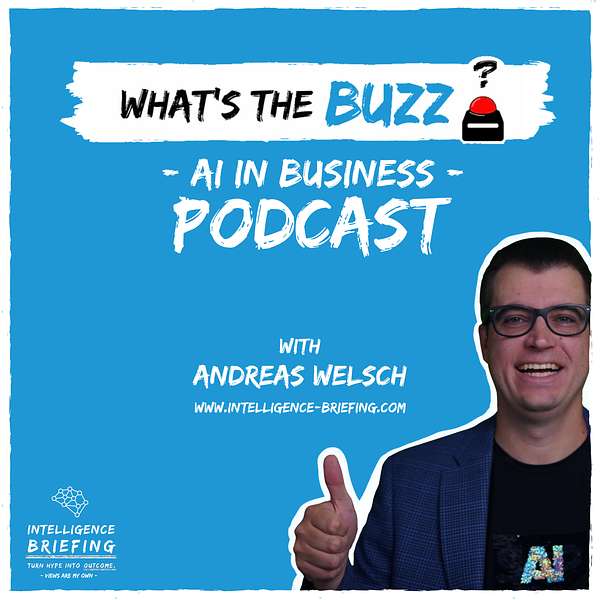
What’s the BUZZ? — AI in Business
“What’s the 𝘽𝙐𝙕𝙕?” is a bi-weekly live format where leaders and hands-on practitioners in the field of artificial intelligence, generative AI, and automation share their insights and experiences on how they have successfully turned hype into outcome.
Each episode features a different guest who shares their journey in implementing AI and automation in business. From overcoming challenges to seeing real results, our guests provide valuable insights and practical advice for those looking to leverage the power of AI, generative AI, and process automation.
Whether you're just starting out or looking to take your efforts to the next level, “What’s the 𝘽𝙐𝙕𝙕?” is the perfect resource for staying up-to-date on the latest trends and best practices in the world of AI and automation.
What’s the BUZZ? — AI in Business
Drive Sustainability And Energy Efficiency With AI Leadership (Guest: Philippe Rambach)
In this episode, Philippe Rambach (Chief AI Officer, Schneider Electric) and Andreas Welsch discuss driving sustainability and energy efficiency with AI leadership. Philippe shares (their story/journey/insights/perspective/learnings/examples) on (specific aspect of topic) and provides valuable (advice/tips/insights) for listeners looking to (achieve specific outcome/learn more about topic).
Key topics:
- Get support for AI projects within your company
- Prioritize AI scenarios based on business value and momentum-building
- Anticipate future expected energy use for AI and its impact
- Seize opportunities for AI in manufacturing
Listen to the full episode to hear how you can:
- Start from business value instead of the AI technology
- AI can optimize energy consumption and lower carbon emissions depending upon its use case
- Select AI technology based on cost and emissions
- Machine Learning remains highly relevant for processing structured data and forecasting
Watch this episode on YouTube:
https://youtu.be/i5lwGFvYU_c
Questions or suggestions? Send me a Text Message.
***********
Disclaimer: Views are the participants’ own and do not represent those of any participant’s past, present, or future employers. Participation in this event is independent of any potential business relationship (past, present, or future) between the participants or between their employers.
Level up your AI Leadership game with the AI Leadership Handbook:
https://www.aileadershiphandbook.com
More details:
https://www.intelligence-briefing.com
All episodes:
https://www.intelligence-briefing.com/podcast
Get a weekly thought-provoking post in your inbox:
https://www.intelligence-briefing.com/newsletter
Today we'll talk about how you can drive sustainability and energy efficiency with AI leadership. And who better to talk about it than someone who does just that, Philippe Rambach. Hey Philippe, thank you so much for joining.
Philippe Rambach:Hi Andreas, nice to be there.
Andreas Welsch:Wonderful. Hey, why don't you tell our audience a little bit about yourself, who you are, and what you do.
