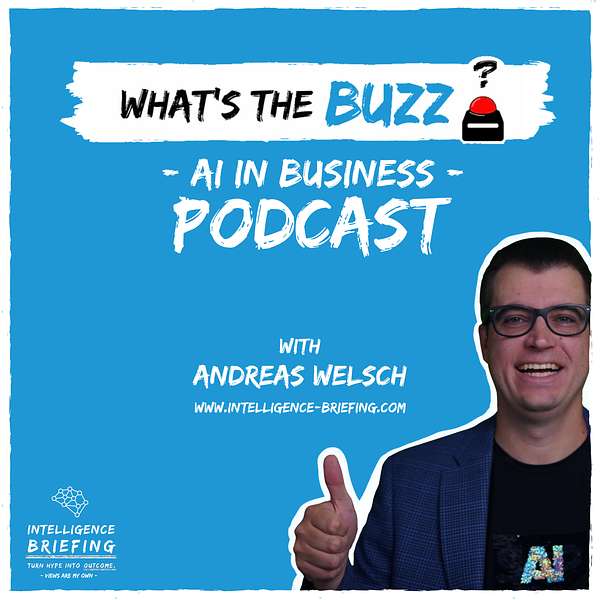
What’s the BUZZ? — AI in Business
“What’s the 𝘽𝙐𝙕𝙕?” is a bi-weekly live format where leaders and hands-on practitioners in the field of artificial intelligence, generative AI, and automation share their insights and experiences on how they have successfully turned hype into outcome.
Each episode features a different guest who shares their journey in implementing AI and automation in business. From overcoming challenges to seeing real results, our guests provide valuable insights and practical advice for those looking to leverage the power of AI, generative AI, and process automation.
Whether you're just starting out or looking to take your efforts to the next level, “What’s the 𝘽𝙐𝙕𝙕?” is the perfect resource for staying up-to-date on the latest trends and best practices in the world of AI and automation.
What’s the BUZZ? — AI in Business
Prepare Your Business Teams For Generative AI (Guest: Bill Schmarzo)
In this episode, Bill Schmarzo (Professor & Author, Dean of Big Data) and Andreas Welsch discuss preparing your business team for Generative AI. Bill shares his learnings from teaching business teams about data literacy and provides valuable insight) for listeners looking to help their stakeholders develop a better understanding of Data, Artificial Intelligence, and how to best use them in a business context.
Key topics:
- Discuss the importance of AI and data literacy
- Explore ways leaders can empower their teams in AI and data
- Identify key partners for AI Centers of Excellence in promoting AI literacy
- Learn a leadership trick to boost organizational AI literacy
Listen to the full episode to hear how you can:
- Understand how your data is being used to personalize information
- Demand transparency about AI-driven decisions
- Apply a Socratic mindset when using Generative AI and ask questions
Watch this episode on YouTube:
https://youtu.be/ghVxy23zzpM
Questions or suggestions? Send me a Text Message.
***********
Disclaimer: Views are the participants’ own and do not represent those of any participant’s past, present, or future employers. Participation in this event is independent of any potential business relationship (past, present, or future) between the participants or between their employers.
Level up your AI Leadership game with the AI Leadership Handbook:
https://www.aileadershiphandbook.com
More details:
https://www.intelligence-briefing.com
All episodes:
https://www.intelligence-briefing.com/podcast
Get a weekly thought-provoking post in your inbox:
https://www.intelligence-briefing.com/newsletter
Today we'll talk about how you can prepare your business team with AI. And who better to talk about it in someone who's recently written a book about just that. Bill Schmarzo. Hey Bill, thank you so much for joining.
Bill Schmarzo:Thanks Andreas for having me. I'm looking forward to this conversation.
Andreas Welsch:Wonderful. Same here. Hey, why don't you tell our audience a little bit about yourself, who you are and what you do.
