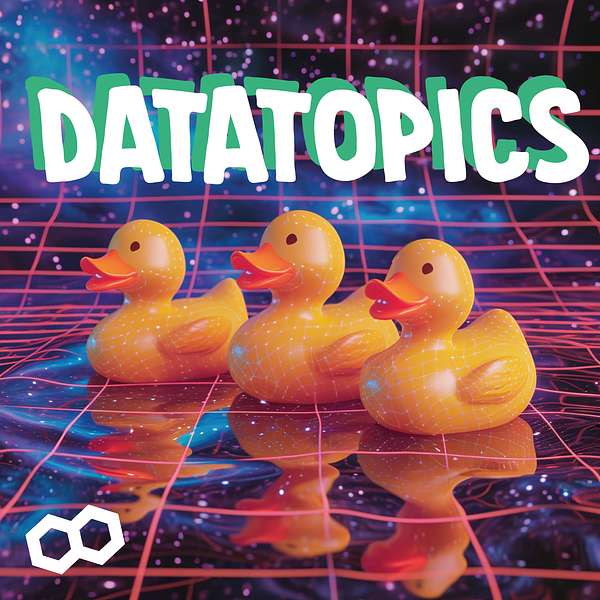
DataTopics Unplugged: All Things Data, AI & Tech
Welcome to the cozy corner of the tech world where ones and zeros mingle with casual chit-chat. Datatopics Unplugged is your go-to spot for relaxed discussions around tech, news, data, and society.
Dive into conversations that should flow as smoothly as your morning coffee (but don't), where industry insights meet laid-back banter. Whether you're a data aficionado or just someone curious about the digital age, pull up a chair, relax, and let's get into the heart of data, unplugged style!
DataTopics Unplugged: All Things Data, AI & Tech
#79 The $6 AI Model? France’s $85B Bet, DeepSeek's Censorship & The Python Upgrades You Need
Welcome to the cozy corner of the tech world where ones and zeros mingle with casual chit-chat. DataTopics Unpluggedis your go-to spot for relaxed discussions around tech, news, data, and society.
Dive into conversations that should flow as smoothly as your morning coffee (but don’t), where industry insights meet laid-back banter. Whether you're a data aficionado or just someone curious about the digital age, pull up a chair, relax, and let's get into the heart of data, unplugged style!
This week, we break down some of the biggest developments in AI, investments, and automation:
- France’s AI Boom: $85 billion in investments – A look at how a mix of international and domestic funds is fueling France’s AI ecosystem, and why Mistral AI might be Europe's best shot at competing with OpenAI.
- Anthropic’s AI Job Index: Who’s using AI at work? – A deep dive into the latest report on how AI is being used in different industries, from software development to education, and the surprising ways automation is creeping into unexpected jobs.
- The $6 AI Model: How low can costs go? – Researchers have managed to create a reasoning model for just $6. We unpack how they pulled it off and what this means for the AI landscape.
- AI Censorship & Model Distillation: What’s really going on? – A discussion on recent claims that certain AI models come with baked-in censorship, and whether fine-tuning is playing a bigger role than we think.
- PromptLayer’s No-Code AI Tools – Are no-code AI development platforms the next big thing?
- Predicted Outputs: OpenAI’s approach to efficient code editing – A look at how OpenAI’s "Predicted Outputs" feature could make AI-assisted coding more efficient.
- MacOS System Monitoring & Dev Tooling: The geeky stuff – A breakdown of system monitoring tools for Mac users who love to keep an eye on every process running in the background.
- Snapshot Testing with Birdie – Exploring the concept of snapshot testing beyond UI testing and into function outputs.
- BeeWare & the Python Ecosystem – A look at how BeeWare is helping Python developers build cross-platform applications.
- Astral, Ruff, and UV: Python’s performance evolution – The latest from Charlie Marsh on the tools shaping Python development.
You ready, I'm ready. You have taste In a way that's meaningful to self-loving people.
Speaker 2:Hello, I'm Bill Gates. I would recommend TypeScript. Yeah, it writes a lot of code for me and usually it's slightly wrong. I'm reminded, incidentally, of Rust here, rust. This almost makes me happy that I didn't become a supermodel. Cooper and Nettix. Well, I'm sorry guys, I don't know what's going on.
Speaker 1:Thank you for the opportunity to speak to you today about large neural networks. It's really an honor to be here.
Speaker 2:Rust Data topics Welcome to Topics. Welcome to the Data Topics Welcome to the Data Topics podcast.
Speaker 1:Hello and welcome to Data Topics Unplugged, your casual corner of the web where we discuss what's new in data every week, from Stargate competition to birdies, anything goes. Check us out on YouTube, your favorite podcast player. Feel free to leave a comment or question, or reach out to us via email. Today is the february 11th of 2024, uh, 2025. My name is morillo. I'll be hosting you today, joined by bart hi, hey, bart, and behind the scenes, as always, alex hello, hey, uh. How are we doing?
Speaker 2:good weekend good weekend, quiet weekend quiet weekend.
Speaker 1:Okay, nice, nice, nice. Um, I keep waiting for the weather to get better. But then it snowed again last night and I was like what like? We had two days of sun last week yeah, right, but like like the days are getting longer and I expect the weather to, you know, follow suit, and some days I'm like, ah, maybe it's gonna be warmer, but then yesterday there was no and I was like thrown off completely.
Speaker 2:But, um right, I think this is still your brazilian fame. Maybe right.
Speaker 1:It doesn't apply in belgium I'm still like putting my flip-flops tank top just like getting outside, ah no okay, that's.
Speaker 2:The hope never amounts to something.
Speaker 1:Yes, yes, indeed, indeed. So what do we have for this week? I see here. I think this is very fresh news Investments in French AI ecosystem reach 85 billion, as Brookfield commits to 20 billion. What is this about, Bart? This is from two days ago.
Speaker 2:This is from two days ago. This is from two days ago. It's a TechRange article. It's that investments in the France AI ecosystem reach up to 85 billion, with a recent commitment of Brookfield. I think it's a venture capital fund. I would say I think it was interesting just to mention because we were discussing, uh, the other day that, like we see a lot of these investments, we had a stargate project like this follows a bit in the search of the stargate project, um, but we don't really see these, these amounts in europe. But I think france is proving us wrong there we go, there we go.
Speaker 1:How does it work actually? Can France just invest this? Does it have anything to do with the EU? Does the EU have to play a role in this?
Speaker 2:Well, I don't think it's France. Actually, this is a consortium of things and I think together they amount to 83 billion euros. I think there's 50 billion from the uae for an ai campus. There is 10 billion from bpi france, which is france's national investment bank, going really to ai startups. There's also 3 billion from iliad for ai data centers. So it's really like it's a it's it's spread across a lot of different initiatives and it's definitely not only just french capital that is coming in, but it is landing in the french ai ecosystem and also, am I correct because I know in in france they have mistral, which is uh I think their headquarters actually in paris yeah yeah, and I think that's the biggest one in the eu, right that they have the big, these large models I think it's arguably the strongest LLM competitor coming from the EU.
Speaker 1:Yes. Does this have anything to do with Mistral? Do you know?
Speaker 2:I don't think that there was a mention of Mistral in the article, but of course, if you have something like Mistral, it's strong for the ecosystem right.
Speaker 1:Yeah, indeed indeed, but I'm sure that they're involved somehow, Right? I see here some I just control F did. Bpi friends is already a shareholder in mistral, so they have similar interests, but there's no maybe direct link there. Cool.