Philippe Rambach:Sure. As you can hear from the way I speak English, I'm French. I'm based in Paris, and I'm actually the first chief AI officer of Schneider Electric. And I hope that I will make a job good enough that there will be a second one after me. A few words about Schneider, because your audience may not know us. So Schneider Electric, we are a large multinational company, more or less 35 billion euro revenue. around 150, 000 employees all over the world. And basically what we do is we provide solutions to our customers for energy management, mostly electricity, and industrial automation. And our aim is to help them to be more sustainable by being more efficient by leveraging our solutions, products, and software.
Andreas Welsch:Awesome. Thank you so much for sharing. Folks, for those of you in the audience, if you're just joining the stream, drop a comment in the chat where you're joining us from. I'm always curious to see how global our audience is, especially today. Philippe, should we play a little game to kick things off? What do you think?
Philippe Rambach:Sure. Let's see what the game is.
Andreas Welsch:Perfect. This game is called In Your Own Words. And when I hit the buzzer, the wheels will start spinning. When they stop, you'll see a sentence. And I'd like you to answer with the first thing that comes to mind and why. In your own words. To make it a little more interesting, you only have 60 seconds for your answer. And for those of you watching this live, drop your answer in the chat and why as well. Are you ready for what's the buzz?
Philippe Rambach:Yeah.
Andreas Welsch:Excellent. Then, here we go. If AI were a song, what would it be? 60 seconds on the clock, go.
Philippe Rambach:I don't know why two things come to my mind I'm almost like in the proofs questionnaire, like in automatic answering. On one side, I don't know why the final countdown come to me. And I really think that's not the case, I don't think AI bring us to doom. But some people say it. The first thing that came to my mind was the final countdown. And then the second thing that came to my mind was love me tender. I don't know why, honestly. Because you, at some point, when you are in AI, you start to like it. It's something which is adorable, where you can do a lot of nice things, and so on.
Andreas Welsch:Yeah, no, no problem. You never know. Maybe it's the final countdown to finally get started. Exactly right. And then to fall in love with it.
Philippe Rambach:Awesome. I love some of the comments in the chat, much better than mine.
Andreas Welsch:Hey, thank you so much for, playing this game with me and, for playing along. That's, awesome. But now to the more serious part of our conversation that we talked about. Like I said in our preparation, I've been following your content for, I think, at least the last two years. And I know you were one of the chief AI officers out there. And you've been championing that vision of an AI leader who brings others along on the journey. So I'm really curious to hear from you. How have you been engaging Schneider Electric as a company on AI in your CAIO role?
Philippe Rambach:First, I would say that how you engage a company, especially in a large company like ours, is definitely the critical part. It is not super easy to build a team of data scientists. It is not super easy to build a platform to run your models. It is not extremely easy to choose your use cases, but none of that is useful if you don't bring your company with you. If you stay an isolated AI innovation team, then you deliver nothing. So your question is really the critical point and the way we try to address it and had some success in it, is first to be obsessed by the idea of AI at scale, which means that from the day one, our obsession was to do AI that impacts, to do AI that help us be more efficient, help us serve our customers better, help our customers, and we'll dive into that later, help our customers use their energy more efficiently, really do AI that impacts. And not fall in the trap of, wow, it's a beautiful technology. Let's play with it and do a shiny object, but really make sure that we use it at scale and always be focused on that. Practically speaking, what does that mean? That mean that you never start with a technology. You always start from the use case, from the business need, and you never start from. Hey, what can gen AI do for me? What can I do with gen AI? You start by, what are my business problems? Where am I not good in serving my customers? And from this business issue, some can be solved using AI, some cannot. The one that can be solved using AI, then this is a use case, and then you start to develop these use cases. The second thing to bring the company in is how you engage with the line of businesses, the functions in your company. which