Bill Schmarzo:Primarily I'm a teacher. I love the opportunity to work with students and with different corporations and organizations to help them understand more about the data, the analytics, and how they can use those to drive value. I also work at Dell Technologies where I'm their Customer AI and Data Innovation Strategist and I get a chance to work with customers of various sizes. Trying to that same mission of how do I unleash the economic value of our data?
Andreas Welsch:Thank you so much for sharing. Sounds like you have a good mix of the industry and real life expertise and where you're getting in front of many leaders trying to do the same thing and then being able to bring that over to academia as well. Now for folks those of you in the audience, if you're just joining the stream, drop a comment in the chat where you're joining us from today. I'm always curious to see how global our audience is. Now, Bill, should we play a little game to kick things off?
Bill Schmarzo:Yeah, I love games. Let's do it.
Andreas Welsch:All right. What if AI were a movie? What would it be? 60 seconds on the clock to make a little more interesting?
Bill Schmarzo:Oh my gosh. It'd be gladiator. Now you're gonna think, why the heck would you pick Gladiator? But there's some lines in that movie and the one that particular that sticks out to me is that what you do in life echoes in eternity. And I think that is such a powerful statement that says that what we are doing today around helping others deliver more relevant, meaningful, responsible ethical outcomes using AI and data will resonate for years even after we are gone. So to me, if AI were a movie, it'd be Gladiator and it'd be holding AI itself accountable for driving those kinds of impacts, even after we ourselves are gone.
Andreas Welsch:That's a really interesting take. I've heard a number of different guests mention other movies, but Gladiator, so it stands the test of time and it's something for, the history books. Look, last week we had Conor Grennan from NYU and he was talking about chat GPT and how easy Chat GPT makes it for anyone to use AI these days. But I also keep hearing that the business needs to become more data in AI literate beyond just playing around with Generative AI. So I'm wondering why is that data and AI literacy still important when tools like Chat GPT make it so easy for anyone to use AI?
Bill Schmarzo:ChatGPT? It can be dangerous. Now I'll tell you right off the bat, I use chat GPT frequently, and I call it my Yoda, your own digital assistant. It helps me to expand my research. It helps me to accelerate exploring things. And even in my class, I mandate the use of GenAI as part of my thinking like a data science methodology because I think it helps students to explore more freely, but it doesn't replace the need for AI and data literacy. We need to first off understand how are organizations capturing data, capturing data about us and about other people right through and all the ways that data's being captured, even as we converse here and a script being created is capturing information about us. We need to understand, number two, how analytics are used to uncover our individualized predicted behavioral and performance propensities,'cause those propensities are being used to influence us sometimes in positive manner, sometimes to manipulate us. Number three, we need to understand some very basics about statistics and probabilities. Not in a big sense, but we need to understand that life is about improving the odds of survival and nothing in the world is a hundred percent. Except for the love of a daughter. And so we need to make certain that we understand some basics around we're making decisions with a goal of improving a probability of making a better decision, which is the fourth component, right? How do I make better decisions? And statistics, analytics data are all about making better decisions. The next component is around value creation. So how are we using GenAI? How are we using analytics? How are we using AI to help create value? Now that's a really hard conversation. It's an economics conversation.'cause it requires you to think very holistically about how you as an individual create value or how your organization you're in creates value and how it defines and measures that value creation effectiveness. The beating heart, by the way, of literacy is ethics. And we need to think holistically about how we are working ethics into our data. So how are we unbiased data sets and how are we working ethics into the models that are generating and recommendations and decisions that impact people? So the ethics is the beating heart, but the last component, the thing that enables everything else, that wraps around this, is cultural empowerment. That everybody needs to feel like they have a voice in how AI and data's being used to them. Everybody needs to understand their role, their responsibilities and their rights when it comes to how AI is being deployed and used.
