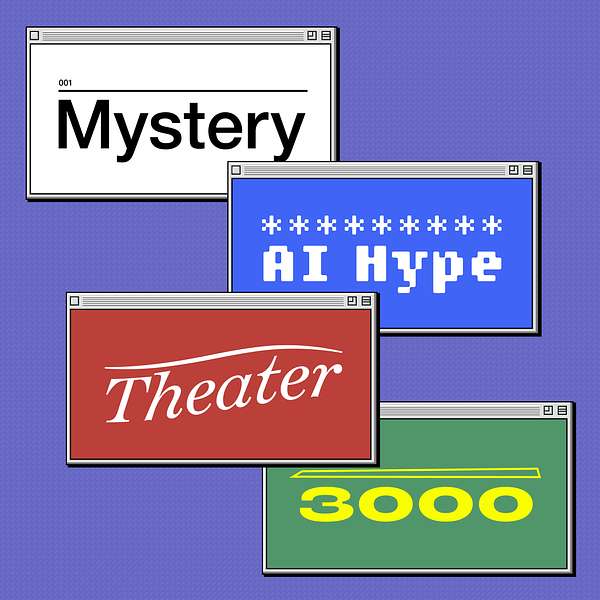
Mystery AI Hype Theater 3000
Mystery AI Hype Theater 3000
Episode 19: The Murky Climate and Environmental Impact of Large Language Models, November 6 2023
Drs. Emma Strubell and Sasha Luccioni join Emily and Alex for an environment-focused hour of AI hype. How much carbon does a single use of ChatGPT emit? What about the water or energy consumption of manufacturing the graphics processing units that train various large language models? Why even catastrophic estimates from well-meaning researchers may not tell the full story.
This episode was recorded on November 6, 2023.
References:
"The Carbon Footprint of Machine Learning Training Will Plateau, Then Shrink"
"The Carbon Emissions of Writing and Illustrating Are Lower for AI than for Humans"
The growing energy footprint of artificial intelligence
- New York Times coverage: "AI Could Soon Need as Much Electricity as an Entire Country"
"Energy and Policy Considerations for Deep Learning in NLP."
"The 'invisible' materiality of information technology."
"Counting Carbon: A Survey of Factors Influencing the Emissions of Machine Learning"
"AI is dangerous, but not for the reasons you think."
Fresh AI Hell:
Not the software to blame for deadly Tesla autopilot crash, but the company selling the software.
4chan Uses Bing to Flood the Internet With Racist Images
Followup from Vice: Generative AI Is a Disaster, and Companies Don’t Seem to Really Care
Is this evidence for LLMs having an internal "world model"?
“Approaching a universal Turing machine”
Americans Are Asking AI: ‘Should I Get Back With My Ex?’
You can check out future livestreams at https://twitch.tv/DAIR_Institute.
Subscribe to our newsletter via Buttondown.
Follow us!
Emily
- Twitter: https://twitter.com/EmilyMBender
- Mastodon: https://dair-community.social/@EmilyMBender
- Bluesky: https://bsky.app/profile/emilymbender.bsky.social
Alex
- Twitter: https://twitter.com/@alexhanna
- Mastodon: https://dair-community.social/@alex
- Bluesky: https://bsky.app/profile/alexhanna.bsky.social
Music by Toby Menon.
Artwork by Naomi Pleasure-Park.
Production by Christie Taylor.
ALEX HANNA: Welcome everyone to Mystery AI Hype Theater 3000, where we seek catharsis in this age of AI hype. We find a worst of it and pop it with the sharpest needles we can find.
EMILY M. BENDER: Along the way, we learn to always read the footnotes and each time we think we've reached peak AI hype, the summit of Bullshit Mountain, we discover there's worse to come. I'm Emily M. Bender, a professor of linguistics at the University of Washington.
ALEX HANNA: And I'm Alex Hanna, director of research for the Distributed AI Research Institute. This is episode 19, which we're recording on October 30th 2023. And we're going to talk about the environmental footprint of widespread adoption of large language models, which rely both on specialized computer chips, and lots and lots and lots of work from servers around the world. Recent peer-reviewed estimates suggest the use of quote "AI" globally requires massive amounts of energy with a correspondingly large carbon footprint.
EMILY M. BENDER: But the AI hypers would have us believe that AI is what's going to save us from the climate crisis, from claims that AI energy use will actually decline down the road, to the ridiculous suggestion that AI uses less energy than human beings to perform the same tasks. And fortunately for us we have two amazing researchers here to help us tear apart these greenwashing claims.
Dr. Emma Strubell is an assistant professor in the Language Technologies Institute in Carnegie Mellon University's School of Computer Science. Her research sits at the intersection of machine learning and natural language processing, with a focus on providing pragmatic solutions to practitioners who wish to gain insights from natural language text via computation and data-efficient AI. Welcome, Emma.
EMMA STRUBELL: Thanks for having me, hi.
EMILY M. BENDER: Thanks for being here.
ALEX HANNA: And Dr. Sasha Luccioni is a researcher in ethical and sustainable artificial intelligence at the company Hugging Face. Her work focuses on having a better understanding about the societal environmental impacts of AI models, data sets and systems. She's also a founding member of of Climate Change AI.
Hey there Sasha.
SASHA LUCCIONI: Hey. I'm also very excited to be here, I'm a big fan of the show.
EMILY M. BENDER: This is cool.
ALEX HANNA: We're so excited to have you on, yeah.
EMILY M. BENDER: Before we get into our uh artifacts for today, I have to show off this earring and Alex has them too.
ALEX HANNA: Yes.
EMILY M. BENDER: Friend and fan of the show Nanna made us little books that have the wonderful icon developed by Naomi Pleasure-Park for Mystery AI Hype Theater and Alex and I are feeling special.
ALEX HANNA: I know, I've never gotten sort of earring jewelry swag on any kind of uh, I don't know what what this is called, a brand. So I'm super excited.
EMILY M. BENDER: All right so let's dive right in. Here is our first artifact. Um Emma do you want to start us off here, and you want to tell us what we're looking at?
EMMA STRUBELL: Um yes sorry let me--I have like lots of feelings about this paper, so let me me try to channel them into something um cohesive. Yeah so um this is a paper that came out of folks at Google, you'll see the affiliations are like Berkeley and things, but like Dave Patterson for example did this as part of his work at Google, mainly. Um uh and um--yeah I mean the I guess the takeaway that they would like for you to have from their paper is sort of very clearly in the title, which is sort of a good PR um thing I guess. So they're claiming that, "The carbon footprint of machine learning training will plateau and then shrink."
Um the paper does not uh really provide evidence to support that hypothesis um [laughter] and uh I don't know how much we want to get into this, but I think this paper was written basically entirely um as a PR thing um uh on behalf of Google in response to papers um, including mine, including one by Emily including--I don't know if at that time by Sasha but since by Sasha for sure, um yeah basically pointing out that maybe we should be worried about the carbon footprint of machine learning training. Um--yeah.
