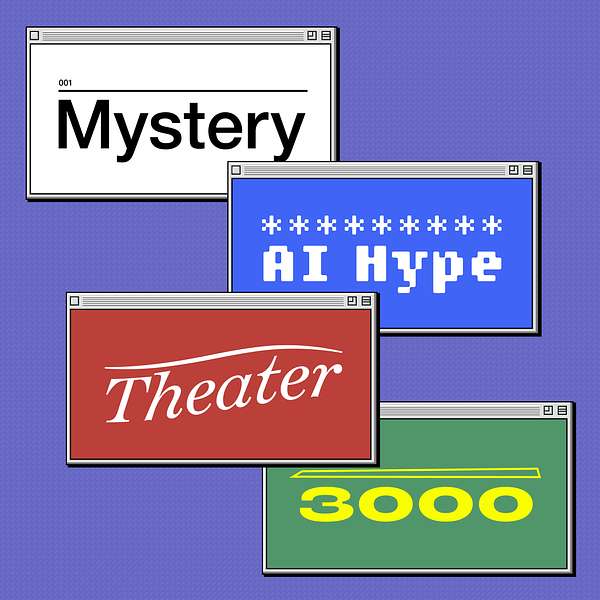
Mystery AI Hype Theater 3000
Mystery AI Hype Theater 3000
Episode 24: AI Won't Solve Structural Inequality (feat. Kerry McInerney & Eleanor Drage), January 8 2024
New year, same Bullshit Mountain. Alex and Emily are joined by feminist technosolutionism critics Eleanor Drage and Kerry McInerney to tear down the ways AI is proposed as a solution to structural inequality, including racism, ableism, and sexism -- and why this hype can occlude the need for more meaningful changes in institutions.
Dr. Eleanor Drage is a Senior Research Fellow at the Leverhulme Centre for the Future of Intelligence. Dr. Kerry McInerney is a Research Fellow at the Leverhulme Centre for the Future of Intelligence and a Research Fellow at the AI Now Institute. Together they host The Good Robot, a podcast about gender, feminism, and whether technology can be "good" in either outcomes or processes.
Watch the video version of this episode on PeerTube.
References:
HireVue promo: How Innovative Hiring Technology Nurtures Diversity, Equity, and Inclusion
Algorithm Watch: The [German Federal Asylum Agency]'s controversial dialect recognition software: new languages and an EU pilot project
Want to see how AI might be processing video of your face during a job interview? Play with React App, a tool that Eleanor helped develop to critique AI-powered video interview tools and the 'personality insights' they offer.
Philosophy & Technology: Does AI Debias Recruitment? Race, Gender, and AI’s “Eradication of Difference” (Drage & McInerney, 2022)
Communication and Critical/Cultural Studies: Copies without an original: the performativity of biometric bordering technologies (Drage & Frabetti, 2023)
Fresh AI Hell
Internet of Shit 2.0: a "smart" bidet
Fake AI “students” enrolled at Michigan University
Synthetic images destroy online crochet groups
“AI” for teacher performance feedback
Palette cleanser: “Stochastic parrot” is the American Dialect Society’s AI-related word of the year for 2023!
Check out future streams at on Twitch, Meanwhile, send us any AI Hell you see.
Our book, 'The AI Con,' comes out in May! Pre-order now.
Subscribe to our newsletter via Buttondown.
Follow us!
Emily
- Bluesky: emilymbender.bsky.social
- Mastodon: dair-community.social/@EmilyMBender
Alex
- Bluesky: alexhanna.bsky.social
- Mastodon: dair-community.social/@alex
- Twitter: @alexhanna
Music by Toby Menon.
Artwork by Naomi Pleasure-Park.
Production by Christie Taylor.
Alex Hanna: Welcome everyone to Mystery AI Hype Theater 3000, where we seek catharsis in this age of AI hype. We find the worst of it and pop it with the sharpest needles we can find.
