a general purpose programming. I feel like it's a bit more.
Speaker 1:I think SQL. It's easier to get in touch with sql via a lot of different fields. Yes, because the the primary analytical language. Yeah, um, python or another language you only get acquainted with if you do a bit more low level stuff, and I think it's an indicator that you know a little bit more about software engineering practices.
Speaker 2:Yeah, if you're good at one of these languages. I think it's like easier to go from python to sql than sql to python. Yeah, in the same way that I think and maybe this is another hot take as well I think it's easy to go from data engineering to data science, then data science to engineer. Actually, I don't think that's a hot topic, but uh, a hot take. But I Wait, wait, tell me again, I think it's easier to go from data engineering to data science than data science to data engineering.
Speaker 1:I think a lot of people will. We're going to leave this as a hot take for the next time.
Speaker 2:Okay, Maybe to wrap up this. I think it alludes to what you mentioned before. On the article. They say SQL is the only skill that every single data engineer uses every single day. No other skill or tool can claim the same. Python is cool. Some folks are using Scala. Snowflake is popular, but there's more data teams not using those tools than those who are, but not for SQL. I'm not sure if I agree with the. There's more data teams not using those tools than those who are, but not for sql. I'm not sure I agree with the. There's more data teams not using those tools than those who are, but I think that's a very that paints a picture on like where, what is the environment that this person has been in?
Speaker 1:yeah, indeed, I also don't think this is very much a blanket statement. I don't believe in this I do feel like.
Speaker 2:So again, I maybe a roadmap is a very strong word and I think a lot of these roadmaps are very detailed to like kubernetes and this and this and that you know like even technology is like not very general purpose things. But if I'm at a university and someone says, oh, I want to be a data, a data engineer, and I still have like two years to go my studies, what would you recommend me doing? I would say, well, sql for sure, and, I think, python. I would tell them like, if you know python and sql, you're in a very good place. So that's again. I would go a bit further. I wouldn't say, sql is all you need. I mean, yeah, it's all you need, but that's not what I would advise you to.
Speaker 1:To go but what this person also been reacting to is all these people selling their courses and but I must say that that irks me a bit as well, but I don't think that's uh specific to this field. Like if you open youtube, the first ad you get like I have been successful in this field for 10 years and now financially independent and I'm here to give you advice. If only you pay me two thousand dollars then I'll give you some insights you don't need a college degree, you know, just pay me 2000 and you get your dream job, you know.
Speaker 2:So yeah, and yeah, this is the quick run to the bottom here. He says a lot of bad advice. He says that some people are just innocently sharing their opinion, but there's a lot of people that are really trying to have a get some money out of it, right? So Believe in yourself, sure. Believe in yourself, sure, sure, and I feel like sometimes we as people, we're really trying to find the optimal way, like you're trying to optimize your efforts, but I really feel like sometimes you just gotta do it, you know, like don't I think we talked about this in the past but like, for me, even like exercise, right, it's like I go work out, I go for a run, whatever, it doesn't need to be the best run, but just go every day, that's fine you know, even if you have a bad day, it's better to have five bad days and have one good day this became very, this became meta very quickly
Speaker 1:yeah, it did, I like that but I agree like uh, even if you're not 100, sure just try it out. Uh, if it doesn't work, try something else, try to improve.
Speaker 2:Exactly, just see what sticks, you know.
Speaker 1:That's why we try every week at Data Topics.
Speaker 2:And with that I think we can wrap this up. Anything else you want to say?
Speaker 1:Thanks everybody for listening.
Speaker 2:Ah, maybe so we're recording on Friday because I'm going on holidays next week. Monday is a holiday as well as a bank holiday in Belgium, you have any special plans?
Speaker 1:Uh, not really, but you have special plans.
Speaker 2:I have. I'll be in Portugal. Yeah, I have some errands to run there, but also I'm going to do some tourism and towards the end, the very end of the trip, I'm going to have a I have a very important commitment appointment, which is the main objective of the trip. Right, I don't know if I would say that, but yes, I am going to be attending a Taylor Swift concert, so probably I wouldn't call myself a Swifty. But, I've understood that you can sing along with every song. Yeah, pretty much, pretty much.
Speaker 1:Not every song maybe, but like how did this get into your life?
Speaker 2:I mean.
Speaker 1:But so first thing is, like, taylor schiff has been prolific, you know, for a very long time, so I even remember but what was the first like if you go back in your life like what was the the like, if you go back in your life like what was the moment that?
Speaker 2:you became a Swifty. I wouldn't say I'm a Swifty, but I do remember. So, like yeah, oh fine, I'm proud of it still. I remember in Brazil I was still in Brazil, I was learning English, right, and I remember we had this like after lunch. So I would come back home eat lunch and there was this thing there was only video clips of the popular songs and they put subtitles in portuguese. And I remember watching, uh, taylor swift's uh, what's the name of the love story, so you know. And then like I remember like the whole story and this, and really says, oh, that's what she said, oh, that's this, oh, that's you thought like this is deep, this is meaning, yeah, yeah it was like, wow, what a poet.
Speaker 1:And that was the defining moment. No, that moment led to you going to Portugal next week.
Speaker 2:I feel like, if it's a bit dangerous, because I feel like I would usually play along with the joke, but I think a lot of people are not going to understand that this is a joke, so I'll just nip it in the bud right now. No, I don't. I wouldn't consider myself a swifty, but I feel like I do know a lot of songs. I do feel like it actually helped me learn english a lot.
Speaker 1:Okay, so, um, would you consider yourself a closeted swifty? Yes that's maybe on that note you know, you know paramore is actually opening.
Speaker 2:You know paramore. You know paramore, bart is no paramore a. You know Paramore, bart doesn't know Paramore. Alex knows Paramore. Yeah, you don't know Paramore, it's another. I mean, it's also something that I grew up listening, so it's going to be something. Well, I grew up listening, maybe Alex, maybe I don't know, maybe Alex as well. What do you? You have any big, any special things, anything you want to share? Uh, I'm going to spain, nice not bad, not bad.
Speaker 1:You will all be enjoying a lot of good weather yeah I'll be here in belgium in the rain yeah, I'll think of you maybe enjoy uh particle, enjoy taylor. Swift, alex, enjoy Spain. Thank you All right.
Speaker 2:Thanks everyone.
Speaker 1:See you next week. Ciao, ciao, you have taste in a way that's meaningful to software people. Hello, I'm Bill Gates. I would recommend TypeScript. Yeah, it writes a lot of code for me and usually it's slightly wrong. I'm reminded, incidentally, of Rust, rust.
Speaker 2:This almost makes me happy that I didn't become a supermodel.
Speaker 1:Huber and Ness. Well, I'm sorry guys, I don't know what's going on.
Speaker 2:Thank you for the opportunity to speak to you today about large neural networks. It's really an honor to be here Rust Rust Data topics.
Speaker 1:Welcome to the data. Welcome to the data topics podcast.