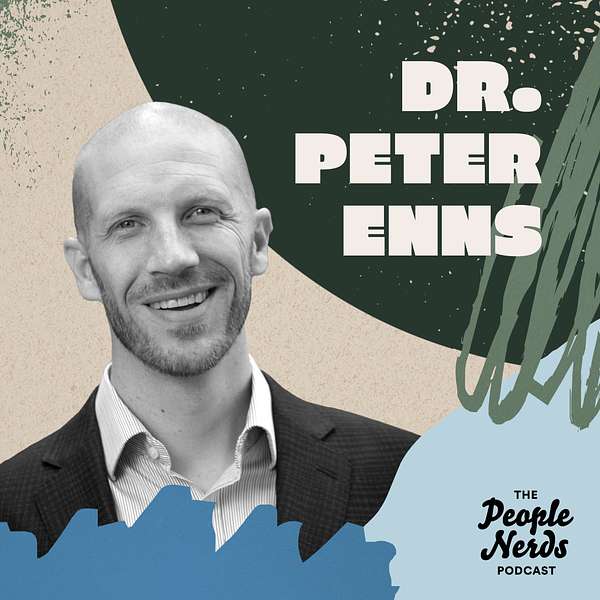
People Nerds by Dscout
The People Nerds blog you know and love, now in your headphones!
People Nerds by Dscout
Data Decisions (w/ Dr. Peter Enns)
Big sample, big impact...right? It's not so simple...
Large samples don't automatically produce more valid, useful outcomes. Survey design, sample representativeness, participant incentive structures, and analysis plan all impact the results. What can mixed-method, qual-leaning researchers learn from this fact?
On this episode, we're joined by Dr. Peter K. Enns, a professor of Government and Public Policy at Cornell University (where he also serves as Director for the Center for Public Opinion and the Center for Social Sciences).
Dr. Enns spends a lot of his time thinking about the impact of his conclusions, because of their political, material, and policy implications. In addition to his work at Cornell, he is a cofounder of Verasight, a consumer insights firm.
He outlines the ways we can collect more representative data that's also less likely to produce spurious conclusions. Experience pros will leave a sharper sense of data hygiene and ways to foster a relationship with the users who make their practices possible.
Show Notes:
Dr. Enns' work, including his books Hijacking the Agenda and Representation Nation
Dr. Katherine Cramer discusses listening in her political science research
Peter:
The more we can move away from what's easy to observe and really drill down to evidence of high quality data, and again, one of the top ones is population benchmarks. We did some work and they said, "Here's some data we've used. We're really happy with it." We analyzed the demographics. The demographics didn't align with the population. They were trying to have a representative picture of the US and the demographics didn't even match up. And we were able to say, "Are you trying to reach 80% women? Because if you're not, you are making bad decisions based on bad data." There are a lot of ways to evaluate data quality, but got to push on that because bad data leads to bad decisions.
Ben:
Welcome to the People Nerds podcast, expanding your human-centered practice with unexpected sources of wisdom. I am Ben, joined as always by my colleague and friend, Karen. Hey, Karen.
Karen:
Hey Ben, how's it going?
Ben:
Not too bad. I'm very excited for today's pod because today we're going big. Big data that is. Huh? Yeah. Not just big data, but big data with public policy implications. You want to tell us a little bit more what I mean there, Karen?
Karen:
Yeah, absolutely, Ben. Today, we are talking political science and we are talking public policy research. We were super excited to delve into this topic because most of us in the user research experience work largely or almost entirely with qualitative research or at best a mixed methods research with still relatively small sample sizes. Maybe sample sizes in the hundreds, but most likely sample sizes with N=8 or N=20, something along those lines. There is so much rich and valuable data to gather from those sizes as well as really crucial methods that can only be conducted at that size, like moderated usability, one-on-one interviews tend to be smaller. However, this is only one end of a very broad research spectrum.
Karen:
Today, we are reaching all the way to the other end to talk big sample survey science with end sizes in the thousands, tens of thousands, or in some cases, even hundreds of thousands. We are super excited to dive into this because although we are on these polar opposite ends, there are a lot of questions that concern both of us, right? Questions of rigor, questions of data quality and data sourcing, and questions of how do I know that my answers, my recommendations, my insights are the right ones that are actually going to push our organizations forward and not steer them in the wrong direction because the data that I got was not the right data or the insights I pulled ended up not being the right insights.
