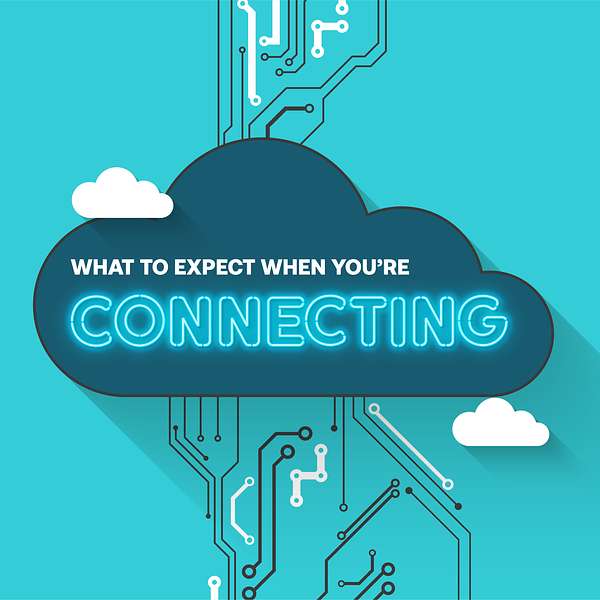
What to Expect When You're Connecting
What to Expect When You're Connecting includes interviews with a wide range of industry subject matter experts who share their journey, advice, and the mistakes they've made along the way in IoT. If you're adding connectivity to your products for the first time or seeking to optimize and scale your existing connectivity operations – welcome to the conversation.
What to Expect When You're Connecting
The New Frontier of Trustworthy AI with Rajeev Dutt of AI Dynamics
In this episode, our host Ryan Carlson talks with Rajeev Dutt, a theoretical physicist and the founder and CEO of AI Dynamics, to discuss the world of artificial intelligence (AI), its defining characteristics, and the challenges of AI governance. Rajeev shares examples of how AI is solving problems in various industries, such as drug target identification in biotech and visual quality inspection in smart factories. They also explore the importance of data quality, potential biases in AI models, and the need for transparency in AI decision-making. Tune in to gain insights into AI and learn about the future of AI building AI.
- An Introduction and Exploration of the World of AI
- Examples of AI Applications in Different Industries
- Understanding the Characteristics and Applications of AI
- The Importance of Data Quality and AI Biases
- Addressing the Challenges of AI Governance
- The Future of AI and AI Building AI
- Concluding Thoughts on Trust and Transparency in AI
Welcome to what to expect when you're connecting a podcast for IOT professionals and the IOT curious. Who find themselves responsible for growing executing or educating others about the challenges with connecting products and services to the internet. You'll learn from industry experts who understand those challenges deeply. And what they've done to overcome them now for your host, Ryan Carlson.
Ryan:We're here with Rajeev Dutt, who is both a theoretical physicist and founder and CEO of AI dynamics, and so many years of being a technologist. Rajeev, thank you so much for coming to share your experiences on ai.
rajeev-ai_dynamics_pt1:It's my pleasure. Thank you for having me.
Ryan:So the thing that I want to do before we talk about what AI is, and its defining characteristics, I'd like to just set the stage with the kinds of things that you are doing with ai. Maybe a couple examples of the problems that you're solving with this technology, and then we can pop the hood and see, the underlying characteristics of how it's suited to solve those types of problems.
rajeev-ai_dynamics_pt1:yeah, so, I guess I could probably start with our platform, what my company does. So we have, the general purpose, artificial intelligence platform that, can essentially solve a range of different types of machine learning problems in different industries. And so traditionally we have been involved with two major sectors. one is in biotech and the other one is Industry 4.0 or smart factories. in the biotech side, we have done everything from, drug target identification, so using machine learning, to identify the set of genes that potentially could cause cancer, or identify how these genes might be related to each other. and also understand the causal mechanism, that might be involved in a gene expressing, that might eventually result in cancer. and in the industry 4.0, we've kind of targeted traditional types of things like, visual quality inspection, being able to predict when, a machine is about to go bad or is there's about to be a failure. and more recently, we are also starting to do work in designing intelligent polymers using machine learning, by understanding the, behavior of how, complex materials behave. And, and then I identifying, certain core characteristics and then optimizing for them. So the way we see it is that machine learning, we're kind of just at the. to use a cliche, we're at the tip of the iceberg there. and I just see that the range of applications will just simply grow and, we are incorporating everything from traditional machine learning, to what's known as unsupervised machine learning, where you have an AI that kind of learns on its own, kind of picks up information, is able to classify and identify patterns on its own without human intervention to kind of, what's all the rage these days? Generative models, where you use machine learning to actually create new things that are currently not, available.