Speaker 2:I think cool, I think it's a yeah, I think there is also a bit like, like, like, like you can debate a bit how good miss role is in the in the global ecosystem, but like missile is definitely a competitor in europe. And I think also they're like talent attracts talent that is true that's what we see here and apparently talent also attracts capital yes, yes, that's true, that's good, I think it's.
Speaker 1:Uh, yeah, I think also now the only thing, because we're talking about the differences financially and then we also have the differences in terms of regulations. That's still to be seen, but it's good to see that there's movement. Yeah, right, so looking forward to see what's going to come out of that, and sure you're going to keep us posted.
Speaker 1:What else do we have Still on going to come out of that, and sure you're going to keep us posted. So, um, what else do we have still on the ai big companies? We have the entropic economic index interesting. What is this?
Speaker 2:yeah, this is an article by entropic. There's also a pdf, but I think the euro. You've opened the website. I think the gist of it is on the website. It's interesting to scroll through. It was released, I want to say, two days ago, um, something like that, and it's uh, it's an analysis of uh people using the free cloud and the pro cloud, um versions of the like, their chat interface and um, basically an analysis. They, they used a tool called uh I think it's called clio c-l-i-o to, in a quote-unquote, anonymous way, analyze in what domain you're using uh. You're using your, their tools. So, are you querying something about software development or are you querying something about, let's say, something about pharmaceuticals, whatever? And what they did here is to try to come a bit with a index to see, like, in which domains are these tools being used a lot, which I think is a very interesting analysis what did I see here?
Speaker 1:so computer, mathematical, 37, arts and media, 10 education%, education and library. What is the educational library? Is it just like, I guess, books and stuff.
Speaker 2:Well, education, I think, speaks for itself, it's an education system within libraries.
Speaker 1:Okay, 9%. And then we have office and administrative Curious what this is. And then we have life, physical and social science.
Speaker 2:60 percent in business and financial, yeah, and I think then, for the people just listening, they have top titles and top tasks for each one of those I think the interesting thing is, like it's and I think it would probably like a bit confirm our suspicions as well as it's like the biggest amount of usage is actually computer mathematical tasks. There you see a lot of AI interaction 37.2% and that over one third of occupations use AI for at least 25% of their tasks. Say that again Over one third of occupations use AI for at least 25 of the tasks.
Speaker 2:okay, like for all your tasks yeah 25 you're using ai in one way or another, like not necessarily to automate, but like, like as a tool. Yeah, um, and then also like a thinking a bit more about ai as a replacement versus enhancement. And what they analyze is that 57% of AI use is augmentation, so collaborating with humans like making yourself more efficient. 43% is automation, like directly performing specific tasks. But that is not necessarily replacing roles, right, but automating specific tasks.
Speaker 1:But very few jobs today only 4% use AI for more than 75% of their tasks. Only 4% use more than 70% of the task.
Speaker 2:So basically, only 4% of the people are at risk for being replaced.
Speaker 2:Well, I think that is to be debated right Depends a bit how much uh, how much uh autonomy you need. For the rest of that, there's also an interesting uh uh graph. If you scroll down a bit lower, it's a bit it uh, it uh. You have there on the x? Uh axis the wage no, a little bit down or up, I don't know, to be honest, this one uh no, yeah, this one. So you have uh, the medium wage on the x-axis and the percentage of conversations. So they basically analyze, like, what is your, what job do you probably have? What is the medium wage linked to that job, based on the conversations you have with?
Speaker 1:they don't know for sure. They don't know for sure you could.
Speaker 2:you could do this as a hobby, right? Yeah, I see you could ask some things about Python, but you're a Brazilian masseuse, right?
Speaker 1:You don't know. You don't know what I do in my spare time, Bart.
Speaker 2:An interesting thing here maybe also is that mid to high wage occupations show the highest AI usage. Interesting to see. But that's also because computer programmers, software developers, so the ones that you would expect are also in that class, of course, like a bit too high. And there you see the very technical jobs. There is also a highlight it's somewhere that I didn't know that, like this was a dedicated job. Um, it's at the lower end of the median wage and also the lower end of the percentage of conversations.
Speaker 1:There is shampoos, shampoos hey, break it down for me what is that?
Speaker 2:I don't know? Like, like. Is it someone that just whole whole day long like is shampooing stuff?
Speaker 1:I'm uh, I'm gonna refer back to alex. I think you have a good guess. What is a shampooer?
Speaker 2:I have no idea.
Speaker 1:I saw it and I was gonna ask you is it things like someone that washes people's hair, like a hairdresser or something? But I would then think it's like a hairdresser, right, yeah?
Speaker 2:shampoo's like a fraction of the time. Yeah, right, but this is someone that is constantly shampooing and they use a bit clod.
Speaker 1:So and they use a bit like maybe to get to the best consistency of bubbles, yeah, right, or like how, yeah, interesting, interesting and maybe also this is uh. So in this analysis, they had, they had the history of uh questions per account, I guess, okay, okay and I'm also a bit worried about the other end of the spectrum.
Speaker 2:Colleges like obstetricians and gynecologists uh, they don't take a lot of big percentage conversations but they do, like you see, some interactions there, right, like on the very high end of the medium-range scale. Like to me that's a bit worrisome. Yeah, right, it's like.
Speaker 1:Yeah like what are you asking there? You know, this is like what do you?
Speaker 2:need? Yeah, yeah, like like. For what type of questions are they like like trusting cloth, right? Yeah, indeed, it's a, it's a bit uh, yeah, curious.
Speaker 1:I'm going to do another poll on that. Just zoom in on the graph, you know. Okay, interesting, and maybe also you mentioned the beginning. This is, uh, the free versus paid. No, no, the. This is and the free plan and the pro plan did they make that and they make a distinction between the two like I don't think so. I don't think so I think.
Speaker 2:I think what they just excluded is the enterprise plans, because there's no, if I'm not mistaken, there's no data retention there okay, okay, okay and maybe with this, it actually makes me wonder how they classified me in this yeah, maybe you're the gynecologist there, barton or I'm the shampooer or the shampooer?
Speaker 1:no, but I think it's too low, but it's just like somewhere, you know, it's like this guy anywhere between shampooer and gynecologist somewhere.
Speaker 1:Just put it right in the middle there. Um, okay, cool. But I think there's a lot of cool graphs here, like the augmentation versus automation and all these things. Uh, maybe makes me think of and then I know there's a lot of hype and it almost hurts me to bring it up but like the agents stuff, they the I think the one of the reasons why agents are attractive because of the promise that it will be more automation, right, it will be able to do things more autonomously. And do you think that, like right now, we see, I don't know, I think we said like 57% was augmentation, meaning that there's someone there that validates.
Speaker 1:I think they have a graph here that I'm showing that they break it down more between validation, task iteration and learning, and then on the automation part, there's feedback loop or directive, and I guess feedback loop is say do this. And then you come back and the LM does something. It's like, okay, no, do that instead of this, or change this, change that. So that's, I guess, is the feedback loop. And then the directive is just saying go and book a meeting and then it just kind of does it in the end and it's over? Um. Do you think by the end of this year this will change a lot, with the hype around agents and all these things? Um, because I think that's a bit what people want, right, they want to automate more well, they want to automate more.
Speaker 2:Well, they want to automate more. Yeah, I think it depends a bit like how easy can we get these tools like Cloud or ChessGPT to interface with all the APIs of the tools that we use?