Ben:
And to help us start to make sense of those questions, we are joined by Dr. Peter K. Enns. Now he has got a big data size blurb. Bear with me as I read it. Lots of cool things that he's working on. Peter K. Enns is a professor of government and a professor of public policy at Cornell University and Robert S. Harrison Director of the Cornell Center for Social Sciences. He is also the executive director of the Roper Center for Public Opinion Research and a co-founder of the consumer insights company, Verasight. Dr. Enns's research focuses on public opinion, political representation, mass incarceration, the legal system, and certainly the topic of the day, data analytics. He is the author of most recently "Hijacking the Agenda: Economic Power and Political Influence," which is the winner of the 2022 Gladys M. Kammerer Award, which is presented by the American Political Science Association to honor the best book published during the previous calendar year. Again, a person with a very full plate, a lot of things that he's working on, and someone who's expertly positioned to help us begin navigating some of these questions.
Karen:
That's right. And we could not ask for a better person to talk to you about these topics. We are so lucky that we got an hour of his time among all of these fantastic pursuits, so let's take it away and hear our conversation with Peter Enns.
Ben:
We are excited to welcome to the pod, Peter Enns. Welcome my friend.
Peter:
Thank you. Great to be here.
Ben:
We have so much that we want to dive with you into, public policy research, your methodological choices, certainly the notions and questions around representativeness in generalizability, but for our audience who might not be that familiar with public policy as a research practice, could you talk a little bit about what that means? What sorts of research practices you do? What does that mean to be a policy researcher?
Peter:
Yeah, sure. Happy to dive into that. At the most basic level, we're thinking about public policies. My particular focus tends to be within the United States, but I think that the key aspect for me is trying to understand real world questions, real world puzzles with impact. And I suspect that aligns with a lot of your listeners and it may not be in the academic realm. Most of my research or much of it is from in the academic sphere, but the focus on real world questions and puzzles is what I'm interested in.
Ben:
And you use quantitative methods specifically. That's another through line we're hoping to pull throughout our conversation here. Could you describe, I guess, is there a qualitative public policy practice, or asked another way, why was quant your methodological home or the way that you approach your policy questions?
Peter:
Yeah, yeah. No, that's great. No, I think quantitative and qualitative analysis play a really important role in public policy research, in the social sciences. And it's just happens to be, I think, my interest and, at this point, maybe my skillset.
Ben:
Sure.
Peter:
But I've also dove at times into archival research. My previous book, "Incarceration Nation," it was looking at understanding the rise of mass incarceration in the United States. Most of the focus was with quantitative data, but also going back to archives of Nixon's campaign and looking at their strategy and how they were using public opinion data from internal memos.
Karen:
Oh, interesting.
Peter:
Because I was showing the relationship between public opinion, how punitive the public was over a 60 year period with the rise of mass incarceration and arguing that public opinion played a really important part. And so to buttress that argument, I said, "Well, I have empirical evidence that policy and the criminal legal system is responding to shifts in public opinion," but how do I dive into the actual mechanism? How do I know they were looking at the polls? And turns out you can go into the archives, see their campaign memos, and so in my book, "Incarceration Nation," I actually have images of specific memos referencing specific polling results.
Karen:
That is fascinating. One thing that comes up a lot in the UX research world is that the quantitative side can tell you what. It can give you these high level correlations or showing patterns in some ways, but that there's something about qualitative. It can really get into the nitty gritty of why. What is the mechanism that is connecting these high level trends that you're seeing?
Peter:
Yeah, absolutely. And I think they're both very important. And I think what tends to happen, in my case, with that book and that research, I recognize the need. And so I did some archival work. Other times people collaborate together, bringing different skill sets. And I think that team orientation, that collaboration, whether it's academic research or the business sphere needs to come together. And I think the other thing that's really critical is just making sure the methods align with the goals of the research. And some people are like, should we do quantitative? Should we do qualitative? It depends on the research question and what we're trying to do. I don't view it as a hierarchy. But within specific applications, one method or certain methods may definitely be more suitable, but that isn't a broad statement about the applicability of various methods.
Karen:
And actually, I was wondering if you could say a little bit more about that when it comes to the work that you do, quantitatively, what are the kinds of questions that come up in your work that you answer through these larger scale survey I'm presuming? I wonder if you could talk actually a little bit too about the nitty gritty of what actually your quant looks like when the rubber hits the road and you're starting a study.