Emily M. Bender: Along the way, we learn to always read the footnotes. And each time we think we've reached peak AI hype, the summit of Bullshit Mountain, we discover there's worse to come.
I'm Emily M. Bender, Professor of Linguistics at the University of Washington.
Alex Hanna: I'm Alex Hanna, Director of Research for the Distributed AI Research Institute. This is episode 24, which we're recording on January 8th, 2024. Kind of a fitting number for our first episode of the New Year. And today we're going to be talking about structural inequality and all the ways people are hyping quote AI as a solution to diversity problems around the world.
Emily M. Bender: From language and dialect recognition algorithms used for asylum seekers to new hiring tools that claim not to magnify bias but instead minimize it, um, which we'll see, um, and our guests today are experts in how all this hype is just another facet of the scourge of technosolutionism.
Alex Hanna: And they're the co hosts of the excellent podcast, The Good Robot, a feminist podcast that explores the limits of quote, good technology and asks us to reimagine what technology can be and how it can be made.
Dr. Eleanor Drage is a senior research fellow at the Lever-- Leverhulme Center for the Future of Intelligence based in London. Welcome Eleanor.
Eleanor Drage: Hi, thanks for having me.
Emily M. Bender: Thanks for joining us. And Dr. Kerry McInerney is a research fellow at the Leverhulme Center for the Future of Intelligence, as well as a research fellow for the AI Now Institute.
She also joins us from London. Welcome, Kerry.
Kerry McInerney: Hi, thanks so much for having me.
Emily M. Bender: We are super excited to have you two on. Your show is amazing. Your insights are amazing. Um, so let's get right to it. I am going to share our first artifact here, um, which might be cued up to the second artifact, but we will see.
Um, this is the one that I wanted to do first. This is the, all the pop ups, the HireVue website's promotional page. It says how innovative hiring technology nurtures diversity, equity, and inclusion. Those are some pretty nice words. Uh, what are we going to find here? Any, any, um, sort of framing that you want to give?
Um, let's say we'll start with Eleanor.
Eleanor Drage: Oh, I was hoping-- Kerry always starts. So, but I'm, I know, so we're, we're a team that begins with Kerry and kind of ends with me. I sort of pick up the pieces usually. We wrote this paper, um, in exactly that way. So I think that, um, I was looking at how ideas about colorblindness that have been debunked a while ago, although still in acting, you get colorblind casting, um, and how those ideas were being brought to life again in these, um, video AI technologies.
So we're specifically looking at video AI hiring systems. And we also didn't want to say that they were racist or sexist per se, but just that they really misunderstood what race and gender are and how they figure in the hiring process. So maybe Kerry, you can tell us a little bit more about how we went about trying to work out what these companies are trying to do, particularly because their algorithms are proprietary.
And so we were really looking at marketing materials. And I really enjoyed this because my first career was in digital marketing. So I was writing a lot of this crap back in the day and I was not very good at it. So, um, I really enjoyed looking at the kinds of assumptions that were being made, um, and how they're being pitched to consumers.
And, and also recently. Um, I should say a lot of the engineers I talked to about these kinds of hiring tools get really upset that when they make tools, they don't make any of these claims themselves. I think the engineers often are like, we do not claim to de-bias hiring. We just claim to, um, we're just trying to create a system that maps perceptions of personality onto different candidates in the hiring process.
And those claims are then being made by the PR people, by the people like me in digital marketing, um, by sales and by CEOs. So there's this mismatch between what engineers know about how a system works and then also, um, how they're being pitched so that they can become successful and scale and gain market share.
Go on, Kerry.
Kerry McInerney: So what Eleanor is saying is she was the original Mystery Hype Theater back in the day. Uh, but the reason why we wanted to look at this page by HireVue is for those of you who are listening, uh, so you might not be able to see the page we have in front of us. Uh, so HireVue is a kind of one of the biggest firms in AI powered hiring and recruitment.