Andreas Welsch:I like especially the last part about making it personal and developing that understanding of how AI is being used, how it influences you and also what you can do with it. So for those of you in the audience, if you have questions for Bill, please put them in the chat and we'll take a look in a couple minutes and pick some of those up. Couple days ago I saw a news article where leaders said, this generative AI thing is great and we love seeing that. So many companies jump on that bandwagon, so many software providers. But we need certainty, right? It, has to be a hundred percent. Right now, it, might occasionally go off the rails. So to me, that's a clear need and a use case, if you will, for creating more data literacy. It's not a hundred percent, it's not always a hundred percent, and it most likely will never be a hundred percent, whether it's statistics, whether it's machine learning, AI Generative, AI, whatever you call it, right? That's the fundamental at the core of it. So thank you for sharing that as well, that there is a need to create more of the literacy. Connected to that example that I just shared, what is your recommendation? How can leaders help their business peers who might not be as deep in tech and in data to get more AI literate and, to become more data literate on the business side?
Bill Schmarzo:On the, Generative AI front, the best way to learn, is to do it right. Just get involved. If you're not using one of the Generative AI products today, then you're already behind the curve. It's a very powerful tool. Now, let's make really clear, it is only a tool, right? It is only a means to an end. So as a user, I still need to understand what my end point is, what am I trying to accomplish? And get involved with it. But when you start interfacing with this thing, I'm, of two faces of this Generative AI stuff. I think it's a marvelous assistant in helping me to do more research and accelerate my learning. But I approach it with Socrates in mind and the Socratic method of how I ask questions. I'm always seeking to validate. I'm always drilling into more detail. I'm asking what's the rationale for what you're telling me? Cite me the sources. So I come in a bit dubious. I still am responsible as a human for the critical thinking that goes into saying, do I believe what is telling me? Have I done enough validation as a detective, as to make certain that what I'm hearing back from that my favorite GenAI tool is of something that I can actually believe in? So ChatGPT, start using it. But understand, it's more of a conversation that you're gonna have. Not a one-point thing. You're not gonna say tell me this, and then be done with it and say, oh, that sounds like great. It's like walking down the street and ask'em, some random person, some tell me the facts about this and they'll make up some crap. But you wanna you gotta validate exactly what it is you want and you as the human in the conversation, it's only your assistant. It's not making decisions. It's sitting on your shoulder like a little Yoda, your own digital assistant whispering in your ear, but it's not making the decisions for you. You still own making those decisions. So think about embracing the Socratic method. Think about critical thinking as you drive this innovation, and it will come up with great stuff. You will be more productive and you will think more broadly about things. It's gonna, it's gonna give you the chance to do that, but you still own it.
Andreas Welsch:I love how you connect that, right? We have to be aware. We have to have some critical thinking and knowledge of our own, whether we already have the knowledge and we seek to validate it or expand it, or after getting the response going and do the fact-checking and validation. Now on the more traditional Data, AI, Statistics side, how can leaders help their business peers there where it's maybe not as immediate, as tangible an app on your phone that you can ask questions?
Bill Schmarzo:So what I see work is the integration or embedding of the business stakeholders with the data scientists trying to solve particular business challenge. I find that the best, most successful organizations always start by trying to figure out what it is we're trying to do, right? How do we create value? What is what's important to our organization over the next 12 to 16 months? And then driving a process that drives a collaboration between the business stakeholders who are the ones trying to define and measure the value creation effectiveness, and the data science and data engineering organizations who are trying to create the data and build the models to help, to optimize the decisions we're making. There's a natural collaborative process where I don't think you're gonna see business executives writing their own AI models. Maybe G n.AI gets us there in 3, 4, 10 years, who knows. But today I'm providing the desired outcomes, concerns, the risks, the KPIs, the metrics around which I'm gonna measure effectiveness. The data science team is building the models, trying to optimize that, and there's a collaborative process around feature engineering and understanding the cost of false positive and false negatives, and creating that feedback loop for when the results come out. Because what you're gonna learn very quickly from the business side, the data scientist won't give me absolute answers. You said it really well, Andreas, I'm not gonna get a hundred percent. So my first one might be maybe it's sixty percent and then I learn, and then it becomes sixty-seven percent, and then I learn it becomes seventy-one percent right? These economies of learning, this continuous learning and adapting process is heavily dependent upon the continued collaboration between the business stakeholders and the data science teams to deliver on the promises of those values creation processes.