Peter:
Yeah, yeah. Absolutely. Most of my research relates to public opinion in some way, and that's using survey data and sometimes what that looks like is collecting original survey data, conducting the research. Other times it's using existing public opinion data. One great source for that at Cornell is the Roper Center for... So Cornell where I'm faculty, the Roper Center for Public Opinion Research. And this is the world's largest public opinion data archive.
Ben:
Wow.
Peter:
Essentially data from around the world, from the US back to 1935. This is a treasure trove of opinion data, and I've done a lot of analyses looking at surveys over time, pooling data together, and it really fuels a lot of approaches. Back to looking at the rise of mass incarceration, if you're trying to understand change, you need over time data. There I was taking public opinion surveys from the 50s to today. Another example, before the last presidential election, with one of my PhD students, Julius Lagodny, we did a forecast of the election. There, we used some economic data and we used some opinion data. And we combined those together to build our forecasting model at the state level. There, we were combining hundreds upon hundreds of surveys, which leads to hundreds upon hundreds of thousands of responses over time and what we did there, our forecast, we released that more than 100 days before the election and correctly forecasted 49 out of 50 states. We were pretty excited with that was result.
Karen:
Wow.
Ben:
Peter, first of all, kudos to you. That is really something. From 2016 on, we've heard so much about the unpredictability of, the ranging confidences of polls, so kudos to you and your co-authors there. Getting back to Karen's point about the what versus the how of quant versus qual and you're piggybacking on and saying that team based work can often combine those approaches, could you give us an example of a time when you used maybe a focus group or interview, what does qual look like in that policy space?
Peter:
I think it's really often that deep dive where you need more information from a few sources, whether it's an existing archive or talking to a person longer, versus I want breadth of information that can't quite go as deep. And so really hearing why people give the, so if we're going back to the survey research world, why people give the responses they give, that's where I think a focus group is ideal. Letting them hear how they talk, hear what they say. A really neat example of this, Kathy Kramer is a professor at the University of Wisconsin. She does what she calls in some of her research, listening investigations. She will go to where people are just sitting and talking and they could be at a restaurant, at a coffee shop. Sometimes it's outside of a gas station and she'll just be on the edge of the group and listening, and then ease over and say, "Hey, do you mind if I listen a little bit more formally?"
Peter:
And the idea there is not only to get more in depth, but to also hear people talk about subject matters, talk about policy issues in the environment and the framework they normally would. And so that's a really neat idea. Now, the counter to that, I've done quite a bit of work in public opinion surveys. And some of this is my own research. Some of this is Verasight, the company I help co-found, which we could talk about if you'd like. One thing I love is asking single words. What word comes to mind? And that's beyond the standard public opinion question where it's multiple choice, but constraining it to a single word. And just that, what is that top of mind consideration? We asked, I've done quite a bit of work, who would you like to most see run on the Democratic ticket or the Republican ticket?
Peter:
Just that top of mind, what name is said, turns out to be incredibly predictive, even two years in advance of who ends up running. Because what you're measuring is name recognition, top of mind considerations, valence considerations. And so that's the distribution. You can go from in this sphere of research, listening to people talk with their friends or peers or coworkers in their exact setting, all the way to a very large end, big data survey, what word comes to mind. And that distribution gives so much information across those two extremes.
Karen:
That is so fascinating that even just a one word open-end has that much power. That's one thing that at DSCOUT, we kind of, not quantum the scale that you're doing it by any means, but we tend to go a little bit more quantitative than some folks who are used to only running interviews or only running focus groups or something like that. We do offer close-ended options. We offer slightly larger sample sizes, and we're often pushing, Hey, you don't need to ask a paragraph long question or you don't need to get five minutes of video response to learn what you need to learn. Sometimes it really is just what's the one word that comes to your mind? Or what's three words you'd use to describe this thing? And you can get a lot of mileage out of not very much there.
Peter:
Yeah. And I think the best case scenario is when you can then validate it against something else. And that's a lot of my research is thinking about measurement, thinking about validation, and also being flexible with the methods. Even if most of my research relates to public opinion and survey research in some sense, that's not always the case.
Ben:
I'm wondering if you might wax a bit on rigor. In the UX world an in the experience space, we hear a lot about sample sizes. And we're going to talk a bit about representativeness and generalizability. That's really one of the key drivers for having you on, as someone who thinks about representation, thinks about the generalizability of their claims. But I'm wondering before we jump there, because you sound a bit like a psychometrician, someone who's thinking about particular questions, the orders of those questions, the balance of those questions, what does rigor in quantitative work in your research mean? How do you define that? How do you know when you see it?