Those technologies can take a wide range of forms. It could be everything from CV scanners through to kind of automated chat bots on hiring websites, uh, through to tools, which you can use to, for example, rephrase a job advert to make it more inclusive. So say to take out certain kinds of gendered words or gender pronouns.
Um, but as Eleanor mentioned, you know, we are interested in a particular kind of hiring tool, which is video powered interviewing. Um, but the HireVue page that you have here in front of you, I think is really emblematic of one of the big selling points of these AI powered hiring tools, because, um, AI hiring has become a really popular buzzword.
There's a lot of hype around it for probably a lot of the same reasons that you've discussed on different episodes of this show, there's this idea that AI will help, you know, streamline recruitment. It will save people time. It will bring us closer to this sort of perfectly automated world. And those things are in and of themselves quite attractive to a lot of people working in HR because they're really time stretched.
It's often a super underappreciated role and they're just looking for a way to make their lives a bit easier. Um, but what's really interesting about these tools in particular is they make another claim beyond that. So saying beyond making your life faster and more efficient, we're going to solve these deeply entrenched structural problems around sexism, racism, and other kinds of, um, discrimination and hiring, because we can not only make your hiring faster, we can also make it fairer.
And that's again, super attractive because these are teams that are often underfunded. They're not given maybe enough resources or support to meaningfully enact different kinds of diversity, equality, and inclusion policies, you know, the big structural changes that might actually be needed. So they think, Hey, this is a win win and it makes our organization look really techie because they're bringing in an AI powered tool.
Uh, and so that's why I think this high view page is really, uh, emblematic of all those different selling points. But as we kind of want to go on and talk about, can these tools actually do the things that they're claiming they say they do on the tin, make things faster and fairer, I'm personally not convinced.
Emily M. Bender: Yeah. So this, this page starts with, um, "DEI, diversity, equity, and inclusion are good for both society and business. And it all starts with innovative hiring practices," which sounds like some of that PR garbage that you were talking about, Eleanor. And what's interesting is that they go into this McKinsey study saying that, uh, companies with more racial diversity in leadership "are more likely to have above average financial returns."
So basically it's good for business. And I'm reading this thinking. Just how diverse is HireVue itself? Like if they're out there peddling this stuff, have they actually created an inclusive environment in, for their own workforce? I didn't go and look, but I really doubt it.
Eleanor Drage: I would say this is--
Alex Hanna: [Crosstalk]
Yeah, go ahead.
Go ahead, Eleanor.
Eleanor Drage: I would say that, you know, for these kinds of tools, I also spent a lot of time listening to podcasts and interviews by the people who, um, whose idea it was to start these kinds of tools. So all these aspects of tools, um, that dealt with diversity and inclusion. And I remember listening to the CEO of Sensia, which is one of these, a similar tool that does video AI, hiring.
Um, and, you know, she really meant well, and she really did want to solve this issue. This was the kind of primary reason, right? Why she started doing video AI hiring was in order to turn humans into neutral data points. Um, and while we know that this kind of perpetuates this myth of sameness and that we can all become um, that underneath it all, we are all just human and that race and gender are these, um, superficial things that are layered on.
She really, she didn't know that. You know, I'm, we're lucky that we have been taught these things, that we've sat through feminist courses about bell hooks, that we've experienced the reality of what this is. But what it really sung out to me is that there's a severe lack of education around these ideas, but also that you shouldn't be able to create AI tools that deal with race and gender without having some sort of substantive knowledge about them.
Um, because while, you know, lots of these people are well intentioned, and I don't doubt that that that is a part of why they do this. I don't think that they're trying to, um, con people. I don't think that they're specifically trying to perpetuate racism through this ideology of colorblindness. Um, but it's not, it's not good enough anyway.
We still need to make sure that, um, that people are educated in this way before they're allowed to create tools that claim to be able to do this.
