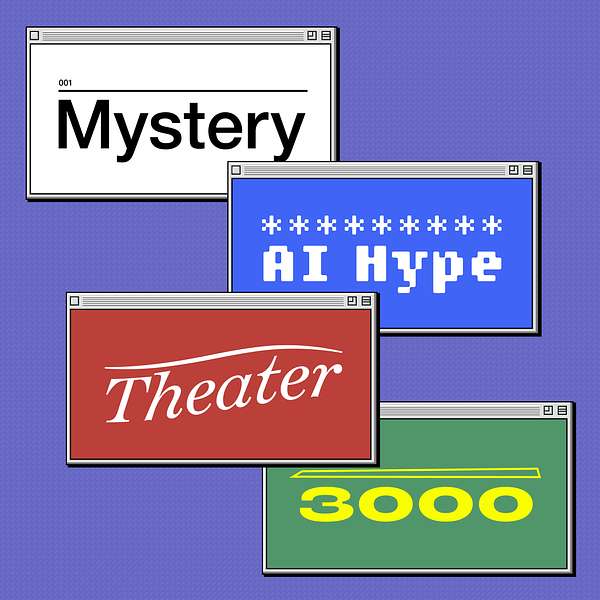
Mystery AI Hype Theater 3000
Mystery AI Hype Theater 3000
Episode 16: Med-PaLM or Facepalm? A Second Opinion On LLMs In Healthcare (feat. Roxana Daneshjou), August 28, 2023
Alex and Emily are taking another stab at Google and other companies' aspirations to be part of the healthcare system - this time with the expertise of Stanford incoming assistant professor of dermatology and biomedical data science Roxana Daneshjou. A look at the gap between medical licensing examination questions and real life, and the inherently two-tiered system that might emerge if LLMs are brought into the diagnostic process.
References:
Google blog post describing Med-PaLM
Nature: Large language models encode clinical knowledge
MedRXiv: Beyond the hype: large language models propagate race-based medicine (Omiye, Daneshjou, et al)
Fresh AI hell:
Fake summaries of fake reviews
https://bsky.app/profile/hypervisible.bsky.social/post/3k4wouet3pg2u
School administrators asking ChatGPT which books they have to remove from school libraries, given Iowa’s book ban
Mason City Globe Gazette: “Each of these texts was reviewed using AI software to determine if it contains a depiction of a sex act. Based on this review, there are 19 texts that will be removed from our 7-12 school library collections and stored in the Administrative Center while we await further guidance or clarity.”
Loquacity and Visible Emotion: ChatGPT as a Policy Advisor
Written by authors at the Bank of Italy
AI generated school bus routes get students home at 10pm
Lethal AI generated mushroom-hunting books
You can check out future livestreams at https://twitch.tv/DAIR_Institute.
Subscribe to our newsletter via Buttondown.
Follow us!
Emily
- Twitter: https://twitter.com/EmilyMBender
- Mastodon: https://dair-community.social/@EmilyMBender
- Bluesky: https://bsky.app/profile/emilymbender.bsky.social
Alex
- Twitter: https://twitter.com/@alexhanna
- Mastodon: https://dair-community.social/@alex
- Bluesky: https://bsky.app/profile/alexhanna.bsky.social
Music by Toby Menon.
Artwork by Naomi Pleasure-Park.
Production by Christie Taylor.
ALEX HANNA: Welcome everyone to Mystery AI Hype Theater 3000, where we seek catharsis in this age of AI hype. We find the worst of it and pop it with the sharpest needles we can find.
EMILY M. BENDER: Along the way we learned to always read the footnotes and each time we think we've reached peak AI hype, the summit of Bullshit Mountain, we discover there's worse to come. I'm Emily M. Bender, a professor of linguistics at the University of Washington.
ALEX HANNA: And I'm Alex Hanna, director of research for the Distributed AI Research Institute. This is episode 16, which we're recording on August 28th of 2023. And we're here to talk about AI and medicine. This isn't our first time doing this but the interest in "slapping an LLM on it" in healthcare settings has only intensified since the last time we tore this premise apart. And we're going to talk in particular about Google's large language model for healthcare, Med-PaLM.
EMILY M. BENDER: With us today is Dr. Roxana Daneshjou, an incoming assistant professor of biomedical data science and dermatology at Stanford University. She's also a clinical practitioner at Stanford Healthcare and Stanford Medicine Children's Health. Dr. Daneshjou did her postdoctoral work with Dr. James Zou, working on artificial intelligence for healthcare. Her current research focuses on the performance of new technologies in clinical settings, including machine learning and generative AI. We are so excited to have you here, welcome Roxana.
ROXANA DANESHJOU: Thank you so much for having me.
EMILY M. BENDER: And before we get started, I have to show off my hat. So people can see this.
ALEX HANNA: Yes!
EMILY M. BENDER: This is the birthday hat. Why am I wearing the birthday hat? It's not my birthday.
ALEX HANNA: It's the podcast birthday!
EMILY M. BENDER: It's the podcast birthday. We've been doing this for a year.
ALEX HANNA: Oh my gosh, that's wild. Oh happy happy birthday Virgo baby, we're glad you're in this world.
Um yeah I mean who could have thought, you know one article and then after another it just became a habit.
EMILY M. BENDER: This became a habit, yep. And I love this comment--I love our our commenters--and we have Abstract Tesseract here saying, "More like Face-PaLM, am I right?" Hey definitely what we're going to be seeing with Med-PaLM. So speaking of that should I transition us and share the main courses for today?
ALEX HANNA: Yeah let's do it.
EMILY M. BENDER: Okay so. Here we are. We are starting in um with the Med-PaLM, or Face-PaLM as Abstract Tesseract has it, um blog post from Google um and do we have a date on this? When was this?
ALEX HANNA: Was this the first--this is Med-PaLM 2, right? So this one I think--
EMILY M. BENDER: Is there no date on this? That's kind of bad.