are not part of your AI core team. What we were thinking in Schneider is if we really want to bring value and really impact, really deliver AI at scale as I was saying, you need to combine domain knowledge with AI knowledge. AI alone will not make it, and domain alone of course will not bring AI, that's obvious. You need to merge the two. Our choice in Schneider was to develop what we call the Hub and Spoke model. Hub and spoke means everything and nothing. Everybody will use these words. In our case, it means that we centrally have the core, core team of AI, data scientists, experts, and so on. And when we do use case, the function, the line of business brings all the rest. Domain knowledge, go to markets, sales, marketing, technical integrations, the software and so on. So that was also very important to bring the people in to have this merging using Agile methodology, merging in a squad domain knowledge with with AI expertise. And to some extent one of the best reward I have is we have this quarterly meeting where we present to our CEO a few use cases that we have delivered in the previous quarter. And we do that. With this counterpart of the domain, the function can be HR, finance, supply chain, or our line of businesses would deliver products to our customers. And when we share to our CEO, they speak most of the time. They explain the value of what we have done, what it brings, how it changes the life of the customers. And then we spend two minutes talking about what kind of neural networks we have leveraged or whatever. And for me, that's a proof of the pudding. That has become their babies. They are pushing us to deliver to them. And that's really what's working well. So in short, start with the business, find a way in your company to merge domain knowledge with AI knowledge, and make sure that the one who has a business need is the real owner of the, solution being developed.
Andreas Welsch:Awesome. Thank you so much for sharing. I think for, many, that's the ideal model and the ideal end state that they want to get to, right? But I'm sure it wasn't, that simple getting. Getting there, I assume, right? At least if I listen to others and see what they share and what they say, right? It's, hey, there's this technology. My board is asking me, what are we doing with generative AI? What's our AI strategy? Or we, need to, first of all, chase the shiny object. So we have something that we can build momentum around. And then you get to the business value question and you find out maybe it's not necessarily there or it's more expensive to run it with AI than to do it yourself and whatever else. So it's, super, super exciting for me to hear how you've established this.
Philippe Rambach:Rebounding on what you said, and maybe that was a clever choice of our CEO when he appointed me Chief AI Officer. In many companies that I see, the chief AI officer is a guy with a lot of knowledge in AI, who has spent 20 years or two or three theses on AI, and that can be a good choice in many cases. In our case, he chose to take somebody like me, who had not that much AI knowledge, but a lot of business experience and a lot of company experience. And the idea was to say, if we want to do AI that really impacts, we need somebody who understands our business to choose the right use case. And we need somebody who understands the company very well to find a model that works, an organizational model that works for Schneider. And I still see a lot of companies choosing somebody with super difficulties in AI. Sometimes it may fit the needs, but sometimes it may not.
Andreas Welsch:Yeah. And then to your point, then you missed that, that link and that understanding of the business and with the business. Absolutely. That makes a lot of sense. Awesome. Now, folks, if you're in the audience and you have a question for Philipp, please don't be shy, put it in the chat. We'll take a look in a couple of minutes right after the next question. Look we, set up this, this, session today. I think four weeks in advance or almost two, two months in advance, if I'm not mistaken, and we said, hey let's, talk about energy, let's talk about sustainability, and just in these last two weeks, three weeks, probably, there, there was so much news in, the industry about Energy consumption of LLMs being so high, being increasing. Some companies are thinking about, their own chips for, energy efficiency, or even their, own energy sources. So there's a lot of, Discussion happening in the industry and personally I'm expecting it'll only get more when we look at things like sustainability But I'm wondering with all of these things coming more to the forefront of AI deployments Where do you expect the energy use for AI will go? And what do you think will have the biggest impact from what you are seeing?