Ryan:And with the generative stuff, I mean, we're talking about the chat GPTs of the world and we can't get past some of the buzz. I mean, it's neat that there's so much awareness of what's possible and it feels almost eerie and people like, oh, that's so weird that it was able to come up with such a, an answer. But it's not new. There's nothing really new. It's just combining a bunch of data in. In a way that follows whatever the prompt might have been.
rajeev-ai_dynamics_pt1:Well, I think this is essentially understanding, getting to the heart of what AI is. if you really look at what machine learning and what AI is all about, it's about understanding patterns. what AI and machine learning is all about is you see tons and tons of data. And you understand, and the machine, looks at that and is able to understand some underlying patterns within that data. And based on those patterns, you can either draw conclusions from that or use the machine to predict what other patterns might emerge in the future. with, chatGPT and all these other, kind of generative models that we've been hearing about. effectively what it's doing is it's seeing, it's ingesting billions and billions of, pieces of text from around the world. Potentially even also in different languages. So it develops an intrinsic understanding of how, the patterns of human communication and understands how it is that we communicate, how it is that we relate concepts to each other and so on, without really understanding really what is being said or what is actually happening. So all it is doing is in, in a way, Playing a tape back to us, but in a way that's more, that's smarter. So we've already had like, like all these conversations. So for example, it's able to relate. a good example would be, Depending upon how you use the word wet. So you can, for example, say the dog is wet, or you can say it's wet outside. so those two of course have very different meanings. The dog is wet, meaning, that, I have to dry'em up so the dog is wet. Would then be associated with concepts like towels or blow dryers and things like that, whereas it's wet outside would be considered, would be connected to concepts like umbrellas or staying inside with a hot cup of coffee or some chocolate or something. so, so the idea is that what it's able to do is it's able to group concepts and relate concepts to each other, and that gives, it's the illusion of able being able to understand the context, when in fact what it's being. What it's really doing is it's built up a model where certain concepts are related to other concepts and it's able to ba to be able to create the illusion of context within that information.
Ryan:Let's talk about the qualities that go into artificial intelligence. You started with pattern discovery, the idea of finding a pattern, and so identifying, discovering, and then that's where you get the generative models. It's where we as the. the trainer of data are adding prompts to say, this is how we want you to interpret things, but let's talk about data quality or data quantity. That's something that you've talked about in the past.
rajeev-ai_dynamics_pt1:Yeah, so the, one of the challenges that we have is in a way, our AI models are only as good as the data that is used to train them. if the data is poor, then you expect that the AI model itself will reflect that underlying data. The, so, so Graham, I mean, this is not a, this is kind of, there were a lot of other problems with it, but you've probably heard of something called Tay ai, which is something that Microsoft created some time ago. It was supposed to be a bot, that was supposed to learn From interactions with people. Well, at the beginning it sounded like this really like, Hey, I'm stoked to be in this world. It's wonderful. Humans are great, and so on. By the end of it, it was a raving neo-Nazi, because it had been kind of taught by the people who were using it to kind of. like work that way. So it's basically a machine is only as good as the data that it has, and there are some real risks involved that people oftentimes, ignore. so a great example would be some of these, resume ranking or rating systems that, Some companies are beginning to use to filter out resumes. well, it turns out that, the type of resumes that go through tend to be a reflection of the people that work in that company already. So, which means that if you're trying to diversify your, potential pool of employees, you're not gonna get that because your machine is already patterned on. a type of person who graduates from a type of university may even come from a spec specific ethnic background, specific names even, which is that kind of frightening actually, that it keys off certain names, backgrounds, all that kind of information and goes into building this model of what it thinks an ideal employee is
Ryan:So you're talking about bias.
rajeev-ai_dynamics_pt1:Huge amounts of bias. the, the, I think it was the Metropolitan police in the, uk implemented a system where to try and understand how people were behaving and whether there was aggression there, and was more often than not identifying, People of a certain ethnicity as being the ones who are instigating crime. there's another AI that was looking at human emotional, like basically it was an emotional recognition system and it was more often than not targeting African Americans as being less happy or more upset than non-African Americans because again, it was keyed off of data that was trained and did not have enough samples of, African Americans. And so the trick with any machine learning model is, To obliterate or get rid of those biases. So even if, African Americans might make whatever, 10% or 20% of the population, they should still make 50%. If you're just looking at white or and black to different groups, then they should still be, 50% of the population of samples should be white and 50% of population should be black or whatever. However other ethnic groups, you're kind of taking into consideration there. because machines are only statistical devices. And, what you need to do is make sure it is able to be completely balanced and from a statistical point of view, that it's not more inclined to pick one, data than another. with all things being equal.
