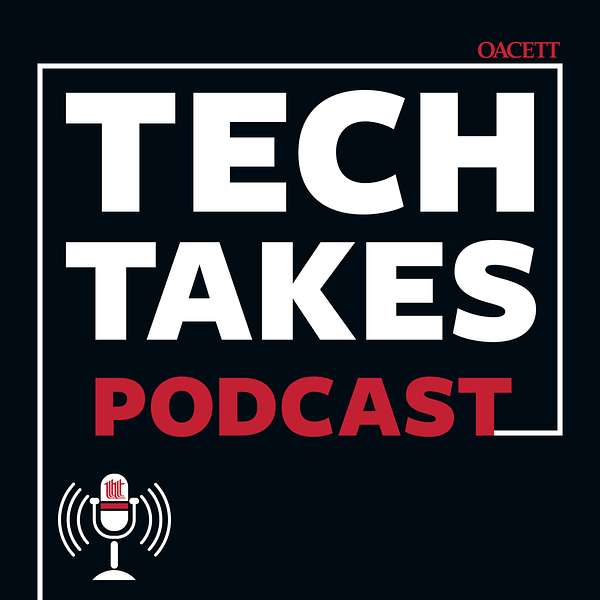
TECH TAKES
TECH TAKES
The Role of AI in Modern Engineering: Opportunities and Challenges
In this episode of Tech Takes, host Louis Savard delves into the transformative potential of AI in revolutionizing work processes and industries.
With our guests, Doug Nix, Co-owner of Compliance Insight Consulting Inc., and Mayy Habayeb, Artificial Intelligence Program Coordinator at Centennial College, they explore the impact of AI on engineering tasks such as design optimization and testing and how automation enhances productivity and efficiency.
They also discuss the importance of acquiring new skills to adapt to the evolving workforce shaped by AI technologies and touch on concerns such as inequality, privacy, safety, and bias arising from widespread AI adoption.
Have a topic you’d like to discuss or comments about the episode? Reach us at techtakes@oacett.org.
Tech Takes - Episode 9
David Terlizzi:
Tech Takes' Podcast is brought to you by Niagara College's Walker Advanced Manufacturing Innovation Center. From day-to-day support in our quality department to long-range new product plans, WAMIC is your competitive advantage. Learn more at ncinnovation.ca / WAMIC. That's ncinnovation.ca/ W-A-M-I-C. This is Tech Takes, a podcast that explores the many facets of the engineering and applied science profession. It is brought to you by OSET, the Ontario Association of Certified Engineering Technicians and Technologists.
Louis Savard:
Hi, I'm Louis Savard, and welcome to Tech Takes. The relationship between artificial intelligence and the workforce is multifaceted and evolving. Automation, augmentation, reskilling and upskilling, new job creation, and ethical and social implications are just a few examples of how AI shapes our world and how we work. In the engineering technology world, AI technologies are beginning to or have already automated various engineering tasks, including design optimization, simulation, and testing. This significantly enhances productivity and efficiency in engineering projects, allowing technologists to focus on more complex and creative aspects of their work. In addition, AI's integration into how we work and its reshaping of industries have created a growing need for workers to acquire new skills to remain relevant in the workforce. The explosive, widespread AI adoption in our industries has also raised concern about inequality, privacy, safety, and bias. Overall, AI's impact in the workforce depends on various factors, including the industry, the type of AI technologies deployed, and societal responses to these changes. While AI has the potential to revolutionize the way we work, It also presents challenges that many believe need to be addressed proactively. This episode of Tech Tips podcast dives into the role, benefits, implications, risks, and regulation of AI in the engineering world and beyond. You'll also learn about Centennial College's software engineering technology Artificial Intelligence 3-Year Advanced Diploma Program, which, through collaboration with industry, provides its students with skills in state-of-the-art design and AI application development technologies. Joining me today is Doug Nix, CET, co-owner of Compliance Insight Consulting, Inc., and writer of the Machinery Safety 101 blog. Since 1985, his experience has included control system design, testing, certification, and electrical equipment and automation systems consulting. He specializes in risk assessment and machinery safety. Doug has been a member of the Ontario Association of Certified Engineering Technicians and Technologists, our beloved OSET, for more than 30 years, and a senior member of the Institute for Electrical and Electronic Engineers, IEEE. Also with me today is Mayy Habayeb. Mayy is a Software Engineering Technology Artificial Intelligence Professor at Centennial College and currently holds a position of Artificial Intelligence Program Coordinator at the college. She has practical hands-on experience in developing machine learning models, natural language processing models, and big data recommended systems. Mayy has developed and delivered courses in the areas of natural language processing, recommended systems, machine learning, big data, and predictive analytics at universities and colleges across Canada. Her research work has been published in the IEEE Transactions on Software Engineering Journal, the TSE. She has also presented and published conference papers at the IEEE Big Data the Data Mining Software Repositories, and the International Conference of Software Engineering, ICSE, conferences. She has led many projects in fields of business intelligence, CRM, geo-marketing research, and channel migration across several countries worldwide. Doug and Mayy, thank you for joining me today.
Mayy Habayeb:
Thank you, Louis.