Philippe Rambach:First, I would say that, as you say if you read the newspapers if you listen to the news people, most of the time when they think AI and sustainability they will think it in what I would call a negative way. AI comsumes energy, adds to the problem. Actually, we in Schneider believe that it'll help the de carbonation, it'll support the fight against climate change. And I will explain why. First thing I would say, and would not be surprised being working in AI, I would start with facts and data. As of today, the total consumption of data centers of energy is, I think, something like 0.2% of the worldwide energy. Out of this 0.2%, AI in that is maybe 10% of it. So we may be at 0.02% of the energy of the world used for AI today. And even with the biggest forecast of generative AI, done by seller of data centers, we may go up to 0.04%. All of that to say that if we're able to save only 5 or 10% of the remaining 99.96% by using this 0.04% of energy, we are really on the safe side. And now we find more precise on how we see it. In Schneider, we see AI playing a very key role in the decarbonation and the fight against climate change in three ways. The first one is, and maybe I should start by something even more important, really a belief that we have. We believe that the access to energy is almost a basic human right. Without energy, you cannot develop, you cannot grow. At the same time, we need to find a way to provide energy without damaging too much the planet. So that's a kind of the dilemma. And we believe that AI can really help, not do everything of course, but really help in solving this dilemma. The first thing where AI can really help is basically using less energy to provide the same outcome. If you want to optimize a process to use less energy, and the process can be an industrial process to manufacture glass. It can be a process to heat or cool a building. It can be a process to manage a data center. It can be any kind of process. If you want to optimize it to get the same outcome with less energy, you need a model, because you need a way to simulate, optimize, test hypotheses. The best model, most of the cases, is the physical model. But in many cases, it's extremely difficult to build a physical model. AI can help you, leveraging data, build a model at a much cheaper cost than building a physical model, it's not even possible. A lot of the energy of the world is wasted in heating and cooling buildings in a stupid way. Not stupid, just basic way on off. We are releasing on the market a room controller, which will, thanks to AI, learn the thermal behavior of the room and therefore be able to optimize the way we heat and cool and reduce by 20 or 30 percent the energy used to heat or cool buildings without touching the the comfort. Same for factories and so on. So the first thing is even if you burn all your gas to get your energy, fundamentally, if you use less energy for the same outcome, naturally, you generate less carbon and you generate less pollution and so on. So that's the first pillar. The second pillar where we think AI can play a strong role, and that is a bit more complicated, so stay with me, is if you really want to decarbonize, the only energy that can be fully decarbonized is electricity. However, electricity, if you produce it by burning oil or burning gas, you're back to square one, and you actually have a heavily decarbonated energy. Most people, when they think about moving to green electricity, and they are right, by many extent, think about the production side of the equation. So they think about how can we put more solar panels, more wind, more water generation, and that's all good and great. They often underestimate the demand side of the equation. And there is a lot of work on which we work on optimizing the demand side of the equation. Because if you are able to optimize the demand side, then you can reduce, as an example, your request for energy or electricity at the time of peak of demand on the electrical grid. And when there is a peak of demand, the only solution for the utility to satisfy the demand is to start gas powered power plants and therefore produce carbon heavy electricity. I will give you an example of something that we sell to customers today, which is available. Let's say you are a big data center or a big university, you want to be more green so you have both solar panels, maybe some wind generation, maybe some batteries in the basement. Already if you use what is produced when the sun is shining or the wind is blowing it's great. But you can do probably much better because if you are able to forecast your production, forecast your consumption, forecast or get from the grid the information about the quality of the power, and the percentage of carbon,. Then you can make, we recommend to do that every 15 minutes, a choice between should I use what is coming from my solar panels or should I sell it to the grid because I will help the grid decarbonize, or should I store it in my battery for tomorrow because I will need it tomorrow? Or you see where I'm going... We do that at building levels, home levels, etc. That is exactly what I'm talking about, the microgrid example. So that's the second pillar, which is using AI to optimize the demand. That can be also using AI to optimize how you charge a fleet of vehicles to make it the clever way. Plenty of applications, not all by Schneider, of course, but a key role of AI to optimize the demand. And the third one is great to have solutions to reduce your energy needs for the same outcome. Great to have solutions to optimize your demand to move towards green electricity. But if you really want to impact the planet, you also need to scale them. If you install them only in five buildings or ten factories, you did not move the needle. So the last place where we see AI playing a very strong role is getting away some of the difficulties of scaling. I will give you a very concrete and basic example. California state is pushing very hard to install solar panels in the roof of almost every single home. What is slowing the deployment is the first step you have. You need an electrician to come to your home, open your electrical panel and check if your panel, if your home, can accept solar panels or not. There is not enough electricians in California. This is slowing down the program. We have developed an AI tool for one of our company playing in that field where as an individual homeowner, you take a picture of your panel and the AI tells you, yes, you're ready, or no, you're not ready. Three pillars, and I stop here, that AI can really help in this fight against climate change. Reducing the amount of energy needed for a given process, optimizing the demand to help move to green electricity, and also removing the barriers to adoption to help scale.