Ryan:I'm hearing then that. the data that goes in is just as important. So, the selection process for the data sets that you would need access to are going to color the results and the patterns that you're going to find after the fact
rajeev-ai_dynamics_pt1:Exactly. And I think that this is, this is really leading to, it's one area that unfortunately, within machine learning is oftentimes underappreciated That. it's wonderful to come up with brilliant algorithms to understand the, and this is kind of where the excitement is as a machine learning researcher, it's all about uncovering patterns in, in the data, but oftentimes without the consideration of where that data comes from, what biases it might have, whether there is, anything that. Whether there's anomalous concepts within the data. for medical data, for example, are we over-index? If you, for example, train a model in the United States, are you over-indexing on heart issues and less than on other issues and so on? So all these things start to come into play there, and. Cleaning up that data is a massive effort. and the other thing is also something called feature engineering that is, which set of features are more relevant to what I'm actually looking at. Because if I look at, so for example, if I'm comparing a group of people and looking at their performance at work, there are a lot of ways to classify people. You can have like age, gender, you can have, years of experience. You can have, like a raw skill sets, et cetera, et cetera. And if I build a model with all of these parameters, I might not get what I want because, for example, it may key off gender, it may key off, age, or it might key off other things that were probably not relevant to somebody's actual performance, but, There was that there were biases in the original data that suggest that, for example, that age might be a factor or, gender might be a certain factor and so on. Even though we know that's not really the case, it's just a reflection of what the data, what was, what it was actually trained on. So being able to narrow down the set of features to what's relevant is a huge challenge as well.
Ryan:I'm hearing that this is, the classic black box scenario then, right? if you've got this magic oracle that you assume the answers are to be true, that can be very dangerous without the ability to actually knowing what's going on underneath, you end up with bias that you weren't aware of. So talk to me about how, how as a technologist you are addressing the black box. Of AI and shining some light on what's actually happening.
rajeev-ai_dynamics_pt1:because we have been heavily involved in the biotech and medical fields, in particular in these areas. People care about, not only, that you're getting the result that is predicting that this chemical might actually treat this disease, but why does it think that way? explainability is very important in certain domains especially in the medical domains, financial domains and so on, it's not just good enough to know. That, a decision was made. It's also important to understand exactly why that decision was made. So if, for example, if doctor diagnosis that somebody's got pneumonia, it's not good enough to say, Hey, the patient's got pneumonia. The doctorate wants to know, well, why did, does the AI think this person's got pneumonia? and especially With the new privacy laws, and, there's the the European Union, passed a set of laws a few years ago, and one of these was that if you have, a. Financial decision about someone, then you have to be able to explain why that decision was made. So if somebody was refused a house loan, what was the reason for that decision? And so this is part of, the European rules about, how we can make decisions about that. so we have been, and one of the interesting things is That sounds important to kind of open the black box, but sometimes that gives you real insight. So, for example, when we were able to identify, which genes were responsible for what kind of, set of characteristics of cancer, we passed these genes and got a set of characteristics, but we, what we're able to do? Is to reverse in like go backwards reverse engineer that go from the characteristics and identify which genes most contributed to those set of characteristics. So we were able to open the black box and actually gain insights into the underlying mechanism. And so this is, it's a very critical piece of, the overall, how things, like to be able to understand. Why a machine makes this decision. And there have been some interesting stories in the past. one of them is a story about tanks I think it was in the early 1990s, where they, there was a US military trained a bunch of tanks, trained in AI to detect the difference between, a Soviet or Russian tank and the US tank. And so they showed a bunch of photos of us tanks, a bunch of photos of Russian tanks, and the AI consistently. During the test and training part, they were, it was able to determine what a Russian tank looked like, what a US tank looks like. So they went out into the Mojave Desert and put out cutouts of Russian tanks and US tanks and the ai, like basically make, claimed that every tank was American. And they were scratching their heads wondering what was wrong. And it turns out that in the pictures of the American tanks, it was always sunny in the pictures of the Russian tanks. It was always cloudy. So the AI had just learned the difference between cloudy and sunny, not, anything else. And so, and there's some other examples like, there was a case where someone who was classifying horses and, there were Arabian horses and a bunch of others, and, again, in the training data, it looked like the AI was consistently, able to tell the user whether something was an Arabian horse or was some other kind of horse. but it failed in reality. And the reason why was that the AI was learning about the copyright symbol. so certain images have been copyrighted and others not. So understanding why an AI makes its, makes a decision is very critical. And in some cases it can provide a lot of insight, as in the case that we have pointed out where we are able to identify, what are the most likely genes responsible for certain types of, cancer characteristics.