EMILY M. BENDER: I I have to share a story right now, Emma, which is how I met you. So in in 2019 in September I was at the Seattle Climate March and I saw this sign that said, "Ask me about the carbon impact of AI." And I thought, I've got to go tell that person about Emma Strubell's amazing paper at ACL 2019.' So I pushed my way through the crowd and it was you.
ALEX HANNA: That's amazing. Wow.
EMILY M. BENDER: So which is to say that that you know Emma has been on this beat for a while, and really you know raising important questions and doing really careful work sort of saying, 'Okay how do we think about this, how big is it, and like what does that mean for us as researchers, and what does it mean I think for industry?' Um and apparently um between you know Emma and others you know like--in the the Stochastic Parrots paper frequently gets cited um as like you know, 'we should worry about the carbon impact' citation to Stochastic Parrots. And I'm like anytime they do that, they really should be citing you know your work, and your work Sasha.
Like we we point to that work and the only thing Stochastic Parrots really added was sort of reminding people to think about the environmental racism angle. But the underlying like research about what's going on here is yours.
Um so collectively I guess we got under their skin and so they wanted to say actually it's not so bad. And so where should we go in this paper to see how they're trying to say that?
SASHA LUCCIONI: I mean it's a lot of predictions right? I think that's kind of what makes it I mean for me very unnerving is that it's really hard to argue with [laughter]--it's really hard to argue with things that are happening in the future because either way you can't really prove they're happening or not right? And so I think that this was one of the examples of of pointing a finger--I mean this is actually trying to downplay future uh risks whereas currently we're seeing a kind of the opposite effect, but um but and any way you can't really say anything because it's in the future, so what are you going to argue with? Right so that that's what really pisses me off about it.
EMILY M. BENDER: Yeah, citations to the future, really not science.
EMMA STRUBELL: So yeah yeah and I feel like this paper is both--so part of it is sort of like setting the record straight maybe, uh because they were quite upset about um like estimates that I made using the information that I had available at the time. Then this gets back--well so this gets to um I think a point that Sasha and I have both liked to make which is that like transparency is important. And so this is like a wonderful example of like Google like not reporting--not disclosing various numbers and then so we tried to estimate these things, and then they were really upset about that. Um and so so part of the thing--so I actually I cite this paper um, and I cite it for some of these um like actual concrete estimates that they provide using their data. So that's actually like a valuable useful thing that does come out of this paper, um so uh yeah so so for example--and this is actually like--yeah I don't I don't know that I want to get into this but like um--they they wanted feedback from me like on this paper early on and kind of wanted me to be a part of this paper, and like one of the things that I asked them to do was just like report the numbers, like report the actual numbers so that people can like refer to this and use these estimates.
Um so I think that's sort of like what the first part of the paper is, um they're sort of talking about how they're like actually much more efficient than I don't know, like global estimates of data center efficiency and things like this and um some of their energy is renewable. Um.
SASHA LUCCIONI: Which is all true, right? To some extent.
EMMA STRUBELL: Yeah, I think--
ALEX HANNA: Yeah--
EMMA STRUBELL: So I have-- [crosstalk] Go ahead.
ALEX HANNA: Go ahead Emma.
EMMA STRUBELL: No no no, go ahead, I'm gonna sort of change topics so. [laughter]
ALEX HANNA: Well I--yeah I mean I also want to--for folks who are listening I mean that are not familiar with the paper, I mean I also want to just straight up give a shout out to your paper um uh uh with Ganesh and and McCallum, which is, "Energy and Policy Considerations for Deep Learning in NLP."
And I want to say I mean the kind of big things on this paper and and and you know--you were focusing on a number of Google models uh including I believe the the Vizier model, the kind of um neural architecture search model, which which which is the model that they describe that attempts to identify certain kinds of neural network architectures um that that are going to be the most um optimal for for prediction. And this paper I mean this paper got a lot of press, it got a lot of notice.
And especially because the findings were and and and I'm not going to summarize the findings correctly because I don't have the paper in front of me but it was a huge carbon cost, I mean the kind of you know some--training some of these models, one training run had something like the kind of lifetime um kind of like lifetime carbon emissions of of a car, or something and yeah and so it was--and it was the training one model. And so and these are estimates after doing very carefully trying to identify and you know they they were really hung up on this.
I mean it was also within Google, I mean we saw a lot of hang up on this. We even made sort of a joke that the kind of hangup that Jeff Dean had was on section three of Stochastic Parrots, and that was sort of the the sort of thing. And and to some degree I feel like this paper came out as a bit of um, and I'm stealing this this uh phrase from Meredith Whittaker, but a bit of a Potemkin citation, where we need a new citation to sort of point people to when people say that things are you know not or things are not energy efficient. So yeah that I wanted to put that out there and give a little background there.
EMMA STRUBELL: Thanks yeah, definitely um yeah I guess that's another thing that--like earlier drafts of this paper were just like--it was just like this like angry takedown of like basically, this--the paper [unintelligible] like our energy and policy considerations paper and then the Stochastic Parrots paper, that cited our paper in section three, um uh and it's still--I mean some of those aspects still remain, and I think contribute to the the fact that like the claims in the paper do not actually support the title. Like the very sort of, I don't know, extreme and drastic and like claim that we'd like to be true of the title, um because they basically sort of they take down how like actually wrong our estimate is of the carbon footprint of this one specific model, sort of like not addressing the fact that like many other super large models have been trained by them and at other places.
And you know Sasha and I have done work since then, like again like actually very accurately in collaboration with Microsoft for example like uh measuring--where we do have access to their internal sort of numbers, um--measuring the carbon footprint of training large models that have happened since then. And it's like it's very large, so it's like not--so just because our estimate was wrong about this specific sort of one thing at Google, that doesn't support their claim. Um. The-- [crosstalk]
SASHA LUCCIONI: Sorry I was just gonna say that uh people always confound energy and carbon. So maybe your carbon estimate--because the thing is for context, right, the the quantity of energy that you use is going to be pretty much the same if you use like the same hardware, um I mean or very similar hardware.
Like that's something you can compare apples to apples, where the push back often is is like oh well for example, 'Emily--sorry Emma, the experiments that she ran were on a different energy grid so the carbon was higher, whereas at Google it's renewable energy.' But it's like, okay fine that's that's possible, right. I mean numbers or it didn't happen. But also like, how about we compare the energy and in that case you know it's going to be a huge amount of energy. Even if it's renewable energy there's still so much of it right.
EMILY M. BENDER: And one thing we say in Stochastic Parrots is using renewable energy is great but we aren't to the point where there is more renewable energy than we actually need for like you know life necessities, right. So any renewable energy that's being used by data centers is not being used for something else.
SASHA LUCCIONI: Yeah and and often what what's--I mean sometimes it's actual renewable energy that's like built out, like an actual solar--uh solar panels or wind farms but usually what's actually done is um is what's called renewable energy credits, and that's like the the the accounting scheme that a lot of big tech companies set up. It's like they'll use whatever energy is available which is often coal or gas or or whatever you know whatever is--if you have a data center in Iowa you use the energy that's in Iowa and then you will buy equivalent amount of renewable energy in order to say that you're actually carbon neutral, but it doesn't like magically erase the emissions from the energy that you initially used, right it's just accounting it's just on paper that you can it can somehow be neutralized.