Andreas Welsch:I've seen this work really well in individual projects where you have data scientists, where you have some business stakeholders and experts and you put them together and with joint sponsorship and ownership from both the technology and the business side, you have them work on a problem or on a project. And what I've always found interesting is when you see them over a couple of weeks or maybe a month or two, depending on how long the project goes, how the data scientists learn more about the business and what questions do we need to ask and, say, what is the finance business function really about in a particular problem? When do we need to send a reminder to our customers? Are you really going to pay us? You're seven days late. You're 14 days late. And, how can you predict, for example, who has a higher propensity of paying you? Yes, there's a technology component to it, but it only comes to life through the business context. So having that, the dialogue and on the other hand for folks, for example, in the finance function to learn more about what can I do with AI and how can it help me? But I know that's not the only way, right? You don't just only learn through projects, about each other and about each other's projects and problems. So I'm wondering with whom should say AI Centers of Excellence or AI teams partner to increase that AI literacy in a business? It's not just projects.
Bill Schmarzo:I think overall that organizations need to have a foundation of understanding about components of how AI models work, how data's being used. So the kind of the topics I covered are a base foundation. I would mix in that design thinking as a base foundation as well, where I have the ability for the organization to be able to understand and empathize with who their key stakeholders are, their key constituents. To me the overarching opportunity and the challenge is around understanding how to create value. And that does require the collaboration. Now, if you have an AI Center of Excellence and you know they're partner, they should be, they're probably partnering with universities or other organizations to really learn more about this. For example, the new Transformers capabilities and the new technologies are there, which is great. You really wanna learn about the new tools, but it always comes back to application. Learning about all these great tools is great. And, but knowing where to apply them to create. If as an AI organization, all you're doing is learning about great new tools and right, and posting research studies and things, and maybe you're at a university and that makes something gets you get pats in the back for that. But for most organizations that doesn't matter. It Just doesn't matter, right? It's all about application. And so I think at the end of the day, if you're not creating that tight linkage between the business and the data science team with the expectations that we're going to build a solution that's going to evolve over time. It's gonna embrace the economies of learning and the power of compounding to become more and more effective. Then I think all the stuff you do in the AI labs is it's kinda like monkey tricks. Who really cares, right?
Andreas Welsch:Yeah. I love monkey tricks as a term, not so much as the result of the work. Bill I see a question from Dan in the chat and he's asking, how do you prepare organizations to deal with unintended consequences? I think that's a real good one because it's not always easy and straightforward in sunshine. But there might be some unintended consequences when you work with data and AI. So how do you prepare them?
Bill Schmarzo:Great question. Number one, there will always, be unintended consequences, so we start by understanding that they're gonna happen. It's, unavoidable. What we can do is to basically start to unleash that collaborative organization to start brainstorming all the things that could happen both from the positive and negative side. What are the unintended consequences of this business initiative failing, and what are the ramifications and employees get laid off. We have to close offices. It impacts communities. There are a range of variables and metrics we can measure against the things around when initiative fails, but what happens when initiative is successful? Are there second and third or ramifications if an initiative's successful, how does that impact your employees, your customers, the community you're in? Are there environmental impacts? Are there ethical impacts? What I found is that when you bring people together, leverage from the design thinking kind of concepts to start ideation where all ideas are worthy of consideration. Where you're allowed to put all kinds of things On my blank wall here with Post-it notes, maybe 90% of the stuff you put up on the wall here, maybe ninety-five percent of this I put on the wall here has no relevance. But the 5% might be critical because if we can imagine all the ways that it can go wrong, we can start to identify the KPIs and metrics to monitor for that. And if we can do that, we can put those KPIs and metrics into our models to try to mitigate the unintended consequences. It takes a lot of work, and it scares me because we have leaders who are very quick to make policy decisions without going through the process. Thinking of the second, third, and fourth order ramifications as citizens of data science, we cannot allow that to happen. We cannot allow AI models to be built. That have a negative impact on people, and we need to think through all the ways this thing can go wrong before we put it into production.
