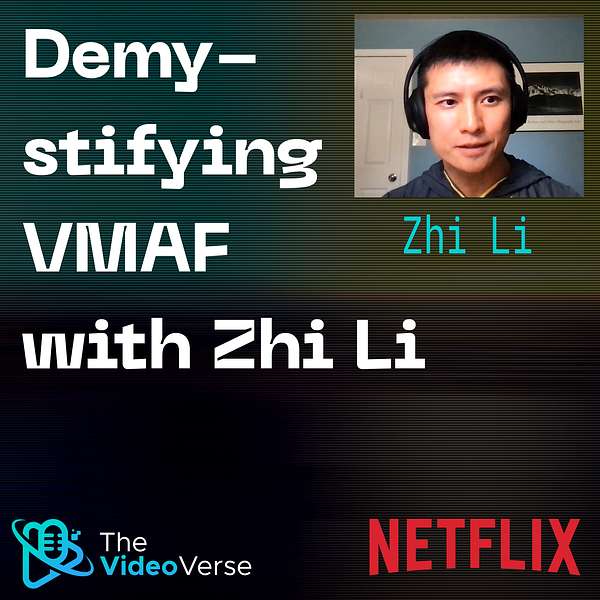
The VideoVerse
The VideoVerse
TVV EP 20 - Demystifying VMAF with Zhi Li
The VMAF video quality metric is ubiquitous in the streaming world, and any discussion of video codec performance is seldom complete without poring over VMAFscores. But what is it? In this episode VMAF guru and leader of the Netflix Video Codecs and Quality team joins us to delve into the mysteries of the metric. How did it originate and why was it needed? How has VMAF been developed and what does the future hold for improvements? Along the way we talk about the work he and his team do at Netflix and where quality assessment and codec development is leading in a world of generative AI.
Watch on YouTube
Welcome to "VideoVerse."
Zoe: Hi, everybody, and welcome back to our "The VideoVerse Podcast." So today here I'm actually sitting at Purdue University in the city of West Lafayette, Indiana. So this is a video and image processing laboratory, short as VIPER. So in the VIPER lab, of course, we are just going to continue talking about video and multimedia in general. And we are actually very glad to invite Zhi Li from Netflix to come as our guest for this episode. Again, I have Thomas. Hi, Thomas.
Thomas: Hi, there.
Zoe: Yeah, from London to join me as a co-host from the Visionular side. So this is Zoe again, and Zhi, I will like you to introduce yourself.
Zhi: Hello everyone, I'm Zhi Li from Netflix. Just to give a brief introduction about myself. I'm with Netflix, encoding technology, I'm currently leading a team that's working in a few areas. The first one is codecs. We contribute to the next-generation royalty-free codec, code name AV2, I don't think it's official yet, but let's just call it AV2. We're also curating the adoption of AV1, just to make sure it's gonna be a success.
The other area we work on is video coding metrics. So we actively develop and maintain VMAF as an open source project but also intensively use the internally, together with a number of the other metrics. If you have heard about CAMBI, that's a metric that addressing prediction of the banding artifacts.
And the third area is we're actively doing research and development about optimizing Netflix video encoding pipeline. Anything that we could deliver a better quality to our end users, we work on that. And we work very closely with our client team to make sure the end-to-end quality experience is the best, any devices they stream from in any network conditions.
So personally, as a background, I obtained my bachelor's and master's degree from National University of Singapore. Then I did my PhD at Stanford advised by Professor Bernd Girod. After that, I had a two years stint at Cisco, working with a service provider video technology team. So I work closely with Dave Oren and Ali Begen. That's actually one of my pretty productive period of time where my most highly cited papers actually have been produced during that period of time. So just to.... That's my brief introduction about myself.
Zoe: Wow, thank you. And basically, I think based on what you described, at least from the PhD, right, so we all know that Professor Bernd Girod is mainly have contributed a lot in academia area and potentially to the industry and a few very well known, like video codec and processing and the streaming solutions behind that. So believe that your career grow from there and all have been in the area and in the field of video and something related to that.
I didn't even know that AV2 has not come to as a official name yet. I thought it's AV1, then AV2, that's some standard is going to be finalized soon. So we'll expect that. So talking about, I think because you mentioned AV1 that you are translate having your team dedicated to that because AV1's finalized. So definitely that's related to the product you are doing. And AV2 is a standard and I believe you are mentioning you try to make new proposals to that. So talking about all this video codec type of work, you may want to like introduce a little bit further about your team's current effort along that line.