But it yeah so a lot of this is also based on this creative accounting situation.
EMILY M. BENDER: And it's not just carbon right, it's also water is an issue. So [laughter].
ALEX HANNA: They don't they--I don't think they they don't discuss--I mean I'd love to get into this paper a little bit, I don't think they discuss--they don't discuss water water in this paper.
EMILY M. BENDER: Not found.
ALEX HANNA: Um yeah yeah and it's um but I I would I--the thing that I mean the thing that grinds my gears about this paper, speaking about um transparency, is that they have these data um that I don't know if this data was available--it's this is Figure Two in the paper where they're kind of coming up with these um these sort of estimates of how much um um CFE's--so I think that's uh carbon carbon free energy, um they characterize I don't know how they defined this um metric.
But they sort of had these these kinds of metrics for each of these data centers. And this and this graph that's very hard to read because it's sort of all clocks, also Iowa is spelled "Lowa," uh which is interesting. [laughter] Um which I just I just noticed um um incredible uh. And so you know and they said--they have a citation to this but it's not dated, it's to sustainab– sustainability.Google/progress/energy, I believe when they put this on arXiv, like this the stuff just wasn't available.
It was just kind of--they were sort of having this per data center um CFE metric uh and they were sort of saying well if you kind of train this, you know in Iowa at like 6 a.m. then you know you're not having--you're not going to have this huge kind of carbon output. But it was like very particular sort of constraint that they're putting on this with kind of proprietary data and that just really sent me on this paper. So much.
EMILY M. BENDER: So I I have to share my enjoyment about what's going on in the chat here, so Abstract Tesseract says, "Lowa mission models, I'll see myself out." Arcane Sciences comes back with, "Iowa has split into Lowa and High-owa."
ALEX HANNA: Oh yeah, North and--North and South High-owa.
EMILY M. BENDER: So what what must have happened was it must have been actually a lowercase L, um and then someone went through and said oh we have to capitalize these things or something it, just didn't--
SASHA LUCCIONI: PDF parsing maybe, no?
EMILY M. BENDER: Who knows, ridiculous.
ALEX HANNA: Could be, yeah. I don't want to--I don't want to continue to dog on somebodyfor for for for typos and their and manuscripts because gosh what a nightmare, but humorous nonetheless.
EMILY M. BENDER: Yes, it's a good one, it's absolutely a good one. And maybe the typesetter, like if you aren't in the US, the name of the state Iowa maybe is less obvious. Um so it's just very funny to those of us who are familiar with the state of Iowa. All right is there anything else you want to do um in this paper in particular? I--my search isn't working on this so the water word water might show up. I am a little bit curious where they cite Stochastic Parrots which is citation 20.
ALEX HANNA: Citation 20, yeah down near the end. It's kind of a throwaway citation.
SASHA LUCCIONI: I thought this paper came out before Parrots, no?
EMILY M. BENDER: No.
ALEX HANNA: It came out after Parrots, it was kind of in response almost directly to your paper, Emma, and Stochastic Parrots because they needed basically something to--because people were citing Stochastic Parrots as sort of the climate carbon paper, but um so they really wanted to--yeah so it came out after, yeah.
EMILY M. BENDER: Yeah. Oh this is them just being sore so, uh, "The opening quote in this article is based on a 2019 project from the University of Massachusetts Amherst that estimated the environmental impact of training."
Um and that's the citation to your paper, Emma. "More than 1,250 papers site Strubell et al. as the source for the impact ML models have on carbon emissions--" Go Emma, you had a really impactful paper, it's important. "--including Bender et al., Freitag et al., Schwartz et al., Thompson et al., and Thompson et al. Uh the study calculated the energy consumed by the carbon footprint of the NAS by So et al. that led to the Evolved Transformer. Their estimate (they did not run the NAS code) was 284 t-CO-2-e--"
Tons of carbon equivalent? "--for NAS. The actual number was only 3.2, a factor of 88 smaller." And then they go into reasons why. And you know, I don't think that you in your paper claim to say we know how much it actually was. You say, 'we estimated it,' and in doing so you you know raise an extremely important issue that clearly was very very impactful. Um and--
EMMA STRUBELL: Yeah just--go ahead.
EMILY M. BENDER: No no, go ahead.
ES: I was just gonna say, not to be pedantic but we literally have a table, and it has like blanks in the table because we did not try to estimate the carbon emissions on TPUs because we didn't have like available information to do that. And so that--for example that's one of the differences but we were very clear in the paper that like we were estimating it if it was on this specific hardware with this specific carbon intensity, which maybe is not, right like we--you know.
EMILY M. BENDER: Yeah, yeah.
EMMA STRUBELL: Science.
EMILY M. BENDER: My takeaway was however big these things are precisely, they're big enough that we need to think about it. And like this is um--there's a wonderful paper paper that Batya Friedman and others wrote about the physicality of the cloud. Right, that we think of this stuff as just being off in abstract space but no it's actually material, and that matters. And you know your work, both of you, has sort of brought that into sharp relief, and for Google to come back and say oh no no no it's not that bad is just, you know, all kinds of problematic and condescending.
SASHA LUCCIONI: But also I mean we make these estimates because there is no information available. If if people were more transparent, fine, like you know just--actually got they've probably gotten worse since this paper was published. For a while they kind of did a couple of estimates, like they would they would actually say oh--I think Gopher or whatever they actually estimated the carbon footprint. But since then it's been a you know complete lack of transparency.
So it's like well, help us do our job or otherwise like we'll try to do our jobs and you won't like it, sorry.
EMILY M. BENDER: Yeah.
EMMA STRUBELL: Yeah. I have one last comment that I want to make about this paper, and um like why I think that their sort of argument doesn't support their claims. And it gets back to what Sasha was saying earlier about like it's really frustrating when a paper is just a bunch of estimates because like we can all make estimates, you can kind of shape estimates to sort of support whatever hypothesis you have. Um but in this case like--I mean for one thing there's like a lot of like economists and environmental scientists and other people who like their job and their research is to try to make these types of estimates um and um and there's um a thing--so and so so basically the other sort of main argument in this paper aside from the fact that like our specific estimate was wrong is also that generally like people are working on making machine learning more efficient and so therefore it is going to just use less energy in the future, and it'll be fine.
Um but they completely ignore like uh--it's a vast oversimplification of the problem um and so there's like a phenomenon known as Jevons's Paradox, which is basically um and it's been it's you know it's observed throughout economics and in other places, and it's basically the idea that when you reduce the cost of something, like it's uh the number of times that thing is performed is going to increase like because the access increases and things like that. Leading to like overall more of that thing happening.