ALEX HANNA: Well yeah, I think it was in July, I mean it's July or August because I think they released this maybe co--I want to say they released this pretty soon after the next thing we're going to discuss, which is the Nature article, which was the original Med-PaLM which they published um on July 12th, I think, mid-July. Yeah.
EMILY M. BENDER: All right so I'm gonna read the couple--first couple paragraphs here to get us started. "Med-PaLM is a large language model (LLM) designed to provide high quality answers to medical questions."
Um okay so we're already off on a bad foot here because large language models provide synthetic text, they're synthetic text extruding machines, not question answering machines. "Med- PaLM harnesses the power of Google's large language models, which we have aligned to the medical domain and evaluated using medical exams, medical research, and consumer queries."
All right I'm gonna hold back because I want to hear you all's critique of that, though I have things to say.
"Our first version of Med-PaLM, pre-printed in late 2022 and published in Nature in July 2023, was the first AI system to surpass the pass mark on U.S. Medical Licensing Exam-style questions. Med-PaLM also generates accurate, helpful long-form answers to consumer health questions as judged by panels of physicians and users."
So, what do we think?
ROXANA DANESHJOU: I just want to thank uh the technology companies for helping make a point that I've been trying to make for years, um so in the House of Medicine, the U.S. Medical License Exam scores actually--so the first there's there's three step exams. Step one, step two, step three.
And step one scores, for the longest time until it became recently pass/fail again, were used as a gatekeeper for what specialties you could apply to. Which was deeply ironic, because when the exam was first designed it was really designed as sort of a pass/fail exam, like your basic medical knowledge but not to be a gatekeeper. And so for years, like even before you know any of these models came out, I've been saying, 'Hey these exams, we know from previous study, do not represent the ability to practice medicine.' They're they represent the ability to answer tests--this is for humans of course right, um we can delve into all the issues of using like exam type questions to try to you know evaluate LLMs.
But it even even before you bring LLMs into the mix like--this has been something I think it's been hard for people to grasp, that it's not the same. There are definitely people who get it but it was used as a gatekeeper, and and studies have shown that these exam scores didn't actually correlate with clinical skills, ability to practice medicine, and they were very gatekeepy, essentially. But now that large language models can quote "answer these questions," people are realizing, oh yeah this is definitely not the practice of medicine. Because it's not.
So--
ALEX HANNA: Yeah.
ROXANA DANESHJOU: --for that one positive thing that has come out of that.
ALEX HANNA: One question I have for you Roxana is that they say this this surpasses the pass mark uh on the exam. And I want to know--I mean holding in our mind that both the gatekeeping function of these exams but also um one of the things that they know is that this is the I--in the longer text from the pre-print, they said this is the commonly quoted past pass mark.
Um which is--so I mean it's it seems like--I'm I'm curious what what kind of evaluation metric this is even, if they're sort of using it as an ad hoc or without if there's not kind of agreement within a particular domain.
Yeah so I'm curious on your your thoughts on that.
ROXANA DANESHJOU: I mean I think I mean first of all the exam scoring, I don't remember the exact mechanics of it but they're I think there is some curve to it, which is why the pass mark has changed. There's definitely been drift in the exam scores as people are scoring quote "higher." Um it's now pass/fail again, I think uh thankfully but I mean yeah I I don't know--I don't know what it means to say that an LLM can quote "pass" some exam especially when we don't know anything--I mean there's a lot of material on USMLE-style questions online. There's a lot of it. There's no way to know how much trained tests leak there is because as we know companies don't release what they train their data on.
ALEX HANNA: Right.
ROXANA DANESHJOU: There's no way to know that they could literally be seeing you know the same very very very similar questions. And of course these questions are written to be very clear-cut.
ALEX HANNA: That's right.
ROXANA DANESHJOU: And medicine is [ laughter ] never--I mean I wish it were but it it's not clear-cut, there's so much uncertainty, there's so much you know gathering information over time.
Um people don't come in a nice little package like you know, "25 year old presents with this kind of pain and the exam result--you know the exam results are perfectly aligned with this diagnosis, and the lab results are also surprisingly perfectly aligned with this diagnosis." That's really not how the world works. And that's how the exam question world works.
EMILY M. BENDER: Yeah so we're back to the lack of construct validity here, right? So you--we can talk about how useful it is to ask people these questions, you know certainly not in a specialty gatekeeping kind of a way, but like what is the function of this exam when people take it? But that's an entirely separate question of what's the function of it for this kind of work. And if you look in the longer paper as we'll get to they start talking about it as a benchmark. And it's like this wasn't developed to be a benchmark for anything machine learning and I kept being astonished by talking about passing uh "surpass the pass mark on USMLE-style questions." So it's not actually the exam right?
ALEX HANNA: Right.
EMILY M. BENDER: They're things in the same style. And they do talk in the Nature paper a little bit--they look to see if there was overlap between their training data and the particular questions. And we should go look at it but they said "25-word sliding windows." So they were looking for like verbatim the same 25 words in a row, which doesn't seem like a very thorough check to me for overlap. Right, you could have something that was you know--had a synonym in there, things in a slightly different order and then it--I don't think it would have been flagged as having been in the training data, but even aside from that it's like this doesn't tell us anything interesting about the large language model because that's not what these--that's not what the actual exam is designed for, let alone questions in the style of that exam.
Um all right there's one other thing in this first paragraph that I wanted to highlight, which is um they talk about this being "aligned" to the medical domain--
ALEX HANNA: Right.
EMILY M. BENDER: --um and because of the way the word 'alignment' gets used in the AI safety discourse that's a huge red flag for me. And it's throughout these papers.
ALEX HANNA: They they go into that a bit more too in the in the pre-print, where they basically say um--and this is again a continual thing that we come back to--uh but they say "the longer sentences--"
Um and this is more about their methodological contribution, "however human evaluation um uh revealed that further work was needed--" And this is also admitting one of the the weaknesses, um, "further work was needed to ensure the AI output, including long-form answers to open-ended questions, are safe and aligned with human values and expectations, and the safety-critical domain."