Doug Nix:
Thanks for having me, Louis
Louis Savard:
These days, there's a lot of hype about artificial intelligence technology, what it is, how it works, how it's impacting our lives and what it means for business. This is a topic close and dear to my heart, especially working in the IT world and trying to dip our big toes into the big bad or big wide world, I should say, of AI. So I'm looking really, I'm really looking forward to our discussions tonight. So let's not waste any more time and let's get right into prompting. So from your perspective, Mayy, on the college side, how would you define AI?
Mayy Habayeb:
I would think of it as an effort to make machines carry out and act as humans. That would be the ultimate goal. So they would carry out tasks that humans are taking, like decision-making tasks. With the integration of robotics, they might be taking tasks like helping in manufacturing plants and industries. under the umbrella of AI, there are many areas like machine learning and deep learning. There's all this natural language processing there is, recommender systems. There is intelligence robotics and computer vision. So, bringing all these categories together, we're still at the beginning, but a lot of advancement have happened.
Louis Savard:
Yeah, it feels like it's, it feels like it's moving at warp speed. And I mean, we're, we're scratching the surface, so to speak, you know, AI has been around for, I'm going to say for a long time in various different forms, but only in the past, I want to say, six to eight months, are we really seeing this big push and this big boom? How does that look like on the industry side, Doug?
Doug Nix:
So my focus is primarily on the machinery safety side of things. But of course, we're also seeing the implementation of AI from the perspective of predictive maintenance. And that's an area that has been fairly widely accepted, I think, from the perspective of large manufacturers who have continuous process systems where they can't afford to have much or any downtime. And so by having AI systems trained to monitor that equipment and to flag maintenance personnel when a bearing is starting to overheat or something needs greasing or there's some other condition that appears to be going out of spec, then those AI systems can help to avoid downtime. and to allow the maintenance personnel to schedule maintenance activities when the demand on the equipment is not there. So there's a lot of ways that AI is coming into play in industry. But from the safety side of things, the safety practitioners tend to be notably conservative in adopting new technologies like this because we're concerned with keeping people from getting hurt. And so as a consequence to that, we want to know that the systems that people are using to protect workers are going to be reliable, and will function when needed. And at this point, there isn't enough proof available to us yet to start to feel confident about that. And I think that's going to start to change in the next little while, but it's still early days.
Louis Savard:
Yeah. When you layer the safety aspect of any systems is it's, it adds the layer of complexity to it. Now we were talking layering over AI and its potential and I can only imagine how complex that could be. The example you gave was quite interesting though on the maintenance side, right? A bearing overheating as an example, right? My check engine light comes on in my car once in a while, but that's been happening for 20 years. Right. So it's, it's, it's, it's, it's nice for our listeners to, to, to kind of put two and two together that there's, it's been around for a while. It's just now it's really, really taking off. And it's really that the potential is really being, being, I guess, achieved or we getting there. But there's so many different types of AI it's, it's head spinning. It really is. And the two top ones that come to mind is generative versus deterministic AI. And Mayy, I wonder if you could enlighten us a little bit on what the difference between the two would be.
Mayy Habayeb:
Thank you, Louis, for asking that. Actually, we started off with deterministic AI, sometimes we call it traditional AI, where basically we are trying to find patterns in the data. and built using algorithms to predict. So it just started off in the 1990s with, is this email a spam or a ham using an A-based algorithm? So deterministic is still evolving and every day new algorithms, especially in the 80s when neural networks started coming out and deep learning. Actually, generative AI came out of the deterministic AI as basically introduction of, let's say, transformers allowed for models to generate new context instead of generating a pattern or a prediction. Now, this new context could be text, could be a video, could be art, And one of the examples is chat GPT, where you prompt to the model and it generates content for you. It can actually write a thesis. And that's the main difference. But generative AI is built on the algorithms that were that the only difference is the large amount of data and computation power that went into it.
Louis Savard:
Yeah, the computational power, I mean, we're going to have a whole other episode about the needs for that. But it's interesting when we went from, you know, looking for patterns to now. looking for pattern, but generic context and the response is conversational AI almost is where, is where we're getting into. And Doug, just maybe get your thoughts on this in an industry. Um, we're still looking more into the, what we'll call traditional style AI, where you're looking for flags to flag other people to do something. Are you seeing a place for generative in there other than to send out notices in a nice way?
Mayy Habayeb:
Not yet. You know, on the safety side of things, we are always concerned about having deterministic outputs from any kind of safety algorithm, any equipment. So you need to be able to know that when a given set of input conditions occur, we will always get the same safe output condition from the system. So I don't really see a place where generative AI is going to be applicable in that particular kind of application. That may change in time, but certainly right now that's where things are.
Louis Savard:
Okay, so let's dive a bit deeper into that then. How does the engineering world, you know, now we've determined that deterministic is the way to go, how does it really use AI in software or maintenance engineering?