And so there they sort of ignore that complexity um and they don't seem to be aware of that sort of phenomenon and that's just like one way in which um I think that their claim is sort of not really supported, um because I think as I think--what I think is going to happen and I suspect we are already seeing happen is that as--and I you know--as machine learning models become maybe more efficient and uh maybe hardware becomes more accessible or using these models and developing these models becomes more accessible, like probably--like you can imagine basically making the opposite like having the opposite thing happen, basically where the emissions actually increases, um.
EMILY M. BENDER: Yeah, has anyone estimated what's gone on with with ChatGPT and everybody using it to play and do their homework?
SASHA LUCCIONI: Yeah exactly.
EMMA STRUBELL: Sasha and I are very interested in doing that. We were just chatting about that in a meeting a couple weeks ago, uh sorry go ahead Sasha.
SASHA LUCCIONI: No but I was going to say that, I mean of course we don't have the numbers and this is always the debate, because it's like you know even when you do get some numbers about some data centers or you know you see some, like whatever the the emissions factors that are that are published in these ESG--ESG reports every year, it's always like, 'Well you don't know what percentage of that is due to AI usage.'
And so like every time I try to bring it up by like being like well you can't really argue that people are using AI less now, even if it's more efficient hypothetically. But people are like, 'Oh there's no numbers about that, right, like you can't actually prove that's the case.' And you're like okay well we're back to square one, right. Like why don't you publish the numbers that people are using AI less? And then right well we'll talk about it.
EMILY M. BENDER: Yeah. All right. Shall we move on to our um comic relief sort of interlude?
ALEX HANNA: Oh let's do it. And so we talked about this paper a few weeks ago in episode 12 right? And it was--
EMILY M. BENDER: 'It's All Hell' episode.
ALEX HANNA: It's--it was the our 'all Hell' episode, one of our most downloaded episodes. And this one's the pap--the title of this paper is, "The Carbon Emissions of Writing and Illustrating are Lower for AI than for Humans." And this is um on arXiV. Uh the authors are Bill Tomlinson, Rebecca Black, Donald Patterson, Andrew Torrance. A mix set of a few different universities, um--
EMILY M. BENDER: And Donald Patterson not to be confused with David Patterson.
SASHA LUCCIONI: I just gonna say.
EMILY M. BENDER: Yeah different people.
ALEX HANNA: These are different people. Related? Who knows. Um and so uh yeah so the um--this one we we trolled on a bit uh for the first figure of this, but just to read the the abstract: "As AI systems proliferate, their greenhouse gas emissions are increasingly--an increasingly important concern for human societies." Uh incredible sentence just to start off.
"We analyze the emissions for several AI systems (ChatGPT, BLOOM, DALL-E2, Midjourney) relative to those of humans completing these tasks--these same tasks. We find an AI writing a page of text emits 130 to 1500 times less CO2-e than a human doing it.
Similarly an AI creating an image emits 310 to 2900 times less. Emissions analysis do not account for social impacts such as professional displacement, legality--" Uh sorry, I don't know what that means. "--and rebound effects. In addition, AI is not a substitute for all human tasks. Nevertheless at present the use of AI holds the potential to carry out several major activities at much lower emission levels than humans."
Oof, okay. I just had to read--read that. I don't think I've actually read that out loud and--but it's what a doozy.
EMILY M. BENDER: Yeah. So--
SASHA LUCCIONI: What's interesting is that like on one hand I'm like okay people are thinking about this and that's kind of like part of why we do what we do, right. So sometimes I'm like okay it's great like people--and then it's like but they're they're not doing it properly, and so it's really frustrating because it's like you don't want to be super pedantic and being like you're doing a bad job, but you literally cannot compare humans and computers or or whatever models in terms of of of of emissions.
It just--it makes no sense right. And so it's like often the people who are like, 'Oh hey let's work on the carbon footprint, and let's like--' At some point um for one of the workshops I was re er--reviewing, for someone submitted a paper saying that they compared several like machine translation systems and then they concluded that Arabic was more polluting than French or whatever, and I'm like--
ALEX HANNA: Oh my gosh.
SASHA LUCCIONI: --that's not--that's not what--no. Like I mean ahh, you know. And then they were like well maybe it's because of blah blah and they went on this whole thing about why Arabic is like more intensive or whatever. And it's like on one hand you're like okay well people care somehow but on the other hand it's like but just do your homework.
ALEX HANNA: I--that that can you I I would love to see that paper, because you're you're not only--
SASHA LUCCIONI: I I blocked it but I think it was up on arXiV at some point yeah. Well I mean on the other hand, it's it's not it's not like it's not that complicated. They essentially like compare two machine or multiple machine translation systems and they found that for whatever reason, right the one that had like English to Arabic or Arabic to English was more intensive but could have been a multitude factors and like at the end of the day it doesn't freaking matter.
Like I mean why are you even doing this study? What are you trying to prove here, right?
EMILY M. BENDER: Right, so if you're measuring carbon impact is basically carbon impact of um you know, overall, this research that we're doing, is it to the level that we should be concerned? Right there's sort of that kind of a question. There's the um, okay I'm going to start a project, I've got a couple different ways of doing it. Which is the lower carbon impact way of doing that? Um and you know so on, sort of questions along those lines.
Not things like, well which languages should we translate because we want to translate the ones with less carbon impact. That makes no sense. And um well, 'the AIs can do this with less carbon than the people' makes no sense because people exist, right. [Laughter]
ALEX HANNA: Right.
EMILY M. BENDER: And and we're gonna keep existing and we should think about like how we move through the world and how we can reduce our carbon impact, but this paper just sets up the most ridiculous question.
ALEX HANNA: Yeah, the premise of which language is more cost-effective to translate I mean now you're just--now you're just introducing a whole new brand new kind of racism into it. 'Well Arabic is too costly to translate, so we don't need to you know--they don't need to be integrated into the world, but the French people the French are fine.' So it's just like new--new dimensions.
EMILY M. BENDER: I I can tell you with absolute certainly--certainty which language has had the biggest carbon impact across all of NLP.
SASHA LUCCIONI: English.
EMILY M. BENDER: Yeah, English, it's English. Although--
ALEX HANNA: 100 percent.
EMILY M. BENDER: --they don't tell you so, because in most of those papers they don't mention English so the data is kind of hidden, you know but--
ALEX HANNA: Bender rule alert, pew pew pew. We need like a soundboard every time the Bender rule comes up. [Laughter]
EMILY M. BENDER: All right, um do we have a ridiculous graph in here?
ALEX HANNA: Yeah, Figure 1.
SASHA LUCCIONI: Yeah that one, yeah the histogram.
ALEX HANNA: Yeah, yeah yeah.
SASHA LUCCIONI: Also like, "human from India," "human in the US," well I mean like honestly it's not where you're from that impacts how much carbon you emit. If you put a person from India in in the states, I mean uh-- [laughter] I mean it's not the person, it's the it's just the worst, just like oh.
ALEX HANNA: Right and just to describe this, this is the one--this is the chart we looked at in episode 12, where it's the histogram where it has uh a kind of a um log scale uh Y. axis and then X scale uh different categories. So, "BLOOM writing one page, ChatGBC--ChatGPT writing one page, laptop computer for the duration of human writing one page, desktop computer for the--" and then, "human from India writing one page," and "human from US." And it just goes up up and up and the the you know the the the the height is very close to one from BLOOM and ChatGPT and then for laptop, desktop it just is 10 100 1,000. So exponentially more carbon intensive.