Andreas Welsch:Thank you so much for sharing. I really like how you're thinking about it in these three different aspects. And what you mentioned as well, right? A lot of the discussion focuses on there are carbon emissions created from training these models, from using these models. And I think too little is the conversation also about what is the amount of carbon that we're taking out? As a result of it, because we're doing things faster, more, more efficient and so on.
Philippe Rambach:And maybe, by the way, on that topic, and then we can move, but we wanted to check if we are not wrong. We've done a bit of checks on very precise use cases, looking at what we call carbon return on investment. So how much carbon do you generate by running the model? That is how much you save. The ratios are between 1:1,000 and 1:500. Okay. So we are still at the stage where we can say much more than generate.
Andreas Welsch:That's a very powerful message. Awesome. Now what you mentioned, the use case, taking pictures of your panel. How do you identify these opportunities? I think there was a question here from somebody in the chat. How are you finding value with AI or gen AI? And to me, that question is how do you find that the good use case that delivers business value and how do you build that roadmap?
Philippe Rambach:We start from good problems. So we really don't start from, hey, who can use my AI? We really start where the team dedicated to that experts, people which are product ownership capabilities. They sit with our functions, HR, supply chain, or our line of businesses, like the one doing the microgrid offer I was mentioning. And they don't speak about AI first. They first start by speaking about what are your business problems? What do you want to achieve? What are your customers requesting? And finding together where AI can help. And we always start from the business need. Our finding in Schneider was we cannot just wait for business to come to us, the AI team. So we have a team of people who make sure that we maintain an AI roadmap for each of our functions and AI roadmap for each of our businesses. And that's how we do it. And what we look at is really when we look at a use case, we look mostly at two things. What is the business value? And what is the technical feasibility? And then during the development of the project, we always check the two and we stop projects either because technically, unfortunately, we cannot do them, often by lack of data, or because the business is not anymore sure of how to bring it to the market.
Andreas Welsch:Now, how do you manage that expectation and if you're investing in a project and it turns out that technologically it's not feasible or hey, we thought there's more business value that we can actually generate than what we're finding out as we're going along. How do you manage that expectation towards your leadership, for example, that hey, there are some projects that will turn out great and they deliver business value? Otherwise, it's maybe more of an exploration and until we then can say with certainty this will become part of a product or no, this is not.
Philippe Rambach:The issue is less the manager than the team. It's much more difficult to convince the squad to stop a use case. That is very difficult, because they always think that, yeah, around the corner they're going to make it. So actually the management, I explained to them when we started that probably, if we are very good, two out of three use cases will go to the end, and most likely maybe one out of three, that our job is not to bring the three of them, but to stop them as early as we can. And that is pretty well accepted by the management. Actually, the difficulty is more with the team who never want to drop a project. They work hard on it. They love it. Actually, it's more difficult, again, to convince the teams to stop it.