Speaker 1:Yeah, that's true.
Speaker 2:Because that's a bit like. You probably use like 5 to ten different SaaS offerings every day for a variety of things.
Speaker 1:Yeah.
Speaker 2:And what you want to say is just okay. I want to book a meeting with Marilo. Make sure to block my time in that other time scheduling thing that I have and also send them an invite. Oh, it's remote, so include something or find a like a physical spot for us to meet and book the meeting room, like, like these type of things. But it also allows a certain maturity on all of the apis that you need to interact with and then also a certain robustness and making sure it doesn't fuck up yeah, right again.
Speaker 1:I'm not sure if like it's, it's a.
Speaker 2:There's a lot of different variables, variables playing at the same time. There, I think, we will definitely see a lot of steps towards it.
Speaker 1:Yeah.
Speaker 2:But maybe, like I think, it will still improve majorly after this year, right?
Speaker 1:Yeah, yeah, no, I think we're going to continue improving, indeed, and yeah, I'm also not sure if how much, because I mean, sometimes APIs are not available as well. It's not not like for one reason or another, it's not. It's not easy to integrate, to plug these things in yeah and that's why we also saw the other.
Speaker 1:I think we talked about, like that, the thing that takes screenshots of your desktop and all these things to try to go around it, right, but yeah, but yeah. I think it's hard to like, but you haven't quite answered. Do you think this will by the end of this year? Do you think this will be something that everyone will be doing? This would be the next big thing, or do you think it's more hype than anything right now?
Speaker 2:um, I don't think everybody, but the early adopters definitely okay.
Speaker 1:But then it was like so yeah, I see what you're saying like maybe we take a few more years, yeah and I think it depends very much on the maturity of APIs.
Speaker 2:And I think like, if you like, extend it, like the question like what will it do to the SaaS ecosystem? I think it will heavily disrupt our SaaS ecosystem, but because why do you need an interface to all these things? It gets automated for you, that's true. But at the same time, like I think a lot of the more legacy or more corporate b2b, uh, things are also safe for the coming five years because it's much harder to interrupt as long as you're not.
Speaker 1:I'm sure that what is done is correct and robust and, yeah, I also yeah, the accountability of tasks, right, like if that line up, who's gonna answer? Right, there's still, we still, even as an organization, we still need that accountability chain.
Speaker 2:Yeah. So it's like yeah, but I think it also depends very much on, like, how far will these interfaces like Cloud, like Chachipity, like others like, help us integrate these things right.
Speaker 1:Yeah true. I think, yeah, we mentioned two weeks ago that OpenAI released. I think they call it operator or something that also kind of goes through, yeah so so, yeah, I do, yeah, indeed, to be seen.
Speaker 1:I think it's cool. I think it's cool to see what can be done, because I also I think we can all see a bit of tasks that you wish you could automate, right, like I was in london this past weekend and it's like okay, where you want to eat or what's the best schedule you know, and you want to group things that are nearby, or maybe you want to check the weather forecast because you don't want to be outside for these days. And I think it takes a lot of work today. But I think it's like a bit of a dream, right, like if you can just say these are the things I like, or even give, these are the things I want to see. Just come up with a schedule.
Speaker 2:And even very simple things like we're recording a podcast here.
Speaker 1:Yeah.
Speaker 2:Like from the moment we publish our podcast on Buzzsprout, and you would like an automation where you say, like, okay, it's published on Buzzsprout, so take the content, listen to it, summarize it and share that on the right social channels. Right, indeed, like with a certain tone of voice or with some certain instructions. Like ideally just want to tell that to chat gpt or to plot, and you, just like, every time it's published, do it.
Speaker 1:Yeah, indeed like, take it out of my hands, or even if you have not, there yet right.
Speaker 2:I mean, the technology is not like all these steps on its own.
Speaker 1:You can do but like to instantly glue that together for you like it's not there yet yeah, and I think sometimes you feel like that's the easy part because you're just gluing stuff together, but it's really not. I think it takes. It takes a lot of work. I think I'll be happy as well, even if you just like draft stuff and it just says hey, I'm going to publish this in a week, yeah, this is the draft. You have until then to edit it and I can just go there whenever I have time, just read it, change a few things or say this is okay and just go for it.
Speaker 1:Right, but like, have it in one place. I think it would be, it would be very nice. Same thing for like, uh, we have the python user group as well, and I think it would be nice to also share a bit about the speakers and the sessions you know and gather a bit and maybe create like a simple image. Just changing the, the person's avatar and doing these things I think as well could be very, very valuable. We'll see, let's see where we land at the end of this year, maybe. Last thing anything else you want to mention on this, anything that really surprised you, anything that you also wanted to bring here.
Speaker 2:No.
Speaker 1:I'll leave it at that, cool. And then are you ready to move on to maybe the tech corner? Bart, let's Alrighty. And then are?
Speaker 2:you ready to move on to, maybe, the tech corner?
Speaker 1:Bart, let's All righty. So maybe, while we're on topic on the add-on lamps and prompts, let's say Prompt Layer. Prompt Layer is building tools to put non-techies in the driver's seat of AI app development. What is this about?
Speaker 2:So I wanted to have this discussion with you, oof, okay. So maybe a disclaimer, that I never tried promptly on myself, so I'm not, uh, super knowledgeable on all the features, but I think their, their value proposition is a bit like they are a library slash, content management system for all the prompts that you use as a team slash as a company, um, to making it easier to to uh to share these prompts with others and but also making it easier to iterate on prompts and a versioning on prompts and these type of things. I think what they focus on mainly is end users, so not machine learning engineers. They build a specific problem but let's say, use chat gpt, that you have this library. Okay, you need to get that specific task done.
Speaker 2:Okay, use this prompt okay and I wanted to have this discussion with you, like what do you, do you believe in this value proposition? Why, why not?
Speaker 1:so just having heard what you just said yeah, so maybe there's more to it I don't see a lot of value Also, I mean aside from the value. So I think this is something very it looks like something very complicated for something very simple, Like if it's problem management. Okay, I understand that there are some things here and there, it's problem management Indeed.
Speaker 2:That's what you could say.
Speaker 1:But I think it's like to me to me this feels like too much, like I wouldn't build a product, like it feels like something so simple and also these things changes with news models as well and I think he's like I think it's better to teach people the what makes a good prompt, like adding examples or adding this or adding that, and let people come up with their things and maybe share what worked, what didn't, than to kind of have this structure. I don't know, I feel like I don't see, I don't know. I don't see myself proposing this at any place. I see myself more like doing a workshop what is a good prompt and why than to kind of have this problem management system.
Speaker 2:But that does assume that everybody's going to take you very seriously during the workshop and that they could. They're going to take these learnings and that's. They're going to translate that into a prompt yeah.
Speaker 1:But for example, like okay, imagine okay, and I agree, maybe there are people that don't care about this, they just want to use this stuff, right?
Speaker 2:why wouldn't we just open a notion page and just copy paste prompts from there, like it's, you know, like yeah, like if it's just a library you can do exactly, or like google docs or something that you also have a bit of version like to me it feels like a bit like what we built, like it feels like too much yeah and, to be honest, I think we're a bit uh, maybe not deep diving into everything that they do, because I think you can also save some metadata on the performance, on things, on what type of model was used, like, so you can build a bit of confidence also on like within the setting that you're at how good is this prompt? So I think it adds a bit there. But I also had a feeling like it's um, it is a big dependency or maybe it's a lot to have like this extra separate platform for this in such a, let's say, quickly evolving world. Yeah, that's, that's maybe my major concern that it's still so much moving every day. Whether you need this today, that is also.