EMILY M. BENDER: Yeah, also I don't think I noticed this last time, but um so we have BLOOM and we have ChatGPT, which are sort of on their own. And then there's the laptop computer and the desktop computer "for the duration of the human writing one page," so presumably these bars are added to or part of the bars that are for the humans? Like this is--
ALEX HANNA: Or does it mean by hand, it's it's not clear what the writing of one page--
EMILY M. BENDER: Yeah.
ALEX HANNA: --en--entails. I don't know if they talk about about this in the in the text.
EMILY M. BENDER: Right but I think we should probably actually get on to the other um well not so much comic relief paper at this point. But it seemed seemed worthwhile to dog on this paper a little bit more.
SASHA LUCCIONI: So why we why we came back to this right, it--kind of people started talking about it again recently uh because somebody retweeted it and it became a whole a whole discussion and people were like you know, 'See actually like AI is is better than--is more sustainable than huma--I don't know like, all these right.' And and then I spent like three days just trying to like quash all the rumors being like you you literally just can't compare humans and and and AI systems.
I mean like what portion of your carbon footprint would be even dedicated to whatever you're trying to measure, right? Like there's just so many holes in the in the in the comparison. But I mean I guess for me the the crux of the issue is is that we don't have this kind of um education or awareness right even in the AI community. Like these are people who know what AI is and how it works but it's just that like they don't necessarily have like the broader uh impacts or the broader um consequences. And so it's like they were just talking past each other in terms of um in terms of the carbon footprint, yeah."
EMILY M. BENDER: You say, 'someone' tweeted it. It was Yann LeCun wasn't it?
SASHA LUCCIONI: Oh I don't remember, I just remember it was--like I I debunked a lot of rumors. Like because people kept on like tagging me and being like, oh hey Sasha did you see this?
And I was like yeah I saw it and like I ended up writing like a bunch of tweets. So yeah he probably tweeted the initial one but then people like went on a on a whole um you know tangent about it, and I'm sure like Emma has encountered this with her recent paper. I mean her initial paper rather. Like people like ran with this idea of like the five cars in their lifetimes and then started being like well--like I remember people going to me being like well whatever work you do is actually just like screwing over the environment, because you saw this one paper and it's like people don't have the necessary context and we're not doing a very good job like as a community or multiple communities of like explaining the impacts of AI to people.
Like even people who do AI don't really get it, right, in a lot of cases and so and then they take this one one figure and they're like oh you ran a Random Forest on your laptop. Five cars in their lifetimes. And like well, not really.
ALEX HANNA: Yeah.
SASHA LUCCIONI: And it actually created a lot of tension--sorry I just like want to add one thing. So I am um part of Climate Change AI and like it created a lot of tension because people were like well all this like shit that you're selling essentially, that you're peddling is actually just like har--harmful more harmful but like most of the stuff that people do in terms of like climate modeling or you know whatever all the AI stuff that's done in the cl--climate AI space, like--well maybe recently it's become more LLM-oriented but for like years it was like literally like classifiers, Random Forest, SVM, and people were like even you know like doing better climate prediction or detecting you know like whatever deforestation or whatnot and they're like actually like positive applications and then they got all put in this under this umbrella, like any AI model you deploy is the equivalent of five cars in their lifetimes and that created a lot of like tension there too.
EMILY M. BENDER: Which I think is probably a good segue to this next one.
EMMA STRUBELL: I was gonna say I think that's like a perfect segue to the next paper, because I feel like one of the biggest issues here is like clear--I would say it's to me it seems like the author like does not understand that exact issue. Like just like does not understand the different phases of like the machine learning model life cycle, and like how they have very different like frequencies of use and also like magnitudes of emissions during those different phases, and they're like sort of conf using things um in making this very drastic estimate.
EMILY M. BENDER: So this paper is published in Joule, which is one of the Cell.com imprints and the title is, "The growing energy footprint of artificial intelligence. It's a commentary.
SASHA LUCCIONI: It's a commentary [laughter].
EMILY M. BENDER: Okay, so it's not a paper, it's a commentary.
SASHA LUCCIONI: It's not a paper it's a commentary, yeah.
EMILY M. BENDER: All right. "The growing energy footprint of artificial intelligence," written by someone named Alex de Vries, um who is a PhD candidate at the VU Amsterdam School of Business and Economics, and the founder of Digiconomist, "a research company dedicated to--" uh I can't read this because I've got an advertisement over it, "--the unintended consequences--" So 'exploring' probably, "the unintended consequences of digital trends. His research focuses on the environmental impact of emerging technologies and has played a major role in the global discussion regarding the sustainability of blockchain technology."
So sounds like heart in the right place, for sure um, and you know looking at things from the perspective of business and economics, which you know we welcome multiple perspectives, but apparently missing something important about the way I--the way so-called AI technology is actually used, so.
Um Emma is there a particular part of this text that we should go to to see that clearly?
EMMA STRUBELL: Um I think Sasha pointed this text out to me, so I don't know--
SASHA LUCCIONI: There's a couple of parts, but um well for example if you go to the plot we can talk about the plot, since it's kind of more uh visual. Yeah that one. So essentially on the plot it compares a Google search, a ChatGPT query, a BLOOM query uh--which is an open large language model--an AI-powered Google search query according to New State Research and the same thing according to SemiAnalysis. And essentially it shows--the plot shows that Google search is like a tiny quantity of K--of watt-hours per request and then goes ChatGPT then goes BLOOM, then AI-powered Google search one and then two, essentially. But the thing is except the BLOOM paper, none of those other figures are trustwor--I mean like they're all in inferred or guessed right because Google at no point has gone on record and been like, 'Every time you search Google, we chop down a tree,' right. Like they never did that so like none of those figures actually--and of course neither did OpenAI, 'Every time you ask ChatGPT to tell you a knock knock joke, like a fish dies.'
Right like they didn't do that. And so [laughter] it's like those commercials, it's like you know, 'Every time you use a paper straw, a turtle's gonna get it in its nose.' Um and it's kind of like that. And so it's like for me when you do plots like that because obviously that's most of what people are going to or or that estimate that AI is gonna what was it use as much energy as Switzerland or some--anyway people are going to focalize on that and you you have to signal that this is not real data you can't just use it in your prediction and then you know assume people read the fine print or or some footnote that you put somewhere.
ALEX HANNA: There was that one study or I don't know what it was, it was a headline, something like every query to ChatGPT was like dumping a bottle of water. And I don't know what the origin of that was--
SASHA LUCCIONI: So that's also uh I mean that's a very well-intentioned paper once again, but it also uses these um estimates and actually the proxy that they use for ChatGPT is BLOOM, my work, and I I take offense because you know, people will--I mean actually in the paper it the the the metaphor, like the the actual focus of the paper is ChatGPT and it's just so happens that the most recent comparable or not number is BLOOM, and so it's like you know using research that I've done that I put a lot of like--a lot of care and detail into for a pro--as a proxy for something that's completely like black books--black box and opaque and we have absolutely no idea how big it is, or where it's deployed or anything. So same thing with the with with the water paper.