And then the parentheses, "a process generally referred to as alignment." Uh I was a big sociology conference this past weekend the, American Sociological Association, and I was telling someone that works in demography um, which is kind of the study of the study of many things but many of them are about health disparities, um kind of birth rates and death rates, modeling, doing that kind of modeling. I had told them about alignment, and they kind of--their head started to spin and they're like, 'This is a thing they're saying? That you can go ahead and try to have a machine align with a unified set of values?'
I mean we can go on and on about alignment but it makes me very um--it's just kind of wild that this that this thing about kind of what's considered, you know, expectations of in in safety-critical domains, has one sort of a set of agreement um and within within particular different different uh you know different kinds of professional associations or rather in a discipline and those disciplines and kind of convening their professional associations is a contested field, for one and like is contested as things change and people change, um and the second is that um uh uh that that this is that it could be I mean in the fact that these kind of crystallize into these institutions, so yeah the fact that alignment is here and like using this is is just it's it's just really--they're they're wrapping it into a particular sort of agenda.
EMILY M. BENDER: Yeah. Right. There's something I need to lift up from the comments here because it's hilarious.
Abstract Tesseract says, "I feel by this logic if I took the answer key to an exam, cut out individual sentences and tossed those clippings into a fishbowl, that fishbowl would also be qualified to practice medicine." And then LSchultz82 says, "And it'd be three days until that fishbowl is hired to evaluate insurance claims data."
ROXANA DANESHJOU: Oh gosh. That's the--can I make a comment on--
EMILY M. BENDER: Please.
ROXANA DANESHJOU: --insurance claims? To me, this is the most frightening terrifying thing that we need to be discussing because it's already happening. Right because people have been saying, 'Hey, we can use these models--' You know first of all I want to acknowledge that doctors are overburdened by paperwork, and our system is strained, and I recognize that we need solutions to help us. And people have been using models to write, for example, um appeals to insurance denial letters, and they look them over and make sure and of course there's automation bias. And and I can understand because doing those appeals is a huge pain, um really structurally we need to think about um policies that regulate this better, because insurance companies are just denying things all the time, um inappropriately.
Uh but the flip thing has been happening, where you know it's come out maybe not large language models but definitely AI algorithms are being used by insurance companies, and there was a great report out in STAT about this, about a company that was using an algorithm to deny care to patients, deny coverage of care.
And no human was getting involved, patients didn't even know this was being used to you know deny them care, and so to me that's that's terrifying um that this is kind of already happening um and you know, I don't think that--I don't think those companies worry about sort of accuracy of the models and how much harm is being caused when they use them to make these decisions.
And and I think you know we all have to kind of speak up on this because it's already happening, there's no--I mean there's no regulation there, it's already happening.
EMILY M. BENDER: Yeah yeah and when we talk to regulators and ask for transparency, right you said patients didn't even know this was happening, they should know, and recourse, right?
ROXANA DANESHJOU: Right, when this happens.
EMILY M. BENDER: Yeah, those are totally key and I'm I'm terrified right along with you. All right we're only in the introduction here, I've got some things highlighted but Roxana is there something that you would like to take us to in this document, um to particularly rant on?
ROXANA DANESHJOU: Let's scroll down, let's scroll down, let's see, introduction.
EMILY M. BENDER: And this graph design is hilarious to me.
ROXANA DANESHJOU: Yeah.
ALEX HANNA: Oh that's great.
ROXANA DANESHJOU: We're--just to describe we're seeing a graph of performance of other models, um on this exam and then we see the Med-paLM and Med-PaLM 2. And it's just like a huge bar graph that's so much better performance.
ALEX HANNA: It's just incredible, this-- this is like a--this is like a Fox News-style graph where the X-axis makes no sense because PubMed-GPT is December '22 and so is Med-PaLM 2, and so they separated it out. It's uh it's uh approximate medical pass mark, uh and it kind of grows itself.
Um it's unfortunately just like very um very phallic, uh I'm just gonna say, uh because it just like grows from Med-PaLM 1 to Med-PaLM 2 and it makes the sort of growth from like 50 percent, uh which should be the kind of natural midpoint of this, it just makes it seem much greater so if you're yeah if you're at a like in a car listening to this, like check this out when it goes home. It's pretty ridiculous.
EMILY M. BENDER: All right-- RD: I think--oh yeah I was going to say talking about quality, how do you evaluate answer quality? Because I think you know anytime you have human raters on something you have the um subjectivity of the human, and the biases of the human, how do you grade the quality of a medical answer? Like how do you do that?
EMILY M. BENDER: Yeah so here they're just doing the multiple choicing, but later they get into this and they've got their weird axes that we should get to. But before we go past it, I want to dog on a couple things here. So they say uh, "Letting generative AI move beyond the limit--limited pattern spotting of earlier AIs--" Earlier mathy maths. "--and into the creation of novel expressions of content, from speech to scientific modeling."
Uh so "novel expressions of content" is synthetic media and that is the last thing that I want in the practice of medicine, right, I don't want random stuff extruded from these machines um. And
the scientific modeling like--yes, data science in lots of scientific fields is a real thing, it can be quite useful. Scientific modeling is a thing but uh not LLMs. Like that's not scientific modeling.
[ Laughter ]
So anyway I had to dog on that.
ALEX HANNA: Yeah yeah, no. I mean the kind of novel expressions of content--um yeah like the making up of citations.
EMILY M. BENDER: Yeah all right and then below their their sample USMLE-style question, they say uh, "Answering the question accurately requires the reader to understand symptoms, examine findings from a patient's tests, perform complex reasoning about the likely diagnosis and ultimately pick the right answer for what disease, test, or treatment is most appropriate."
Um so that is maybe what the test is trying to evoke in human test takers, but that's not what the LLMs are doing here. Right? Answering this question for an LLM requires extruding text that matches one of the multiple choice inputs, period.
Right, this is a huge misrepresentation. All right but then um now now um Roxana was talking about the way they evaluate the long-form answers, and there's some more confusing graphs here.