Andreas Welsch:That sounds familiar. I remember being in that position as well when we had to make tough choices on do we continue or not. Exactly. Everybody was hoping just, one more one more tweak, a couple more days. No, it makes sense. But that brings up or brings back other memories, right? Now, generative AI is certainly the latest hype and clearly for the last, what, 18 months, 20 months
Restream recording May 28, 2024 • 04:00:45 PM:it's
Andreas Welsch:seems like the industry hasn't been talking about anything else other than that. But we also know that it's not always just about generating, summarizing information. There's some dependencies based on the industry and sometimes you might just have bigger success and big opportunities with traditional machine learning and structured data. You gave a couple examples around the greater micro grids and these kind of things. So where do you see the opportunity in your industry between gen AI, machine learning, and what are those opportunities?
Philippe Rambach:The first thing I would say, it's super important to be very clear with everybody, because it's unclear for people, not specialists of the domain, generative AI does not replace AI. Generative AI adds new possibilities to what we were doing before with AI, but it does not replace AI. And by the way, for many of the tasks where we as an industry need AI, AI is not the answer. Many things we do is about forecasting, optimizing, detecting anomalies, Generative AI is not super good at that. And even if it could do it, it would be very expensive in terms of carbon, energy, and money. So the first thing I would say is, use gen AI only when it makes sense. And if you can avoid using gen AI, that's great. And by the way, what I say also, if you can avoid using AI, that's better also. If you can find ways to do things without AI, it's usually better than AI. Then some stuff can be done only with AI, and some others only with generative AI. First thing is, yeah, it does not replace AI, and, even sometimes it could. I was seeing papers saying, hey, we are so happy now with generative AI, we can even do time series. Yes, but at which cost of carbon? At which cost of energy? For what additional benefit to a normal functional technique? It's very doubtful. If I take Schneider, gen AI is 20% of the use cases we do. 80% is still more traditional AI. If anything like traditional AI exists, I'm not sure that's true, but other techniques of AI, I would say. For me, where I see gen AI for a company like us, at least in the next 18 months, because forecasting the future in AI is a very dangerous game, but it's a lot about knowledge management, RAG technology helping customer care centers, helping customers find information. We all still spend a lot of time or even lose a lot of time trying to find the right information. And here, generative AI for a company like ours can play a very strong role. And then, of course, software development. sales support, marketing content generation, all of that will be strongly impacted by generative AI and bring you a lot, of productivity.
Andreas Welsch:Awesome. Sounds like there is still a lot more opportunity, but also that a lot of times it comes back to structured data, forecasting and in these types of things. Just by the nature of the industry and what you're working on. Now, that's awesome. Philippe, we're coming up close to the end of the show, and I was wondering if you can summarize the three key takeaways for our audience today.
Philippe Rambach:What would I say? I would probably say always start from the business need, never start from the technique. And everybody says it, but it's so easy to forget that. Every morning you should come to your office and read the paper, reading, start from the business, not from the technique. People will, the technique is so fascinating that it's super easy to fall into, Ah, I can build something fun. Start from the business. Second thing, I hope that you are now all convinced that you should leverage AI for sustainability, either by using our solutions or developing yours. But there's a lot of progress we can do leveraging AI for sustainability. And probably the last one is, don't be obsessed by the newest technology. It's not because gen AI is a new one that you should use gen AI for everything. Always stay from what is my business need? What is the cheapest, fastest way to solve my business needs? Gen AI can be, but really not always.
Andreas Welsch:Wonderful. Thank you so much for sharing. That's a message that I hear from many consistently as well. Make sure you understand the business problem and you select the right technology. I think it's super important to hear that again and from as many leaders as possible. So thank you for sharing that. Also Philippe, thank you so much for joining us and for sharing your expertise with us. I really appreciate it. Enjoyed our conversation today and I hope you in the audience did the same.
Philippe Rambach:It was a pleasure for me. Hope it was useful for the people who listened to us.
Andreas Welsch:Yeah, awesome. All right.
Philippe Rambach:Thanks. Thank you. Bye bye.