Ryan:That's fantastic. So. if you're building into your AI software, the ability to always understand what's going into the answer. let's talk about things like things like a governance or, these things that you need to build into any sort of critical system that would be leveraging ai.
rajeev-ai_dynamics_pt1:As we move on, in like, AI's gonna become more and more integral into all aspects of our lives, I mean, we already use it in quite a number of places. Everything to do cr spelling and grammar checks to, kind of auto complete and all kinds of other things. but. These are kind of quotation marks, non-critical. Because if these fail, I mean, if autocorrect fails in your phone, it's not the end of the world. if, even if your resume system fails, it's, it doesn't, like you can have humans review the resume, but what happens if you have a critical military system that fails? So, for example, increasingly we're designing, kind of weapon systems that are able to make decisions on the on its own. So, for example, being able to evade, anti-missile systems and things like that. So I, in these cases, what you want to do is make sure that the AI that it's trained on is solid. So, of course, data matters, of course, the algorithms matter. But most AI algorithms today, when you train a model today, you're actually not, the algorithms are great, but what's becoming more critical is what's known as pre-trained models. So the way to think about a pre-trained model is suppose I'm building a model that can tell the difference between a cat and a dog. So I do it and I do it really well. but now I wanna kind of classify squirrel. I go to somebody and says, yeah, cabin dogs are really great, but I really, I care about squirrels, whether something's a squirrel or a rabbit. So on. At the surface, it seems that cats and dogs and squirrels are unrelated, but actually of course not, because they have a lot of similar features like ears, noses, legs, and so on. So you train an AI model on a cat and a dog, and it picks up certain core features of what a cat and dog is. And it turns out that you can then take those features and Mo and apply them to other areas like for example, telling the difference between squirrel and rabbit. So this is akin to. The way to think about it is that you're giving the AI an intuition of. Shapes of animals. So by training it on cats and dogs, you're not only training it to tell the difference, but if you go into the model itself, you're, there's a, there are parts of the model that there are layers of the model that understand characteristics of animals in general. And so you're kind of giving it a sort of a language to understand animals that can be applied to all animals from that point onwards. And those are what's known as pre-trained models. So pre-trained models are used more and more. a lot of, older models were based on a pre-trained model called resnet 50, and nowadays you have, they're Vgt 16. There are a whole bunch of models out there that are image-based. Efficient. Net is another one that, came out a few years ago. So these are all a pre-trained models which are able to very effectively, be integrated into your particular problem and therefore reduce the amount of data that you need to train. On and accelerate the pro the process of building machine learning models. The challenge is where did these pre-trained models come from? If I give you a pre-trained model and say, Hey, this does image classification wonderfully, and you integrate it in your system, sure it on the surface it may do everything that you want, but in a critical moment, that model fails because the pre-trained model that you were basing it on has some. Intentional or unintentional, weaknesses that prevented from understanding certain key things and that, those are the kind of things where you need to not only understand. So if you build an AI model, you need to understand where did that data come from? Who created that data? If there are pre-trained models involved in the process, where did those, where did that? Can't come from and what data was that trained on? and so on. So, which means that building this entire layer of understanding what the model does and also its basic characteristics, what was its, false positive rate, what was its false negative rates, and understanding those general characteristics to allow you to then pick a model and utilize it for whatever purpose you intend. so if I build a diagnostic system, I might have a certain tolerance for false negatives, but I absolutely wanna make sure that my false positives are great. So I want to be able to pick and choose these ki, these type of models. So it's a critical, all part of what's known as AI governance, which is building a framework to pick and choose machine learning models. so there are a lot of things I need to know about it. It's like, going back to the cat and dog and squirrel and Rabbit case, the model that was used to tell the difference between a cat and the dog. That's great. but how do I know that it's really as good as I the person claims to be? have I, has it been properly tested and does it truly apply to my particular problem? So are there characteristics that this model may not necessarily