Um so they talk about "high quality answer traits" and "potential answer risks." And these graphs are uh horizontal bar graphs that have something that look like error bars in them that I don't fully understand, and then it's like on the left there's some gold which represents Med-PaLM 2, the middle is gray and it's labeled "tie," and the right is blue and it's labeled "physician." And these all add up to 100, so the idea is that apparently Med-PaLM 2's answers and the physician's answers were rated on these same criteria and the question is which one was rated higher?
Um.
ALEX HANNA: Yeah, it's such a weird thing, it's--why would you present data this way, I was just puzzling about this.
EMILY M. BENDER: Yeah.
ALEX HANNA: Uh you know in there kind of in their defense in the paper--although this is sort of the marketing copy that they publi--that they you know no one from the tech press is really going to dig into the paper. But then they do have um these evaluations where that looked more like a standard sort of um evaluation, where it has an error bar and has a point estimate and basically if the summation in the paper is effectively for if you're looking at physician raters and you're rating this--all these different things which we'll go into in a bit, which I think I really want to go into, especially these kind of potential answer risks one--the physicians are effectively um equivalent uh for all the first high quality answer traits, but the physicians do a significantly better um--or rather the the places where there is um the biggest delta is in this place that says no inaccurate--"more inaccurate or irrelevant information" in which Med-PaLM 2 gives a lot more crap basically compared to physicians.
Um however physicians give more--omit more information in that kind of rating.
Um and nearly tie on "more evidence of demographic bias." So this is this is--first off I got a lot to say. [ Laughter ] because the way that rating or kind of content analysis happens in in computer science, this drives me up the wall. I mean the kind of things that computer scientists often think that they can have human raters evaluate with some kind of exactitude, as Roxana was was was basically saying, is is wild. And that they are doing it in such a way for a particular sort of practice or a particular domain, um you know that you can--what does it mean to sort of say this answer is supported by consensus? Or what does it mean that this is possible harm extent? And uh and what does it mean to you know what--how is this necessarily play, like what kind of validity does this have internally to the field of medicine um and what kind of validity that this has does this have for um for the kind of evaluation that clinicians do?
And so the panels themselves are constructed uh of--they have this expert physician panel that um is somewhat limited, it was pretty small from what I from what I saw. Um so they had--and and then they had um people on, I'm assuming they're Mechanical Turk workers or some sort of crowdsourced workers that they use, because they are located all in India and the physicians are all either in the US or the UK.
Um so okay hold on I misspoke, so the physician raters were pulled from 15 individuals [ unintelligible ], six based in the US, four based in the UK, and five based in India.
Um and then the layperson raters were six raters uh all based in India. Um my assumption is what they did given this is that they effectively put this kind of rating on the same platform, um probably a crowdsource platform, asked if anybody had kind of a medical sort of background, and then allow them to do the tasks. Given that how unspecific that they're talking about them.
Um but they don't really talk about kind of what other things, what other kind of knowledge bases these people are going like thinking through, um what kind of other biases they may have, um and you know don't really talk about the kind of testing and piloting that you really need to do any kind of quality rating work.
Um so yeah this this sort of thing is just like it's just it's just so I--I had to get I had to get into the pre-print to see it I had to see what was going on because I knew it was going to just annoy me uh to heck and back.
ROXANA DANESHJOU: Yeah yeah I just can I can I inject some like you know experience from real medicine?
EMILY M. BENDER: Please.
ALEX HANNA: Yeah.
ROXANA DANESHJOU: You know I think I think when you're in the space, you kind of begin to understand some things. Like you were saying very very sparse on the details of who the raters were. So I am a practicing dermatologist. If you ask me to rate questions that have to do with--and I'm board certified in dermatology and I did one year of internal medicine--but if you asked me to rate questions that have to do with like a cardiology problem, I am not going to know what the latest and greatest is in cardiology.
I'm just not, because you know medicine is a very specialized domain and so who your rater is and what their experiences does matter. The second thing is, a lot of medicine, I mean sorry to say this but it's just true, is not fully evidence-based. It's it's just it's when you go into training you sort of learn how things are done at your training institution, and you see cases like--for example I trained on the West coast I saw very little Lyme disease because that's not something that's prevalent over here.
But not only that, there are differences in what medications people will go for, even between Stanford and UCSF. We manage--there can be differences in what we think--how we think a uh skin disease should be managed because we--each institution has their own world expert in that disease and there's not you know good randomized control trial data and so we're basically going off of expert opinion here.
And so then you even have variation in the same regional area between two major academic centers in how a disease is approached, never mind between like the US and India and the UK, and I've sometimes even had colleagues who are dermatologists from other countries like ask my opinion on the drugs that they have on formulary are different, they have similar mechanisms but they're different drugs than what we use. And so who is to say like what the consensus is, right, and what what is it right and you know what is the right answer now or what information being omitted is right or wrong?
Now I mean there are things that are obviously flagrantly wrong um and so if I saw something like that uh that would be a problem, but um you know and then with regards to demographic bias, I would say that many physicians don't even understand or know their own biases and and so I it would be it's hard to say like how would you how would you rate that if you don't even know sort of your own biases or if you're not--haven't specifically had training in what medical bias looks like?
ALEX HANNA: Yeah just on the specialty that they say in the paper, "Specialty expertise spanned family medicine and general practice, internal medicine, cardiology, respiratory--" Nothing else, just respiratory. "--pediatrics, and surgery." And that seems like a remarkable spread to me, I mean it's kind of I'd imagine if if you want to turn this to computer scientists on its head you'd say, well you know we got we got six computer scientists, you know one from architecture one from uh formal um uh uh what's it called formal analysis, uh another from programming languages, and one from machine learning and we you know we're just--we asked them if this description of a machine learning architecture was correct, you know? It was it I mean you're all computer scientists right?
