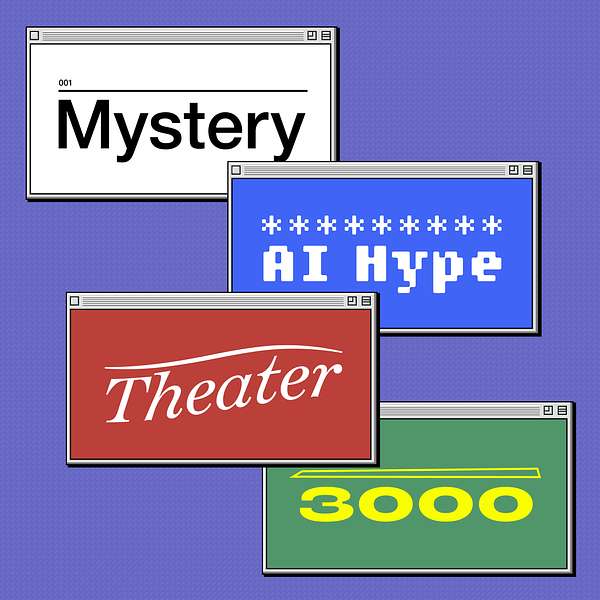
Mystery AI Hype Theater 3000
Mystery AI Hype Theater 3000
Episode 18: Rumors of Artificial General Intelligence Have Been Greatly Exaggerated, October 23 2023
Emily and Alex read through Google vice president Blaise Aguera y Arcas' recent proclamation that "artificial general intelligence is already here." Why this claim is a maze of hype and moving goalposts.
References:
Noema Magazine: "Artificial General Intelligence Is Already Here."
"AI and the Everything in the Whole Wide World Benchmark"
"Targeting the Benchmark: On Methodology and Current Natural Language Processing Research"
"Recoding Gender: Women's Changing Participation in Computing"
"The Computer Boys Take Over: Computers, Programmers, and the Politics of Technical Expertise"
"Is chess the drosophila of artificial intelligence? A social history of an algorithm"
Fresh AI Hell:
Using AI to meet "diversity goals" in modeling
AI ushering in a "post-plagiarism" era in writing
"Wildly effective and dirt cheap AI therapy."
Applying AI to "improve diagnosis for patients with rare diseases."
Using LLMs in scientific research
Health insurance company Cigna using AI to deny medical claims.
AI for your wearable-based workout
Check out future streams at on Twitch, Meanwhile, send us any AI Hell you see.
Our book, 'The AI Con,' comes out in May! Pre-order now.
Subscribe to our newsletter via Buttondown.
Follow us!
Emily
- Bluesky: emilymbender.bsky.social
- Mastodon: dair-community.social/@EmilyMBender
Alex
- Bluesky: alexhanna.bsky.social
- Mastodon: dair-community.social/@alex
- Twitter: @alexhanna
Music by Toby Menon.
Artwork by Naomi Pleasure-Park.
Production by Christie Taylor.
ALEX HANNA: Welcome everyone to Mystery AI Hype Theater 3000, where we seek catharsis in this age of AI hype. We find the worst of it and pop it with the sharpest needles we can find.
EMILY M. BENDER: Along the way we learn to always read the footnotes and each time we think we've reached peak AI hype, the summit of Bullshit Mountain, we discover there's worse to come. I'm Emily M. Bender, professor of linguistics at the University of Washington.
ALEX HANNA: And I'm Alex Hanna, director of research for the Distributed AI Research Institute. This is episode 18 which we're recording on October 23rd, 2023. And we've got another article from Blaise Aguera y Arcas to pick apart. You might remember Blaise from our first three episodes - he's a vice president over at Google and he recently wrote a piece with Stanford computer scientist Peter Norvig proclaiming that despite the many flaws of our current large language models, artificial general intelligence is already here.
EMILY M. BENDER: As you may have guessed, we disagree wholeheartedly with that claim but we won't leave it at that. Instead we're going to dig through this article and see just how they're constructing boulders to add to the mountain of bullshit that is AI hype. So with that why don't we admire this artifact. Here we go.
ALEX HANNA: I'm excited--I'm excited just to to come back you know.
EMILY M. BENDER: We're returning to our roots, Alex.
ALEX HANNA: Back to our roots. Taking down stuff that Blaise and uh Peter Norvig, who is a huge name in artificial intelligence. He with Stuart Russell uh wrote you know one of the most influential textbooks in artificial intelligence, so yeah let's just get into it.
EMILY M. BENDER: All right so paragraph one, "Artificial General Intelligence (AGI) means many different things to different people but the most important parts of it have already been achieved by the current generation of advanced AI large language models such as ChatGPT, Bard, LLaMA and Claude." All right we have a link here what does this point to Alex? Why can't I see?
ALEX HANNA: It goes to our "Sparks of Artificial General Intelligence." Not ours like but Seb Bubeck and crew's "Sparks of AGI: Early experiments with GPT-4." Which we uh addressed I think in episode eight or nine? Uh but earlier in the podcast we've addressed this before. Uh so great article to start with.
EMILY M. BENDER: Yeah that that really is is building up the credibility from sentence one.
ALEX HANNA: Sets the tone.
EMILY M. BENDER: Okay, "These--" in quotes "'frontier models' have many flaws--" And somebody was just asking the other day, do we know a good critique of sort of the settler colonialist viewpoint behind the phrase "frontier model."
ALEX HANNA: And yeah that was that was that was a question posed by Dan McQuillan who's written a book called "Resisting AI." And I know um Syed uh Ali has also kind of addressed this, uh has done some work on decolonial computing. I don't know of any but I mean this word "frontier" has lots of connotations, of course.
EMILY M. BENDER: Yeah, yeah. And I would love if anyone you know in the comments or listening later and wants to leave us a--send us a note letting us know where that's been written about. Would love to hear of it. Okay so, "These--" in quotes "'frontier models' have many flaws.
They hallucinate scholarly citations and court cases, perpetuate biases from the training data, and make simple arithmetic mistakes." Okay I'm going to be stopping us like every two words here. We've already talked about the problem with "hallucinate" but also, "they hallucinate scholarly citations and court cases" not just of their own accord, right? That happened because somebody asked ChatGPT for a court case. Or if you ask it for a citation. If you give it input like that, then it's going to come back with fabricated output.
All right. This is what made me really mad: "Fixing every flaw, including those often exhibited by humans, would involve--" Hang on [laughter] right. "Including those often exhibited by humans" is basically equating the output of these synthetic text extruding machines with what people do, but just sort of parenthetically so you can't disagree. But I disagree.