[ 00:04:40 Team's current effort on video codec]
Zhi: Sure, yeah. So Netflix, heavily investing in supporting AV1 as a current generation codec. So we have, it's the most advanced encoding family we used for our encodes. So we curate this initiative, and by doing lots of engineering resources around that including, so we develop optimized recipes for encoding our content using AV1. And we also work with device partners to unlock the decodabilty on those devices. So as of today, if you are on the newer model that supports AV1 on newer models of TVs and if you stream Netflix shows in 4K, you are very likely to get AV1 streams. And I have a LG OLED TV 2020 model at home and I can stream AV1 from my TV.
Thomas: Hm, fantastic.
Zoe: Yeah, good to know that.
Zhi: Yeah, so we... I mean, we are continuing this initiative by investing in AV2 as well. So this is by contributing to the so-called AVM. AVM, is the official name that we've been using, but everybody has just called it AV2. So what we feel is we're bringing to the table some of the more unique perspectives from a Netflix point of view. I think there's a few other stream services that's similar to us.
Basically we maintain a catalog of professionally created TV shows and movies and those typically come with their unique characteristics because they're professionally generated. We're trying to create from user's home a cinematic experience when they're watching Netflix. And so I think one of the aspects is to try to preserve the creative intent from those content when they're being created. So we do want to have the best preservation of the creative intent, things like film grain preservation is one of our top priorities to maintain that feel about high video quality.
So we tailor our investment with respect to some of the use cases for Netflix. So film grain is definitely one of them. And the other area I could mention is about banding. I think banding has been especially a notorious problem when it comes to professionally generated content. I think it is when people typically don't realize the problem with it, because it's typically associated with proper viewing conditions. Once you've been set up like in a cinematic, a home theater kind of a set up, very low ambient light and very low reflection on the screen, then you start to see those things pop up.
Things are especially problematic when you encode with some of the older generation codec and especially when you're encoding at 8 bit, I think it's more obvious to have banding but things don't completely go away even if you're encoding at 10 bits. So what can we do about it? For example, should we be going after 12 bits like moving for the future generation codec or is there a better way we can do? And that's sounds one of the things we've been looking at here is to contribute a tool to AV2 that could address banding better and in a low cost way. Banding can actually be addressed pretty sufficiently by doing the so-called dithering.
So you introduce this random noise model into your picture because the human eye will essentially function as a low pass filter, so with that you are actually being perceived, the dithering pattern will be perceived as the intermediate gray scale by the human eye. So that's a pretty efficient way of how we would be able to tackle this problem in reality. So we're hoping to get this into the next-generation codec.
Thomas: Yeah, so one interesting thing is that there are often some strange overlaps or interesting overlaps with different application domains, because I've come across banding as a real problem actually in screen content applications. There's lots of people who want to use modern codecs for screen share for computer aided design for example. So they have very, very high resolution, very, very clean video and then banding is a real issue for them.
And there are also contributions from conversion from RGB to YUV as well that you see less banding in RGB but then you throw away resolution in YUV. So it's really interesting to tackle it. But one thing that is of interest to me too is how you control the complexity in trying to solve these kind of problems, because lots of codec implementations do 8 bit because it's much more complex to do higher bit depth. So do you see dithering as a way of solving both problems really, the banding and maintaining reasonable complexity?
Zoe: Go ahead and like, I like you 'cause it actually recall me I think back to 2008 when the time I joined Apple, that was really 15 years ago. And then I also got involved into the Apple store, is also the movie recording like you mentioned and it's basically also professional PGC content and at the time we tried to like have a higher quality for those contents. It's the same two issues that you just mentioned. One is the resolve the banding, I think one banding, just Thomas mentioned, for like screen content at the time is mainly on the skies. One is on the skies of the nitro scenes. The other one is animation movies, same thing with the screen count because all generated content and that the banding is very annoying. Then when you try to squeeze bits, it seems that those, you can throw less bits into this area, but instead you have to throw more bits to this area. Another one is film green and then this is something really still, I think, hanging around, but it's likely we have more tools in the new codecs standard that we can address them, right?
[00:12:17 How to control the complexity in less banding and higher resolution]
Zhi: I think one of the good things about addressing banding is it's pretty well understood through a human visual system point of view. So essentially everything just boils down to this contrast sensitivity function. Which is pretty well understood over the research work of the past 100 of the years. And I'm just talking about the gray scale contrast sensitivity function. I think when it comes to color it becomes a little bit more tricky to model.
I don't think for the chroma channel as of today there has been a like, well acknowledged model that works across the board, but at least for the gray scale I would definitely see it's a well understood problem. So the solution is also fairly simple, that we need to develop a model to predict where this banding is visible. And that will allow us to very fiercely tackle those areas when it's being detected. You only need to tackle those areas when it's being visible. And I think it's well understood and it's well predictable and I believe this solution is just out there, it's fairly close for us to tackle this notorious problem hopefully in the next-generation codec.