Andreas Welsch:That's very powerful. That's speaks to my engineering mindset as well, really taking it apart and looking at it from all different angles and understanding, like I said, what could go wrong. And to me, there's a strong connection to what you said earlier, right? Ethics being a foundational component as well, not just an afterthought, but really from the beginning. So I see a strong connection there as well, should we even build this, right?
Bill Schmarzo:What's, interesting is you can actually use ChatGPT helping to explore where do all the potential unintended consequences. It'll give you all kinds of answers. Some of'em are nonsense. It's okay, I'm a human. I can throw the nonsense ones out. But if you have this conversation and keep asking, gimme more. Gimme more. Think about it from the perspective of customers. Think about it from the perspective of community. Think about our perspective from environmental, and it'll start generating all these things. You know what? Great starting point. I still doesn't replace the fact that I wanna bring a diverse set of stakeholders into a room. Stakeholders may not even like each other. I don't care. In fact, if they don't like each other, that's better. And let them brainstorm all the things and argue and go all go all crazy here. That's okay. We want to capture all of that so that we come into this world of AI with our eyes wide open, not Pollyannish.
Andreas Welsch:Perfect. I just want to let that sink in for a second. And there's one question from Naz in the chat here. The last one where she's asking about data privacy and Generative AI. I think that's an important one that we see come up time and again that I also see in conversations when I talk to business leaders. What about data privacy? Am I just sending that off to some vendor? Is it really protected? Or what do I need to do? And I think that the same is even true for AI, for machine learning, for other means that they will all subsume under AI. But, thinking about data privacy, what are some of the thoughts or recommendations you have there when it comes to data and AI literacy? What do business people need to know about the aspect of AI and data privacy?
Bill Schmarzo:So I think you should always assume bad intentions. Always start off by assuming bad intentions, right? Because the cost of being wrong and assuming good intentions and things going bad can be so expensive. So come in as a doubting Thomas. Come in and say I'm not convinced in any way that my data is gonna be protected. All the terms and agreements you get from websites about how we're gonna protect your data. I don't believe it. For example, the idea of me giving my, DNA information to 23AndMe, no way. No way. Because I'm just gonna assume bad in all cases. And so I am gonna do everything in my power to make sure my data's protected. I usually, for example, have a tab over my camera here. Because I don't want anybody watching what I'm doing and collecting that. I usually stub out the speaker on my thing so that people can't hear me talking. I use anonymous browsing. I don't trust anyone. And even good organizations who have all the right intentions, who are most ethical and honorable can get hacked. And so your data's gonna get out there. Just assume your data's gonna get out there. And I guess when you think about it from that perspective that you know your data's gonna get out there, apply the mom rule, right? What would your mom think if you put that data out there, if you said, Hey mom, I just told everybody about blah, blah, blah, would your mom be like, that was a smart move, or that was, what are you an idiot? So, we have to be cautious. We have to be, again, embrace critical thinking to think about what are all the ways that my personal data can be used against me? And then realize that your data will be used against you. Some organizations use it in a positive sense or recommend you might want this these products show to buy, or these are the places of vacation, or whatever you might wanna do, right? Most companies are gonna use it for really good intentions. It only takes one or two bad actors to have your bad experience. That data gets misused and put out there. Data privacy is a big, a huge issue. I love what GDPR has done. I think it's a step in the right direction. I think it needs to go 10 more steps.
Andreas Welsch:That's a statement. Usually we hear the opposite, right? GDPR complicates everything and it's just a big rule book and everything. But I love how you raise awareness to be conscious and to be data conscious. And be conscious of all the breadcrumbs that we all leave. Sumi is saying in the chat I think I love the mom role too. I might incorporate that, too. That's awesome. But I'm wondering then building off all of that that, we just captured over the last couple of minutes, what's one action that AI leaders should take to increase the literacy in their organization? What's the number one thing that they should do?