ALEX HANNA: Right right so it would and--well I mean this is an incredible sentence I mean, all the assumptions that go into it because the second part of it is, "--would involve building this artificial super intelligence, which is a whole other project." So not only is it the fact that you know um a super intelligence will fix the artificial intelligence hallucina--hallucination hallucinations but also will fix humans. Really really really great stuff here.
EMILY M. BENDER: And and we have this again the usual thing about intelligence is this linear scale, and humans sit in a certain place and look AGI has caught up to us, and anything better with the flaws fixed is further down that linear scale.
ALEX HANNA: Yeah. This--the thing we're going to we're going to get into now, and I want to say this, I want to read these next two paragraphs and then I I have a bit of a comment about the methodology here. Um so the next two paragraphs say, "Nevertheless, today's frontier models perform competently even on novel tasks they were not trained for, crossing a threshold that previous generations of AI and supervised deep learning systems never managed.
Decades from now they will be recognized as the first true examples of AGI just as the 1945 ENIAC--" or um I think this is how you pronounce the acronym, "--is now recognized as the first true general purpose electronic computer." And then the next thing is about comparing it with the differential analyzer computer. Um and to me this is--speaks a little bit to the kind of maneuvers that I think Blaise was really doing in his other article. I went back to that article recently Emily for for um for the book that we're writing, and um one of the things that I noticed is the--now that I know a lot more about the kind of historical, um the historical bases of of AI and and the Dartmouth conference and all that, and the--such an interesting thing is that the way that Blaise really like uses and abuses the history of computing. It's like someone went ahead and did a really poor reading of uh the history of computing, and then they they um and then they they said oh yeah but we can really tell these Just So Stories about technology.
And it's like it's like yeah kind of a skimming of the reading, but I don't want to I don't want to like stay there too much. Like this idea of "ENIAC is now being recognized," like I don't know if that's necessarily necessarily true. Um uh but at the same time ENIAC at one point and if there's a certain reading of even AI as being read as--was not characterized as--programming programming languages were not characterized as such, but even programming languages were considered a form of AI because the symbolic manipulation that they entailed, which is a really weird history.
And this is this is something um Jonnie Penn's dissertation, which is uh "Inventing Intelligence" has like some really interesting parts of that. So yeah this is a meta comment on just the note just how to how to tell falsehoods with history, that I want to point out.
EMILY M. BENDER: Yeah, so basically if something that Blaise Aguera y Arcas has written has the word ENIAC in it, um do a double take and go check that history.
ALEX HANNA: Yeah.
EMILY M. BENDER: Yeah. So he this says, "Today's computers far exceed ENIAC's speed, memory, reliability and ease of use, and in the same way tomorrow's frontier AI will improve on today's. But the key property of generality? It has already been achieved."
ALEX HANNA: Oh dear. All right.
EMILY M. BENDER: I'm reminded of a paper we wrote, Alex, are you?
ALEX HANNA: I am I am very aware. Yeah that that's the Grover paper.
EMILY M. BENDER: The Grover paper, yeah.
ALEX HANNA: We we come to that.
EMILY M. BENDER: Yeah. So I mean we we wrote a whole paper um led by Deb Raji, the incomparable Deb Raji--Deb Raji on um how uh the benchmarks that people have put forward have been misconstrued as um able to test something that that's actually not testable, that is the idea these things could be fully general, and it was inspired by this wonderful children's book, "Grover and the Everything in the Whole Wide World Museum." Um which I we wore the hats in one episode didn't we?
ALEX HANNA: We did wear the hats, yeah the hats the hats are are classic I think they we wrote--we wore them for the third episode?
EMILY M. BENDER: Sounds about right.
ALEX HANNA: So let's get into this, "What is General Intelligence?" Okay so first off, the audacity of posing in a no-Noema and this is in Noema Magazine. The audacity of posing in a magazine article, "What is general intelligence?" Uh already incredible. Um and so coming into it, uh you you know they ask, "Early AI systems exhibited artificial narrow intelligence, uh concentrating on a single task and sometimes performing it at near or above human level. Uh MYCIN--" which is M-Y-C-I-N, "--a program developed uh by Ted Shortliffe at Stanford in the 1970s, only diagnosed and recommended treatment for bacterial infections. SYSTRAN--" uh S-Y-S, these are all in caps, T-R-A-N, "--only did machine translation. IBM's DeepBlue only played chess."
I like I want to stop here because this kind of this is doing a lot of work already. That a lot of these a lot of these programs were considered to be a sort of general intelligence. So MYCIN, there's a there's a there's a paper by--I believe the paper um addresses this--but there's a paper by um David Ribes and a number of other people, I think Geoffrey Bowker is also on this paper, called "The Logic of Domains," and it effectively talks a lot about MYCIN and the kind of epistemology of this. Basically how you can sort of bring a computational bearing on any kind of thing, this could be kind of a general tool, and so maybe if MYCIN even only focused on medical uh treatments or um--it envisioned itself as a general purpose type of technology that did mimic general intelligence.
I want to say the same thing for IBM's Deep Blue, uh and I've I've referenced this paper multiple times on this podcast but the um the Nathan Ensmenger paper, "Is Chess Playing the Drosophila of of Artificial Intelligence?" Uh meaning that it did even though it only played chess, it quickly became a symbolic stand-in for all intelligence, and so while these things played one thing, they did a lot of historical lifting that I think this article is doing.
EMILY M. BENDER: Yeah. Although I want to I'd be really curious to look at the history of SYSTRAN because um you know my experience in the field of NLP, um and you know through the Association for Computational Linguistics, which used to be the Association for Computational Linguistics and Machine Translation, is that there's a whole myriad reasons why you might be interested in building language technology that are not at all involved with or interested in the project of AI.
So I would be not very surprised if SYSTRAN weren't marketed as AI in its time.
ALEX HANNA: Yeah.
EMILY M. BENDER: Especially because I think SYSTRAN is probably 1980s, which was a point where you didn't go around--like it didn't make something sound cooler if you called it AI in the 1980s.
ALEX HANNA: Right right. Yeah they're probably downplaying that it was AI in the 80s, right. And now we've come back historically to reclaim it as AI now that AI is hot again.
EMILY M. BENDER: Yeah all right so I need this next sentence here, "Later deep neural network models trained with supervised learning such as AlexNet and AlphaGo successfully took on a number of tasks in machine perception and judgment that had long eluded earlier heuristic rule-based or knowledge-based systems."
I have two things to say here. One is they're what they're calling AGI when they say it's already arrived is large language models. Those are also a supervised learning setup, because their training task is "predict the next word or predict a masked word and then compare that to the word that was actually in the text. That is still supervised learning. They're pretending that
it's not. And um "machine perception and judgment," um that's the bat signal for Weizenbaum, right?