Mayy Habayeb:
Well, that's where the standards work that I'm doing in ISO-IEC Joint Technical Committee 1 is coming in. We're starting to develop a number of different standards. And for anyone that's interested, if you hit the ISO website and look up JTC1 Subcommittee 42, you can see there's about 28 standards that we published already with another 33 in work. We published last year a new technical report, ISO-IC-TR-5469, which deals with AI applications and functional safety. And functional safety basically is the engineering discipline that deals with the correct application or correct operation of control systems that are used for safety purposes, this actual safety functionality of the machine. So, in those particular applications, what we're concerned with is ensuring that if AI is used, the appropriate type of AI is used, so deterministic versus generative, and also that it's being used in a way that makes sense based on the risk levels that people are exposed to. And we have sort of a three level approach that we've developed that's outlined in that document. And so basically, if we're looking at a high risk application, it might be possible to use AI, for instance, to digest a large quantity of data and then generate an algorithm that's used, that is deterministic in itself, and that is used to actually create the safety outputs. Or if it's a moderate risk kind of an application, it might be possible to have an AI working in parallel with a conventional hardwired type of safety system. And the two channels in that organization would then have to agree, and if the AI comes up with a different answer than the hardware does, then the hardware takes precedence and keeps people from getting injured. And then the third low-level kind of approach might be where you could use AI for the entire safety function. At this point, I'm not yet seeing AI applications that I think anyone in this committee anyway would be comfortable with seeing used for the full aspect of a safety function. But it's certainly possible to have the high risk and the moderate risk approaches used. So the work that we're doing on 5469 and then converting that into a full standard with requirements in it will help to guide system designers who want to use AI in those ways in how to determine what AI to use and how best to implement it in those systems.
Louis Savard:
That's interesting. Now, I want to Go back to what you mentioned where, you know, if AI and machinery are in disagreement, you know, the machinery will be take precedent. Right. Now, let me, let me throw this situation your way. Um, there's a, there, there's a safety issue. The gate needs to be closed and it's, it's a heavy gate. The machinery says I have to close. Otherwise the buildings get on fire. Okay. But there's a sensor inside and the sensor says, yes, it's more than just an obstruction. It's somebody's hand that's there. I want to give you 30 seconds to remove that hand before you slam that gate down.
Doug Nix:
OK.
Louis Savard:
What what happens in that situation?
Louis Savard:
I figured it was a tough one. So I just, I just figured I'd toss it up there anyway. Right. Uh, but it's, it's, it's so, so let's, let's dive a little bit deeper into that again. And so we talked about sort of the applications of where AI is used within industry, but, but the actual function, right? So one function here that we just, you just mentioned is, you know, it's looking for a human hand and it's going to really back the hardware. Whoa, hang on a second here. Let, let the hand go out before, but what other functions, uh, is there for AI within the engineering world.
Doug Nix:
Well, if you're talking about process type of applications, you know, you might be measuring things like liquid flow in a pipe or pressure, pH, temperature in a reactor or something like that, right? So over time, the AI gets to understand what normal operations look like. And so it can then begin to predict trends. So if it sees that the pH is going in a certain direction and the temperature is going in another direction, That then indicates that the reactor is going to go outside of its normal operating parameters, and that's a dangerous state for it to be in. Then that AI would be able to generate a safety output to shut down the reactor, whatever that means, shutting off reagent flows to the reactor or something else. So, that sort of predictive capability of the AI is what actually would add value in that circumstance because typically a hardwired control system isn't going to have the same sort of predictive capability. I mean, there are some kinds that do, you know, if you think about a PID temperature controller or something like that, that has a certain amount of predictive capacity to it. And I suppose you could think of that PID loop actually as being a very basic AI. But when we're talking about larger process control systems, where we have multiple variables that need to be monitored and so on, that would be very hard to do in a PID loop. And so AI systems that can cope with the amount of data that's coming in and then make correct predictions based upon where those, the directions that those various variables are going in, that's where the value added is going to be.
Louis Savard:
Fantastic. And Mayy, across your breaths of experience, where do you see the AI functions lying in the engineering world?
Mayy Habayeb:
Well, let me start with the field that I'm in, and that's the software engineering field. We have had a lot of research at the level of the software quality. For example, bug reports, a lot of systems like Mozilla have been created to collect from the public, let's say, bug reports. A lot of deterministic AI, let's say, algorithms have been employed like support vector machines or hidden Markov chains to predict if a bug report is going to take two months to fix or less than two months. So, test case generation. Systems have been generating test cases for software systems, you know. Test case building takes time. And that was one of the fields. Another field that generative AI has brought exploding to the table is code generation. And you might have heard of CoPilot or XCoPilot or Tempean. And many of the large players right now are encouraging their software developers to use these models to generate at least 70% of the code. So in this field of engineering, I see that AI is moving very quick to adopting the technologies.
Doug Nix:
Yeah, anytime you can replace 70% of typing with a, here's what I want,
Mayy Habayeb:
Yeah, but the key question is, here's what I want. How do you formulate, here's what I want? That's exactly it. The prompting side of it, the human interface. And one of the skills for a software developer or a software engineer is to manage to do abstraction. So you need to look at the business problem, try to abstract this solution and then convey it to this large language model to generate the text in Java and Python or C sharp or coding language. And that's something that we have to teach these days and emphasize on with our students.