Speaker 1:Yeah, we also saw a few examples in where depends?
Speaker 2:also I don't, by the way. I don't know what the pricing is, etc.
Speaker 1:But well, I also like I think that would factor in skimming through the page.
Speaker 1:I think they also have like cost and how many tokens and all these things right. So there is more to it. But I feel like, if the foundation is indeed just like prompt management, because we even saw some lms and I think even if you use chat gpt for generating images, you already see that the lm creates prompts for you as well. I think we also saw some models that can also enhance the prompts as well. So I feel like it's a bit I don't know the foundations. The foundations are I'm not very convinced, right. I mean, they can definitely prove me wrong, but I wouldn't. Yeah, I'm a bit skeptical, healthy skepticism, let's say.
Speaker 2:Yeah, I think it's one of the very few actually, let's say, more mature platforms in this space.
Speaker 1:I never heard of it. I mean, they look.
Speaker 2:And I do think like and that's a bit how I look at it today is more of an educational platform more than anything else like, and to me because, because I do think like, if she's saying, like, for specific tasks, these prompts perform very well, just use the prompt, that's in certain domains it might be so just to share what you have, what you know works well, versus like, teaching everybody like what is good, prompting yeah, that's true, but I guess it's like yeah but today, and I think maybe to make a bit of a parallel there and try to find the name as we speak, um so, so I've talked about bolted you right like, which is like your, your gen ai driven application development thingy and uh, only a few days ago, uh, like a small plugin for it was launched and I would love if I could find the name, but I can't, let me just give me a why are you looking for that?
Speaker 1:I'll also say that personally, in the beginning of the whole genii llm, I wasn't as excited, if I'm being honest, but I think it's because it was a lot around prompting and I feel like, personally, yeah, so we're talking about the value proposition, but personally it's also not something that really excites me. I think now I'm a bit more excited about llms and gen ei because I think of how interact with systems and what you can do and like what you deliver. But I remember before it was like oh, now we have prompt engineers and to me felt like a bit like man like, and no one really knew what was the best way. No one, there was no like. It was like you try stuff, this looks a bit better, so let's do that.
Speaker 2:And I was a bit like, yeah so I'll make an argument for it let's do it right so I've been using bolted new quite a bit and on the 7th of February okay four days ago, skippy I think it's an alias, his username on x launched supercharge, which is a chrome extension for bolded new, and it basically like adds a few buttons to your bolded new interface.
Speaker 2:And it basically like adds a few buttons to your bolded new interface, okay, and it has like auto completes on. Like I want this type of component, like this type of button or this type of dialogue box, and like you just need to click the button and like it it's basically fills in the prompt for that specific, for that specific component. Okay. And I do think, like I very much believe, that the software development space will be changed by, let's say, ai guide and development, and I do think like having like a consistent set of prompts as a team or as a company allows you to at the very least like build something that is somewhat uniform across teams, across people, like this is how we build components, this is how we set up end-to-end testing, like this is like a new, just by using the same prompts or these type of things that you typically run into for every project.
Speaker 1:Okay, so you're saying like for software development. Having the same like and against the prompts, I guess would say use this framework or prioritize this over that. Almost like you.
Speaker 2:Let's say where before you would have like this GitHub template and you say to your team everybody that starts new projects starts from this template and it's like a bit of a Kickstarter template, Like maybe it has some of the layout already done, Maybe it has some configuration that you find important, Maybe it has some CICD that you find important already done. But instead of having that, you now have like this kickstarter prompt, or a prompt where you say, okay, now add end-to-end testing with Playwright, or a prompt that's very simple, like just add a button, but in our style or in the style for this customer.
Speaker 1:And.
Speaker 2:I think there is some value in saying like to be able to do that. You need some consistency on how you prompt these things, because today, the interface I think that's debatable whether it's the best interface, business is a chat interface, and if you say we want to make something that is more or less consistent, whether whether I build it or you build it like maybe we need to reduce the same type of chats for the same type of tasks and do we need multiple prompts or can we just get away with just a system prompt, like if you say I think you need multiple.
Speaker 2:I think it very much depends on, like, where in the project you're in and what are you doing, like I think you could use some like a Kickstarter prompt to kickstart a project. Right, but for specific things, you want specific things.
Speaker 1:Yeah, I see what you're saying Today. I for specific things, you want specific things. Yeah, I see what you're saying today I mean again to be seen right. I think this world that you're describing, where jna is very much hand-in-hand with software development. We're not there yet, so I'm not saying you're wrong but okay, I want to break something up.
Speaker 2:Okay, so in the I think there's a difference in perceived outrage, okay, between the general population and software developers.
Speaker 1:Okay, same one.
Speaker 2:So you say something like okay, maybe like something around office management will be automated. And then you see in the general population a little bit of outrage. Maybe like something around office management will be automated. And then you see in the general population a little bit of outrage, like what will this mean for people? And software developers are like oh yeah, okay, that's logical. And then you see like okay, maybe something around the creative space or photography, like will be automated because we can generate this now. So maybe people will lose their jobs, maybe it will become harder to find a career. General population there's outreach, outreach right.
Speaker 2:Software development. Yeah, it's logical, right Like. This is the direction of our head, until you suggest maybe part of software development will be taken over by Gen EI. And then there's what the fuck? No, no, this is not possible. We're not there yet. No, no, no, what I'm doing is so special. No one can replace this, and I think today that is the case. Today it's not possible to just autonomously let Jenny do something, but I think today you can already, for yourself, achieve a bit of super agency by using all these tools. True, already for yourself, achieve a bit of super agency by using all these tools. True, and I think the expectation two years from now is that you do all this, because otherwise you're not relevant yeah, I see what you're saying.
Speaker 1:I just say because we're the ones building these tools, so we just kind of like go around it like every time like you, you discuss like there will be an impact on the world of the engineers.
Speaker 2:Like no, no, we're so special. Yeah, no, we cannot do it.
Speaker 1:No, I know what you're saying, I feel like, but it's also in general, like maybe the, the doctors and the artists will also look at me like, yeah, of course it makes sense, right, like, um, I see, I see what you're saying and I think and I agree that it will come at some point, right?
Speaker 1:Um, I think, yeah, and I think it's the same as, like doctors and stuff, I think you still need someone to understand a bit, to be accountable, to understand, like, if you need to change, if you look, need to look under the hood. But I think that would be definitely an impact. I mean, there has already been an impact, right, but there's more like autonomous stuff. If I were to do today what you're saying, I mean I agree, but, like, if we, if we have a bot, like a chat gpt, I would probably have different ones for different types of projects with like a different system prompt kind of thing, you know. But instead of managing different prompts for different things, right, like so, because even if you have like two different types of applications and you have prompts that you can just use across them, I also feel like you can also mess up a bit the consistency within the application that you have right Well.
Speaker 2:I guess that's why you need these type of tools. Right To say, for this type of customer, we only use this set of prompts.
Speaker 1:I see what you're saying, yeah, yeah, yeah, I see what you're saying, maybe, maybe I can see it Look like I'm skeptical on implementing something like that today.
Speaker 2:Like from the moment you say like AI-guided software development becomes like 50% of the day-to-day stack, there might be a good value added for this.