ALEX HANNA: It is, it is it's completely the the Weizenbaum um perception--I mean it's it's actually writing against Weizenbaum, right?
EMILY M. BENDER: Exactly, no but so--judgment, machines don't have judgment.
ALEX HANNA: Exact--exactly, yeah. And and this is something that Weizenbaum basically says, you know like machines don't--I mean this is his point, machines don't have judgment. This is a point that's reemphasized by um Brian Cantwell Smith, uh in in uh his book which I'm completely blanking on the name right now, but the sort of idea of he actually distinguishes between judgment and what he calls "reckoning" and he says what machines do is reckoning. And I don't love Cantwell Smith's book but it it still emphasizes this judgment. Also this idea of machine perception is also attributing perception as being a thing where you notice something or you uh are getting insight.
No, AlexNet was an image classification algorithm right, it's not--
EMILY M. BENDER: AphaGo is a um game playing system.
ALEX HANNA: Game playing yeah.
EMILY M. BENDER: Yeah.
ALEX HANNA: So game playing is not is not judgment, right, it is it is game playing, right.
Um so okay so next part and they've got a whole list and this is kind of the meat of of this. So I'm going to read this uh uh kind of um--we can go peace meal or can go through it depending on um uh--
EMILY M. BENDER: How angry we get.
ALEX HANNA: How how angry we get, exactly. Okay, so number one, "Topics." So excuse me--prior, it says, "Most recently we have seen frontier models that can perform a wide variety of tasks without being explicitly trained on each one. These models have achieved artificial general intelligence in five important ways."
EMILY M. BENDER: Wait wait wait they are they are asserting this thing that they're arguing for has happened.
ALEX HANNA: Yeah yeah yeah yeah. Exactly. So, "1. Topics: Frontier models are trained on hundreds of gigabytes of text from a wide variety of internet sources, covering any topic that has been written about online. Some are also trained on large and varied collections of audio, video and other media."
Ooof. Okay so just because any topic--there's any topic that's on online, it can cover anything, it is just all knowledgeable.
EMILY M. BENDER: Yeah so so yes it's true that these things can extrude plausible sounding text on a very wide variety of topics but--
ALEX HANNA: Yeah.
EMILY M. BENDER: --that's like general mimicry which isn't necessarily worth anything.
ALEX HANNA: Yeah, and I mean also that the topics I know--I'm gonna I'm going to go back to to this on number four because I think that pairs well but um, "2, Tasks: These models can perform a wide variety of tasks, including answering questions, generating stories, summarizing, transcribing speech, translating language, explaining, making decisions, doing customer support, calling out to other services to take actions, and combining words and images." I mean this is this is wild because it has this kind of idea of a task and there's there's something that--I mean there's a paper here, free paper idea to anybody in the in the chat--but this this notion of task I think has this particular sort of epistemic status in AI, that a task itself can be bounded and well-defined enough that if you have a sufficient number of tasks that means you have a kind of thing that looks like intelligence.
Which is very questionable to begin with, uh and even the way that they anthropomorphize many of these like "explaining," okay what's an explanation? Or "making decisions," okay um what is what is the decision and what's the boundary of these decisions right? Um and who is empowering the machine to actually have meaningful input into things that require human judgment. So David Schlangen has a great paper where he distinguishes between intentional and extensional definitions of tasks.
So the intentional definition is something like translating language, which is a really vague one but let's say you know translating from Japanese to Thai, um that's a little bit more specific um. But the extensional definition is done in the data set, right here's the inputs here's the expected outputs, and then the way we evaluate. And so in a lot of these cases the these are these are very brief very vague intentional descriptions, and they just sort of let you imagine what the extensional description would be, or extensional definition would be um, and if you look at these--so a bunch of them, "answering questions, generating stories, summarizing, explaining, making decisions, customer support--" uh um those ones are all basically, take an input string and provide a likely continuation.
So it's actually all the same task just interpreted differently depending on how we come at it um. And then a few of these are in the um translation sense, so transcribing speech you can think of this translation from audio to text, translating language is you know text in one language to text in another, um "calling out to other services to take actions" I think what that is is basically extruding text that happens to look like a command to other services and then being hooked up by some external thing to other services, and then "combining words and images," um that's not even a useful task. I'm not sure what they mean by that.
ALEX HANNA: Yeah, yeah. I'm not quite sure I imagine it it's related to this next part, "Modalities." I want to call out this comment in the chat by Khorish who says, "It's not even the task, it's a digital representation of the task to get it into a computable form. Straight cheating."
Yeah, so I mean you're not even necessarily you have to--it has to be computable, it has to be legible. Um okay so moving on, "Modalities: The most popular models operate on images and text but some systems pro--also process audio and video and some are connected to robotic sensors and actuators by using modality specific tokenizers and processing raw data streams. Frontier models can in principle handle any known sensory or modal--uh motor modality."
Uh so this is effectively saying, 'Okay we could we could hook this up and it could be a robot or something of this nature.'
EMILY M. BENDER: This is a citation to the future, right? They're not pointing to actually existing technology and they're also not talking about how well it's handling it.
ALEX HANNA: Yeah. And I'm looking at the citations and it seem to just link to other transformer models to um to basically deal with audio--effectively audio effectively, I mean I don't think they really have mentioned um, "it allows it to compete--competitively subword models on large--on long context language model achieve state of the art density estimation on ImageNet and model audio from raw files." Oof. Okay.
EMILY M. BENDER: Okay--
ALEX HANNA: So...
EMILY M. BENDER: Yeah this this isn't this isn't sensory or motor modalities, it's you know digital representations of yeah you--this this is a system that can take in bytes and output bytes. Sure.
ALEX HANNA: Yeah.
EMILY M. BENDER: Like.
ALEX HANNA: Yeah.
EMILY M. BENDER: That's--
ALEX HANNA: Yeah, totally. All right, "Languages: English is over represented in the training rad--data of most systems--" Holla Bender rule. "--but large models can converse in dozens of languages and translate between them, even for language pairs that have no example translations in the in the in the training data." Is that actually true, because I know this is like uh a thing that they had um you know that Sundar Pichai was claiming with uh with with Bard or LaMDA or one of the Google ones--
EMILY M. BENDER: There was that terrible 60 Minutes thing where it basically said, 'Yes it it it learned all of Bengali without any Bengali.' It's like no it absolutely did not.
ALEX HANNA: No it had it had Bengali yeah.