be able to pick up on or is kind of biasing my model towards? so, so for example, if I have a squirrel and snake, and I apply this model, it may not be able to work as effectively or as efficiently. so the same thing applies to, kind of mission critical applications because if I'm building an entire framework, on the idea that the AI models themselves are trustworthy, well, you know what? There are a lot of things I need to know. Where did the data come from? If there are pre-trained models involved, where did the data to train those come from? Are there any biases in the underlying model? Are there biases in the data? Are there any anomalies that are known? How does, what is the level of testing that's gone into it? And also just broader characteristics of the AI models that are being trained. What is the false positive and false negative rate? Does it match the kind of expectations that I have? And a lot of those questions are going unanswered. When, we talk about machine learning, which is kind of resulting in a lot of, misuse, or I wouldn't say necessarily misuse is not necessarily the right word, because that kind of implies malicious intent. It's more, the accidental, non applicability of certain ai models. So, people start with the best of intentions, but because of the fact that there was no governance framework in place, This means that the end result of the AI model is that the model either does not meet the specifications that I set out to establish from the beginning, or if they do it does it in a biased way or it is in a way that. For example, might have gaps or so on and so on. So being able to that, and that can be embarrassing in the best case scenario. or it can be outright dangerous in cases where you're asking for a machine to, for example, determine whether, a certain. Person or object is a target. and it, for example, ends up killing the wrong person or destroying the wrong target. or if it is responsible in a more benign case, it's responsible for diagnosing a problem in a patient. And it is, it either fails to diagnose or diagnosises it with. Excessive enthusiasm and kind of, it's false. It's false. Negative rates or false positive rates are, far too high And so these can either lead to misery in people or sometimes, which is even more frightening, some missed results. So you don't wanna miss a cancer diagnosis.
Ryan:I was planning on saving this for the very end, but we're talking about this very concerning part about AI in which. All of pop culture, most of science fiction. It's not a utopian, but a dystopian future in which AI leads to not making the best of possible choices, but far too often it's making choices that are bad for us humans. How is it that we can look at AI not as a future Skynet, where, what's the, which results in the eradication of all humanity? I mean, what. What does the future hold for someone who is both a technologist but also has to consider the ethical implications of AI development?
rajeev-ai_dynamics_pt1:Well, I think the core thing, which I would like to see happen, is to build up a governance model for machine learning to, to begin with. so quite like, quite aside from the, science fiction themes of building self-aware machines and intelligent machines, which I'd love to discuss at one point. Definitely would, it would be great to talk about it. but. With the more current types of issues where we have, we're building out, machines to solve kind of day-to-day problems like image diagnosis or designing new materials or quality issues in manufacturing or things like that. So be having a machine that's able to pick up on those kind of. Issues or flaws in, in the system? being able to diagnose diseases, being able to design new materials, being able to, in the military application, being able to pinpoint the right target, being able to operate planes because if you have an AI that's increasingly able to understand how to fly and adjust and so on, it's like you being able to determine the plains health and operability and whatnot. There's like a billion in one applications. As we start to see more and more of our lives kind of, having AI either integrated into it or we take a dependency on it. We should also have a framework that helps us understand the underlying AI models better. that what is it that we are actually getting ourselves into? What are the characteristics of the system that I'm taking a dependency on? And it's not just simply the AI researcher who needs to, who is trying to understand the underlying data. or the under underlying pre-trained models or algorithms, et cetera. But from a consumer perspective, do you really know what you're using? Do you really know whether this wonderful new TikTok filter is really all about? and so these are the kind of things where as consumers, do we have the right set of tools available to make informed decisions about the machine learning systems that are surrounding us and we're taking dependencies on. So that's kind of like the more. Pragmatic concerns? I would say the ones that would apply to us in the, I'd say in the immediate future,
Ryan:The idea of sentience or self-awareness is so far into the future we're still focusing on the utility use cases of ai.