EMILY M. BENDER: Yeah. All right there's one more thing I want to do in this and I think I should take us over to the Nature thing and then the Politico one is short but we're going to have I think a lot of angst to release about it. And the thing that I want to bring us down to in this one is they talk about the sort of future vision of multimodal um processing, so: "Extending Med-PaLM 2 beyond language: The practice of medicine is inherently multimodal and incorporates information from images, electronic health records, sensors, wearables, genomics, and more."
And I'm like--the practice of medicine is inherently face to face and embodied and like the sort of you know interaction between the physician and the patient is super duper important, and that's not what they mean by multimodal, so there was that. Um, but they talk about um uh, "We believe AI systems that leverage these data at scale using self-supervised learning with careful consideration of privacy, safety and health equity will be the foundation of the next generation of medical AI systems that scale world-class healthcare to everyone." So once again it's like you know people who we have failed to provide adequate health care for, we're going to fob them off on these text synthesis machines and media synthesis machines.
Um but they talk about creating this vision language model, um a multimodal version of Med-PaLM. "This system can synthesize and communicate information from images like chest X-rays, mammograms and more to help doctors provide better patient care. Within scope are several modalities alongside language: dermatology, retina, radiology (3D and 2D), pathology, health records, and genomics." Which is a weirdly diverse list of things.
"We're excited to explore how this technology can benefit clinicians in the future." And then they give this example where they've got a picture of an X-ray, just went away on the screen, "Can you write me a report analyzing this chest X-ray?" and then outputs findings, blah blah blah.
Footnote: "Example only. This image reflects early exploration of Med-PaLM 2's future capabilities. So they are not exploring future capabilities because that is unknown. You can't just go explore the future like that. It's advertising, it's hype, it's fake.
ALEX HANNA: Yeah there's just so many parts of this that just has alarms going off. Again things that no one's really asking for, so I mean the sensors, the wearables, the genomics, I mean all these things are you know--two things kind of um just ring all my alarms, um kind of the biases that are in here in for instance electronical electronic health records. I think I was um uh there's a paper I either--well I guess I can't say that I was a reviewer for it so I can't mention it but I know um so ignore you heard that--but I won't well you can hear but I'm not going to say anything else about that.
But there are biases effectively in all these aspects, I mean sensors um the inability uh to to "see" Black skin um on wearables, uh genomics as as as being attributing genomic kind of elements to race.
Um and then the kind of pri--you know they say, "With the key for--careful consideration of privacy..." Well I mean you know I feel like these attentions to health and equity are given um a certain amount of lip service but I mean if--unless they really go out and they are doing these kinds of studies and showing--I mean you haven't been able to show Med-PaLM in practice um on a clinician setting, and what that would mean for health equity, and already you're going to integrate all this other data, EHRs, sensors, et cetera. I'm pretty wary of that just from the jump.
ROXANA DANESHJOU: Yeah I mean I think they've actually put out a preprint in this space right, and yeah--I would say another--so so they've put out a preprint which we're not going to probably go into discussion here, but I'd say two two things here that you know based on our discussion: How do we evaluate this?
And I you know have had the opportunity to be in rooms where there are people who are involved in regulation and things like that. And I say, you know with models where there's some diagnostic prediction and there's a straightforward answer you can start to build frameworks where you look at you know its accuracy, its sensitivity, its specificity, and you can you know have a way of building or thinking about building a true evaluation framework. Um there's still obviously a lot of problems in that space too with fairness, generalization, and so on and so forth. I don't know how you build--like like to our previous discussion when we're talking about people grading these long form answers, I don't understand how you build a framework that actually evaluates whether or not something is truly working, and what metrics you use to look at being able to monitor harm.
And I think that's a huge problem that we're sort of building all these things but we have--not only we have no framework for truly evaluating them set in place or agreed upon, but two, that you know you're doing deployments without having frameworks to evaluate or that you're doing deployments at all is obviously to me terrifying. But um and then I would say the second thing I wanted to mention is this really this this sentence about "the foundation of the next generation of medical AI systems that scale world-class healthcare to everyone." Um that to me is is very hype--I believe that you know the senior people who work at these tech companies would never accept such a system being used on them for making medical diagnoses, right.
ALEX HANNA: Oh they've said as much.
ROXANA DANESHJOU: They've said that, they've said it. Right?
ALEX HANNA: Shout out to VP of Google Health Greg Corrado, who said he did not want to have Med-PaLM be part of his family's medical journey. I think we talked about on a prior episode of the pod.
ROXANA DANESHJOU: And so and so that is hugely problematic to me, because it's you know there's so much money and resources that go into building these models that could actually go into actually build you know thinking about how to build a fair health care system, improving access for everybody. Technology is not this magical panacea that's going to fix everything, um and we have to actually do the work and really what you're setting up here is not a world-class system for everyone, it's almost like a two-tiered system--which we live in a two-tiered system already. I acknowledge that.
But there's going to be no effort put in to actually building systems for public health and improving health care if your belief system is that we'll just build this technology solution that we'll you know use on the on on on people who who can't afford access, and I think that's going to cause a lot of real harm because you have these systems that are not actually truly validated and and also it's taking away res--like it's taking away people--the hype is kind of taking sucking the air out of the room of doing things that are maybe is not as glamorous but actually from a public health perspective have real impact on improving health.
EMILY M. BENDER: Yeah absolutely. And early in this blog post they talk about Google Health's annual health event, The Check Up, and I am so not reassured that there's an organization within Google called Google Health and that they run an annual public event like that just seems like so many misdirected resources.
ALEX HANNA: Oh there there is there there is an institution called Google Health within Google and they are highly problematic. Um they are I think I saw somebody on Twitter say something that they're not much more than the marketing firm for kind of their own kinds of technologies and not really concerned about things around equity. And Abstract Tesseract said in the chat, "They talk a big game about equity but there sure is a noticeable absence of the voices of the people who are most impacted by this BS and literally any understanding of the systems and communities though--those people are a part of."