EMILY M. BENDER: Yeah. I think that it's plausible that if you had some translation data for let's say Bengali but not Bengali-English let's say you had you know Bengali um to Hindi and Hindi to English um that you could get some kind of a translation between English and Bengali out of that, but again how well would it work right--
ALEX HANNA: Right.
EMILY M. BENDER: --to what quality? Um but that's not that's not completely implausible and in fact you can get interestingly far with just monolingual data in a bunch of languages and then a seed lexicon for connecting up the distributions. So it's like non-trivial amounts of stuff comes out. Would you want to use that in any situation where you care about the accuracy of the output? No. But as sort of a research question you can do something there.
ALEX HANNA: Yeah yeah, absolutely. Um I want to get to this last one and then I really want to get to this this incredible incredible next set of of paragraphs and claims. Um, "Instructability: These models are capable of quote "in-context learning" where they learn from a prompt rather than from training data. In quote "few-shot learning" a new task is demonstrated with several example input-output pairs and the system then gives outputs for novel inputs. In quote "zero-shot learning" a novel task is described but no examples are given. For example 'write a poem about cats in the style of Hemingway' or quote--" I don't know if I can pronounce this, "--'equa-equiantonyms are pairs of words that are the opposite of each other in have the same number of letters. What are some equiantonyms?'"
And this one is you know I mean and in this whole language of few-shot learning and zero-shot learning is a bit disingenuous right because you still have to have massive pre-training on anything, and to give a few things. So if you have errors or you have biases or you have quote unquote "hallucinations" in your in your pre-training, then that's going to show up downstream in any of the few shot or zero shot um instances right.
So it's it's a it's a bit of a um a a a misnomer. I know I find the continued discussion of that to be a little disingenuous.
EMILY M. BENDER: Yeah yeah. And also the whole--this is sort of a side point but the zero-shot few-shot stuff has always struck me as just so macho like it's--
ALEX HANNA: Yeah. Oh completely, yeah.
EMILY M. BENDER: Yeah.
ALEX HANNA: Yeah it's the it's the opposite of size, it's it's it's like um it's like uh that old uh TV show, "Name That Tune." You know.
EMILY M. BENDER: Oh yeah.
ALEX HANNA: I could I could name name it in three notes. I could name it in no notes.
EMILY M. BENDER: Yeah. [Laughter] We can we--so zero-shot learning is yeah we'll give you the answer you were thinking of before you even asked the question.
ALEX HANNA: I know. Zero-shot learning is precognition. You heard it here fi--first folks. So--
EMILY M. BENDER: You heard it here zero-th.
ALEX HANNA: You heard it here zero--oh gosh we have to zero index it. Um all right. This is this is um so they they they give this short definition or not even a a short def-- I wouldn't call this definitional either but it was sort of it is sort of a pragmatic scoping of what general intelligence is. So I want to read this and then I'm going to go off about a small rant about general intelligence, and then we should probably get on to the the how they address the criticisms of of of AGI.
Uh so there so they said, "'General intelligence' in quotes must be thought of in terms of a multi-dimensional scorecard, not a single yes-no proposition. Ne--nevertheless there is a meaningful discontinuity between narrow and general intelligence. Narrowly intelligent systems typically perform a single or predetermined set of tasks, for which they are explicitly trained. Even multitask learning yields only narrow intelligence, because the models still operate within the confines of tasks envisioned by the engineers. Indeed much of the hard engineering work involved in developing narrow AI amounts to curating and labeling task specific data sets. By contrast, frontier language models can perform competently at pretty much any information task that can be done by humans, can be posed and answered by using natural language as quantifiable performance. The ability to do in-context learning is especially meaningful uh meaningful metatask for general AI. In-context learning extends the range of tasks from anything observed in the training corpus to anything that can be described, which is a big upgrade. A general AI model can perform the task that this designers never envisioned."
And here they link to a Quanta Magazine article that is about this kind of um--hyping up this kind of notion of emergent behavior uh and and it covers some of the research that um some folks at Stanford and at uh Anthropic have done. So okay, so we're getting we're getting here into this like kind of debate around emergence, but I want to go back just to this idea of general intelligence, this idea of general intelligence oh--and I just realized I threw my phone I think cuz my cat was on it, onto this. And I had a backup of our audio on my phone, so sorry sorry Christie, our producer. But general intelligence, I mean they don't get into it here um but this idea of general intelligence has this long sordid history right.
And I I think we might have hinted at it on the on the pod before, but the idea of general intelligence as being this thing, just kind of measurable single proposition which has this history in in in IQ tests, um by by Spearman and his concept of G, uh the concept that and and effectively that these things can are ingrained differences. They they they do this huge side-stepping of discussing any of that, and a little bit by saying, well it's not a single yes-no uh, instead we need to give it a scorecard, and also uh uh this thing that should be considered to be general intelligence is this question of emergence. Um which is yeah--that's incredibly questionable.
EMILY M. BENDER: Yeah. Yeah.
ALEX HANNA: And I mean it's it's yeah--go ahead and I mean it's yeah sorry I have more to say on it, but I want to--that was my pause to let you jump in.
EMILY M. BENDER: All right, so the first sentence here, "General intelligence must be thought of in terms of a multi-dimensional scorecard, not a single yes no proposition." I said but but defined how? Like as you said they're not actually defining it. They're contrasting it to narrow intelligence which is--or narrowly intell--no narrowly functional systems right? I think it makes a whole lot more sense to talk about functionality but I was particularly angry at this last sentence. "Indeed, much of the hard engineering work involved in developing narrow AI amounts to curating and labeling task-specific data sets." And I was angry about this because that is hard work, and it is skilled work and it is never valorized.
ALEX HANNA: Yeah.
EMILY M. BENDER: And so now when they're saying well that's not important because it's just for narrow AI, now they're going to valorize it.
ALEX HANNA: Yeah, yeah. Yeah and I mean that's that's such a that's such a good point right, and that's al--also untrue right because so much of the the RLHF work, the red teaming work the um the content moderation still falls on those same people doing you know that did all that hard creating and labeling right.
It's also really interesting on how they frame it as 'hard engineering work' too because this this kind of--excuse me this kind of strikes back to how computing work became masculinized uh which which actually they they they do get into in the article but you know because but it but and they don't they don't refer to anybody who's done that important work, like like um um um Janet Abbate or um which I think I refer I refer to in the the first uh one of the first podcasts that we did, and also Nathan Ensmenger's book, 'The Computer Boys Take Over.' And so you know they so they're saying but now it's engineering work. So yeah thanks for calling that out, that's that's really something.
EMILY M. BENDER: And so the the other thing that I wanted to say was this this next little bit here, right. "By contrast, frontier language models can perform competently at pretty much any information task that can be done by humans, can be posed and answered using natural language, and has quantifiable performance."