Louis Savard:
Absolutely. And I'll give you another, another side example. Then I have a little bit of a follow-up question, but, you know, even, even in the pure and simple research, right, write a paper on X, right. I've, I've had discussions with, with peers and coworkers where, you know, ourselves, even as a, you know, as a part-time professor, I put more value on my students leveraging these tools to generate most of the content and then focus all of their efforts in proofing what's been put out. Right. So the research, like spend your research time finding the right, um, you know, the bibliography and the references that, that, that, that the AI has bought back for you, because it's so easy to say, write this for me. But it's really not easy to prove that what, what's been written is not a false positive. It's just a mishmash of things, right? So that's a skillset that I think is, is, is valuable, you know, proofing what you're getting. So the same thing with on the coding side, right? Yeah, give me this, but if it's not prompted properly, if you don't know what you need to ask it properly, you're going to get code. There's no doubt about it. You might not get what you're looking for, but you're going to get something. So, I mean, that's what you're, what you mentioned there Mayy is, is definitely what I would qualify as a positive use of AI. Um, so let's dive a little bit deeper into that. You know, I can think of things like, I mean, I'm going to say self-driving cars and policy work or policing in its own military uses, health, healthcare, even in municipal world. Um, Can you elaborate on what you see as positive, responsible use of these AI technologies?
Mayy Habayeb:
So let's just go to policing. There was actually a couple of years ago, a few companies in the US that studied the, let's say, crime data sets. And that's a good thing. Even Toronto police have their own open data portal that we use. They came up with, let's say, some application that would help police to focus on certain neighborhoods based on historical patterns that they found in the data. The positive side that I see there is that you can mobilize your resources or, let's say, have a better efficiency of using resources to cover certain geographic areas. In autonomous driving, there is a lot of advancement. Tesla has done considerably well and right now a lot of other manufacturers are following pursuit. And there are some statistics out there comparing number of accidents done by human drivers versus the autonomous vehicle. And we can see that they're comparable and sometimes the autonomous driving is more safe if we look at numbers. Yet, there are challenges in that area.
Doug Nix:
And if I can speak to that as well, Louis, you know, I was reviewing an article today talking about how the NHTSA in the States is pursuing a probe into Tesla's autopilot. And one of the things that motivated that was a recent accident where a Tesla driver who was operating his vehicle in autopilot mode, he was distracted by his phone, didn't realize that he was approaching a motorcyclist from behind rather rapidly and didn't know that there was any obstacle in front of him until he hit the motorcyclist and killed him. And this is not the first time that this has happened with Tesla's operating in autopilot mode. Now, I'm sure that these similar sorts of accidents are going to be happening with other automated driving applications like this because the technology is at a certain level and, you know, this is part of the problem. One of the things that you frequently hear AI professionals talking about is that you need a human in the loop. And that actually means that the human has to be in the loop. You can't be sitting there staring at your phone, reading a book, having a nap, doing something else while you're sitting behind the wheel. You actually have to be paying attention to where the car is going. Sure, the car is maintaining direction and speed and doing those things, but you have to be there to ensure that if the AI fails to notice a hazard of some kind or a vehicle or an overturned transport truck or something else on the road in front of you, that you can actually take control and bring the vehicle to a safe stop before you get there. And unfortunately, a lot of people are putting far too much confidence in the capabilities of these automated driving systems and they're getting themselves into trouble. And unfortunately, some people are dying. You know, between 2018 and this year, there were about 476 accidents in the U.S. that are being investigated as part of this NHTSA probe, and of those, about 14 fatalities involved. So, 14 fatalities in terms of all of the miles driven in a year is fairly low, but nevertheless, it's a concerning trend that we're seeing, and that's why, you know, the regulators are trying to figure out how to deal with some of these kinds of concerns.
Louis Savard:
Yeah, I mean, it's definitely concerning, right? So what, let me ask you this, if you can't answer it, if either you or me can't answer it, if you just want to give your insight, that would be great, but we're talking cybersecurity, right? We're talking self-driving vehicles, AI driven, there is a vector of entry for bad actors to do really, really bad things with that technology. Did you want to speak to that for the next 30 seconds?
Doug Nix:
I think from my perspective, one of the things that opens that vector is having updates over the year. You know, if there's a way to ensure that your Tesla or whatever your automated driving vehicle is, if that car can only get system updates from your home network, as an example, and you have a way to ensure that that's true, then maybe updates over the air are not so bad. Personally, I would much rather see an Ethernet jack on the dashboard of my car where I could plug the car in and let it connect to my network and get the updates that it needs. There have been a number of instances where let's call them white hat hackers, have demonstrated the ability to hack into the control systems on vehicles, turn the windshield wipers on and off, apply the brakes, alter the vehicle speed. And these are not systems necessarily with autopilot. These are, you know, I've seen it done on Lincoln Navigators and other vehicles like that. So it's possible to hack remotely into some modern cars. And part of the problem, I think, is that the automotive industry has not been as aware of cybersecurity issues as conventional IT managers and IT departments have been. And we've seen that in the industry, in industrial control systems as well. There's a long list of dam control systems and water supply, water treatment systems and so on that have been hacked into by people in a variety of ways and certain levels of havoc created. So, you know, it's an ongoing concern. The European Union has taken steps by adding clauses to the new machinery regulation that deal with cybersecurity requirements, and machine builders are expected to ensure the cybersecurity of their equipment. They're dealing with the implementation of AI in machinery through the perspective of software in general. and the need to prevent the software from becoming corrupted in any way. Whether it's, you know, through the effects of electromagnetic interference or whether it's unauthorized people getting access to the control systems, there's a variety of ways that could happen. From the regulator's perspective, they don't care what the source is. They just care that you protect against those things. So that's my perspective on that.