Zoe: Well, thank you for actually bringing at least the mathematical foundation behind this problem actually.
Thomas: So I also wanted to ask you a bit more, if you could expand a bit more, about your work on optimizing encoding chains and so on because I think many people may not realize that when you build an encoder, you build something with an enormous number of knobs and controls that you can tune and there is pre-processing as well that you can do. So it's not simply a matter of tuning your core encoder to do things, but there may be special things that you can do for particular kinds of content or particular scenes. Can you tell me a bit about how that works at Netflix?
Zhi: Sure. So I guess I need to first talk about the uniqueness of the problem space we're tackling here. For Netflix, we have a relatively small scale catalog. If you compare our scale versus YouTube or Meta, I think we have a relatively small scale and the other aspect is that our catalog is being professionally generated but it's being, like, encoded once but you stream it millions of times.
In other words, there is a very asymmetric relationship between encoding versus decoding. So that gives us this leverage that we would really be able to spend lots of resources trying to optimize our encoding. And when you're amortizing that among the streams processed, that cost is relatively low as compared to other use cases. So with that caveat in mind, we do have lots of, like the kind of tuning we do can be understood as out of the loop. So you have this video encoding, you can do in codec in-loop optimization. For example, two-pass encoding is one of them, but you can also apply search in an out-of-loop fashion.
So one way to think about this is that if you think about the video content, essentially it's an aggregate of multiple modular coherent or homogeneous pieces, but you can think of them at different granularity. At the highest granularity you have different genres. Different genres will have different characteristics. You can optimize on a per genre basis but have a fixed recipe for any of the titles in one genre, or if you move one level down, you can optimize on the per title basis. Each of the titles, you can analyze them and decide on what will be the complexity and you can apply the optimization accordingly and you can further, going down that path, you can do it treating each of the shots as a homogeneous unit.
And I think the problem essentially boils down to how you would want to allocate your resources across all those units. So essentially, like a resource allocation problem and think it mathematically it will boil down to a constraint optimization where you have to optimize against a certain metric. And in this particular case, at Netflix lots of things are depending on the VMAF metric. So the journey for us is also, it's gradually being built up. If you recall we had a tech blog about per title encoding. That was 2015. If you read about that tech blog, we didn't mention about VMAF at all because at that moment that metric hasn't been mature enough to be applied here and we're still like sort of depending on using PSNR as a metric but combined with a number of other tricks, like controlling the QP that you sample and together like you form a solution and you'd be able to allocate resources on the per title basis and applying VMAF to this encoding optimization is actually a afterthought.
It's when we see this accuracy, gradually we build more and more confidence into accuracy. We figure, well, maybe this is good enough for us to be using it as actively being used in the encoder optimization. And eventually in 2018, we have this solution called dynamic optimizer, where essentially folding in this metric to guide us to optimize streams. So as I said, VMAF is a big part of this optimization framework and lots of things are related to this resource optimization.
There are other components within our encoding pipeline, as you said. I think the pre-processing and post, I think mostly pre-processing, or one example would be, how you do downscaling of the video. So if you are bit-rate constrained, it's not always guaranteed that the best results will be that you encode the video at its native resolution. Sometimes you want to downscale the video and to limit its number of pixels and then you can efficiently apply encoding and you're relying on the decoder after decoding on the devices, you do an upscaling based on the own device upscaling to be displayed on the end user's screen.
And we have techniques that we will be able to tackle each one of those encoding and scaling separately. I think the main approach we do is still like fixing eight, the other components within this pipeline, but just by looking at individual ones, hoping that things don't interfere and we'll be able to afford to optimize each of the models individually.
Thomas: Okay. So do you take into account what you know about the client? I mean, would you serve a different recipe to different categories of client potentially based on, for example, whether it's worth upscaling on the client or what upscaling the client might have available? It might well be a very different experience watching on a large home television versus watching on an iPad or a laptop.
[00:21:10 Serve different recipes to different categories of clients]
Zhi: Right, absolutely. I think there's always an ecosystem of the devices that we have to take into account and the way is to look at the spread. And there are high quality upscaler and there are low quality upscaler and we cannot optimize for upscaling that's already super high quality. I think the way we tackle is, is by looking at the medium. So if we can find a little bit more conservative, we're hoping that we can operate somewhere in the middle. I think to be more specific, in the case of the upscaler, I think state-of-the-art, there are always machine learning based, super fancy upscaler.