rajeev-ai_dynamics_pt1:correct
Ryan:so if we're thinking things like industry 4.0, smart factories, connected safety, where we have a starting set of data points. Talk to me about the process of looking for and integrating additional discreet data points in order to hone models over time.
rajeev-ai_dynamics_pt1:So one of the, important traits is, the fact, so is the recognition that. Firstly, a, you may not have capped what's not. Is there something, this concept called ground truth, which is ground truth for is a basic name for reality. So, that the first thing is that for ground truth, you may not have captured all of the ground truth that you're missing out on certain key features. This is a problem with self-driving cars because obviously most self-driving cars still cannot be used completely freely because it doesn't anticipate or doesn't, it does not. Every end use case has been considered. but the same thing is applicable for factory systems and so on because have you really considered all the possible defects that might happen or all the things that can potentially stop or slow down a, an assembly line? so that those are basically, limitations of your ground truth. The second aspect is that your ground truth might change over time. so for example, suppose I design a machine learning model that is able to understand headlines and understand articles that are written, while the world changes. And so if I build a model that, for example, in the early two thousands and, it knew about like George W. Bush and Carl Rove and nine 11 and all of that. And then suddenly, Teleported that to the year 2022, where your news reports are about, like Joe Biden and, Ukraine and everything else. It's like the same model wouldn't apply. It wouldn't have any understanding of modern headlines. So that's a very drastic difference. But even on a month by month basis, what's in the news today, it's not gonna be in the news like three months from now. some of it will be, but. There's gonna be a lot of variants. so being able to incorporate all of those is incredibly hard. So even, for example, chatGPT was only trained on data until 2021. so if you ask chatGPT, so what is the likelihood of a Full scale conflict between Ukraine and Russia, it will come back with there's no full scale war and it's not likely, or something like that. I can't remember. because it has no knowledge or no understanding of that. So you need to constantly keep the AI models up to date, or risk that the, it will become obsolete and be given bad information. So that's, so you've cov covered two cases. One is where your ground truth is incomplete. Second one is your ground. Truth is, is basically constantly changing. And then there's a third, which is more malicious, that your ground truth may not be honestly represented. That, which may be intentional or unintentional, but if your ground truth is not being honestly represented, that could either be the result of bias, like, either intentional or unintentional, which will of course mean that your system is not gonna necessarily represent that. But fortunately, models can be retrained. In which case then if you are aware or if you can understand where those biases came from, then potentially you can repair your system. The good news is that in all of those cases, you can repair the AI model in quotation mark. Repairing quotation marks. and that is simply by obtaining, a better understanding of your ground truth and characteristics are of ground truth. How often does it change? How much does it change by? So as you can see, it's like, it gets really complicated. Everything from understanding raw data, understanding, like building out the entire governance process, understanding characteristics of your data. Do you really understand the system that you're looking at? if you ask, researcher to say, Hey, like, go in and look at the news articles and build a model from that. Do they really. Get that, the news changes on a day-to-day basis? Or is, do they see it as something static? like if you go to a factory, they're churn out widgets. It's a pretty static environment. Unless they change their product, the defects that occur in a widget, you can keep adding more and more data to it, and it gets better and better at detecting quality issues in the widget. But, It in the case of, AI models representing, it's something that changes over time. It becomes more tricky because, you have to constantly update it with the latest information. And even then, it may not necessarily be as accurate as you want because, you may not have enough of that latest information, if that makes sense.
Ryan:It does something that you've said, previously is you talk about AI building ai. Could you give me a few minutes of kind of some context around, around that statement?