Louis Savard:
Thank you for that. Right. And, and we, I know if you can, can follow sort of my bouncing ball, I'm trying to steer us into the, we were talking about the glory of AI and how positive a good thing is. And now we're kind of a little bit of a, the sky is grayer right now. Right. Uh, so Mayy, going back to you, uh, we share some of your thoughts on some of the negative implications of AI and in particular things like chat GPT, generative AI in general, it's used in the military, policing, et cetera.
Mayy Habayeb:
Okay. So as much as Giants of AI and chat GPT, at least the large language models are taking people by surprise, but researchers have came up and tested a lot of these models. And we have to understand that these models do generate new content and Although it's fascinating that they speak like humans, they are not accurate. So that's one thing. And one phenomenon, at least for large language models, is they tend – not large models, let's say – is they tend to hallucinate. One recent incident was in the mayor's, Toronto mayor's race just recently when in one of the campaigns, the campaign manager just decided to produce all the artwork and images and One of these images showed a family with a lady with three arms and nobody noticed it. It just went out. And CTV News and all the news channels just managed to make a big report, a big reporting about it. and that is the hallucination. Other things that researchers have noticed, they tend to speak like humans and they tend to give you an affirmative nature, especially in chatbots. And last March in Euronews, it was reported that a person was having a conversation with a large language chatbot and ended up taking his life about climate change because the chatbot convinced him to take his life for the cause. Other things is we need to look at how we use AI, for example, in recommender systems. So we know Netflix, we know Amazon, everybody is using recommender algorithms in order to recommend things to people that they didn't know about. And that's quite fine and it's helping the helping me when I'm shopping, helping the viewer when they're watching, helping somebody listening on Spotify to find them. But if you're using a recommender system on Spotify and you recommend, let's say, a song and it turns out to be not your taste, I mean, you'd be mad for, let's say, that day or a couple of hours. But if you use the same algorithm to recommend a foster parents for a child and it goes wrong there, then the effect of that is a lifetime, not a couple of hours. So we should be taking reason in how we use AI and in what domain we're using it. The levels of risk change. Facial recognition. when it was used to decide in the courts if somebody can be remanded or can get out on bail. And it turned out that the facial recognition apps could not identify features of faces when the skin is very dark. And that was really, if a judge would just depend on AI's recommendation, it wasn't fair, simply that.
Doug Nix:
There's a few cases in the States too with the court systems where some of the U.S. courts were using recommender systems to suggest to judges what sentence was appropriate in a case. And there was significant bias in those systems based on having the ethnicity of the defendant noted as African-American. And so, they were pushing for harsher sentences for African-American defendants than they would have for a white defendant. So there's lots of places where these things can go terribly wrong if the bias and the ethical application of the software is not looked at carefully.
Louis Savard:
Yeah, it's not a single lane highway, right? It's a multiple lane and it's two ways and it's, there's maybe even no median and some crossroads across, you know, around the way. So it's more complex and people, they make a sound.
Mayy Habayeb:
And that's why we would advise that for bias, the data that we train our models on to be checked against the domain and against, so it can be like we can handle it, but it needs to be checked at the data in the pre-processing and transformation of the data that we're going to use to build those models or those recommender systems.
Louis Savard:
Yeah. You can't remove the human aspect. It has to be there in some form. Yeah. There has to be some validation. Right. Um, so that that's great. So now go back to Doug in your world and in the systems maintenance world, what are some of those negative implications of using AI?
Doug Nix:
Well, I think from the maintenance side of things, probably the only real downside I can see is the cost of initial implementation. You have to instrument the equipment, so you've got additional sensors and so on in places where you might not normally have thought of having them before. Depending on the nature of the equipment, the sensors themselves may have to be made in a particularly robust manner if they have to work outside or in harsh temperatures or very dusty conditions or with corrosives or whatever. So there's that aspect of things. Also, it typically takes those predictive maintenance systems some time before they've learned enough about the operation of the equipment under normal conditions before they can start to make any useful recommendations. So, you know, it's not like you can bring a system like that in and turn the switch and then tomorrow it's going to start giving you good information. You know, you're probably still going to have to run a conventional either paper-based or database-based preventive maintenance schedule for a while, maybe a year or so before there's enough data accumulated in the AI system that it can begin to make useful recommendations. So, you know, it's that whole time and cost side of things. That's probably the biggest one that I see. And then on the safety side, it would just be having a system decide poorly about a situation, decide something is safe when it's not, and then end up with either, you know, a catastrophic disaster of some kind, like a reactor running out of control, and then, you know, a chemical explosion or something at a plant. Or, you know, on the individual human protection side of things, having the system decide that the system was safe when in fact it wasn't. And then, you know, somebody ends out with a broken arm or at worst dead because the system made a bad choice.