Speaker 1:No, I think there could be a good value. I mean, again, I'm not saying no, I'm saying I don't see it yet. Because to me it's like again, even if you, if you say, okay, we need to manage prompts, to me it's more logical to say let's have a notion page, that we just add some prompts, you can control effort, you can even ask the notion ai and then just copy paste and that's it right to like. To me it feels like it's a big abstraction, like even if you say there is a need for that, it's a big abstraction.
Speaker 1:Like, even if you say there is a need for that, it's a big abstraction for something simple, malcolm Right, okay, yeah, okay, but I will say that it's interesting. I think they're bold as well to give this a try. You know, like you said, they're the most mature in the space. So let's see, what else do we have? We have, well, maybe now moving a bit away, hopefully, from the whole.
Speaker 2:Gen AI stuff. Maybe we have one. I think we have one Gen AI stuff related thingy left. On the tech corner or on the the food for thought corner, food for thought corner. Maybe we need to jump to there and then we let's go, let's do it, let's do it.
Speaker 1:Let's do it, let's do it. So, let's do it, let's do it, let's do it. So we have here DeepSeq R1, 70 billion versus 32 billion parameter model.
Speaker 2:Yeah, so this was a discussion on Mastodon, which I find interesting. So I think there's a lot of rumors and I'm not 100% sure what the truth is, but there's a lot of rumors on censorship right Like of DeepSeek.
Speaker 1:Yes.
Speaker 2:I think most people know that when you use the DeepSeek chat interface, like when you ask about Tiananmen Square, Tiananmen Square never happened.
Speaker 1:Yes.
Speaker 2:I mean that is more or less the gist of the censorship. The interesting thing is that this person says, and also in the discussion, that he tried the 70 billion parameter and the 32 billion parameter model locally. And in the 70 billion parameter model there is a censorship, basically it's so baked into the model, and in the 32 billion parameter model there is no censorship. Parameter model there's no censorship, which I found very interesting because I I naively assumed that they did this at the server level, like that was just like a layer on top of the model where they did censorship yeah I see.
Speaker 2:But it's really like like the 70 billion model, like it's part of the training data set, like in some way or another. Yeah, that they did that. They included this like, and I'm wondering, like, is it part of the standard data set or is some way or another? Yeah, that.
Speaker 2:They did that they included this like, and I'm wondering like is it part of the standard data set or is it like a separate training phase that they went through for this? Um? But and that also makes you wonder, like the maybe there there is some documentation that, to be honest, I don't know but like it makes you wonder like, how did they go from the big model, the 70 billion parameter model, to the smaller one?
Speaker 1:and we know that they went from the bigger to the smaller.
Speaker 2:They didn't do the opposite uh, to be honest, I don't know. Okay, to be honest, I don't know, but I would assume, like so what you have a lot with these type of models is that you have like a very good performing model, which is the large one, and then you have methods to scale that down, either to to distillation, to repute, to pruning, to, to some extent, to quantization, um, maybe also for the people hearing these things for the first time.
Speaker 1:I can also take a crack like quantization. Basically, you have numbers that are bits in the end, right? So if you have a floating point number, you have so many decimals and you to quantize, say like this number is zero point, zero, zero, zero, zero one, and then it says, okay, let's just pretend that it's zero, yeah, and then the memory representation, because there are less decimals that you need to remember you also save.
Speaker 1:some infer like the computation mathematically is faster, but also the amount of memory you use it's smaller, right? And I think this is maybe not a great example, because if you say it's almost zero, so let's just say it's zero. That's also like weight pruning, right? Like you basically cut these connections in the neural network and just say pretend that it's not there. So maybe a better example would be 2.7722, whatever, and then you say let's pretend that's 2.7.
Speaker 2:Yeah, and there are different things, right, you can hypothesize a bit like, for example, like what you do with pruning is that you take a big model and that you run that against a testing set and you see, like which parameters are being used and the ones that are not being used, you prune them away. Yeah, so maybe in this just hypothesize now, maybe in this pruning phase, this dataset that they tested against didn't contain the censorship.
Speaker 2:I see what you're saying, which is possible if they trained the censorship at a later stage as a fine-tuning step. Yeah, yeah, yeah, interesting.
Speaker 1:And if that would not be included in the dataset, really like at a later stage, like as a fine tuning step. Yeah, yeah, yeah, hmm, interesting.
Speaker 2:And if they would not like, if that would not be included in the data set that they test again when pruning, like, potentially, the parameters that are using censorship are different parameters and they're just stripped away in the pruning process? True, but it's interesting to like, it's an interesting thought model. I think it's also interesting to see that they actually baked the censorship into the model yeah, which I didn't expect.
Speaker 1:Yeah, indeed I know that there are also ways to. I have a friend that is in research that he also mentioned. There are ways you can add layers to these open models to really jailbreak them, like really they just say everything that's there.
Speaker 2:But yeah, yeah, and, like I said at the beginning, like there's still a lot of rumors on this and there are very few people that can actually run a 70 billion model locally, like this person apparently did.
Speaker 1:But, yeah, interesting to see indeed, one other thing that it's actually an article that you shared. That did it to touch a bit on this as well. It's called S1 the $6 R1 competitor. So basically, someone there was a paper that was released Friday. Basically, they really just tried to make R1-like performance but way, way, way, way, way cheaper. And one of the things and that's how it links a bit to what you're describing If you use R1, you basically have this HTML-like tags that has the think within greater than, less than, and then it finishes the reasoning part with the forward slash think, right, one thing that they did.
Speaker 1:So basically you can, this is just a string, right, like, the model will keep track of all the things that has been produced, but you can actually force the model to think for longer, and that's what they did. So they replaced the forward slash think that's the stop thinking with wait and then the model before you could actually output the answer. And also, maybe one thing that they mentioned as well in between the think tags, the model was it's more casual, it's less authoritative. So then, after the think, they become more like yeah, this is this and this and this, and that's something you can see as well if you try out R1. But one thing that they did is to replace the end tag with a wait To basically say the model keeps thinking about it, right? So they kind of like, as the model is producing tokens before that token becomes the input for the next step, they cannot replace it, and that was like a hack, right, that they could do to try to get the performance to improve or not, and one of the things they did.
Speaker 1:Now, with regards to the censorship, they also can start the sentence with like a forward slash think I know this and they actually were able to kind of bypass a bit the censorship that the R1 model had. So, for example, they asked something about the Tiananmen Square, which, yeah, I guess the model was fine-tuned to avoid discussing events like this. But if you start, if you prepend your string with think I know this, it would nudge the model to actually spit out everything that it knew. So it was another way that they found out how to jailbreak these things. So I thought it was quite interesting. Another thing that they also mentioned on this article that I wanted to bring up the data frugality, right. So basically, they selected really the best examples and and yeah, like they kind of over-trained on that. Not over-trained, right, because these models they do require a lot of loops, a lot of epochs, but I think they took the best 1000 examples and they said that everything after that was actually not didn't add the performance much.
Speaker 2:Okay, interesting.
Speaker 1:And I think also again of like years ago they also had the data-centric competitions, right.
Speaker 1:That was the idea that garbage in, garbage out, so there were competitions like kind of like kaggle, that instead of having you give me your model, I will tell you exactly what the model is and how it's going to be trained, and then you give me the data set for that, right. So, and then I think it kind of went out of hype or out of fashion, right. But I think this also kind of made me think about it, right, like how, yeah, more work on the actual examples actually can lead to very big performance differences, right, and they try different things Like, for example, I mentioned the weight. They try, like, instead of saying weight, they try saying mm or saying alternatively, and they actually could see how the performance would change on that. So they kind of took the, the, the bed of r1, and they kind of made a whole bunch of little experiments to see how much they could push these things right, um, and they actually got this s1 model here, so I thought it was pretty cool.