It's similar. It uses figures as proxies for things that yeah are not exact--not necessarily the same, probably not the same at all actually.
EMILY M. BENDER: So the takeaway that I'm hearing here is on the one hand um we need the transparency, and on the other hand we shouldn't trust OpenAI or Google to be doing the right thing unless they are being transparent about it. So like being suspicious that they are being profligate with energy and water use is probably not wrong, but to to make specific claims about them is unfounded because they're the ones with the data and they haven't said. Is that a fair summary?
SASHA LUCCIONI: Yeah and I think it's counterproductive because um well for example like you know as soon as people start using these numbers, they really kind of don't need to say anything anymore because people are kind of like now using it. So I feel like you know that this 500 milliliters of water per query thing, for me I mean when I hear people talk about it they they take it as given.
And so now it's like well OpenAI has absolutely no reason--not that they would but have absolutely no reason to disclose anything, because people are already using this number, which may be a lot less than the actual number. And so it's like why would they even uh set the record straight on that right?
ALEX HANNA: I guess I have one one question for both of you and and it's a little not necessarily on this, but it's um in the absence of any kind of transparency or kind of minimal transparency, I mean what are what are--are there other ways to sort of infer these sorts of costs or I mean otherwise we're sort of guessing. And I know there's a lot of people either in the chat or listening who do work on kind of energy and AI or focusing on things like lithium mining or the mining of rare minerals or you know the the literal child labor and slavery that's um involved in the mining of these things.
So I mean what are the I mean what are the other sort of signals that folks can use to get a sense of just the cost of--yeah the environmental costs and the human cost.
SASHA LUCCIONI: Well I guess some of what now Emma and I are working on is is taking open source models and trying to at least give people ranges, but of course the fact that we don't even know neither the architecture nor the size nor anything about for example ChatGPT or or Bard or anything is--means that we can't even you know locate it on a on a range, right. If we're like well like the smallest model is this and then the biggest model is that, well ChatGPT could be even bigger, we don't really know.
Um but I do think that at least it helps us get some data points and some having some um orders of magnitude as well about like different tasks and different um setups and and also just like general education. Like for example when the LLaMA-1 paper came out um they actually did a--I think it was the 1--anyway a carbon footprint estimation and I think that either they didn't want to divulge or anyway they didn't want to say like where the model was trained, and so they did like a a hypothetical one, like 'If the model were trained here, this is what the carbon footprint would be.' Which works if you're not the person training the model, because you actually had-- like I mean Emma did it in her paper because she didn't have the information, right. But it doesn't work if you're the you have the numbers but you don't want to divulge the numbers.
And so I feel that there's so much just like misunderstanding about how this stuff is calculated, or or you know like what do you need to be transparent about for it to make sense and stuff like that that like any kind of work that that that we can do that to shed more light and even if it's just like super basic like even just like a blog post or like what are the factors right could really could really be helpful.
And I got into like a a massive Twitter fight with the one of the authors of the paper because I was like, this is not true, this is not how carbon footprint estimation works. You can't be like, 'Well I came here on a Hummer but I'm gonna give you the number from a Smartcar because that's what I chose to give you.' Right, like but then you literally can't do that, that's not how it works. You need to give the actual numbers um--and so and so yeah. But they they didn't change it.
EMILY M. BENDER: [laughter] 'The carbon foot from my travel, if I had gone by bicycle, would have been--'
SASHA LUCCIONI: Exactly yeah. There you go but I won't tell you what I came in but I'll give you this estimate. It was like it was like an US average estimate so it's like, 'Well if I came on an average car, but I'm not going to tell you what car I actually came in.'
ALEX HANNA: Oh my gosh.
EMMA STRUBELL: Yeah and I want to say one more thing like I think--and I think again Sasha and I also have sort of looked into this but then I think we both found is a little bit of a dead end in terms of um like any type of information that's available regarding water, regarding like rare earth minerals--
SASHA LUCCIONI: Embodied emissions, yeah.
EMMA STRUBELL: --embodied emissions. It's like it's so propri--it's even more proprietary and locked down I think than like the machine learning energy stuff. And so I feel like we're-- you know we focus on like the machine learning like operational um uh carbon footprint because it's like there like we should be able to do like just a fantastic job. And I think as people who are not necessarily at the companies or like despite the companies not wanting to disclose things, we can sort of like maybe start to do an estimate. But like looking at--you know Nvidia isn't telling us like how much palladium or whatever they're using, or like even if they're using palladium maybe like in their GPUs.
Um and similarly I think the amount of water is also sort of like a proprietary process thing, um and I think that th--those aspects are potentially like much worse. Particularly like the sort of human rights aspects of like where rare earth minerals come from, and like other just even not rare earth like mining operations and things. But I think you need people like on the ground doing like investigation of that stuff, um because like no one is reporting that.
SASHA LUCCIONI: And and and right now it's kind of like what I've heard as well is like well we know that this is so ridiculous--'so we know how bad the numbers are from like the actual usage, so we're sure that the manufacturing emissions are less than that, so we're not going to share them because it doesn't really matter, because usage is like so much more intensive than than manufacturing.' You're like but like how are we supposed to actually measure that unless you actually give us numbers right? Like you really can't and we know that like manufacturing GPUs is actually a super--it's like wired ran this article about like these these fabs, like the fab like where they actually make the the GPUs and it's like they use ultra pure water because they have to like clean each wafer, and there's like a bunch of wafers stacked and it's like a lot of energy and the energy is is coal-powered because they're in Taiwan.
I mean there's all these like different uh parts of the puzzle and at the end of the day, they're just like, 'We're not going to tell you the environmental footprint because it doesn't matter, yay.' Right?
EMILY M. BENDER: So to tie this to today's um executive order that came out of the White House, um one of the things that's in there is uh Biden's directing the NSF to do sort of a start--like trial run of the the what they're calling the NAIRR, the National AI Research Resource um and it seems to me that if the um if the White House and the executive branch is trying to like lead by example with transparency, then we could say look you know NAIRR is going to need to be transparent about, you know, what's the energy source. Um anybody who's doing experiments on this needs to report these numbers about how much they used, um maybe some things about you know where did these chips come from?
Where were they produced? Like sort of like trying to see how far we can push on transparency there could be really valuable.
SASHA LUCCIONI: The thing is is that the companies like for example Nvidia and others, they're US-based. But most of uh the fabs are actually not in the states and I think that that actually scares--I mean because of this whole catastrophic risk situation. I mean like for example Taiwan has like most of the factories right and it's right near China and people are anyway--so there's that whole like geopolitical thing and I think think that as a consequence of this panic, they're trying to bring back chip manufacturing to the states which is obviously going to be more expensive and the companies obviously don't want to do it, but with like maybe as like a indirect impact of this of this whole existential risk thing, maybe we're going to have local production of of of GPUs for example and there's going to be a more transparent--like for example if the factory's in California, there's legislation right, there's all these like specific legislations in the states so they're they're going to have to share something, which in Taiwan is not the case just because it's it's not the same know laws right.