More traditionally we do have Lanczos, bicubic and bilinear, so somewhere in the middle that we choose to optimize against is mostly on bicubic. We kind of like looking more conservatively at bicubic and bilinear together, if we can devise the solution. That's basically the assumption we make. This is something we use for our VMAF calculation as well. We kind of talked about this in one of our tech blogs that we assume that if the upscaling is bicubic, that's recommended that way, we recommend people how to calculate VMAF score by assuming a certain median kind of an upscaling.
Thomas: Yeah, so to talk a bit more about VMAF in particular, it's often treated as a kind of oracle or black box and it's deemed to be a machine learning based algorithm, but it has a number of components, doesn't it? Perhaps you could talk about what's involved in it and how it's put together and demystify it a little bit.
Zhi: Right, so let me just go back to the history of this project, VMAF. As many of you might already know, this started as a university collaboration project back in 2013, '14, starting with University of Southern California, Jay Kuo's group. So the original motivation for us is a need for an automated way to evaluate video quality on the per video stream basis, video encoded stream basis. Obviously we cannot do that manually, right, using humans. It's also not sufficient for us to just use a metric doing a codec evaluation based on a subset of the corpus, using just a single dataset to make sure that that codec works. Because every single stream matters to us. Especially once being created, it's gonna be streamed over so many, many times. It's worse for us to evaluate each single one of them.
So we want to do this at scale, but when we surveyed existing video quality metrics solutions in the field back then, none of them actually met our needs. Taking the simple PSNR for example, I think it's still being used extensively for codec evaluation. But one of the main pain points using PSNR is when you're comparing across different content. Just PSNR on this video piece and PSNR value on the other video piece. There is no coherent notion of what is the score threshold for when we mean by excellent quality. It's especially hard when you're trying to do that comparison across content. So there's a need for that, and it is a motivation we started working with Jay Kuo's group deriving a solution. And so this project actually started before I joined.
So when I was at Netflix there had already been a summer internship project and there's a prototype. I would give lots of credit to that prototype. I think it essentially established a framework, the fusion based framework based on machine learning but also relying on a preliminary round of feature selection that's pretty adequate back then. But of course as a research prototype there are always many holes that you have to bridge before you can take these things into production. So we actually spent two years refining that solution, like filling the holes, right, and to make it production ready. And that's when we have this first open source version in 2016 and then we continue working and working on it and keep on refining, release new versions.
I think in the meantime we get lots of help from other university collaborators. One is UT Austin and the other one is University of Nantes from France. So there are Al Bovik's group from UT Austin and Patrick Le Callet's group from University of Nantes, France. So the version that has been released in 2017, I would call it, is the algorithm that has remained stable as of today. At least in the open source fashion. So that's on purpose because we know in order for this adoption to take place, I think we need to have a stable version such that one thing is, I think there is a stable comparison of the results and in the meantime, I think it gives the open source community to give us a space to help us to optimize the performance.
Instead of having a moving target, we want to have a fixed target. And so the basic algorithm is there, but we are building additional tools into it, like additional arsenal to make the observability better. One example is we're building, like how we quantify the confidence interval, right, for the prediction of VMAF and we develop ways to do that and we have other tools, like you can use in our open source package, we have a tool called Local Explainer. This is essentially based on a machine learning framework to create explanatory results from your prediction called LIME, L-I-M-E.
Using this approach, you can, even if it's a black box, for example SVM, you could kind of extract a localized interpretation of how much weight you have for each of the features. That will give you an idea of whether this is a feature that's worth keeping. So that will help us to build extra confidence on the features we're selecting in there. And we're also continuing building, extending the model into different viewing conditions, one example. For example, like a phone VMAF model is a model that I think works better when you're viewing on the phone.
So I think there is an assumption about the relative viewing distances versus the screen size. So on the phone, 1080p probably doesn't look too differently from 720p. So that model captured this effort. So we keep on expanding on that and in 2020 we introduced another model called VMAF NEG model. NEG stands for no enhancement gain. So this is motivated by using VMAF to be more tailored for the codec evaluation purposes, especially for proprietary codec where you cannot strictly separate the pre-processing which contribute to the enhancement effects on the video versus like the actual coding game.
As a human observer, I think humans really respond to both. If you are applying enhancement, humans would consider it as a higher quality, but you can possibly abuse this enhancement because beyond a certain threshold, if you're overdoing your sharpening, things could become worse. And so the motivation is to... Like disabling that enhancement effects such that it will become a fair comparison, it's a pure evaluation of the capacity of the codec itself.
Thomas: Yeah, one thing that we've noticed a lot, you know, building encoders is that it has come to dominate a lot of the way that people develop in encoders as well and maybe you could describe it as a certain amount of trying to gain the metric or trying to optimize VMAF figures by doing things like pre-processing and so on. Do you think it's possible to exploit VMAF a little bit in some ways, you know, that in there are some parts of it, there may be if things could actually look worse even if they get higher VMAF scores? Or do you think it's pretty robust to that kind of treatment and behavior?