Peter:
I don't have a simple answer to that, Ben. I think a few things, one is the data source or data sources. It's is it the appropriate analysis, but that doesn't necessarily mean a sophisticated or complicated methodological analysis and no matter what the analysis has done, if the initial data either aren't the right data or aren't measured correctly, or don't relate close enough, that's a first test. The second, I'm really looking for an explanation of the methodological choices. And that's for me to both understand what was done, but also when we're thinking about quantitative analysis, there's often statistical tests to try to conclude... We might hear is group A different than group B? Or do people like this product more than this other product or this design? Ultimately, there's often statistical tests. All right. And the logic of a statistical test is we often talk about statistical significance.
Peter:
And what we're basically saying is how often we think we could have reached this conclusion incorrectly. Every single choice is essentially a different analysis. Every potential choice is another potential analysis. And what that means is if we splice our choices and analyses enough different ways, we're going to find a statistically significant result at some point. And so looking at what choices were made, when in the research process were they made, why were they made, isn't just helping us understand the rigor of the research and the analytic decisions, but also, it's a little bit of a glimpse into how many potential analyses could have been done, which speaks to the potential false positive rate, or could we have got a spurious statistically significant result? And so in rigor, I'm looking at, I guess to summarize, the data source and thinking about the data and the quality of the data, measurement's always important, and then what choices were made in the analysis that was done and when were they made and why?
Karen:
That is so fascinating. And I think really crucially, even for smaller or even more qualitative methods to have that in mind, of when are you making the choices to analyze the way that you do? But one of the other things I'm really interested to hear more about is this question of measurement. I think earlier you said something like, are we sure that this is measuring what it purports to be measuring? I was wondering if you could speak more about that in terms of survey design. What's an example of something measuring correctly versus maybe not measuring what it wants to be measuring.
Peter:
Yeah. Yeah. And at the most basic level is often language. Are we asking the survey question, and this would apply to focus groups too, in the way that our respondents of interest typically think about the issue? So that's one area. Another area relates to what we're trying to understand. And I'll give an example in the field of criminal justice, criminal legal system that relates to the book I mentioned, my previous book, "Incarceration Nation." For a long time, scholars said the public did not have coherent attitudes about criminal justice. And the reason is if you asked the public should courts and judges be more punitive? Should they give harsher sentences? You would always see a lot of support for this. Even a lot of support for the death penalty. If you asked, should prisons be more rehabilitative? You also saw a lot of support. What scholars would do is point to these similar questions that would yield seemingly conflicting results. What I did, I tracked these questions over time.
Peter:
And what I showed is even though you would get different levels of support, the public becomes more punitive or less punitive over time in parallel. This is your standard question wording effect, how we frame the question affects how responses look. But if we ask the same question over time, we can measure whether responses are going in one direction, in this case, more punitive or the other, less punitive. I was able to show over time all these questions, which were thought to be evidence of incoherent public attitudes, tell a very coherent, streamlined story of what direction public opinion is changing. And so this comes back to this measurement question, Karen, of what are we trying to measure? Are we trying to understand a snapshot of the public's views at any one time? And the views can be complicated. People often hold conflicting views. Or are we trying to understand is public opinion changing? And now, all these different survey questions reveal the same story about the direction of public opinion.
Peter:
And for me, back to this conversation thread of public policy, policy and politicians tend to follow changes in public opinion. If you get elected, you are elected based on voters at that time. If those voters change their opinion, usually you need to update your position to not lose voters in the next round. It's opinion change that's driving the political system and we can measure opinion change through surveys over time. But we've got to look at that overtime change where people were looking at a single snapshot, measuring something they weren't intending to and reaching the wrong conclusion. It's about question wording, but it's about more than that. Are we trying to understand public opinion at a certain time? Are we trying to understand how it changes? Look, I'm talking about policy and the political world, but if you're trying to understand consumers' views of the economy, what product or brand is about to take off, it's all about change and that needs to drive the measurement strategy.