rajeev-ai_dynamics_pt1:So Neo Pulse, which is our core platform, is capable of, AI building ai. There's a whole field called Auto ML, which is, basically using. like some level of intelligence to figure out how to determine the right algorithm for a problem. How to determine what's, what are called hyper parameters for a problem of hyper parameters are essentially the parameters that the algorithm uses for training purposes. like how fast it learns, how much flexibility does it have in terms of the knowledge it absorbs. How quickly, like what is the, how much should it forget as well? All, all of these things are what are called hyper parameters. so you have systems that optimize in hyper parameters, optimize for algorithms and so on. And this is what, we do as well. and we're kind of evolving this to understand what kind of, kind of, pre-trained models should be used for your particular problem and so on. As a result of doing this, this AI to build ai, one of this is why we care so much about, about governance, is that what we realize is that when you're, building an AI to train ai, it's not just simply. hey, let's pick the best algorithm, the best, hyper set of hyper parameters, but it involves a whole slew of things, of where did the model come from? What was the original training like, what was the data that was used to train it? And so that kind of all comes into play when you're thinking about AI that builds AI. the ideal system should be, a system that is able to allocate if you want, To, for example, for it to learn. It understands the context of what you're trying to learn. so it, depending upon what you wanna do, it's able to draw the data. If the data is available, it's able to draw the data from individual, sources. It's able to identify at a high level, whether there are any anomalies within that data. It might do some what are known as, semi supervised learning, which means it's able to kind of pre classify things into buckets and so on, on its own. and that's completely unsupervised. It's able to do a whole bunch of things like that. And, when you have, a system like that, then, what you can then do is you can, our vision is that it can absorb all of this data and it can on the fly, train up a model. So we're not there yet. We still have a lot of steps to go, but, I think we're making important strides and in the process, uncovering of course, A lot of challenges in machine learning in general. And our vision ultimately is that you can, on the fly, create machine learning models without necessarily any or very minimal human involvement, which is a lot easier said than done because it goes back to understanding the data and whether they're inherent biases in that data and, and so on, which is a much more complicated question and would involve, more sophisticated ai. One of the interesting things, by the way, that we have also learned in building our AI to build AI is to build up an understanding of the underlying data as well. so what that means is, not just simply taking in the data, but actually applying machine learning to the data to assist with feature engineering, to do this sort of automatic, clustering of that data, potentially also exposing, biases in the data so that you actually as a, as a. As a machine learning engineer, you can immediately see that, oh, my data's really like key. There's a lot of these kind of factors in my data that I really don't want the model picking up on because I don't really see how this can be applicable to the general problem. so giving people that deeper insight into data before training, could. is a very important characteristic and maybe at some point, maybe 10, 15 years from now, we're able to figure out how to automate that fully. But, but that's, baby steps. So the first thing is I think we're getting there
Ryan:I'm seeing a future that's very similar to the early days of the web in which it required a. Going in hand coding things in H T M L in order to build a website and there was a very select few people who could actually learn how to do those things and have all of the visual representations, backend and front end. Like we didn't really have a delineation between those two. Code turned into that visual representation. And now we've got the wix and Squarespaces' of the world where you do not need to know anything about code at all, and it's just picking which patterns you like. It is. What goals do you have? I'm gonna drag and drop a shopping cart application, which could have a whole nother series of different workflows. the more I've learned about what your vision is and the work that you've been doing is, I see it's that similar path of making AI accessible to organizations that don't have that deep line by line understanding. but empowering them and their teams to leverage AI now, and learn moving forward.
rajeev-ai_dynamics_pt1:that is exactly kind of the direction we wanna go. like we don't wanna take away from specialist knowledge. You'll have like physicians who specialize in neurology or in specific areas of radiology, like pediatric radiology and so on. They will, they'll be looking for certain types of things. And so what you want is that the AI building system can cater to their needs. The pediatrician who wants to build up an anti model that picks up a certain type of tumor in, in, Like the stomach of a child or whatever. I dunno. I'm not a pediatrician, so, and I don't play one on tv. so the, but basically the idea that can you have an AI that, can be trained by a pediatrician because the pediatrician is able to, using this interface is able to draw on data that has already been prepared by maybe some other group or some other researchers who have done a really good job of actually putting together, Training d training models that reflect this particular type of, malignant tumor in a child's stomach, for example, Which now starts to become really interesting because that for me gets really exciting because it's not just building out this tool that does build ai, but it, implies a vast network behind it where trust is important. So if you. Kind of have this layer where the pediatrician, for example, goes into the machine and says, okay, I really need a model that can under understand this particular issue in child's stomach. the system will have to know, where should I get that data? How can I train up that model really quickly? So it's, are there pre-trained models that can work with that data so it's able to kind of marry all these things together in the background. But in order to do that, we have to