Speaker 2:Um, yeah, that's kind of that I wanted to mention, uh, but uh, yeah it's very like intuitive hacks indeed like this is something that you would like very naively think about like instead of net, of stop thinking, you just insert weight, or indeed yeah yeah, just let's just see how it goes and measure it, right.
Speaker 1:So I think that's that was. That was quite fun. And the other thing they could also do is like they could introduce the stop think token, right. So if it's going to say something that you want to censor it, you can just say if this word appears, just insert the stop thing, and then you can also censor the model that way. Uh, very intuitive, very cool. Um, and you knew about this s1 as well. How did this come up in your feed?
Speaker 2:I'm not sure to be honest somewhere along my feet, but there you have a. Since that, since r1 was released, I think there was a, an iteration of an R1-alike model with the same type of reasoning training. That was already super cheap.
Speaker 1:Yeah.
Speaker 2:I want to say something like 60 euros or dollars, and then, as a response to that, this thing got published.
Speaker 1:Ah, okay, which was?
Speaker 2:$6, which is crazy right To get that performance. I think it's roughly 01 mini performance on some datasets.
Speaker 1:Yeah, indeed, maybe one thing that yeah.
Speaker 2:I think which is good to see.
Speaker 1:It's really good to see. I think it's also like the that's how I see the engineering research and the academic research pendulum, kind of Like. On one end, you see, it swings to the research side and then people are trying to prove that things are possible, and then it swings more to the engineering side and try to make it accessible to people. And I think that when it's like that, they say oh, you can achieve this performance by shifting this and this, and now people are trying to make it very easy for everyone to adopt it. So I think it's cool.
Speaker 2:I think it's also the quote maybe, while we're on the topic, I have your predicted outputs from OpenAI oh yeah, one more thing before we move on predicted outputs is actually something that is not that new anymore I want to say it's from November of last year but something that I run into when using um gen ai for for code development is that it's very slow, right, I think most of these tools today default to sonnet 3.5. Um, like, if you, for example, bolt, it uses I think Lovable also uses Sonnet if you use Klein not sure if I'm pronouncing that correctly C-L-I-N-E VS Code plugin like it. Also like I think it's Jest Cloud Sonnet 3.5. And the problem with Sonnet 3.5 is, well, the not problem is that it's very good at these things. Yeah, the challenge is that, like in order to change a file, it has to rewrite the whole file, which is one is slow.
Speaker 1:Yeah.
Speaker 2:And two, it uses a lot of tokens.
Speaker 1:Yes.
Speaker 2:And predicted outputs are not, and I'm not sure how good the performance on these things is, because it didn't test it, but predicted outputs is something by by open AI and it's, and it's a bit built on the notion that, okay, I'm gonna edit this file. So what I'm gonna do is, like I know what the output is for most of these things. I only want gonna uh make concrete instructions on what needs to be changed and the rest will remain the same. I see, I see which, if it performs well, like and again, I haven't tested it, but I think like we need to go towards this for for uh, for co-development, like it's, because it will be much, much, much more efficient yeah, for sure.
Speaker 1:But then so because I know, like I use cursor and cursor they do have like the diffs per yeah, code segments I guess. So you can just say, like, use the composer, which is like the agent to edit files, and then they will go through the file and just say these are the things I need to change. I'm not sure if they actually generate the whole string of the file and then they just kind of show the diffs in the UI, but I'm not sure how cursor does it and which model it uses.
Speaker 1:And then for this, so very practically speaking, the LLM outputs like a segment saying like I don't know, comment top of the code, and then they show the difference, like, how, like, because in the end it's still an LLM right, they still have a prompt in and a prompt out.
Speaker 2:Yeah, but so in the prompt out you get. That's how I understand it. I think you can also see an example on positions of predicted text and response. I should go down a bit Down.
Speaker 1:Let's see here.
Speaker 2:And the promise is basically that you get like concrete instructions on what needs to be changed on the input. I see, instead of a fully rewritten input in the output.
Speaker 1:But basically we just say, between this, this line and this line, I don't know how the exact instructions look like.
Speaker 2:But I think that is the the premise and I think if we would get there would make uh yeah, indeed, our lives a lot more efficient, for sure, for sure, and cheaper as well. Right to to run these things Well. Yeah, of course Also the compute power needed, for that would theoretically be less right If you can get to a good model on this.
Speaker 1:True, true, true, true. Yeah, curious to see how this goes. I mean, this is OpenAI as well, Like you said. I have a feeling that most of the programming things go to cloud or cloud is ahead somehow on these things, I think the consensus today is that your best bet is Sonnet 3.5.
Speaker 2:Yeah, and I think what a lot of people are doing today is using DeepSeek V3, more specifically, because it's very cheap and almost as good.
Speaker 1:Yeah, yeah, indeed. Yeah. I wonder if cloud's going to well DeepSeek. I know it's an cheap and almost as good. Yeah, yeah, indeed. Yeah. I wonder if Cloud's going to Well DeepSeek. I know it's an open source thing, but I wonder if Cloud is also going to, because actually I haven't heard from Entropic. We haven't heard from Entropic for a while. Right, it's actually a very old model.
Speaker 2:Well, in this space it's a very old model.
Speaker 1:I want to say it's from April-ish 2024, yeah, something like that. I think so. Yeah, because, like, since then open, ai came out with the 01 models and dpc came out with their things, but I feel like I think even even 40 was released around the time first yeah, curious.
Speaker 1:I mean surely they're not just sitting on, sitting on island, their thumbs right like they're cooking something. So I'm curious to see what's gonna, what they're gonna release all right now for the less ai thingies and actually if we listen to like community rumors, so you have a.
Speaker 2:If you have a pro account on cloud, you have you more quickly these days. Their usage limits so there's a lot of chatter going on that they are using their compute resources for something else. I see In other words to train something new?
Speaker 1:Indeed, Because they don't have like a reasoning model at all. Even though Cloud is compared with O1 and stuff.
Speaker 2:It's like I don't think they have a reasoning model today. They don't think they have one. Yeah.
Speaker 1:Cool, all right. Yeah, cool, all right, maybe. Uh, let's check some stats stats mac os mac os system monitor in your menu bar. So now we are back at the tech corner. Um, I have a guess what this is about, but do you want to tell me what this is about? What?
Speaker 2:yeah, this is just a bit. If you want to geek out on the stats of your, of your system, uh, install this. All right, like this is a. This is an open source. Uh mac package, uh mac application by I don't know you moved away from the page by uh, excel, ban, excel, ban. Um, it's, uh, it's popped up on hackeracker News, I think last week somewhere, or the week before even. It's basically in your taskbar. It installs some cool stats monitors. It's very configurable so you can show whatever sensor you want. It really made me think of back in the day when I was playing with Openbox and I had all kinds of sensors on my desktop. I'm using it on my mac for mini. I'm very happy about it. You're using this yeah, yeah, okay.
Speaker 1:So yeah, if you want to just have like yours, like like stupid stuff, like I've also sometimes.
Speaker 2:Uh, um, like I'm using, I'm doing google meet and the connection is of someone is bad or it's my connection. I'm not sure. I'm going to look at this little sensor in my taskbar and it shows my network in and out so I can verify a little bit, like does it make sense, or is it me, or is it someone else?