Ben:
We are seeing an influx of designers, of marketing folks, of product folks. I'm using air quotes here, listeners, doing research. They might not be as you are, Peter, a PhD card carrying "researcher," but they're folks who are, they need access to insights and data. Do you have any recommendations or any tips you can share, especially if they're thinking about programming a survey question? Karen alluded at the top to this seven sentence closed-ended question, or even a 15 barreled question that wants 15 different things. Is there something that you found, Peter, in your time with Verasight that really helps create engaging data, data that gets to the heart of the matter that you might share?
Peter:
Yeah. I would say two things. One on the question writing, simple is typically better, and the best audience is you and those around you. Meaning if you write a survey question and you ask whoever the next three people you bump into that question, if you get a confused look, you know there's trouble.
Ben:
Sure.
Peter:
And if you-
Ben:
The great [inaudible 00:24:41]
Peter:
If you get answers that seem sensible and you probe a little bit, and the reasoning makes sense, you probably are onto a pretty good question. My colleague, John Schuldt, always says, "There's no perfect survey question, but there's always a better question." And so to remember this is an iterative process. Now, the other side of that is the data quality. And I think what I see happening over and over and over again is people evaluating data quality based on what's easy to see. How quickly did they get the data back? I needed this tomorrow. Oh, I can get it in three days. That seems fast. That must be a good thing. Or what's the cost of the data? I've got a limited budget. This is affordable. That must be a good thing. Or these deliverables are so easy to understand. And all of those things you should expect, right? Affordable, fast, easy to understand deliverables. Those are all good things, but they're not telling you about the quality of the data. It's a little bit like sports analytics have evolved so much.
Peter:
And one of the biggest shifts in sports analytics is not just basing decisions on the easiest to observe statistics. A great example in basketball is offensive rebounding. It's easy to track offensive rebounds. What they've found as they dig more is often high offensive rebound rates correlate with terrible defense, because if you miss the offensive rebound, the other team has a fast break. And so if you're evaluating players based on their offensive rebound number, that might be very high. And when they're in, it may contribute to you losing the game. But it's this tendency to evaluate based on what's in front of us. And again, with data in the consumer space, it's often speed, price, and the deliverables, but none of that tells us where did the data come from? How do we know this is valid data? If it's a survey, if it was an online survey, how do we know these are valid responses and not just somebody clicking through as fast as they could? How do we know it wasn't a bot going through that?
Peter:
How do we know this is a verified person? How do we know that company A did not go to company B who went to company C who went to company one through 500? And so it's often hard to observe direct indicators of quality, but that's what I think people need to ask, they need to understand, and they really need to dive deep. And it's so hard because there's so many, "Oh, we have so many quality checks." "Okay. Tell me a little bit more about those quality checks," or, "We pay the most attention to data quality. We're top in the industry." "Well, tell us a little bit more about that." And so I think the more we can move away from what's easy to observe and really drill down to evidence of high quality data. And again, one of the top ones is population benchmarks. We did some work, a company that Verasight works with, and they said, "Here's some data we've used. We're really happy with it."
Peter:
We analyzed the demographics. Demographics didn't align with the population. They were trying to have a representative picture of the US and the demographics didn't even match up. And we were able to say, "Are you trying to reach 80% women? Because if you're not, you are making bad decisions based on bad data." And we didn't know. And it turned out that no. They wanted it to be representative of US adults. And so there are a lot of ways to evaluate data quality, but got to push on that because bad data leads to bad decisions.
Karen:
Awesome. That's actually a great segue into the next set of questions we want to ask, which is about representation, representative data, and research practices and generalizability. But we will return to that after a quick break.
Ben:
Welcome to Scout Sound Off where we use DSCOUT Express, a quick turn qualitative research tool, to deliver thoughts and opinions straight from our participants about the topic of the day. As we just heard, and we'll continue to hear, Peter talks a lot about data quality and really good survey design. We asked Scouts what they think about a good project. Karen, what did we learn?
Karen:
Yeah, we learned a lot, actually. Just for some extra context, we launched a quick express mission a couple of days ago. Within about three hours, we had 55 Scouts reporting in what their opinions about what they think a good survey design looks like that makes them feel valued and respected and that the mission that they're in is not a waste of time. And I went through these responses and, Ben, you'd think that the top thing might be something like compensation, incentive, that they're not being paid poor money for the amount of time they're putting in, but it wasn't. That was number four on the list..
Ben:
Whoa.
Karen:
Number one on the list was communication and clarity. I found that at least for DSCOUT, our Scouts, they really want to do a good job.
Ben:
Sure.