build a governance framework. We have to be able to trust where that data's coming from. We have to trust the quality of the data, have to understand its intrinsic biases, have to understand the, like that entire framework of like whether pre-trained models are correct or not. that's gonna have to, that's gonna have to be important. So in a sense, we're kind of talking about a worldwide web for ai. so, which is kind of hiding behind this. So the browser would literally be the thing that. Sits in front of you, that allows you to then design the machine learning model as you want it by you giving requirements and specifying exactly what it is that you're looking for. And in the background, the AI will start to collect from all these millions of sources being able to, put together, AI models and there are certain websites, for example, like hugging face, which is really quite nice, where they have a whole bunch of models and so on. And I think that we'll probably see much more of that going on in the world in the future. So, we'll see that universities may choose to publish some of the, training models, but again, not just publish the training models, but also explain how the model was trained and give access to the data that was used to train it. and even that, it's like sometimes there's a. There's a wall where you can't necessarily have access to the data, but you still wanna trust the AI model in which it was based, in which case there should still be some sort of linkage saying that, okay, you can't see the original data because it was trained on proprietary information, or it was trained on secret information or whatever. But I, but you can, the, it has been quotation mark signed off by people who are trustworthy, so people who are sort of intermediaries. Which, what does that come, what does that, bring to mind? these, trusted providers that, that create certificates that are so important for sending encrypted information over the wire. So you would need these sort of trusted intermediaries who can see the under, can see both sides of the fence and say, okay, well you know what, I trust this data and I'm gonna put my seal of approval on it saying, This data is certified as being trustworthy and it can then be, utilized by people. And of course then you start to build organizations whose mission is to produce this kind of quality stamp of approval. And, their reputations will be based on how trustworthy that data is. So of course, they wanna do a good job with and with, preparing that. But the way I see it is that there's gonna be an analog between the current worldwide web where you draw in information and. Pull in from different sources and of course they're gonna be bad websites and good websites and so on, but the diff, but, and, but having the ability to differentiate that and having the ability to identify. Like where that data comes from. And so, so if you type in HTPs something, so you at least hope that the website matches the certificate, and those kind of things. So, but you'd now it gets more complicated of course, with machine learning, because there are a lot, there's a lot more than just simply saying that the, this model can be trusted or something. But it's also, that entire network of, where it came from and so on. I see, for example, blockchain potentially having a huge, Can blockchain influencing this quite a bit? Because you're thinking really of building a chain of trust around, the model that you're built, that you're putting together.
Ryan:That's the whole point of the ledger, is to create visibility into the source of data and trying to eliminate, bad actors or unintentional malfeasance. So, well that's great. I think we could, there, there's so much ground to cover on ai, but just talking or talking about, basically what it is. I think the governance conversation is a really important one given the black box nature of so much ai. And the big takeaway for me here is Know where the training happened, right? know about those models. I think it's no different than taking your dog and taking it to, some sort of good dog training class, new puppy class. Like, do you trust the methodology that they're using? Do you trust the people that are behind it? Are they teaching them the right behaviors? Because when they come back home, the behaviors that you see, You weren't there, you could be dropping'em off at, to be, to learning these things. But when you're still getting the results and the ability to understand either you had the ability to be there and you could look under the hood and know why you're getting the results you are, or you need to in lieu of. The ability to go and poke and touch and inspect. There needs to be a level of trust. So I'm here that there's a trade off here, right? Speed to results, wanna reuse someone's stuff.
rajeev-ai_dynamics_pt1:Yep.
Ryan:If you can't look under the hood, then you need to know that, there're in. So now I'm hearing that there's a whole new. Ecosystem. just like we've got SSL certificates for a website and there's, the verified stamp for social media accounts, there's gonna be, there's a whole world of AI models and, people saying you can trust it based off of the
rajeev-ai_dynamics_pt1:Exactly. sadly, I think that what's going to happen, I think this is a necessity for the future. but just to inject a note of pessimism there is that normally humans are not really motivated by what might happen, but what actually did happen. So, which means that the first time we start to see a push towards this sort of governance is when we see our first catastrophe based on a machine learning model that was, really faulty data or, that was, that were kind of badly trained or something like that. So, uh, we, are very driven by experience, not by our ability to project into the future.
Ryan:And that is the weakness of us mere meat sacks, is that we need to feel the burn of the fire and go, ow, hot before we're willing to make course corrections in our life. So let's just hope that people don't have to feel the burn of AI and they engage with, experts like yourself, Rajiv, and AI dynamics, and have the opportunity to get the right baseline, have confidence in the models that they're using, and then, build towards the future of better efficiency, productivity, and even new polymer materials, if that's the way that you're going. So thank you for your time and sharing your
rajeev-ai_dynamics_pt1:expertise it's my pleasure and thank you for having me.
This has been another episode of what to expect when you're connecting. Until next time.