[00:32:07 Gray zone of VMAF]
Zhi: Well, I will say for the initial version of VMAF actually, it's a little bit tricky to describe because I don't think there is a black and white. There's a gray zone, about what kind of pre-processing or what kind of processing can apply to the video. Even in today's modern codec, you have filtering within, in-loop filtering. And you do see quality improvements over, by doing those operations. And I think that this can be reflected in terms of PSNR. You could even get a higher PSNR value of this. If you got a better human score and you get a higher PSNR, it's probably give you a high confidence that this solution works.
So I guess the question is more on the gray zone. So what if you're having resulted in a higher perceptual score? I think it can be reflected through subjective experiments but it gives you sort of like lowering score, right, PSNR. How do you think about that? And in that regard, VMAF might give you a slightly different interpretation of that score. So by design, VMAF is not trying to measure the fidelity of the signal as compared to the original signal. It's not like a distortion metric. If you closely examine the formula that has been used in its features, right, you can always interpret that ratio. It's always a ratio between two terms and each of the terms, it's essentially accumulating energy. And you're looking at the numerator versus the denominator, how much energy, one compared to the other.
That's the formula that makes VMAF work. It's not to measure how close two signals are, the reference versus the distorted. So that gives the VMAF the power to be more closely to how humans perceive quality and that's why it can also help to explain why when you're having enhancement, you're applying sharpening. You're optimizing local distribution of the energy within your video picture and it kind of can capture that effect.
On the other hand, we all know that if you're overly sharpened or overly applying contrast enhancement, that you could have a negative impact. The key is, like to what extent you are allowing those enhancements to happen. I think up to today it's still an open question there. I don't think VMAF sufficiently addresses that. We do hope in future VMAF versions we'll be able to have a better prediction along those lines.
Thomas: Hm. And so-
Zoe: So, I'm a bit curious. Thomas you can go first and then I'll raise my question after your question.
Thomas: Yeah, so I was kind of interested what kind of focus you had when you were developing and training VMAF. Was it very much at this very high quality asymmetric scenario that you describe where you're really looking ideally for something visually lossless or at least visually lossless for the resolution that you are able to encode? Or did you consider, you know, a larger span of compression ratios where there might be visible artifacts for example or there might be quite a lot of softness that the encoder would introduce? Was it very much focused on your use case or did you look at much, much wider range of use cases?
Zhi: Right, so VMAF was developed with the use case of adaptive streaming that we have in mind. So in adaptive streaming we're building up this bitrate ladder which covers different bit rates which tailor to, depending on the user, network condition, we have to select the right representation with a proper bit rate and with a proper video quality. So the aim is really to look at a span of the quality range. I think in the more technical terms we're looking at a supra-threshold instead of a near-threshold. It's like really, the span of a wide range, how you're aligned that right range of quality, how is that compared to how human rating? In other words, it's really trying to predict how the subjective rating of those videos at the wide separation of video quality, picture quality is gonna be, that's the original motivation.
Zoe: Yeah. So I just go back to my own question because you mentioned, I really like that statement that VMAF is not only just a distortion measurement between two signals, right? It's not just a encoded version as opposed to at least a source or another version fed into the encoder. And then you mentioned that really wanna have this measurement at least aligned with the human visual system perception so that I look at the VMAF and VMAF NEG, well, VMAF NEG. Basically you mentioned that the VMAF, because sometimes oversharpening and contrast needs overdue, then VMAF scores not quite aligned with, I think, subjective quality. 'Cause you might have a very good objective score but then subjective evaluation may be kind of in opposite result.
But on the other side I was thinking maybe for VMAF NEG because you said that it's try not to consider the pre-processing or processing effect but you focus on VMAF to align with subjective quality evaluation. So by ignoring that part, maybe VMAF NEG could be a little overdue from the opposite direction. So I want to have your opinion comparing VMAF and VMAF NEG. On the other side, I also mentioned why you have two down there because eventually we really want to have one score to really matter, not only the distortion but also align with subject quality evaluation. So this is my question on how do you think about these two quality metrics at this moment?
Zhi: Right. Yeah, I have to make a disclaimer that the VMAF capturing that enhancement effect is not the original intention of how we bring those features into the picture. I think the motivation is mainly driven by their performance, in terms of how well their predictive power against the human rating. That was the original motivation and when we bring them in, I think the major use cases for us are still like within our encoding pipeline for professionally generated content. And I think in that regard, as long as our encoding pipeline has been concerned, for most of the cases when you're looking at encodes with VMAF or with VMAF NEG, they yield very, very similar results.