Karen:
They really enjoy what they do. They want to help. And they love being sure, through clear questions and communications, that they're actually providing the value that they think that you want. Not in terms of answers, But in terms of quality and time spent. While there's no such thing as a perfect survey question, just like Peter just said, our Scouts do still have a couple pieces of advice about how to make your project feel clear, how to communicate well, and make a project worth participating in. Here they are.
Speaker 5:
I feel that a researcher can make it a much better experience simply by keeping an open line of communication.
Speaker 6:
The best ones are really clear and easy to complete. Easy doesn't mean it's not time consuming. It can be time consuming, but it's very clearly laid out what you need to do.
Speaker 7:
Being very specific with the directions, the ideas of the outline of your project.
Speaker 8:
What this information is for and how it's going to be used. I feel like that's really helpful in terms of trying to gear my answers better and also feeling more just engaged in the mission.
Speaker 9:
Just because we give so much of us, but we don't know anything about them, that it could build a better bond to give yourself a full explanation and actually hear and get a level to level detail about the research that you want to hear.
Speaker 10:
Just setting the expectation for what I, as a researcher, if you will, can expect to do when I'm accepting the mission. More clarity would be helpful.
Karen:
All right. Thank you, Scouts.
Ben:
Yes. Thank you to our Scouts. And if you would like to conduct quick turn qualitative research for things like pulse checks, concept testing, or micro interviews, check out dscout.com for more. Our thanks again to our Scouts for sounding off. Let's get back to our conversation with Peter.
Karen:
And we're back with Peter Enns. And for the second half of our podcast today, we want to talk a little bit more in depth about issues of representation. Peter, we were so excited to talk with you about this, because I know in your past, you actually have a whole other book that you have helped to write called, I believe, "Who Gets Represented?" Is that correct?
Peter:
Yes. Yes.
Karen:
We'd love to hear just a little bit on a high level first about what are the kinds of things that you cover in that book and in other work? And at a high level, what does it mean here when we're talking about representation in your research world?
Peter:
Yeah. Terrific. With the book "Who Gets Represented?" and some of my other research, it's really thinking about do public policies follow public opinion? And then if so, whose opinions, whose preferences are being translated into policy outcomes? And it turns out this is a really difficult question because it comes down to what is our definition of representation? And so there's a lot of disagreement in the social sciences. And one of the things we have to do is well, one, think about which groups are we trying to understand? And then how do we measure the preferences of those groups? And that comes back a little bit to what we were talking about before. One of the challenging factors is often sample size. As we get to certain groups and then within those groups, our sample sizes get smaller and smaller, making it more and more difficult to measure what policies these individuals or these segments of the population want.
Peter:
And so that book itself was an edited volume of a bunch of the most prominent political scientists. And that surprisingly led to a lot of different answers about who gets represented because it really, turns out, it depends. And one of the best illustrations, especially now as politics within the United States are so polarized, so partisan, who gets represented depends a lot on the party in power and the partisanship of individuals. And another factor that matters immensely is what level of government are we looking at? Are we looking at federal policy? Are we looking at state policy? Are we looking at local policy? And that can be a frustrating answer, it depends, but often complex answers are the most accurate.
Ben:
Peter, you mentioned earlier the importance of having benchmarks or gut checks that you and your team can use to ensure that the data you're getting is valid and representative, and that therefore, your conclusions are similarly representative and similarly valid. When you're doing consumer research, or I guess, are there checks and balances that you might share for our audience who are researching user bases? Maybe they'd like to think they're the entire US population, but folks who use a ride sharing app or folks who have a meal delivered. Are there strategies that you might share for checking the representativeness of one's data? Are there other things you might share?
Peter:
Yeah. And this turns out to be super, super important, Ben, because most people have an intuition of, if they're trying to understand consumers in general or the adult population, that it should look like the adult population. That's pretty straightforward.
Ben:
Sure.
Peter:
We often forget that when we go to groups. Then it's, oh, my customers or young people or folks who live in an urban area. Well, there's a lot of variety of those individuals. And so it's not just enough to say, "Oh, I have my customers that I'm asking questions of." Are they truly representative of all your customers? Or if I'm looking at young people, young potential consumers, are these just the young individuals who this company happen to bump into or happen to get to respond to a survey, or are they truly representative? I'll give an example of something we just ran into with Verasight. It was related to a company who was wanted to know what their consumers thought and they did what at first seemed like a pretty straightforward, pretty reasonable approach. They offered a coupon to any of their current customers who answered the survey. The problem with that was those who wanted the coupon were the most excited about that product.