In other words if you are willing to take the output text extruded by these machines as the answer to what you're looking for, and you can shape the question so that you can make sense of it that way then they can do it. In other words it's all on the people using it and not on the machine. But they just aren't recognizing their own sense-making capability in here.
ALEX HANNA: Yeah yeah, that's a really good point. Um so we got about like--so now now we we've as we did in the first Blaise artifact where it took us three episodes to get through, we're only about one page through this, and and there's still four--the four criticisms. So maybe in the next 15 minutes we can do a skim on it.
EMILY M. BENDER: Yeah.
ALEX HANNA: So the next part says, "So why the reluctance to acknowledge AGI? Frontier models have achieved a significant level of general intelligence according to the everyday meanings of those two words." Okay that's already doing a ton of work um. "And yet, most commenters have been reluctant to say so far uh so far to say so--" I guess that's a typo, "--so far it seems to us um so--" No sorry that's not a typo, excuse me. "--to say so for, it seems to us, four main reasons. 1. A healthy skepticism about the metrics for AGI. 2. An ideological commitment to alternative AI theories or techniques. 3. A devotion to human or biological exceptionalism. And 4. A concern about the economic implications of AGI." Okay.
EMILY M. BENDER: I got to say something before we get into any of these.
ALEX HANNA: Yeah do it okay do it.
EMILY M. BENDER: So as you say, um the sort of appealing to the everyday meanings of general intelligence is is a total cop out, but also this question, "Why the reluctance to acknowledge AGI?" is presupposing the existence of AGI. They're basically saying it exists, why are people not doing it? And then yeah they basically say well here's the possible reasons, and none of them are, 'actually it's bullshit.'
ALEX HANNA: Yeah.
EMILY M. BENDER: [Laughter] Right?
ALEX HANNA: Right. I mean it's--
EMILY M. BENDER: They can't admit that.
ALEX HANNA: It's that it is it is is also doing I mean and the Stanford people have done a bit of an end run about this. Already we're talking about 'frontier models,' I mean which is a term that they came up with as this coalition, there's this center for frontier models, whatever, the same with 'foundation models' which I guess we've already given up on and I mean--
EMILY M. BENDER: No I still I still object when I see it.
ALEX HANNA: Yeah well no I mean I no I I object too, I'm saying they've given up on it right, and I mean and I just think I mean you can you know you can you can call you know--well I don't want to be too uh vulgar on the podcast but you can call a a pig a pig right it's uh or you can call a pig a um--I don't want to be offensive to pigs here you--
EMILY M. BENDER: Yeah I think we gotta keep moving Alex, to not be offensive and also I don't think we have time for Suleyman's stupid um "raise money" test.
ALEX HANNA: Yeah yeah, let's let's let's--so the met so the metrics test, I mean they they're effectively going and and and and saying, like let's let's find out how to do metrics um you know and and effectively they're going into and saying like well what are what are we doing here, um um you know and they're they they are to some degree acknowledging the kind of problem with metrics um but also saying that this is you know we are solving other metrics. So they point to Percy Liangs work on HELM if you remember our uh podcast with uh Jeremy Khan, we address--
EMILY M. BENDER: Episode seven.
ALEX HANNA: Yeah episode seven, we addressed that. We addressed the kind of moving standard of of of these test suites and the problem with those right. Um and but that's not even--and so even if we object to metrics we can't even really agree on metrics right, um and that's--
EMILY M. BENDER: Yeah and now we're back to Grover, right, they're they're they're saying--you know if we say that metric's not good then they'll say okay what's the metric that tests for general intelligence? Like well, you can't test the everything machine. It's by definition untestable. And number two, it's not the job of the critics saying, 'Hey your test isn't working, your claims are false,' to come up with the alternative test. Because we're not trying to build the thing. The burden of proof lies with the people making the extraordinary claims.
ALEX HANNA: Yeah, yeah, 100 percent.
EMILY M. BENDER: Yeah, and I was mad at this paragraph.
ALEX HANNA: Yeah yeah.
EMILY M. BENDER: Um yeah because I mean I agree, right. "It's also important--"
ALEX HANNA: Well reading this paragraph, "It is important--" Oh sorry, you were reading it, go ahead.
EMILY M. BENDER: "It is also important not to confuse linguistic fluency with intelligence--" Yes, absolutely true um. And yet they somehow don't recognize that they're being fooled in the same way, um--
ALEX HANNA: Yeah.
EMILY M. BENDER: And then they say, "We call this the 'Chauncey Gardiner effect" after the hero in 'Being There.' Chauncy is taken very seriously solely because he looks like someone who should be taken seriously." It's like you couldn't cite any of the people who've already been talking about this?
ALEX HANNA: No they gotta--they gotta--they gotta claim some new words.
EMILY M. BENDER: Yeah. Okay.
ALEX HANNA: Yeah, okay.
EMILY M. BENDER: I think we're gonna move on from metrics.
ALEX HANNA: Yeah so going to, "Alternative Theories." So so, "The prehistory of AI includes many competing theories--" Here they go after "Good Old-Fashioned AI" or GOFAI. "The GOFAI credo, drawing from a line back from Gottfried Wilhelm Leibniz, the 17th-century German mathematician, is exemplified by Alan Newell and Herman Simon's physical symbol--um system hypothesis, which holds that intelligence can be expressed in terms of calculus, wherein symbols represent ideas and thinking consisting of symbol manipulation according to the rules of logic." Um and it's and so then they they go into sort of GOFAI and why people are are more invested in kind of a symbolic um kind of a symbolic reasoning um and so they you know they talk about um um and then--and they talk about Chomsky's criticism I I'm gonna I'm gonna let you yeah I want you to weigh here because you're you're highlighting here--
EMILY M. BENDER: I'm highlighting something yeah.
ALEX HANNA: --and and it's and and yeah go into it.
EMILY M. BENDER: So the thing that got me here is they say, "That's why for decades, concerted efforts to bring together computer programming and linguistics failed to produce anything resembling AGI." It's like actually plenty of people were bringing together computer programming and linguistics to build language technology with zero interest in AGI.
ALEX HANNA: Yeah.
EMILY M. BENDER: Right like you cannot paint all of that work as if it were a failed attempt to do the thing that you want. That's just--
ALEX HANNA: I also think it's very interesting that this is kind of like the kind of um referent here to Newell and--Newell and Simon here kind of as they wanted to effectively say that we could you know establish everything as a predicate calculus. Um this is another thing I kind of got from Jonnie Penn's dissertation, which is effectively this effort wasn't necessarily like focused--as I understood this and if anyone has any more knowledge of this history hit me up in the comments--but it wasn't necessarily aimed at doing this as as part of an ideological commitment, but a lot of it was methods of convenience as if they got basically access to uh Bertrand Russell's um um Precipita--I'm not saying this right--Principia Mathematica, and effectively saying, okay we can try to prove some of these axioms in here and if we can do this this is kind of like an effective test of this.