And it is actually, I have to thank you, Zoe, because you are the one who brought me the attention about how VMAF has been used in the wild beyond Netflix use case. I think that conversation was about, that was many years ago actually. That was the first time it got my attention if you can remember that, because I haven't really thought about beyond our use cases how people would try to come up with a very inventive way to use VMAF and to guide their performance. And what would be the kind of a typical encoding pipeline people use in the wild. When it comes to professionals, like a user generated content or gaming content. There are many things we're not aware about and it's actually thanks to you that bring our attention to that.
But we also realize this actually becomes a problem when it comes to code evaluation because it's proprietary. It's close to the source and we don't really have a good way to separate what will be like essentially in the encoding versus the pre-processing. And that motivates us to have this additional model. So we're not proposing to completely eliminate the original model because we believe there is value. Because like, whatever it takes to make the prediction more aligned with a human perception, I think that's a good thing to have and that's what we intend to keep in there.
But like, based on the specific need and we think there's value to introduce the second version that can be tailored. So as of today, even internal code evaluation purposes, we look at both metrics. We're not just looking at one number, we're also looking at PSNR. PSNR is the picture and we're also relying on subject evaluation. We look at everything holistically to decide whether this is a solution that warrants its merit.
Zoe: Right, thank you. Actually, you did mention that, again, that I would like to bring out is even though here we talk about the Netflix like PGC type pop content, yes, VMAF has been widely considered to all, like especially we would say user generated content or half PGC and half UGC 'cause everybody's looking for, with the explosion of video volume, the right objective quality score because there's no way, ideally we really want to like the human justification. Even human justification, you have to set up the right, I think subjective evaluation environment.
But video is huge and we need the simplest, which is one single score to evaluate that.
We really appreciate, indeed, VMAF, the contribution in this field. At least I think right now, we'll say that so far VMAF in many cases has been regarded as the one that most lay aligned with the subjective evaluation even though it's not perfect.
But on the other side, I think because of this way at least is a bring in the motion, excuse me, the motion learning factor because you want that to be aligned with human justification and there's some learning part down there. And that one is also I think, at least some motion learning driving technologies that see the really applicable to a usable metric or product that actually benefit the field. So along that line I just mentioned because everybody talk about generative AI, so I want to learn what your views, not into academia, but potentially how Netflix for your team considered about that at this moment, the both codec and metric wise.
[00:44:38 Machine learning based model]
Zhi: Since we start talking about the topic of machine learning, let me first make a disclaimer on VMAFs, let's still talk about VMAF on that front. There's a general perception that VMAF is a machine learning based model. And we have always made the claim that we've been trained VMAF based on professionally generated content. And one of the worries is that if it's being trained on professionally generated content, what if? What if, can this be applied to user generated content or gaming or et cetera, et cetera.
There has always been this worry and I think it's good that we make the clarification that there is the rule of thumb that we have been using internally, mostly internally, is that if you think about the predictive power of VMAF, maybe about 30% of them can be attributed to the machine learning based model. And 70% is actually thanks to it's the elementary feature that was incorporated into VMAF, which is essentially modeled in the human vision system. I'm talking about the VIF feature and the DLM feature in there and what the machine learning model contributes essentially. I think it's a big weight on deciding on how much we should put in the weight of the temporal feature, right, the motion.
Like motion feature is actually pretty simple one, but I think it's play essential role in the VMAF model because it really decide on like up to what extent when the picture is moving the human still be able to perceive the coding artifacts in it. I think it's a big contributing factor to how VMAF is different from the more traditional PSNR or SSIM, which is essentially only a static image based metric. Of course there are like a video version of them. But, I mean, there are different kinds of perception on how well they perform versus their complexity that we really adapted a fairly low complexity version but it turns out to be working pretty well.
So that was a digression into this, what machine learning gives you, but I would still consider VMAF as a psycho visual based model because the building block it is riding on is essentially standing on the shoulder of the giants. That's based on hundreds of years of human vision research.
Zoe: But particularly I want to mention because you particularly mention, so here's the thing. So whenever we actually introduce you even to our team, I always bring out the first at least the VMAF tech blog written by your team, Netflix. 'Cause you are the first author and then but actually today, this is the first time I hear directly from you as a first author of that first VMAF blog that actually before you joined the team actually there's some essential features already being decided at early stage, developing VMAF.
And then you also emphasize that that actually gave the foundation of the VMAF, even though the model was finalized earlier. There's some refinement version like VMAF NEG but you still try to keep the original version. You also mentioned right now, 70% actually of the VMAF success contributing to actually attribute to those 70% features. So this is actually the first time, it's actually to me as a new knowledge, I believe it to quite some audience right now listening to our podcast actually. So we appreciate that. We appreciate the features actually right now contributing to VMAF. I just want to emphasize that.