Peter:
That's why they wanted the coupon, to go out and buy another product. They've got these immensely positive responses, which they were super happy about. Well, when we did the the parallel study at Verasight with a representative sample of their customers, the ratings were much less positive. And that's a tough lesson to receive when you do it one way and you see that everybody loves what we're doing, but it turns out that, well, it was because of the folks who were on that email list were the folks who naturally liked that product. And then when they got the coupon, it was even a subset of that group compared to what we delivered, which was very representative data of their customers, but a much more mixed picture of the product evaluation. That's a tough lesson to get. People naturally want to gravitate sometimes toward data that validate their views. But if they would've made their decisions based on that, it would've been the wrong decision and down the road, it would've been so much worse off.
Karen:
Peter, now I don't want to make you spill your trade secrets live on air or anything, but I'm curious about you were mentioning that you were able to obtain a very representative sample. And I'm curious if there's anything you can say about what considerations that you had in your mind that maybe your clients or this other group didn't have in mind that ensured that you did have this representative sample.
Peter:
Yeah, yeah. No, I need to choose my words carefully here to not reveal the secret sauce. I can easily say two things. One has to do with, again, the incentive provided to the people providing information. Okay. And so in the case of the coupon, that was really problematic. But another issue we see very often is people in the industry, providing survey data, the incentives aren't quite aligned. And so they're trying to find people who match whatever the category is, young respondents, people who bought this brand, people who haven't bought that brand. And so they're asking questions to see if they qualify for the survey. And that creates really problematic incentives because survey takers know this, and sometimes they might have incentive to say, "Oh yeah, I've used that product." Some surveys, if you say you've used a bunch of products, your survey becomes longer. That creates a negative incentive. But anyways, the incentive structure for how people qualify for a survey, why they're taking the survey, why they were invited, what they get has to be done in a neutral way.
Karen:
Something that's been really on my mind as well this last year is how to transition the survey practice from this extractive disposable model of how do I get this data? How do I get data from, and maybe not even thinking, a person? It's just like, how do I get the sample size I need, the questions I need answered to, I am entering into a relationship with however many participants I have, and this is an equitable relationship in some equitable maybe long term exchange, but more of an equal footing and how to design towards building that trust. And the first thought that I had in my mind was, oh, well, because it's the thing to do that isn't you being a jerk. It's the more ethical thing to do.
Karen:
But what I'm hearing from you too, is that if you're able to establish that level of trust, you may actually decrease that sense of if it's this extractive model, if they're just like, well, if I'm a data mine, then you are a money mine. How do I get the maximum incentive out of this relationship? Versus if you're able to build a more equitable relationship that both parties may be more willing to yield honest and complete information.
Peter:
What I think it does is right now in so much of the survey and data industry, it feels like a race to the bottom. Cheaper data, that's a worse experience for the people actually taking the time to answer questions or provide the data. And so that means lower quality data, which means the companies buying the data are making worse decisions. And we're trying to create a virtuous positive cycle where it's a partnership that's ethical, that's providing more to the people providing their information. And then that leads to better decisions from the end customer, whether that's an academic researcher trying to make sure they reach valid conclusions or a company trying to make product decisions or a non-profit organization trying strategize.
Peter:
Those decisions get better. Their outcomes get better. More and more people come to us because they realize they're losing out if they don't have high quality data. Because it's back to this point, bad data leads to bad decisions. And oftentimes, multimillion dollar decisions are being made based on bad data. And so this positive virtuous cycle is good for business, of course, but it's good for everybody involved, including our community who are our core partners in this.
Ben:
Peter, I'm so glad that you mentioned and we've been talking about the notion of, not the notion of, but segments, demographics, specific communities. This is another series of conversations that's happening in the user experience world, along with what Karen was just describing, this shift from a reciprocal relationship based approach to data wherein, Hey, the folks who are sharing their experiences are also using our products and services. We'd like them to continue doing that. Let's move away from a, give us what you like and don't like, and then we'll, not see you again. One of the other shifts is from demographic based data wherein let's make decisions based on elective categories or categories that we deem to be important, two more behavioral based sorts of recruitment and sampling. Do you see that in the public policy world?