And that's 100 percent true. I mean just the kind of getting into it. I do want to say one thing just about and and this is this is uh you know kind of a more of a mention to anyone who's out there who wants to really pursue a certain kind of research agenda, which would be around machine learning and unspecified evaluation uh and construct validity. I mean we've talked about this kind of consistent thing that we've talked about. And there are folks who focus on it a lot uh Deb Raji, uh shout out to Deb and and her work on evaluation. Um shout out to um uh to uh uh Abby Jacobs and Hannah Wallach and their paper on on measurement and fairness.
But there's a whole kind of agenda around that so if you're an enterprising PhD student or someone in the chat--I see you in the chat Ruth, I know you have a bunch of students--there's a whole agenda around the ill specification of evaluation in machine learning and AI. And I would say one way you could even study this is to consider um do we--looking at who is making these benchmarks and who's making these evaluation benchmarks and then who is checking against them.
Because probably not going to be a surprise, my hypothesis would be the people making the benchmarks are the ones the only ones using the--actually using them for evaluation. Um and so you already have this kind of thing of this kind of this elite--eliteness coming to evaluations um and yet no one's using your evaluations.
EMILY M. BENDER: Yeah. All right. We don't have very much time left and I really want to get to this like just terrifying thing that came out in Politico and hand the mic to Roxana.
So the headline here is, "A health care AI pact that matters." It's a short little article from Politico um Roxana would you like to read and react here?
ROXANA DANESHJOU: Sure, um: "Microsoft is teaming up with Epic Systems, the healthcare software company, to integrate generative AI tools which scour data to generate answers to questions or create other content into Epic's electronic health record system."
So I just want to--I'll read a little bit more but Epic is like they have the monopoly and--
ALEX HANNA: Yeah.
ROXANA DANESHJOU: --and the hold on the electronic medical--like that's what many many physicians use. That means there's a good chance artificial intelligence is coming soon to your doctor's office, if it's not already there.
"The companies originally announced the partnership in April. Now they're providing more details on what they have planned: AI-assisted doctors note summarization and text suggestion, transcriptions of doctor-patient conversations, AI-powered coding and billing procedures, generative AI exploration for filling gaps in clinical evidence--" I don't know what that last one means but--
ALEX HANNA: No.
ROXANA DANESHJOU: Oh yeah, why--why it matters. Epic's market reach in the US is huge, sorry Alex to cut you off.
ALEX HANNA: No no, go I'm just I'm just live reacting with terror.
ROXANA DANESHJOU: Yeah. So here is the thing about medicine. I will tell you that I have spoken to many colleagues, some of them incredibly senior in data science, and you know developing systems and the thing that has been most shocking and I think this is going back to hype coming before science, is in medicine we usually don't move fast and break things. And I understand in some cases, things move slower than people want. You do want to get innovation out to your patients, that's important--it's important I mean I I work in this space, this is my research space.
So I I believe that innovation can make our healthcare system better. I want to think about how we can do that in a safe, fair, appropriate, accurate way, but taking a large language model that again we have no framework for evaluating its accuracy and appropriateness, we have no framework in place for evaluating its biases, and many patients--in many cases patients are not are not even going to be made aware that this is being used on them, even though the uh you know AI Bill of Rights claims that you know there should be patient knowledge of the use of any AI system--I mean all of these things seem so antithetical to how health care has really integrated technology, and I don't I don't--I to be hon--to be frank I don't understand what's happening.
I don't know I--I think because um you know these LLMs have been sort of sold as like magic, which we know they're not, but to a lot of physicians um it seems magical, all of a sudden it's gotten pushed into systems and we don't--you know these are conversations we have here at Stanford and elsewhere--we don't what are our frameworks for knowing that this is working?
How do we know this is not harming patients, how do we know that it's not uh acting in a biased way? Especially when you're talking about things like filling gaps in clinical evidence and that we know that the models will just generate things that are absolutely untrue, with references that don't exist. And so I just don't understand why we are not taking a step back and saying, hey listen maybe there are some small narrow appropriate use cases for these models, but to even identify those you need to have evaluation metrics and testing testing testing testing not on just benchmarks but sort of uh you know testing in ways that really reflect the real world use case, and monitoring systems in place and all these sort of things to be able to you know to be able to do that.
And none of that's in place. So I'm just uh--every day I'm just sort of baff--I would say baff--I am baffled.
ALEX HANNA: Yeah okay--oh go ahead Emily.
EMILY M. BENDER: So the the like you said the gener--generative AI exploration for filling gaps in clinical evidence, you can't--that is not a case where you want something that just makes shit up, right, and that's what it is designed to do. So you're absolutely right that we don't have systems in place to evaluate these things and at the same time we have good a priori reasons to believe they are a bad match for this need.
And I've done some work looking at what would have to be true for an application to be a good one for a synthetic media generation machine. And one of the things is that the people involved would have to be able to thoroughly and efficiently check that it is accurate, right? What doctor is going to have time to babysit the output of one of these machines?
ALEX HANNA: Right.
ROXANA DANESHJOU: My favorite thing to show when I talk about this is um that news headline where it's like uh, a Taurus has driven into the uh into the--a body of water again for the second time in Hawaii because it's--they're following the GPS systems, and it's sort of like I say like that's what will happen happen with automation bias.
Your brain has come to trust the GPS system so much that you are not using with your eye--you know what your eyes are telling you which is hey you're about to drive straight into a body of water. Like this is like the perfect example of what automation bias--I mean if you ask anyone hey do you think you would drive into a body of water, they're of course they're going to say no. So if you ask a doctor do you think you would take a bad output from a large language model and send it to a patient, of course they're going to say no. But then we have real world evidence that that's not how--I mean what could be more obvious than like hey don't drive straight into a body of water?
But because you're told by a technology system that you have come to trust and believe, it overrides your own basic reasoning.
ALEX HANNA: Yeah and just I mean a few things on this um before I move on to Hell, uh but but the but the kinds of just the I want to note a few things here uh just in in one one thing I want to note--this is our Wisconsin connection here because Epic is headquartered in Verona, Wisconsin so it is um uh outside of Madison. Uh our producer uh this is where our producer Christie Taylor and I I met, um but also kind of noting that we know a lot of people that work at Epic.