Zhi: That's why we're here, to demystify VMAF, right?
Zoe: Right, yeah. Actually I want to respect the time and then this is some, I know we're going to move a little bit on the at least 30% from machine learning 'cause it's just simply so hot at this moment because everybody knows that, because the ChatGPT generative AI is down there. So we just want to, maybe you can only touch the surface at this moment. 'cause between the time also because some other things we just want to hear a little bit of thoughts from you on that direction.
Zhi: Right, so it's a big topic that's even beyond what we've been talking about video encoding and the video quality. I mean within every single one of the tech companies these days, I think it's a big topic for everybody too, like how we can cope with the new wave of technological improvements and everybody's being afraid of being left behind. And I think it's a much bigger discussion beyond what we can discuss here. But I can probably just focus on this particular domain on video encoding, right, on that new technology in this front. I think to us, I think it is still a very big consideration, like how much we should respect the creative intent.
Artistic expression from the artist is when it comes to professionally created and curated content, I think that's one of the top priorities in our minds. And some of the technology, I think when it's being used for content creation, that's beyond that consideration here. But for us it's like we're given the source ingested to us. What can we deal with? I think there are some certain levels of leverage we can use, but probably, I think we have always been mindful about that creative artistic intent aspect of it. And just take, I don't think there is any clear cut on what is allowed and what is not allowed, just given this video scaling as an example.
Given a low resolution version of video, pretty soon you might be discovering that you're going beyond, we're trying to recreate a high detailed, high resolution version. Once this multiplication factor becomes slightly larger beyond the 2x for example, I think there's no way we can continue to keep on refining this performance without hallucinating the content. Some of the details might need to be recreated and it's just still an open question and a very tricky question on what will be allowed versus what wouldn't be allowed. So let's just keep it an open question. I don't have an answer to that.
Zoe: Well, at least you brought out some of the essential problems in that field. And then talking about arts and then I'll leave one more question to Thomas from that side. But before that I just want to bring the background 'cause you have art, you have two poster, I will say one poster on the side and then there's some artist at the background. So do you want to talk a little bit about that because it's actually we don't have a background replacement down here.
Zhi: Right, I don't... Okay, I didn't really stage my background in order to talk about those, just as a disclaimer. But if you can see, the poster is actually a Netflix show called the "Santa Clarita Diet." It's a horror comedy show I like quite well. I think it was made a few years back and it has three seasons. Unfortunately, it didn’t get renewed for the fourth season.
But if you haven't watched it, give it a try. I think you might like it. And it's the other ones, the photograph you are referring to framed on my background. So that's a photograph by Ansel Adams, which is a landscape photography. I think he mostly did his work in the 1940s. This is one of the pictures about the Sierra Nevada in the inland of California, I think I'm not entirely sure I would be through that place. I might, but this is one of the things like almost 70 years ago was made, 70 years ago.
Zoe: Yeah, I was sitting here. I just look at your background and seeing and thinking that actually for the scene on your window is typical head and shoulder. That's not too hard to compress in terms of video codec wise. But then because of those two artworks, if really people want to learn what they are, what they talk about, what they present, maybe when the lower bit weight size and I'm not sure how they look like because of the compression when we talk about the really perceptual significant area focusing on the head and shoulders. That's just what I was thinking. So that's why I was just asking bring the audience to the attention if they happen to not just listen but actually working the videos that we share for this episode. And Thomas, you have-
Zhi: Definitely hope there is no banding in the background.
Zoe: Yeah, we're talking about banding, sound background. Yeah. All right. So Thomas, do you have any.?
Thomas: I guess I would want to ask you, thinking about your work on AV2, AVM and your work also on optimizing your AV1 chain. How long do you think we can carry on with the progress that we've been making in recent decades of doubling in coder performance or codec performance, every generation? Do you think we're close to the limit or do you think there's a generation beyond AV2 where we could still get very significant compression improvements maybe using AI type techniques?
[00:55:40 Trade the performance versus the complexity]
Zhi: Right. So first of all, I don't think I have as steep a knowledge on the codec space as some of my teammates who have been working on this codec world for decades. I think my answer might be based on my general observation in this domain. I think, essentially where we've been operating today is to trade the performance versus the complexity, essentially in which those tools allow them to have a higher complexity to squeeze more coding gains out of it, more encoding modes or smart selection of the coding tools. I think that's contributing to the gains we have today. I'm not entirely sure, because I think the source coding, if you're going back to the information theory point of view, I think it's not as well defined as channel coding.