Louis Savard:
What about morale? Right. And I'm just going to put it out there because, you know, it's the, you know, I'm losing my job. AI is coming in. I'm, I'm done. Right. So where does that play in the whole equation, uh, on the industry side of the, and then, and then may, I'll be interested in hearing your, your thoughts on that on the college side, uh, the colleges and where that this place. So, so Doug, if you don't mind, well,
Doug Nix:
You know, I think that we're not going to be putting any maintenance employees out of a job with AI because at this point AI can't turn a wrench. So that's not a concern. You know, maybe one day if Boston Dynamics works on their bipedal robots long enough, They'll eventually be a robotic maintenance guy with his very own crescent wrench, then he can go out and give the recalcitrant valve a smack and things get working again. So I'm not worried about that particularly. And from the safety engineering side of things, understanding the interplay of regulations and making sound choices about how to um, design systems and so on to be in compliance with the regulations and also functional, uh, is something that's still complex enough that I, I'm not too concerned about, uh, AI coming from my job anytime soon. Although, you know, like they said at the AI summit, um, it's not that, uh, the AI is coming for, for your job. It's that the, uh, the human practitioner who also uses AI is the person coming for your job. So it's useful to understand how to use it effectively in your day-to-day work. So, you know, that's what I see. I think on the design side, you know, there's a lot of applications where AI is coming into design and at some point we'll be able to do a lot of the drudgery side of the design and allow the equipment designer to be thinking about the bigger picture. But, you know, again, it's early days. Designs can be optimized by AI right now, but I don't think we're at a place where you could sit down in front of an AI-driven AutoCAD application and say, OK, AutoCAD, today I want to design an assembly line that's going to do this and have it spit out the drawings two days late. We're not there yet.
Louis Savard:
And me on your side in the software engineering world or even the college, the college world, where do you see the implication of AI versus employee or even student morale play?
Mayy Habayeb:
So in the college, let's say in the education field, a lot of thought is being, okay, first is plagiarism. How do you test? How do you evaluate? Do we forbid chat to be teeny? Can we forbid it, for example? All the institutions are just trying to put in policies and procedures on the use of generative AI, because generative AI in education has shown a big potential. on the tutoring side. So, sometimes some colleges don't have enough extra tutors to do one-on-one with students. Are they mature enough? No, but there is a lot of advancement happening there. In the schooling system where some of these courses, mathematics, English, that you can build tutors, Asians, chatbots, there is advances that are happening on that side, on the grading. to help professors grade students' work. We can deploy and build models to do that. In software engineering, it is changing the role of the software developer for sure. And that I can see it happening within the next couple of years where software developers will, like Doug said, be looking at the big picture and learning how to prompt models to generate code. Mayybe they would be more focused on the integration testing because software systems need to integrate into each other. And that's where I see that it's moving fast. Even the course levels like the software engineering courses where we teach programming languages need to change to embrace the part where we can show the student how to generate the code using a language a, let's say a generative model to do that.
Louis Savard:
Yeah, that's perfect. Now let's, let's stay on that topic for one more minute and talk about algorithms here. Now, we, you know, we talked about the Netflix algorithm and yeah, you know what, if I, if I get my, you know, true crime documentary and that's not what I was looking for. I mean, I might be bummed out. I know that I'll be extremely upset, but I'll just keep surfing and looking for something, but What happens when an algorithm goes horribly wrong that has large implications? What are those potential outcomes or risks?
Mayy Habayeb:
Think of models as a live piece of software. When you put a model into production, you just can't leave it there and turn your back because this model's logic has been built on data and data changes. One example is a chat bot that was put out there and it was trained to retrain itself based on the chats it received from users. It just took them 16 hours. where they had to shut it down completely because it got so aggressive with the users. Now, you have to shut down the model. It's simply like that. There's a new field. I don't know if it's called machine learning operations or MLOps. And just a few months ago, there is LLMOps, which is large language model operations. And all of this has been developed based on the DevOps, which is now maturing, but in In the field of AI, you just can't throw a model and turn your back on it. You have to continuously monitor the results, put safeguards in the production environment. Let's say you use supervised learning to train a model and you did validation testing, cross-validation, and then you did your testing again and again and you got accuracies of 96%. Mayybe it's acceptable for a certain domain. Medical would not accept even 96% because of light. And then you put it into production. Don't expect that you're going to get a 96% in production. Nobody knows. You have to keep on checking. So there is a field of what we humans in the loop was mentioned, active learning, where you learn the model and create something called feedback loops for the data that is happening during the production of these models.
Doug Nix:
Mayy, I think that chatbot you were talking about was the one that they called Tay, right? Tay, that's correct. Yeah. I didn't want to mention the name. I remember that case pretty well.
Mayy Habayeb:
It was all because of a feedback loop that they put in to automatically retrain the model.
Doug Nix:
Well, and there was another chatbot fiasco just recently with an Air Canada online recommender chatbot. And the particular customer needed a bereavement flight to go and look after a parent or a relative who died. And so we asked the chatbot to recommend the best way to go about getting the bereavement pricing. for the particular flight he needed and the chatbot said oh don't worry about that just buy the thing and then you can apply for the discount afterward. And in fact then it popped up a couple of links one of them was to the bereavement fare web page that gave the FAQ for on bereavement fares but the person never clicked through to those things. So, Air Canada's perspective on that was, well, you know, we don't actually supply the chatbot, you know, it's something that we buy in from somebody else and so it's the other company's responsibility. And in the end, the court held that Air Canada was liable for the difference in the fees and had to refund the difference in the fees to the traveler. So, there are circumstances like that where, you know, that's not a life and death situation, but certainly it's a major inconvenience for the person involved. And, you know, I'm going to come back to standards, one of my favorite topics. There is a standard on risk management for organizations that are implementing AI models. And so, you know, these types of tools are things IT managers can use when they're working at it, decision makers in the organizations can use to help determine how best to mitigate the risks that go along with the implementation of AI in various ways, whether it's a chatbot or something more significant than that.