Bill Schmarzo:Awareness. Awareness is number one. If we wanna have an impact, we need to make sure everybody is aware of how their data's being collected. And how that data's being used to influence or manipulate them. That, to me is step one when I am presenting in a few weeks to a bunch of juniors and seniors at a local school, a local high school, and that's gonna be point number one, is that awareness. You need to be aware that everything that you're doing, walking down the street, typing on your phone, working on the internet everything you do. Is being collected, and you need to be aware that there are people who are gonna use that data to try to influence you, manipulate you, what you buy, and even what you believe. So awareness is number one. If you're not aware, you've got no chance. And once you have awareness, now we have a platform to say, okay, now what's the next level? How do I protect myself? How do I protect my customer's data? How do I ensure that I'm using my customer's data to deliver more relevant, more meaningful, more responsible, and more ethical outcomes? But it all starts with awareness and reading my book.
Andreas Welsch:What is it called, so people can find it?
Bill Schmarzo:Yeah, it's, I published it with Packt. You can find it on Amazon, so they can collect all your information about you shopping for my book on Amazon. It's called AI and Data Literacy, empowering Citizens of Data Science, and I want to think about that last phrase, right? Empowering. It means that you understand that that you're a citizen. And citizen means that you have a proactive role. You need to be active in it. You can't sit back and wait for crap to happen. I can't wait for the EU or the White House to come up with an AI act that's gonna protect me as a citizen. I need to be proactive and I need to understand my roles. My responsibility and my rights as a citizen. My role is to understand how do I ensure my data's being used in an ethical manner, right? How do I get involved? Number two, my responsibility is to bring others into this process. It can't just be about me. I have responsibility for my society, for my community, for others around me. And number three my right, to transparency. And what I mean by that, my right to know when an AI model has been used to make a decision that impacts me. What were the variables and metrics in that model, the rationale, so to speak, that drove that decision, roles, responsibilities, and rights.
Andreas Welsch:Thank you for capturing that. I see one question in the chat again from Dan, and maybe if we can answer that briefly because we're getting close to the end of the show. Dan is asking, can you really get transparency into everything that's being done with your data? And maybe where or how can you get that transparency? If there's something that's more actionable?
Bill Schmarzo:Yeah. Maybe we can't get total transparency, but we can certainly start marching down that path. We can put rules in place that says that when I when a system and AI model makes a decision about me. That it pops up and says, Hey, an AI model made this decision, whether it's on Amazon browsing or on social media. When I get some random thing in my feed it was generated. This was put here by an AI model, and I should be able to click on that thing and says, oh, and we made this recommendation to you based on the following variables. How hard can it be?
Andreas Welsch:Question is, do businesses want to be so transparent?
Bill Schmarzo:God no.
Andreas Welsch:And for what reason? Exactly. Now that's awesome. I didn't expect our conversation to go more in that direction of data and data privacy and, data awareness. But I think it's super important. And as I said it's the first step to data and AI literacy which is also the name of your book. Now, Bill, I was wondering if you can summarize the three key takeaways for our audience today, because we're getting close to the end of the show.
Bill Schmarzo:Number one, do not be afraid of AI. It's only a tool and it will only do what you train it to do. Number two, be involved, right? Be involved in how those models are being trained so you understand, you know how your data's being used and how it might be there to manipulate you. And number three, understand your rights for transparency. Demand them. Don't think someone else is gonna solve that problem for you. It's on you. Demand transparency.
Andreas Welsch:Thank you so much for joining us and for sharing your expertise with us. Really appreciate having you on. Was great.
Bill Schmarzo:Thanks, Andreas, a fun conversation. Like I said, I didn't know it was gonna go this direction, but that's the way it went, so it's always more fun that way.
Andreas Welsch:Exactly.