EMILY M. BENDER: Yeah.
ALEX HANNA: Yeah and if for folks who are interested, AI Now put out a great explainer on kind of compute in AI and the kind of supply chains of chips and kind of how yeah most of it resides with one manufacturer, the Taiwan Semiconductor Manufacturing Company um and so there's--they're worried about the supply chain risk and this involved--and so this has been you know an effort to build factories or build chip factories in the United States, um uh to kind of avoid that. But it's just that you have to really really change the supply chains to even make that a thing.
EMILY M. BENDER: I so wish we'd had this conversation before I was at that uh US House subcommittee hearing a couple weeks ago because one of the people on the committee is this congressman from Indiana who said, 'We've got a new chip fab opening up in my district and I want all four of you witnesses to answer this question: how's AI gonna help us make better chips?' And all four of us kind of went uh, what do we say about that um and I think I managed to say something about like environmental impact, but I sure wish I could have said hey let's have some transparency about these things, like that would have been a really great moment to bring it up. So next time I'll be more prepared.
SASHA LUCCIONI: The thing is is though that like I'm sure Nvidia uses AI to some extent in their design right, but they don't really I mean I don't--for example recently I saw that they're really emphasizing more um efficient usage chips, like like chips that would require less energy to run without--like so I've defin--they've published about that, but they've never published about like less intensive manufacturing of ch--of chips which would which would make more sense right, because of--
ALEX HANNA: Right.
EMILY M. BENDER: All right we gotta we gotta keep moving if we're gonna get to the Fresh AI Hell. I just want to sort of show this uh headline. So um from the New York Times reporting on that not-paper commentary that we were just reading, um, "AI Could Soon Need as Much Electricity as an Entire Country," and I guess this is just like a word to the wise to anybody who is publishing in academic venues, anything touching AI at all is like media magnet right now. So choose your words carefully um and like you know you it it's true that the author of the commentary did not have control over this New York Times headline but we need to be I think a little bit defensive in how we write about things.
SASHA LUCCIONI: I think this article was meant to be a somewhat of a headline grabbing article though, I mean I I think the fact that these comparisons were made--like this once again these are these are predictions, right. Back to my my my it's hard to debunk predictions, because you can't really say either way. And so I think that like part of the part of the this point the point of this article was to generate these kinds of headlines so.
EMILY M. BENDER: Yeah all right so transitioning to Fresh AI Hell. Every time I give Alex a little improv prompt. This time Alex, you are a weather forecaster um describing an upcoming climate catastrophe, as the climate crisis is in fact reaching AI Hell. So you are the AI Hell weather forecaster talking about the whatever climate crisis it is, climate crisis-spawned disaster that's coming your way.
ALEX HANNA: Got it. Uh my name is uh Jim Cantori, I'm over here at Cape Hatteras North Carolina and I've got a weather coat on. And uh I I really uh I I have to tell you I've been in a lot of storms but never never seen this. We see a typhoon coming in, uh Fresh AI Hell, is coming down, fire and brimstone. Uh smoking pieces of GPUs are are are have been hitting major buildings here, uh please uh take shelter uh crawl under a table um put in earphones so you can avoid all kinds of AI Hell and all kinds of bullshit that you're seeing, um that's that's all I've got to say--we we've got to go offline now. That's all I got.
EMILY M. BENDER: Stay--stay safe there! [laughter] Okay here we go thank you for that. Um we've got a little bit of Fresh AI Hell. The first thing is a Wall Street Journal article that I have via LinkedIn because I don't have a Wall Street Journal account. Um so uh headline is, "Americans are asking AI, 'Should I get back with my ex?'" and then the text of the post is, "Should you get back with your ex? New dating apps are employing artificial intelligence to analyze compatibility or beef up your banter. Your results with them may vary."
ALEX HANNA: Jeez. And I mean we I think we were talking about this article offline and that--I mean so what you actually do here is you upload your texts with your ex, um and then it's it's making some assessment of this. So not to mention that you know your ex is is not consenting to this, um and then to having their messages uploaded to this, and then just whatever the privacy policy on this is, you know, terrible.
So yeah, and it actually reminds me of this song um uh by I think the artist's name is ma--Malikana? Anyway, it's called, "I Wish I Missed My Ex," and it makes me think of this every time I see this headline.
EMILY M. BENDER: All right and this 'spice up your banter' also reminds me of the the folks who wanted to like basically put--revive Google Glass and connect it to GPT-4. We have we have a previous Fresh AI Hell segment about that where it's like yeah not creepy at all, that's really gonna make you a popular date.
ALEX HANNA: Well it's like--is wasn't like is wasn't there one where it was it was like that don't we do an AI Hell where it was like where it was like you wear on a date and it tells you what to say?
EMILY M. BENDER: Yes yes.
ALEX HANNA: Yeah.
SASHA LUCCIONI: Oh yeah, I remember that.
ALEX HANNA: Yeah. Good Lord.
EMILY M. BENDER: Emma, you were gonna say?
EMMA STRUBELL: Uh I mean yeah I've never been in a Fresh AI Hell--or an AI Hell segment but um like the ethical concerns here are like insane though actually right? Like so there's the privacy issue but like you know as natural language people, I feel like quite confident that like NLP technology, including ChatGPT like is not going to detect like gaslighting, and like not going to detect honestly like abuse and stuff right, and so like I feel like if you're actually using this you could easily have situations where the AI is saying like, 'Yeah, your ex seems like really nice, they did all this stuff for you,' like uh and then it's actually like a really toxic situation, uh.
SASHA LUCCIONI: It's like the the Sydney chatbot saying that the guy should divorce his wife, right.
EMILY M. BENDER: Right, except set up as, 'take a--relationship advice from this thing.' All right, Ragin' Reptar in the chat says, "How long until Netflix makes a dating show where one of the Bachelors is an AI?"
ALEX HANNA: Oh God--it's don't give them ideas. They're gonna do it.
EMILY M. BENDER: No no no speculative AI Hell, we have enough actual AI Hell.
ALEX HANNA: I know, stop.
EMMA STRUBELL: I'm convinced that Netflix's show that was like, renovating sex rooms, I don't remember what it was called but it was like about--
ALEX HANNA: Yeah.
EMMA STRUBELL: I'm convinced that was like generated by an AI, because I feel like it was optimized for viewing, like I totally get it.
SASHA LUCCIONI: I thought you were gonna mention the one where it's like the people were dressed in like animal or like alien costumes, and yeah like how could you possibly?
ALEX HANNA: Like I feel like I--sorry no go ahead, I was just going to mention love--what is whatever what was the one? Queer Love, the one that was people're really toxic and they swap partners. Anyways sorry.