Mayy Habayeb:
Yeah, I can add to that. There's a lot of AI projects out there that fail. The most successful AI projects that I've seen come from cross-functional teams. When you have subject matter experts in the doing, that is key for the success. So those subject matter experts would understand the risks and would be able to point out that even if you train a model with a 99.9 and the domain is a health domain and the risk will involve death or life, it's not going to be acceptable. But just to run with a project as IT and implement it carries a lot of risk.
Louis Savard:
Yeah, you know what, and that's the world I live in. Right. So, so I'm the IT manager for the municipality. That's the word I live in. It's the, you know, we have to have proper governance and the right tools and processes in place before we can even contemplate implementing a tool that could just run wild. Right. And, you know, the end users have a great, you know, they have an end goal vision in mind where I wanted to do this, but you have to be prepared on the upstream part of it to get there. Right. And that's the hard sell. Right. Why do I have to do this when the tool's there? I just want to use it. That's true. Right. Once they understand though, they understand. Shifting gears a little bit here, let's talk regulations. So, Doug, what are your thoughts on regulating AI? You know, Are there regulations that deal with trust and mistrust? Are there any that are in the hopper coming in soon?
Doug Nix:
They're getting close. The European Union is moving fairly quickly towards putting out a directive or a regulation on AI trustworthiness. It's been in consultation for some time, so it's still rattling around in committee in the European Parliament. But, you know, we are expecting to see that legislation published sometime this year. The last time I saw anybody who was trying to commit to a date, they were saying was going to be in Q1 of 2024. Well, Q1 2024 is gone, so clearly we didn't quite get there, but it's going to happen at some point. You know, the Canadian federal government has published an AI code of conduct that they're trying to get AI developers to follow. And you can find that on the Government of Canada website. People are having trouble finding it. They can reach out to me after the podcast and I'd be happy to point you in the right direction. The US has also done similar things. The National Institute of Standards in the US has published some AI risk management frameworks and so on that are there as well. But no one, no government to my knowledge yet has committed to legislation that's going to govern AI quite yet. I think the EU is going to be the first.
Louis Savard:
Interesting. Now, no need to respond to this question, but I wonder if the governments are using AI to develop AI governance. I mean, this is just some of the heavy lifting anyway, just jokes aside.
Doug Nix:
APT, please write me legislation for.
Louis Savard:
Yeah, that would be, that would be scary. That would be very scary. You know, so Doug, I know you participated in the, in OSS AI summit in April. I'd like to get your thoughts on the event, but at the same time, I know that OSS is working on, you know, guidance, governance documents around AI. And I'd like to hear your thoughts on that as well. And where do you see the value of it?
Doug Nix:
I think it's super important for our professional associations, whether it's PEO or OSET or any of the other engineering and technology societies in Canada, to provide guidance to members about how to implement AI in their particular practices in ways that make sense. And so part of that is obviously risk management, but part of it is also looking at, from an ethical standpoint, what does it make sense to use? You know, just because you can doesn't mean you should. And so we need to look at the moral and ethical implications of what it is we're doing. And, you know, the IEEE's motto is advancing characteristics of humanity through technology, right? And we're always thinking in IEEE activities about how is the thing that we're proposing, the technology that we're proposing going to advance humanity? And so we have to really carefully look at not just financial advancement, but also moral, ethical, social, spiritual advancement through the technologies that we're bringing. And obviously not all those parameters are going to apply to every application, but Certainly, we need to be taking that into consideration. And so, the guidance that comes out of something like the AI Summit is the beginning of that process. It's the place for the practitioner to start, and then they can begin to consider that within the scope of their own work. It's great.
Louis Savard:
We'll give you a break for a couple of minutes here, Doug. I want to focus on Mary for a little bit and more specifically on the software engineering technology AI diploma at Centennial. It's a fantastic program, by the way. Can you tell us more about the program? The graduates, what kind of jobs they land after the fact and the success rate of the program?
Mayy Habayeb:
Okay, yeah, so it's a three year advanced diploma. We launched, like we started planning in 2018, end of 2018 for the program, because we could see that there is a demand in the market. And we launched our program in 2020. And so far we have graduated two batches and we're graduating our third batch this June, this next month. The program focuses on giving our students all the skills required to create an AI capability and embed that that AI capability within an application. So, and within a full stack application, let's say front end, back end. So, they encapsulate the capability. So, it's not just data science. So, we cover data science. We cover machine learning, deep learning, big data. We cover recommender systems, and we have a full course on ethics. as part of the program. In addition to that, our graduates are getting to learn all the coding languages, so they learn C-sharp, they learn Java, they learn Python, JavaScript. They also learn mobile app development where they take a course, a 14-week course in project management. and three courses in communication skills and general education courses that cover global citizenship, social justice. So it's a really rich program that we give all the skills and that's what I think differentiates us from other programs in the market. Our graduates have secured jobs with titles such as machine learning engineer, AI developer, AI technologist, full stack developer, software engineer, quality assurance engineer, We have three of our graduates working at one of the largest banks as data cloud computing engineers. I think that's basically it. If you have any other questions about the program, feel free to ask me.
Louis Savard:
I absolutely do. Uh, I mean, I guess I think it's a fantastic program, but it can't be all roses. Yeah. Right. What any challenges that you faced, uh, either in the development or even into the actual program rolling out right now?