Speaker 1:Yeah, that's true. I think it's like the stuff that you normally open the activity monitor, exactly.
Speaker 2:Yeah, you have like a very succinct, like charts, so like that you cannot see the taskbar pretty cool and it just, it just gets like it's like a sticky note kind of thing. You just stay, no well you can have it, have it as a sticky note, but I don't actually not use it. You can it really have these, these things in your taskbar, like above the, the sticky thing? So that's really really cool and if you click it it opens up the sticky thing I see.
Speaker 1:So it's like, yeah, for people just listening, it's on your progress bar on top like that the thin thing on top that you can kind of hover over, and they have a lot of graphics and stuff. This is cool. This is cool. It's also good to see, like I think, for in jupyter notebooks. Sometimes the kernel just crashes and it took me a while to realize this because it was running out of memory because, yeah, so these types of things, yeah, you can see a lot of that.
Speaker 1:You would normally open up the activity monitor to see like yeah, indeed, because you crash this once and then you try again and crash again and then you need to open the thing and keep traveling. So, yeah, it can be nice. Cool Written in Swift. I saw Cool and swift.
Speaker 2:I saw cool, cool, cool, um birdie I saw you posted something this morning on our internal techno share uh birdie snapshot testing in gleam, uh, the gleam language uh, which I don't use, uh, but uh I. I think the premise of snapshot testing was interesting because I never really thought about it in this context. So snapshot snapshot testing is uh. When people talk about this is typically used in uh application development. So in your ci you uh run your application, you go to a certain page or whatever and you like make a slash screenshot from a page and, for example, you audit to the conversation in your PR and then you approve the PR.
Speaker 1:Yes.
Speaker 2:That is typically when people talk about snapshot testing, like that's really screenshots of your application. But what Birdie actually does is it allows you to take take snapshots of whatever like, really like a function with some inputs and you don't need to specify the output, but what it will do is like it will at the the first run of these tests.
Speaker 2:It will always error and you basically need to approve this snapshot once. So let's say, make it simple. Like I have my plus one add function, I insert one. It will make a snapshot of what happens. Two will come out right, but it will fail the first time and you will have to say, okay, two is correct, yeah, so no. And then every time it is not two in the future, it will error and you will know, like okay, something changed here.
Speaker 1:And then you need to approve it and then you need to approve it, yeah. So then you have like a state of like the snapshots yeah, and then stay somewhere, and then it just looks at that, Exactly yeah.
Speaker 1:Your question on TechnoRisk or TechnoShare was if anyone had any opinions on this, and I was thinking about it as I think it's an interesting concept, but I'm not sure because I think so what I would prefer in Python at least. Right, using PyTest in Python, yeah, if you have a plugin, that, because in the end you're kind of generating the certs, right, the certs are hidden, yeah, and you're just creating and updating them.
Speaker 2:True, but I would still rather because if you look at the, the code sample here, um, there's like you don't know what the value expected value is exactly right.
Speaker 1:You don't know what it's, yeah, at the moment of the first snapshot, and maybe maybe just me being a bit old, but like, I still think I would like to see the assert there. I would still be happy if it updates it for me or if it creates the assert, but I would still like it to be there because I think also, if you're versioning code and if you're looking at it, it's like if you're reviewing apr, it's very easy to see, like this function, because I also like to use functions as almost like a documentation. You know, whenever you add one, the, the function will return two and then I can look at the function definition. Now, okay, I see, I see what you're trying to do. Now I can at the function. So I think it's like having it written. I appreciate that.
Speaker 1:I think I also see the point like if you refactor some function I have seen PyTest that the errors come up and I'm like, okay, I'll just update this because I know I changed this and I know this will influence that and it's fine. So I do think it's useful. But I would rather if the plugin would update the search statements for me then, not knowing what the search statements are.
Speaker 2:Yeah, yeah, and what you mean is like you don't know what the output is Exactly, like just reading the code. Yeah, when you read the code, you want to see what the output is, but I think, to take the other extreme, because it's a bit like where we started the discussion, like with a screenshot. It's logical, right? Yeah, because it's hard to describe, indeed, right.
Speaker 1:Like even there you can make a search right, but it's hard to describe, Like if you're doing, if you have a streamlit application and you want to test stuff, and it's like, yeah, like when the person even you have to simulate clicks in the UI and then you have to wait a bit and you have to this. Yeah, I also feel like having it written is not going to make you more intuitive, to know exactly what's happening.
Speaker 2:Yeah, and, to be very honest, I also have a hard time because we are talking about deterministic things like adding one to something right, yeah. Which is easy. You're not going to use this approach.
Speaker 1:I would assume.
Speaker 2:Yeah, you're not going to use this approach. Yeah, I would assume. Yeah, we have the other extreme, where it's a screenshot. Well, let's be honest, you can also debate the usefulness of that, but let's not go into that. There's a lot of debate on that as well. But like it's a bit more, like from the moment it's very deterministic versus it's less tangible and like there is somewhere like a threshold that you pass where this becomes relevant.
Speaker 1:But I also have to be honest, very hard time coming up with concrete use cases but I think, maybe, but I see, well, I completely agree with you, but I maybe is also, if you're building a lot of front-end applications and stuff, and I don't know, maybe, maybe you find this more more useful. I'm not sure.
Speaker 2:I feel like if you're closer to the snapshot, you can also like, like, let's say, uh, random stuff here, but reinforcement learning you're trying to learn an agent to uh, to teach an agent to play doom? Like it's very hard to define, like to assert it now knows doom, true, true, but at the same time it's also hard to approve on a snapshot for that right like. That's why I'm saying like I see that there are cases where it's hard to define, like in a deterministic way, what is a good output, but I'm not sure whether a snapshot solves that yeah, I see what you're saying, but I think in my well, from the, reinforcement learning is interesting.
Speaker 1:but I think there are two.
Speaker 1:There's like different complexities, right.
Speaker 1:One is like you're interacting with an environment and how do you make assertions about the environment, and I think maybe that's also what the snapshot of desktop of a UI is also a bit like you can think of that a bit of as an environment, right, and for you to, when you click something and there's a lot of stuff that happens in the back, and to really write assertions, for everything is not going to be clean, is not going to be simple. I think reinforcement learning is also the probabilistic part of it, right, that, yeah, in the end it's like machine learning, all these things, so it's a bit hard to make assertions because it's probabilistic. But I think maybe the yeah, maybe whenever you have something that is too, like you said, almost like an environment is like broad, maybe the snapshot testing comes in very handy. Yeah, like if you're interacting with the system instead of having to make assertions about all these things, it's just like, okay, this happens, this is the state after you interact with it. But yeah, I agree with you, I think we're it's hard to define.
Speaker 2:It's hard, it's like.
Speaker 1:I feel like we're very much on like hypothesizing here. I feel like we're very much on like hypothesizing here, but I think it's an interesting concept nonetheless. What else we have here?
Speaker 2:Beware it's a cute logo yeah, beware. So this is. I just want to do a shout out to Beware here Right. Shout out Write once, deploy everywhere. So this is for python developers that basically want to uh, deploy stuff cross-platform, like, uh, we're in 2025 python ecosystem, like it's very hard to be a python developer and deploy on all devices. I think the premise of beware is that you um write once and you can deploy to your ip, you can deploy to an Android, you can deploy to web.