But it prove to be not really able to do so. So I think it's less of a less of an ideological commitment than they like to say um although we might you know they do make reference to um Gary Marcus, who may be more committed to such a thing. Um but I you know it's it's it's such a it's such a weird kind of thing that people have this kind of ideological commitment to uh GOFAI rather than to uh kind of neural network architecture.
EMILY M. BENDER: Yeah I'm just scrolling down here to try to get to--um because we we so there's Gary Marcus, gotta keep moving um wait, I've got this printed out in front of me too.
ALEX HANNA: Yeah.
EMILY M. BENDER: So ah um this one irritated me. "As AI critics work to devise new tests on which current models still perform poorly, they are doing useful work--" Yes yes, keep it up AI critic you're doing useful work. Um, "--although given the increasing speed with which newer larger models are surmounting these hurdles, it might be wise to hold off for a few weeks before once again rushing to claim that AI is (in quotes) 'hype.'" I was just like, first of all nobody is saying that AI is hype, we're saying that your claims of AI are hype. And secondly um they're basically saying all those times in the past we failed, okay we failed. But the next time the the next time we're getting it right well then we've really built AI.
ALEX HANNA: Yeah, this this is what I think--this is what I think I--I sent this to you in the chat in in a chat Emily but I think this we could call this, 'the Hypers Horizon.' It's always five years off. Right?
EMILY M. BENDER: Yeah. [Laughter] And I I want there's one thing I want to pick up back here and then I'm gonna let you say where we need to go next, um.
ALEX HANNA: Yeah.
EMILY M. BENDER: They say, "Without explicit symbols. according to these critics, a merely learned statistical approach cannot produce true understanding." It's telling to me they don't actually take on Bender and Koller 2020.
We aren't saying 'without explicit symbols,' we're saying if the input is only form, you can't learn meaning. But they clearly haven't read that paper, haven't understood it.
ALEX HANNA: Well they link--they actually link to it.
EMILY M. BENDER: They do, where?
ALEX HANNA: They pri--they linked to it prior to the uh block quote uh, the there's the paragraph on Chomsky. But yeah especially if they are trained purely on language.
EMILY M. BENDER: Hey look, that's us. But "purely on language" is wrong--it's purely on linguistic form. Um so they haven't understood. Okay um all right.
ALEX HANNA: Let's uh let's go on to "Human or Biological Exception--Exceptionalism." This is basically saying okay, um you know uh some people are not willing to accept any empirical evidence of AGI. Uh first off you know, okay empirical evidence of AGI is is is not is not uh it's not a thing. Um but the sort of thing that they they go into is they need to argue that--you know these people need to argue that humans are special or humans are uh exceptional.
Um and so um and so then they they they go down and say, you know we need to say that these things are beyond tools so they're they go through Mustafa Suleyman, which I guess in his book says that we need to think about artificial capable intelligence. Which is a purely capitalist enterprise, uh you can turn $100,000 into a million dollars. Incredible, I love this as a measure of intelligence, um uh I say this with such dripping sarcasm. Um--
EMILY M. BENDER: But but Alex don't you know the more millions you have, obviously the more intelligent you are. Like that's how it works, right.
ALEX HANNA: Yeah that's right we--we are doing we're just drawing a line from money to intelligence, wonderful. I love--I love it, incredible. Um so they go down and say um so they they end up here they kind of hem and haw a lot on here. And and what they're trying to do and--and I I kind of want to applaud them on here because they make the distinction between consciousness and intelligence.
Um so they say, "We--we have no idea how to measure, uh verify or falsify the presence of consciousness in an intelligent system. We could ask it but we may may or may not believe its response--" And this is harks backs to Blaise's initial uh impetus for writing the article, which is um basically dogging on Blake Lemoine, who was fired from Google uh for for effectively you know saying AI was sentient, but then uh or uh but then leaking company secrets.
Uh, "Believers in AI sentience will accept a positive response while non-believers will say it's merely 'parroting'--" Uh a little subtweeting of y'all uh. "--or they're 'philosophical zombies.'" Um and then and then um--and then what they kind of go down and say is is sort of um--they they sort of flip this here, they say, "To claim a priori that non-biological systems simply can't be intelligence or conscious because they're quote 'just algorithms' for example seems arbitrary, rooted in untestable spiritual beliefs."
So they're making the claim that if you are saying that they're un not intelligent or conscious this is just as a spiritual belief. Um, which I mean is there is that--is that symmetric there? Um it's it's just like it's it's it's they they and--and what I really and this it's--I want to take this as a rhetorical move because it's effectively like, you know there's a move that I think a lot of people--there's a trend that a lot of people in let's say long-termism and others and the people who be--like believe in super intelligence seem to do, which is saying, well maybe humans aren't at--they they say, 'well maybe humans aren't as exceptional,' um but they they make a bit of an appeal to like an environmentalist or like a kind of a a green kind of thing. Like it's just--and that like really irks me.
Because you're saying like well one, these things are not climate friendly to begin with, right, so like how dare you even put it in the same camp. Two, it's okay to unsettle humanness and if you actually listen to people who you know like uh people like Indigenous scholars and and Indigenous elders yeah actually that's that's actually not a bad sort of thing. Building building a quote unquote 'AGI' is is not it though. You are not decentering the the the human in a way that is actually uh kind of uh writing our relationship with the Earth in any kind of meaningful way, and that just that's a thing that really grinds my gears.
EMILY M. BENDER: Yeah. I just want to put in a plug for the All My Relations podcast, which is a wonderful podcast by two North American Indigenous scholars. And they've got so much to say that's that's really really fruitful about being in relationship you know with the planet, which is not this. And to my knowledge they haven't taken on AI, but it's also like not what they're talking about.
ALEX HANNA: Yeah.
EMILY M. BENDER: Yeah.
ALEX HANNA: Yeah, yeah.
EMILY M. BENDER: Alex we have to get to the economic implications. I'm biting my tongue because I want to hear what you have to say about this. So let let me read that first paragraph, just so you can go off on it, all right.
ALEX HANNA: Yeah.