Um and it's kind of fascinating the kind of way that this this or this organization has taken over, I mean there's lots of ways to show that Epic is a pretty bad place to work, that it is also a place where um has been--had a very conflictual um relationship with um with uh with the surrounding uh neighbors, not surprising, it's really like little little um Silicon Valley in Madison, I mean it's kind of the biggest the biggest tech employer in Madison. Uh on their press--on their presser though they they write one of the initial solutions is already underway uh with UC San Diego Health, UW Health in Madison, Wisconsin and Stanford Health Care as the first organizations to deploy--starting to employ enhancements to automatically draft message responses. And so I mean there's the thing that you rightly bring up Roxana, the automation bias happening, and in this in this that we see on screen right now, things like AI powered coding and billing procedures, things like note summarization and text suggestion, these things are going to quickly become very much relied upon, especially in clinician settings when you're very taxed to--you know going from one appointment to another.
Um and where is going to be the place to actually intervene and actually see this to happen?
There's been some good work in places--other places evaluating different kinds of tools, so shout out here to uh Mark Sendak and Madeline Elish who have a paper um called um, "The human body is a black box." And it's about kind of the organizational constraints of using, not an LLM, but a tool called Sepsis Watch um in the Duke Hospital System to assess whether the patient might be at risk of developing sepsis.
But the organizational--organizational settings basically thought that you know nurses could effectively challenge doctors, or uh and and make them change an assessment or just something of that nature. Um we first off need people to intervene at the point of where the text is extruded, um in these doctor's notes or in these coding and billing procedures, but we don't have that.
We don't even have a test test--a manner of doing that. So you're just going to find more and more errors being introduced and effectively no way to audit or um or or or intervene on that. And that for me is really frightening. Um again a free idea if you are a dissertator, uh but see if you can communicate with one of these places at UC San Diego or UW Health or Stanford and say, hey can I just hang out and see what these things are doing? Can I can I look at your coding and billing procedures?
I don't think they're going to say no but um but we do need those texts and organizational checks and balances.
ROXANA DANESHJOU: I just want to say one word on fairness because it's something I care about a lot um and it was brought up in the Nature paper for Google and I think it's a huge issue, shout out to um MD uh and Masters actually has his MD and Masters, now post-doc uh Dr. Tufunmi Omiye um who uh helped lead the preprint that we put out looking at how these different models actually uh perpetuate and regurgitate race-based tropes that are incorrect. For example if you ask them how to calculate EGFR, which is a measure of kidney function, we now know that it's complete--I mean race is a social construct, it's completely incorrect to use that in the calculation of that and some of these models not only not only say that you should use it uh in the calculation, but will say racist tropes that are incorrect which say oh yeah black people have more muscle mass and that's why you know--like that's that's not true.
ALEX HANNA: Dear God.
ROXANA DANESHJOU: Um and the terrifying thing is that a lot of physicians have uh have biases as well and so one comment we got from someone was, well I didn't know we aren't supposed to use you know race-based EGFR. Well it's like okay that's a huge problem, so if you don't actually know and the model tells you this, and you're inclined to believe the model, we are going down a very bad road.
EMILY M. BENDER: This is dark, this is dark. It is it sounds like the road to Fresh AI Hell. So as I queue up the Fresh AI Hell, Alex you your prompt this time is you are Wayne Brady on Whose Line Is It Anyway and you have been told that you have to sing a song, and I can't do a style of music so style of music up to you, um about a patient who is suffering nightmares of being in Fresh AI Hell.
ALEX HANNA: Oh wow, that that--there's layers to that. Let me think about it. Um um I guess I I will like do this in the style of like Tom Jones uh, you know the sort of, "It's not a unusual..." um let me think. "It's not unusual to be stuck in AI Hell. It's not unusual to be doing neither swell. If you find yourself engulfed in flames, just remember you can--" I I can't think of anything that rhymes with flames, that's all that's all I got, I'm sorry you got, you just put this right on me.
ROXANA DANESHJOU: So you know you're uh--"there'll be denial of your insurance claims."
EMILY M. BENDER: Oh yes, perfect.
ROXANA DANESHJOU: I can't sing so I'm not gonna try to sing that for you.
ALEX HANNA: "there'll be denial of your insurance claims." There we go, all right. I changed key.
EMILY M. BENDER: I love it, I love the collaboration. Ok, we have rapid fire Fresh AI Hell, um first one here from Engadget: "Amazon begins rolling out AI-generated review summaries." So the person--I think I got this on Blue Sky--the comment was, "Great, there's going to be fake summaries of fake reviews now."
ALEX HANNA: Oh just absolute chef's kiss of trash.
EMILY M. BENDER: Yeah.
ROXANA DANESHJOU: I can't wait to read the summaries of you know those products that have like parody you know reviews like--
ALEX HANNA: Yeah.
ROXANA DANESHJOU: --like amazing parody reviews? Like that would uh I think I would love to read those reviews, that would be really entertaining.
EMILY M. BENDER: Yeah.
ROXANA DANESHJOU: That's the only value I can think of it.
EMILY M. BENDER: Nice. Okay, "School district uses ChatGPT to help remove library books: Faced with new legislation, Iowa's Mason City Community School District asked ChatGPT if certain books contain a description or a depiction of a sex act." Um so there's this new statewide law in Iowa about what has to be taken out of school libraries and I gather that this may have actually been a protest on the part of the school district? That basically said this is a ridiculous thing to ask us to do so we're going to do it ridiculously.
ALEX HANNA: I think--yeah because I think basically they needed some kind of--if I recall this correctly, I don't know if it says it in this piece but they needed some kind of a legal--like a legally defensible way or find some kind of authority that they could cite that it had violated some kind of law. Um so so you know facially, book bans, terrible, um um and you know again this as being a reaction to rising right-wing fascism and in the US and beyond and you know these things that are these bans against queer trans uh content, um so I mean if this is being used in a way to subvert these laws I mean you know more power to 'em. You know if you could if you could prompt engineer ChatGPT to say that a book doesn't contain quote sex acts then hey, use it.