Speaker 1:This is for UI applications.
Speaker 2:I think that is the big chunk. I don't think necessarily, but I think that is a big chunk. Okay, I'm always so. You see this popping up a lot of times over the last. I've seen this a lot in the last 20 years. I'm always like, like I've not seen this. The like in python, really like this is the standard for these type of things yeah like it keeps moving.
Speaker 2:I like I hope for the beware project that they get. But what I'm always like super impressed is like the effort to get these type of projects to a level where you can actually deploy to multiple times. Like the effort that is needed for these things is so immense like it's hard to like, it's mind-boggling, like the, the, what, the time that you need to put in this to get, to get to to even like an alpha version that somehow works yeah, it feels like you're.
Speaker 1:I mean, yeah, I think you really need to love Python, like you really love it and you want to see it everywhere because yeah, I think it's impressive and, let's be honest, I don't think it's impossible.
Speaker 2:I think, no, it's not impossible If we say 10 years ago no one thought that Flutter would be as huge as it is today.
Speaker 1:True, but it is true um but yeah, but it's an endeavor, right, it's yeah well that's why I wanted to shout out things.
Speaker 2:It's a crazy big endeavor. It's also good that we explore the boundaries of python.
Speaker 1:These things um I mean, yeah, I think there's a lot of value even if this doesn't succeed. There's a lot of value that can come from trying these things right. It looks like a nice. I mean, I like the. I'm curious to try it out. You haven't tried it out yet, no, no, no, yeah, also this and do you know how they do it? By the way, do they use WebAssembly stuff? Do they go to the sea level? Okay, um, one thing I well came up, came across this a while ago um, pi oxidizer this is a bit of similar idea utility library producing binary binaries that embed python. Um, so, yeah, I think the idea was indeed you, you have Python, you create a binary for it and then you can run in different architectures.
Speaker 1:So, maybe not exactly the same scope.
Speaker 1:Well, I think one of the sub-projects in Beeware is actually also this like to run Python stuff standalone, yeah indeed, because indeed, today, even if you have a CLI tool that's written in Python, the way that it works today is like you have to have a virtual environment that install dependencies and you have all these things. So it's a bit like people work around that as well. Uh, this, this is not maintained, I think, um, but it was also. They try to do this with rust, so with the rust, uh, things, because it's more binary focus, right, but I think, if you go here on the issues, yeah, project status, so I think, um, it's not maintained now, sadly, but I think there are other ways you could now the binary one, right. So python is most popular, c python, so I guess, from the c, maybe you can do some stuff with that. But, um, I also had a talk on fosdm. Uh, fosdm happened like two weeks ago and one was about using web assembly to replace containers. You listened to it or you had a talk. I listened, okay, um, it looked cool. So the idea is like, yeah, web assembly, python now compiles web assembly. Um, web assembly is the stuff that runs in your browser, but like, it's just like a another target, right. And then they were saying like, instead of having the docker thing which is more bloated and all these things. You could compile things to WebAssembly and you can distribute them.
Speaker 1:I don't have a lot of experience with WebAssembly, if I'm being honest, but it is something that I'm very curious about. It looks very interesting. I think the name was Boxer and what he was also mentioning is that the web assembling stuff that, yes, it's this one, the web assembly stuff. It's much, much lighter than docker containers. That's also. It was a bit his his thing, so it was so the idea with this project so this is the guy that wrote the project, by the way. The idea is that you can have the same interface as a Dockerfile, but then you just use Boxer, which is the CLI tool that he's creating, that basically builds a Wasm, exactly but like it's from the Dockerfile, so you don't have to learn new syntax. But instead of creating like, yeah, from this Docker file, copy this, copy that and then in the end, you have a WebAssembly thing. So it was cool, but, yeah, still need to learn on it. Learn upon it. What else? Maybe last topic part? Yes, what else? Maybe last topic part? Yes, you know Astro, right?
Speaker 1:yeah, the organization behind yes, behind UV Roof Roof anyways. So Roof is a linter written in Rust. Uv is a Python package manager.
Speaker 1:Slash other things and you're its fanboy, right uh, I guess, good, I will accept that, even though I don't fully agree. I, yeah, I am a user of uv, of rough, um, um, they also have a replacement of black, so they have a formatter in rough now. And maybe the next thing that you kind of like okay, mypy, right, we have PyWrite. They're all written in Python. These things do. These are some things that actually take longer, like linting. You could say, yeah, I don't wait a lot for my linting and I use pre-commits anyways. But actually, the static type checker, it is something that is that I see a use case for, for speed, um, and I was waiting to see when astra was going to do something about it and apparently there was a tweet or an ex that, uh, the creator of, well, the person that runs astro, basically basically he stated that, astro, they are building a new static type checker from scratch in Rust. So, yeah, ambitious project from a technical perspective, about 100 PRs deep.
Speaker 2:This is from January 29th, so a bit old, let's say, but it is something that Interesting Will come up in the python, yeah and I think I'm also excited, in a way, because astro is not like they employ people right, so I'm expecting them to be able to move fast with these things yeah, and if we uh skepticism aside there's a lot of skepticism I think there are a lot of of community discussions about like they take a lot of the learnings that there are, they just reimplement them in a commercial company. But uh, let's be honest, what they did so far with uv and uh, with rough um, like it just it just works. Yeah, and I think because of that, like a lot of people move there, like it just does things out of the box very well with minimal configuration. I also moved there for most of the most of the things that I do, fanboy um and I think.
Speaker 2:But the whole premise of it's it's very fast, like to be honest, it was not a major thing with things like rough right, or even with things with the uv, unless you have a very complex project. But for a static type checker, like speed becomes a thing, because my pi, we all know, is super slow, like there. And I think from the moment that you go from waiting, let's say, 10 to 20 seconds, and the new norm becomes one to five seconds, yeah, if it's even going to be that, it's very hard to move away from that, right it's an experience indeed, yeah I fully agree I think they will be like if it's, if it's good, a lot of the community will move there also because they have a very strong track record.
Speaker 1:Exactly. I think they built their reputation well, like I agree with you, even when I started using rough. My main argument for going for rough is because they bundle a lot of plugins from flake 8 that I didn't have to add manually and I didn't have to discover them. So it's there. So, yeah, I'm also curious to see how they're going to do because I do think this is the first, let's say, tool that I actually think that speed is a big issue. Curious to see what they're going to come up with. I think hopeful because I feel like the static type checking story in Python. Curious to see what they're going to come up with. I think hopeful because I feel like the static type checking story in Python is always a bit it's the one thing that is like oof, yeah, that's true, but it's cool. Alrighty, anything else you want to. That's it for today. I think that's it for today indeed. Thanks everyone, thanks Alex, thanks Bart, thanks everyone for listening Talk to you next time.
Speaker 2:In a way that's meaningful to software people. Hello, I'm Bill Gates. I would recommend TypeScript. Yeah, it writes a lot of code for me and usually it's slightly wrong. I'm reminded, incidentally, of Rust here, rust, rust. This almost makes me happy that I'm reminded. It's a dust hit, dust, dust.
Speaker 1:This almost makes me happy that I didn't become a supermodel.
Speaker 2:Cooper and Nettie Boy. I'm sorry guys, I don't know what's going on.
Speaker 1:Thank you for the opportunity to speak to you today about large neural networks. It's really an honor to be here Dust, dust Data topics.
Speaker 2:Welcome to the data.
Speaker 1:Welcome to the data topics podcast.