EMILY M. BENDER: All right, so to transition to our next one here, Abstract Tesseract says, "From now on I'll be asking prospective suitors to name all the countries starting with 'K'" Which is a call back to the ChatGPT fail that leaked through Google. But also sort of a new Turing test replacement, which brings us to this Financial Times opinion piece, um "We need a political Alan Turing to design AI safeguards." Um and, "Frontier technology is a challenge that raises a spectrum of concerns," by John Thornhill.
And there's just so much wrong with this, we're just gonna have be really fast. But the two things that I want to say is, can we cut it out with the great man theory? Like we don't need a single person who's going to solve all the problems, we need a broad collaborative um like sort of working session where people are actually represented.
But also there's somewhere in here where they talk about how--yes. "Whereas the electromechanical device that Turing built could perform just one code cracking function well, today's frontier AI models are approaching the quote 'universal computers' he could only imagine, capable of vastly more function." It's like no, we have those universal computers, and LLMs are an example of a piece of software that runs on top of them.
ALEX HANNA: Yeah, we now call them 'computers.'
EMILY M. BENDER: Yeah, and it's true that the code cracking stuff that was going on at Bletchley Park was a specific-function computer right, special purpose, um but that doesn't mean that like it's only now with the LLMs that can tell us answers to any question that we're actually getting to the universal computers. Like what a misunderstanding of what's going on. Um so anyway next, I don't want to spend too much time on this one because I want to get to a little bit more AI Hell.
ALEX HANNA: Oh gosh, yeah, let me read--I can read this one. So this is Wes--Wes Gurnee, the author of this paper says, "Do language models have an internal world model? A sense of time? At multiple spacio--at multiple spatiotemporal scales? In a new paper with Mark Tegmark--" Is it Mark Tegmark?
SASHA LUCCIONI: Max.
ALEX HANNA: Max Max Tegmark, yeah thank you. "--we provide evidence that they do, by finding a literal map of the world inside the activations of LaMDA-2." And there's a gif of the activation layers mapping to different parts of the world. Oh gosh.
EMILY M. BENDER: It's like okay, so it is not surprising that the distribution of named entities in text would cluster based on where in the world they're from, right. You are probably talking about politicians from the US in papers where you're talking about other politicians from the US and so on, right. That does not make a world model, number one. Number two, the most striking thing about this is just how thin the data is in the Global South, right. So if you look at when as it resolves into its thing, if you look at what happens in Latin America and Africa, it's like oh there's hardly any dots there because the text they're basing this on doesn't talk about those places, um. But yeah so um.
Now to get to something truly awful, uh 404 Media--shout out 404 Media, 404 Media is not awful, they're wonderful. This is a recent um new media thing that started by a couple folks from Vice.
ALEX HANNA: Yep.
EMILY M. BENDER: Headline here, "4chan Uses Bing to Flood the Internet With Racist Images," because of course they're doing that, right. Um and so we've got uh, "4chan users are coordinating a posting campaign where they use Bing's AI text-to-image generator to create racist images that they can then post across the internet. The news shows how users are able to manipulate free-to-access, easy to use AI tools to quickly flood the internet with racist garbage, even when those tools are allegedly strictly moderated."
ALEX HANNA: Oh yeah this one this one's pretty pretty awful, yeah, just having a coordinated campaign at supercharging this. Things that people are warning about, you're going to have more and more of these kinds of things, people are going to use this and it's going to supercharge this kind of hateful content.
EMILY M. BENDER: Yeah. And it's not like all of this is carbon impact free. Like just you know. [laughter]
EMMA STRUBELL: [sarcastic] Sasha maybe our next project should be--let's like estimate the carbon footprint of like 4chan users generating racist content, and we can compare like is it more efficient for like the user to generate the racist content, or for like the AI to generate the racist content?
ALEX HANNA: You're--y'all are really bringing new definitions to environmental racism. [laughter]
EMILY M. BENDER: All right, on a similar note um here's Vice uh--stupid advertisement making so I can't see. Vice Motherboard: "Generative AI is a disaster and companies don't seem to really care. In their push for AI-generated content tech companies are dancing on the edge between fucking around and finding out." Illustrated--
SASHA LUCCIONI: I love--this is the best reporter ever, she reached out to me when she was writing this article and I'm like--
ALEX HANNA: Yeah this is Janus--Janus Rose, who's--shout-out Janus Rose, super cool. Also great--a great DJ, and also Jordan Pearson.
EMILY M. BENDER: And here we have a picture of Mickey Mouse in what what's--
ALEX HANNA: Army fatigues. [crosstalk]
EMILY M. BENDER: Except he's also wearing like a brown pair of pants and a belt. Um wait--do you see--what did you just say there Sasha?
SASHA LUCCIONI: Holding a Kalashnikov?
EMILY M. BENDER: That's what that is, okay. Um yeah some sort of very fierce looking weapon with his typical Mickey Mouse smile on his face, um and yeah so this is like, it's really easy apparently to create these things. All right, one last one. What's this? Oh okay. "The final 11 seconds of a fatal Tesla Autopilot crash."
Um so this is a Washington Post thing where they've reconstructed what happens. Um I guess there was a little bit of video and so then they reconstructed from there. Um this is the Washington Post and the thing that I wanted to to raise up out of this aside from the fact that it's really tragic, um is they talk about--they ask the question of like who's responsible um and they say um so, "Banner's family sued after the gruesome 2019 collision, one of at least 10 active lawsuits involving Tesla's autopilot, several which are expected to go to court over the next year. Together the cases could determine whether the driver is solely responsible when things go wrong in a vehicle guided by autopilot or whether the software should also bear some of the blame."
And the thing that got to me about this is no, software can't bear blame, it's the company that's producing and selling the software. And I was just so infuriated to see the Washington Post, who generally do a better than average job in this space, like talking about it that way. All right, I feel like we could have ordered that better because it was really fun the way you all were we're just riffing right now and now we're on this bummer thing, but we are actually at time. And so I'm going to take us um to the outro.
Thank you for joining us in AI Hell. That's it for this week. Dr. Emma Strubell is an assistant professor in the Language Technologies Institute in Carnegie Melon University's School of Computer Science. And Dr. Sasha Luccioni is a researcher in ethical and sustainable artificial intelligence at the company Hugging Face and a founding member of Climate Change AI. Thank you both for coming in and joining us today.
EMMA STRUBELL: Thanks for inviting us, thanks for hosting.
ALEX HANNA: Thank you so much. Our theme song is by Toby Menon, graphic design by Naomi Pleasure-Park, production by Christie Taylor and thanks as always to the Distributed AI Research Institute. If you like this show you can support us by rating and reviewing us on Apple podcast and Spotify, and by donating to DAIR at DAIR-Institute.org. That's D-A-I-R hyphen Institute dot org.
EMILY M. BENDER: Find us and all our past episodes on PeerTube and wherever you get your podcasts. You can watch and comment on the show while it's happening live on our twitch stream. That's twitch.TV/DAIR_Institute. Again that's D-A-I-R underscore Institute. I'm Emily M. Bender.
ALEX HANNA: And I'm Alex Hanna. Stay out of AI Hell, y'all, including all the incoming storm fronts.