EMILY M. BENDER: "Arguments about intelligence and agency readily shade into questions about rights, status, power and class relations -- in short, political economy. Since the Industrial Revolution, tasks deemed rote or repetitive have often been performed by low-paid workers while programming -- in the beginning considered women's work' -- rose in intellectual and financial status only when it became male-dominated in the 1970s. Yet ironically, while playing chess and solving problems in integral calculus turn out to be easy even for GOFAI, manual labor remains a major challenge even for today's most sophisticated AIs." [ Laughter ]
ALEX HANNA: This is this is this is very this is--I mean first off, the separation between mental and manual labor is a bit artificial, right. I mean any kind of manual labor uh entails a very real kind of embodied knowledge and work. So that's already that's already a division I I I refuse.
The thing that really got me here uh was the the second graph in the section, which was um talking about the mid mid-50s and the Dartmouth conference and they say, "At the time, most Americans were optimistic about technological progress. The quote 'great compression' was under way, an era in which the economic gains achieved by rapidly advancing technology were redistributed broadly, albeit certainly not equitably, especially with regards to race and gender. Despite the looming threat of the Cold War, for the majority of people the future looked brighter than the past."
And it's just like 1950s, what else is happening? Oh right, the Civil Rights Movement. And like and you know and you know this this this post-war era itself, which was a bit exceptional in sort of the history of of of of uh of the US, this kind of era of Great Society, and it's sort of so um yeah it's it's so um colorblind not to see that the way that these were drastically awful times, a time of Jim Crow segregation, a time of increasing and very marginal movement. It wasn't but for a mass movement that could even move on that at all.
And so yeah, I mean and it's and so then--they effectively then and I want to take this because I want to include this--they basically like at the next paragraph they say that, "Today that redistributive pump has been thrown in reverse." Okay I and I would say that's a revisionist reading, it's not in reverse actually. That's continuing what we've had. "The--the poor are getting poorer and the rich are getting richer, especially in the global North.
When charact--the word AI is characterized as not 'neither artificial nor intelligent,'--" which is uh and they cite to Kate Crawford's "Atlas of AI" book, "--but merely a repackaging of human intelligence, it is hard not to read this critique through a lens of economic threat and security." And I'm like okay yeah, actually don't disagree actually yeah, there is this Matthew effect, and it is a continuity from a mid-50s uh vision. Uh and so they conclude by saying you know, AGI needs to be asked who benefits, who is harmed, who--how can we maximize benefits and minimize harm, and how can we do this fairly and equitably.
And we would say definitively don't build AGI, it is not anything that is uh fruitful for human flourishing, and and I use this in a way that is not referring to the type of uh effective algorithms human--uh effective uh altruist human flourishing but more in the uh uh envisioning real utopia is human flourishing that Eric Olin Wright and other people use this.
But it's just a really infuriating end of this in which they say, this is an objection, and we agree, and we should just do better. Okay, great.
EMILY M. BENDER: Right and but they also say "the much needed 'ought' debates are best carried out honestly" and if we don't separate what the debates about what it should be and what it is then we're going to be muddying the waters. And I'm like no, because it isn't, it doesn't exist. And if you're making these claims that it does exist and that it's worth trying to pursue and that it's gonna have all these magical properties, that's muddying the waters of the 'ought' debates.
ALEX HANNA: Yeah.
EMILY M. BENDER: And and also when they say, "It is hard not to read this critique through the lens of economic threat and insecurity," I think that's an attempt to discredit the critique. Um.
ALEX HANNA: It--I read this twice and it's and it's and it's--I feel like it's a tacit agreement with the critique and then--but then say we need to we need to envision an a AGI that works for all of us, it is um yeah, I mean it's it's yeah.
EMILY M. BENDER: Yeah. All right Alex, we have only 10 minutes left so I'm going to take us to Fresh AI Hell, and your prompt this week is you're a demon who late for their train to Fresh AI Hell and you're rushing along got to get there.
ALEX HANNA: Oh gosh all right.
[Lower pitched voice] Ah shit, ah shit ah stop stop. Ah ah holy crap oh stop it stop it ah I'm gonna be late at the job, oh my gosh. Last time Lucifer was so mad I oh I didn't pull out enough teeth, oh. I'm just down here torturing Norbert uh uh Weiner--
[Normal voice] and wasn't Norbert Weiner the the cybernetics guy? Sorry that's all I got. Let's move on.
EMILY M. BENDER: [Laughter] Oh man, that was fun. Okay, so we have lots of Fresh AI Hell. We're gonna do this rapid fire.
Uh from Meredith Whittaker, a tweet. Um, "Disturbing to see this offered by a reputable J-school." And what's being offered is from the UT Austin Knight Center for Journalism in the Americas, a post saying, "Generative AI is here to stay and will only improve and expand --it's a good idea to get in now and learn as it develops." Um and then uh below it says, "Nearly 7,000 students from 147 countries already enrolled in online course on AI and newsrooms."
And there's suggestions for how this can be used to help journalists: transcription, sorting disperate data, producing newsletters, and scheduling social media.
Um it is really scary to see a J-school saying, 'Sure you can use gen-AI, synthetic text to write your newsletters.'
ALEX HANNA: Yeah, I mean yeah that's a that's a big 'woof' moment there.
EMILY M. BENDER: Yeah. Okay uh so uh, Will Ahmed tweets, "Breaking: we have partnered with @OpenAI to launch WHOOP-Coach today, the most advanced generative AI feature to ever be released by a wearable. Members can now ask @WHOOP anything about their data and receive instant feedback."
So this is a system for um doing personal coaching, I guess, and they're just they've just put GPT-4 inside of it?
ALEX HANNA: Yeah is it is it on the the watch or something? And I guess it's and it's, you know, I'm here and and and we need to uh work out. If I'm stuck in traffic, and they've got this very flashy w--video uh with things that say um you could go through all this search or you could go through WHOOP to figure it out. Fun stuff.
EMILY M. BENDER: So so my tweet was, "GPT-4 is inside this thing. So are we talking days, hours or minutes before we see the first reports of physically dangerous advice coming out of it?" And of course I got people saying, well you can find dis--physically dangerous advice on the internet all the time.
ALEX HANNA: Yeah.
EMILY M. BENDER: Yeah. Okay, um how about you do this one?
ALEX HANNA: Yeah, so this is a um a toot by Shannon Skinner, uh in which they which she says, "Cigna, a US health insurance provider, is being sued for the second time this year for using automated intelligence to deny medical care claims so they don't have to pay for them." The quote: "Cigna's algorithmic review process trades patient care for profit, allowing the provider to eliminate the cost of necessary review by doctors and qualified professionals and instead rely on impersonable--impersonal, illegal review by almost an almost completely automated algorithm."
And then, Cigna's defense: "They claim that because the review takes place after the patients have received treatment, it does not result in any denials of care." Woof.