Peter:
Yeah, no. The question of behavior, that's a great area of focus, super important. And in my research space, we have the benefit of seeing election outcomes. We don't necessarily know for sure whether an individual voted. We can often look at voter registration and get an indication there, but we can see, was our forecast accurate? Because we see the election outcome. There is a lot of work in social science with behaviors. There's some interesting work on if people put a campaign yard sign up. And so looking at all sorts of behaviors of political expression, a long history of looking at protest and trying to understand both individual level protest participation and aggregate level. And so there is a long history here.
Peter:
I don't think we'll ever move away from demographics. One reason is back to understanding groups. Demographics are a really important measure of, are we getting the full picture of a group. Now, it's sometimes hard to know what is the demographic distribution of any one group. But if we look at, let's say, young voters, young consumers, and they're all from one region of the country or one race or one gender, we know it's skewed. It's not representative. And so that's an important benchmark to keep in mind. The flip side is sometimes we do, I think both in the consumer world and in the social science research world, rely too much on demographic categories. And although, when we reflect, I think most people are aware of there's massive variation and heterogeneity within various demographic groups.
Peter:
It's easy to forget that when we're looking at the data, especially as our sample sizes get small. And that within any one category, there's massive variation to keep in mind. And we can't assume that everybody within that group is the same. I think behaviors and demographics will always need to be analyzed together and we just have to balance it. Another thing I've seen is people trying to look at just purchase data or just social media data. This is actual behavior. Even that isn't always, or I would say, most of the time isn't representative. When a company gets purchased data, well, if it was ordered from their website, they know that those were purchased. But what about if it went through Amazon? Do they have that exact purchase data? It's going to be very different.
Peter:
What if somebody buys a subscription to get data from a certain box store? They might have it from that, but not other smaller stores. This is the other critical component, even when it feels like we have data on actual behavior, often that's a subset of the true population of interest. And if it's a subset, we don't know which subset that is. And it could, again, lead to incorrect decisions because maybe we got the data from the box store, but not the web vendor, or maybe we have it from our website, but not another. Well, if customers are different across those categories, which we know they are, and we base our overall strategy on just one segment, we're going to have a biased strategy.
Karen:
We would love to wrap up with a final question for you, Peter. Being somebody who has all of this expertise in a world that many of our UX folks are not coming from, this large scale public policy and political science academia, do you have one final word of wisdom that you want to share with our listeners in product design and experience research?
Peter:
I guess I would go back to, can you trust the data? How do you know the data you're using are the right data to make good decisions? This concern of bad data is guaranteed to lead to bad decisions. The other thing I would say to that is to recognize how quickly the data environment is changing in often ways for good. It's becoming harder to track people's behavior on the web. You have to opt in increasingly. There's data protections. But what that means is new data strategies need to evolve. And so just because data that seem to work well a year ago, or even six months ago, may not be working well now.
Peter:
Asking that question, where did the data come from? How do we know it's valid? And then not being complacent, making sure we're continually asking that question. And this to me is so important. Whether we're talking about politics or consumer behavior or guiding a nonprofit, most people want to be representing the views of their core audience, whether it's their voters or their consumers. And if you're trying to deliver the best policy, hopefully that's what our politicians are trying to do, trying to deliver the best product. We've got to have the right data as the input for those decisions. And so that's what I come back to.
Ben:
Perfect way to end. Dr. Peter Enns, thank you so very much for some of your time, your smarts, and your considered responses. We really, really appreciate it.
Peter:
Well, thanks, Karen, and thanks, Ben. This was a fun conversation and so glad to be part of it.
Ben:
Our thanks again to Dr. Enns. You can connect with Peter on Twitter with the handle @Pete_Enns.
Karen:
That's right. And we also talked a lot about Peter's scholarship during this episode, including his books, "Incarceration Nation," "Who Gets Represented?" and the award-winning "Hijacking the Agenda." To read more about these, as well as the rest of Peter's scholarship and teaching and research, you can visit peterenns.org.
Ben:
And if you like this podcast, please subscribe. And if you really like this podcast, leave a review. Karen and I read those and we'd love to hear from you. Check out dscout.com for ways to begin conducting mixed methods experience research at scale like Dr. Enns was speaking about today.
Karen:
And if you enjoy being part of this community and listening to this podcast, don't forget, we also have a blog and a newsletter. Visit peoplenerds.com to subscribe, and we will see you next week, Nerds.
Ben:
Nerds.