I think in channel coding, once you have a model of the channel, things suddenly become very clear. Like everything is bound to the Shannon theory. I think, in the source coding domain, right, you do have rate-distortion theory but I think because of the difference between how the distortion is defined mostly as a quadratic distortion essentially PSNR. But we know the human vision system is so much more than that. I think this might be the domain that has not been completely exported and because of that, I don't know if we have a very clear upper bound where this thing is, right, and going forward also, I think there is a relatively touched on philosophical point of view on what can be considered as a compression versus generative.
And if you start to think about, like creating a dictionary of many different contents, you can start to replace components not in your original picture and what we call the generative compression domain. You can easily imagine there are ways you can go beyond the current paradigm in coding, but it's not magical. Even the term we use, hallucination, seems to be very mystical, but it's not, it's really not. So I think from a system point of view, this is well understood, like how things have been exploited. In other words, I think depending on all the definitions and the problem space we're being looking at, the answer is not very clear on whether we've been achieving the limit. I think if we're allowed to have a more flexible definition of video compression, I think there's definitely much, much more we can explore moving forward.
Zoe: Yeah, I actually believe so.
Thomas: Thank you very much.
Zoe: Yeah, thank you for addressing that. 'cause on the other side I also mention that I still remember here in this lab, I actually pursued my PhD down here in this lab between 2000 and 2004. So at that time HL224 ABC was just finalized in 2003 and I was trying to run the, actually the GM, I believe the code base is really slow. Then I try to actually just focus on the QC and most the C for videos at this moment. So resolution is really low. And then 315 or even timeframe per second, that's what we were considered, so because we are bundled, we actually limited by underlying hardware. So on the other side with the computation resources is actually along that lines booming. Right now we have GPU.
So that's why I think that boost out a lot of machine learning applications. We also looking forward a new computational way from the hardware resources. Along all these ways together, I think we all here really believe that this new technology that will come out down the way with more computation available. So compressions, maybe there's a lot more we see that's complicated, but down the road could be a lot easier and simpler to obtain new ways of compression maybe potentially come out. But as you mentioned that we may have to follow the underlying theories 'cause we are living in this universe. We have to follow the laws in this universe. That's some view, yeah, from my side. And we actually already-
Zhi: If I can add one thing.
Zoe: Yes.
[01:00:44 The human appetite for better and better content quality is progressing over time]
Zhi: I think since you mentioned this, I think technology has been progressing. We've got a better computational power for encoding and decoding. We have a better network bandwidth over time. Maybe in the near future the bandwidth is growing faster than the rate we need for the compression. On the other hand, I do want to mention that the human appetite for better and better content quality is progressing over time as well.
I think if you just imagine 10 years ago. 1080p is the state-of-the-art, like the kind of resolution you can provide or maybe, I mean, 15 years ago probably, but now we're approaching 4K and many other technologies. HDR is taking a high frame rate. I think humans have this appetite for better and better experiences. So I think there's still a battle to combat against the human appetite to be more hungry for the better experience. I think the other consideration we haven't really talked about today is like, what about sustainability.
I think like to create those experiences, there's also the aspect about like, what is the carbon footprint we have onto the world. I think we should be a little bit conscious about that too, right I think enabling some of the technology is really very power hungry, that's something we'd really want to be mindful about too. I think most relevant to us is we should be more conscious about encoding and decoding complexity. And beyond that codec perspective, there is an aspect about how the content got distributed, right, and being displayed, being rendered. I think the latest technology could potentially consume more power and we should be mindful about that too.
Zoe: Right, so we all mentioned that nowadays video code are not only just evaluated by the bit rate, quality, speed, delay, but also underlying computational resources, right? Like efficiency, I would say CPO efficiency because we all want to have a green energy in mind regarding a codec development. But then just like you also mentioned that for videos we actually have the needs from the users. They're always greedy, hungry for better video experiences and other side is all kinds of different format as you mentioned. It would be right now even the dimension have boosted to 4K or even higher and then they're always potentially 3D videos, 360 videos, all kinds of different format of videos.
This is still some work that means there's some potential opportunities definitely in this field and we really appreciate actually you come to this episode. We know, because of the time and then also because there's always something we can pursue, there's quite some open problems down there. And then down the road we can actually keep this discussion and we may invite you or other team members with others to talk about the same topics. 'Cause down the road, again, not only in this episode but 'cause with all these kind of discussions openly in this community. And so I need to put actually a closure on this episode. Really thank you for your time to come to this episode and also come, thanks for my co-host Thomas from London and thanks everyone for our audience. We'll see you next time. Thank you.
Zhi: Thank you. Thanks for having me.
Zoe: Bye. Thank you.
Thomas: Thank you, bye-bye.