"No one said you denied care Cigna, you denied payment for care. Hey Cigna maybe you need to be reminded that the service you sell is paying for care."
Yeah, this is a nightmare I mean the kind of idea of I mean--getting things processed by your insurance to begin with is terrible, um, and anyone who's had to deal with any kind of um kind of--I I wanted to say rare exceptional condition or medical condition, but it's really many medical conditions on this.
EMILY M. BENDER: Yeah.
ALEX HANNA: Um and uh trying to be on the phone with insurance for hours and trying to resolve this is a nightmare. Imagine being on the phone or writing a report and getting an automated rejection. Just nightmare conditions.
EMILY M. BENDER: So terrible, so so terrible. I'm actually going to skip ahead to this one, because it's also medical care. "The Madrid Health Service, a pioneer in applying generative artificial intelligence to improve diagnosis for patients with rare diseases."
This is by the way a Microsoft press release, and what's happened is they have--they're piloting, and as I understand it with actual patients, um a system where GPT-4 is meant to be helping the physicians diagnose rare diseases. Has this been tested through a medical trial? I don't think so.
It is really really frustrating, and Microsoft says Madrid Health Service is a 'pioneer' for doing this. I mean it's especially scary because it's rare diseases, right, and I mean I think that's and and that's just we don't know what in the training data, we don't know how rare diseases typically get diagnosed. Um.
EMILY M. BENDER: Also when you're a patient coming in with a disease, you don't know that it's a rare disease initially, right.
ALEX HANNA: Also true, yeah.
EMILY M. BENDER: And you know we've got to go through all the stuff about false positives, false negatives, how does it affect people, yeah. All right um I'm gonna keep us going.
ALEX HANNA: Gosh.
EMILY M. BENDER: You want to do this one?
ALEX HANNA: Yeah, do it. Yeah yeah. So this is a tweet by our friend Mustafa Suleyman, not actually our friend, um and he says, "AI for science is already here. 20% of researchers say they use LLMs as a scientific research--uh scientific search engine or for brainstorming or literature reviews or even to help write manuscripts."
This is an awesome paper published in Nature um, and they have a bar graph. Um the kinds of things that they do is "for creative fun not related to my research," 40%, to help write code, about 33%, to brainstorm research ideas--and Emily's circling "to conduct literature reviews" in particular, um that hangs around 23%. And if yall remember Galactica, uh famous for uh making up citations, incredible stuff.
EMILY M. BENDER: All right I'm gonna keep us moving though because we could wallow in that for a while, we don't have time. Um okay once again the idea that AI is going to be useful for mental health therapy. So Lillian Wang says, "Just had a quite emotional personal conversation with ChatGPT in voice mode, talking about stress, work life balance. Interestingly I felt heard and warm.
Never tried therapy before, but this is probably it? Try it, especially if you usually just use it as a productivity tool." And then that's quote tweeted by Ilya Sutskever: "In the future, once the robustness of our models will exceed some threshold, we will have wildly effective and dirt cheap AI therapy.
Will lead to a radical Improvement in people's experience of life. One of the applications I'm most eagerly awaiting."
ALEX HANNA: Oof. This one--yeah this one's a this one's been on the hypers' horizon for uh for over uh 70 years now. Uh in in uh Weizenbaum's "Computer Power and Human Reason," he talks about how psychologists were saying this about ELIZA. Yeah that ELIZA, the chat bot. And he cites--talks about basically giving the program to his secretary and the secretary spent many--you know a bunch of time with it and uh basically telling it her life. Um and then many popular psychologists were saying, oh we could provide very cheap therapy if we had this. 70 years later we still don't have cheap therapy.
EMILY M. BENDER: Right because we can't.
ALEX HANNA: Yeah. We still have an incredibly backed up mental health system with incredible burnout from many many mental health professionals, especially after COVID.
EMILY M. BENDER: Yeah. All right, um, we are not getting through all these but, "Six Tenets of Postplagiarism: Writing in the Age of Artificial Intelligence." This is a tweet from Dr. Seline--Dr. Sarah Elaine Eaton, um where she seems to be claiming that because there is now synthetic media machines, we have to rethink how we think about plagiarism. I just want to bring up one of the things here in this graphic: "Historical definitions of plagiarism no longer apply."
Um and then it's expanded as, "Historical definitions of plagiarism will not be rewritten because of artificial intelligence, they will be transcended. Policy definitions can and must adapt." And it's like the the defeatism here, it's like no we actually can resist this stuff. And like you know we don't just have to roll over like that. Right, yeah, that's a that's a rough one.
EMILY M. BENDER: Yeah.
ALEX HANNA: Uh this this one is a tweet from Luke Goldstein says, "This is wild. Sarah Ziff from the Models Alliance--" and mind you this is not an alliance of of comp--of statistical models, it is speaking about clothing models and supermodels, etc is speaking at FDC forum--" and this was an f--a forum that the FDC did about creative fields, which is great, I haven't watched it but it's on my list. Um, "--and apparently modeling agencies, which rely almost entirely on independent contractors, have been using AI modeling deep fakes to meet quote 'diversity goals' instead of just actually paying real models." Oh so this is incredibly trash, so just making up uh Black and brown models rather than getting actual models. Truly truly wild stuff.
EMILY M. BENDER: Yeah and I think that's all we have time for this time, so we will um have lots of Fresh AI Hell for our upcoming episodes, um which should be coming pretty fast and furious for a little while which is exciting. And maybe one day we will be on top of the AI Hell. Um but I do want to raise up something that WisewomanForReal has put in the chat. "Resisting seems so hard, as if one is alone out there. Even postdocs talk about finally having help with the quote 'boring literature reviews.'"
And I just want to say please don't feel alone, that's part of our purpose with this podcast and we love the community that's being built up around it, um it's basically hopefully a space to feel like you're not the only one who's saying what are people thinking?
ALEX HANNA: Yeah, absolutely.
That's it for this week. Our theme song is by Toby Menon. Graphic design by Naomi Pleasure-Park. Production by Christie Taylor. And thanks as always to the Distributed AI Research Institute. If you like this show you can support us by rating and reviewing us on Apple Podcasts and Spotify, and by donating to DAIR at Dair-Institute.org. That's D-A-I-R hyphen institute dot O-R-G.
EMILY M. BENDER: Find us and all our past episodes on PeerTube and wherever you get your podcasts. You can watch and comment on the show while it's happening live on our Twitch stream. That's twitch.tv/DAIR_Institute. Again that's D-A-I-R underscore Institute. I'm Emily M. Bender.
ALEX HANNA: And I'm Alex Hanna. Stay out of AI Hell y'all.