Mayy Habayeb:
Yeah, for sure. When we developed it, the pandemic hit. So that was the first challenge, because once we got the ministry, the Ministry of College's approval, I started developing the first course and then suddenly everything closed down. And we had the challenge of converting everything into online. And we actually, the first batch that joined were fast track batch for two years. They took all the, the first time we saw them was on graduation day. So that was one of the challenges. Now we have a continuous, the field is evolving very quick and keeping the program keeping the program in pace with all the new developments, that is a challenge in itself. It requires a lot of resources from our end to do that. On the other end, we also took into account that we use, we have research activities, applied research activities that we do with our research department where we get involved with the market, with the industry. So, small and medium companies are coming in to Centennial and our students are working on projects. with these while they are studying. So instead of a student taking a part-time job at Tim Hortons, what he learns, he applies with these small and medium companies. Another benefit is we do have a co-op option. So if a student And it is competition. We have a very strong co-op department that has ties with the major large players in the market. And if a student qualifies for co-op and there is an opportunity, then he spends an extra year working on the job.
Louis Savard:
That's fantastic. You know, all the partnerships and those, you know, on the job experience that you can get, uh, any student or even, you know, you just want to step ahead when you enter the workforce right there. So I applaud you for that. Um, final words, um, Doug, general outlook on AI, the future within your industry in general, go.
Doug Nix:
Okay. I would say that I am a net pro AI. I think there are a lot of things that we can do well with it. And, uh, uh, you know, it's, it's not the ultimate hammer. You can't turn everything into a nail and smack it with the same hammer. But there are lots of things that it's going to be very, very helpful for. And so I would encourage all of our listeners to become familiar with the tools that are available to you. Get good at using them because they're going to help you a lot in your career. And, you know, in time, some of these other aspects that we've talked about where there are problems like automated driving and other aspects like that, will get better because there are very talented people working on that every day trying to make those systems better. So that's my take.
Louis Savard:
Perfect. And Mayy, what about you? General outlook on AI, the future in software engineering in general?
Mayy Habayeb:
Yeah, I tend to see a very positive outlook. I see awareness. I see people started embracing it. And things are getting better with proper awareness, with the advancement that are happening at the computational levels, which are enabling these algorithms to get access to more and more data. We're going to see more and more apps that are going to hopefully helpless. We're still in the beginning and I can see that it's going to advance and we should embrace it.
Louis Savard:
Yep. I'm going to echo everything you guys said. I'm absolutely pro, you know, done in the proper way. And I think we're getting there. I think the, you know, it's, you have to, you have to get, you know, bumps and bruises along the way to figure out what the right path is. And I think we're, we're at the bumps and bruises stage of it. Um, actually, I mean, for our listeners, you know, can't see me, but I'm holding up my cell phone and I had a conversation with, uh, with someone that was, I'm not going to say anti or, or You know, not for AI, but it was very apprehensive. I said, you know, it's got no place in the business world. And I said, do you have a cell phone? They said, yep. I said, how do you unlock your cell phone? So my thumbprint said, well, did you know that's AI? And they paused for a second and then they, I saw their shoulders drop and said, okay, maybe I have to rethink my position here. So it was, you know, it's once you start realizing how widespread it is into your day to day. But it's not chat GPT. So the connection is not made that, Oh, this isn't chat GPT, so it's safe, but it's AI is AI, right? So it's all around us.
Doug Nix:
It's all around us in ways that people don't even begin to appreciate. So.
Mayy Habayeb:
Yeah. So imagine that we take out the spam filter from that person's email. Yeah, absolutely. And let him do the splitting of all the incoming email that is coming in.
Doug Nix:
I mean... Well, and there's a lot of malware detection systems that are being deployed in organization IT systems that rely on AI to detect malicious behavior on the part of... That's true. That's true. So there's lots and lots and lots of places where AI is already doing really good work. And we need to be aware of that too. You know, if you think back to the early days of the industrial revolution and the introduction of electricity into society in general, you know, there were a lot of false starts. And, you know, Edison's first DC power stations in New York City used the earth as a return conductor. And that was cool until they started to realize that people were getting electric shocks just walking by the power station because the voltages that were being created across the soil were such that people were getting shocks between their feet. So, you know, oops. And so now we use different ways of bringing the power back to the generation station rather than just letting it float through the soil. So we're learning these things and there's always issues when you introduce new technologies. There just is.
Louis Savard:
Absolutely. Absolutely. Well, listen, we'll, we'll, we'll, we'll stop it here. I mean, I feel like we could be here for another two, three hours and then really dive deep. I really, really enjoyed our discussions. Um, and, and for our listeners out there, I, I have to tell you that I, I work in this field into the implementation side and leveraging it. Uh, but I've learned a lot more. I'm adding more to my bag of knowledge after today. And I certainly hope that you have as well. Doug, Mayy, I really enjoyed our conversations. Thank you for providing such insightful information about AI. Thanks, Louis. Thanks, Louis. And as always, I want to thank our listeners for joining us. Remember, if you are interested in learning more about today's topic, or if you had a topic you would like us to feature in a future podcast, please email us at Tech Takes that's T-E-C-H-T-A-K-E-S, at oaset, that's O-A-C-E-T-T, dot org. Until next time, bye for now.