EMILY M. BENDER: So Abstract Tesseract says, "It's pretty on brand that the only kind of compliance that ChatGPT can be used for is malicious compliance." Which is--
ALEX HANNA: Yeah.
EMILY M. BENDER: -great, especially if that's what's actually going on here. Okay um, sorry, accept all cookies. Um uh this is a couple of authors from the Bank of Italy? "Loquacity and visible emotion: ChatGPT as a policy advisor. Abstract: ChatGPT, a software seeking to simulate human conversational abilities, is attracting increasing attention." And I have to say it is so hard for me to keep reading anything when the first line in the abstract has "increasing attention" or "increasing success." Doesn't make your work interesting. Okay. "It is sometimes portrayed as a groundbreaking productivity aid, including for creative work. In this paper we run an experiment to assess its potential in complex writing tasks.
We asked the software to compose a policy brief for the board of the Bank of Italy." And they find that it can accelerate workflows. It's like no no, if your work is actually meaningful you do not want to start with ChatGPT output.
Just--
ALEX HANNA: I'm wondering I mean it was written I mean Italy was--did ban it for a certain amount of time um because under GDPR--but uh this was put out this month so I don't know if it was an effort to--I don't know, I don't wanna--I don't know these authors at all but oof. Um this one is: "JCPS bus routes generated by software with flawed track record in another district." So this is an article about um so this is in the city of Louisville Kentucky.
"The entire city of Louisville was caught off guard when Jefferson County public school officials canceled classes for Thursday and Friday because of because of a transportation disaster that had some elementary students arriving home as late as 10 pm." Hey Roxana it's your example again basically--not driving into the sea but basically they generated routes um uh and buses were just kind of going all around the way for this. Uh and so basically they said they said, "At least one JCPS bus driver said the problems experienced on the first day of school are the result of a flawed software program that uses artificial intelligence to generate routes."
Um so in in in the case of instead of saying to your teacher the dog ate my homework you can say the mathy-maths took the bus off the road.
EMILY M. BENDER: Yeah. It wasn't so much off the road it's just terribly terribly inefficient. Could you imagine being an elementary school student not getting home until 10 pm? Which means that all that time you're either sitting at school waiting for the school bus, which is the less bad option, or sitting on the school bus.
ALEX HANNA: There's only so many rounds of "the wheels on the bus go round and round" that you can do without absolutely losing your mind.
ALEX HANNA: Yeah all right so Roxana this one's medical-ish so I'm going to hand it to you. This is from Mastodon.
ROXANA DANESHJOU: Oh god. There's a-- "I'm not going to link any of them here for a variety of reasons but please be aware of what is probably the deadliest AI scam I've ever heard: plant and fungi foraging guidebooks. The authors are invented, their credentials are invented, and their species IDs will kill you." Oh my goodness no. No um there is a very uh you know uh prominent toxicologist uh on Twitter, Josh Trebach, who does these like tweet you know long form tweets, where you're in a plane crash and he puts a picture of like you know some some plant and he's you know you're you're in a plane crash with a celebrity and you find this--like do you eat it or not? And it's such a fun thing that he does but uh to kind of like teach people about you know all the different poisonous ways--terrible ways that you can die from poisonous plants. And it just seems like this is that but on like in a bizarro terrifying--no absolutely not, no.
EMILY M. BENDER: Yeah and and because of the way we access things like books now, these are going to show up on Amazon as if they were real books. [ Unintelligible due to cross-talk ]
ROXANA DANESHJOU: ...with AI reviews!
ALEX HANNA: That's right, fake AI reviews oh my gosh.
EMILY M. BENDER: All right we don't have time to do all of them but there's one last one that I want to do which is a LinkedIn post. Um so Laura Jeffords Greenberg, whose tagline is, "I help legal professionals optimize their performance | General Counsel | Keynote Speaker and more." "Can you increase the productivity of ChatGPT? You can with custom instructions. I've been having fun playing around with them."
And then her example is, "How would RBG respond?"
And I sure hope that Ruth Bader Ginsburg would respond with a resounding "fuck off, don't do this, this is not speaking for me.” Um and this is--we've seen this idea before, right this came out of that--AI 21 Labs had this RBG bot and I I'm just mad to see it come back.
ROXANA DANESHJOU: There was that one that was so awful where they--wasn't it like they interviewed Harriet Tubman?
EMILY M. BENDER: Yes that was the Washington Post, too.
ALEX HANNA: We talked about that last week yeah.
ROXANA DANESHJOU: Don't do that.
ALEX HANNA: Yeah, just really resounding what the fuck. Uh also never trust anybody whose um byline in their LinkedIn is "keynote speaker." Um yeah that's not for you to decide right, you have to be invited to be a keynote right. Uh anyways--all right uh I think we gotta cut it off there.
EMILY M. BENDER: Yeah. Thank you so much Roxana. This has been wonderful.
ALEX HANNA: That's it for this week! Dr. Roxana Daneshjou is an incoming assistant professor of biomedical data science and dermatology at Stanford Medicine. Our theme song was by Toby Menon, graphic design by Naomi Pleasure-Park, production by Christie Taylor, and thanks as always to the Distributed AI Research Institute. If you like this show you can support us by rating and reviewing us on Apple Podcasts and Spotify, and by donating to DAIR at dair institute.org. That's d-a-i-r hyphen institute.org.
EMILY M. BENDER: Find us and all our past episodes on peer tube and wherever you get your podcasts. You can watch and comment on the show while it's happening live on our Twitch stream. That's twitch.tv slash DAIR underscore Institute. Again that's d-a-i-r underscore Institute. I'm Emily M Bender.
ALEX HANNA: And I'm Alex Hanna. Stay out of AI hell y'all. [ Singing ] It's not unusual...