Emily M. Bender: Yeah,
Alex Hanna: I think something that's helpful to reiterate too, and you've mentioned colorblindness a few times and just to, you know, for, for listeners, you know, um, you know, it'd be helpful to define that idea and kind of what that is, because I think I'm thinking a lot about kind of works of critical race theorists like Eduardo Bonilla-Silva and, you know, his work on racism without racists and, and kind of the way that Racism is much more than erasing kind of these traces of race and then magically you have a deraced type of structure.
No, those elements of racism are really well structured within hiring itself and employment itself and just moving kinds of things. And I think that's kind of the promise of these tools, especially in this idea of de biasing. And so down in this text, they, they talk about this in job descriptions. And so there's a subhead in which they say "Innovating Job Descriptions: When it comes to job listing response rates those with gender--" quote
I'm, I'm, I'm quoting myself "--gender neutral wording get 42 percent more responses." Um, and then they they say "Sentiment analysis software has the ability to analyze, copy and determine potential biases at play." Which seems questionable to start with. "AI driven tool, AI driven," uh, inexplicable comma "tools such as MonkeyLearn, Lexalytics and Brandwatch are programmed to deliver detailed analysis of copy that could be misconstrued based on gender, race, or even sexual orientation." Just an amazing comment there that there's sentiment analysis tools that could give kind of you know, queer vibes or something.
Emily M. Bender: So there's a different approach to that, that I think actually is sensible. And that's what Textio is doing.
So Textio set up a service where, um, they sort of said, we're going to figure out how to reword job ads so that you get more diverse candidate pools. And they did that by providing a service to companies so that they saw the results. So that that's an enormous set of data where they've got job ads and then who applied for it.
And so they just look for correlations between um, words like, so support, understand, affectionate, being gendered as feminine, and aggressive, ambitious, being gendered as masculine, sounds like the kind of result that would come out of their work. And it's not sentiment analysis. It's just correlation of like, okay, if you describe it this way, who do you get?
If you describe it that way, who do you get? Um, and, and that's--
Eleanor Drage: That's a part of the application of AI because, you know, and that the FT do use this, by the way. So I was on a panel with, um, head of diversity inclusion at the Financial Times. And she said she was using, um, AI to point out human biases. AI is great at that. It's great at pointing things out, but it's not great at eradicating difference because that's impossible.
And I think one of the key things that when I was working with second year computer scientists at Cambridge who, um, were helping me replicate one of these tools and see whether it worked or not. Um, one of the things they were most upset about was that recruiters might want to erase part of them. You want to be loved and looked at generously by recruiters. You want to be part of a company that isn't trying to hire you based on closing their eyes. But because they want all of you, and they want to grow and incorporate you in a way that will make you happy as well as them happy.
So that involves cultural change, it involves remedying the gender pay gap, it means solving childcare issues in the workplace, getting rid of breakfast meetings, all these things that, you know, Kerry and I have avoided by working in academia. You know, the breakfast meeting. Or, um, you know, but these are the things that that are actually important when it comes to making workforces more diverse and good recruiters know this and we talked to loads of recruiters who were really suspicious of these tools and they were saying, you know, why didn't they come to us and say, what do you need as an AI tool that will help you speed up your process or help you make your process more effective rather than trying to change the process altogether by creating a system doesn't align at all with your values or your ways of working.
Alex Hanna: Yeah. And I think that's a really good thing to highlight, especially in the hiring aspect of it. I mean, so many organizations say, you know, you want to bring, we want you to bring your whole self to work. And yet, you know, the kind of hiring aspect of it is saying, well, we're going to kind of de race, de bias, de gender, you know, all these types of things.
And somehow, kind of waving magically around, you're going to get a more diverse talent pool, and you're going to have people that come from a variety of backgrounds coming to work. Um, it makes me think a little bit about, uh, thinking as you as you talk about policy, Eleanor, and things like breakfast meetings, which is, I haven't heard of, and sounds nightmarish.
I don't want to have a meeting over breakfast. I just want to have my coffee and stew and think about the state of the world before having to face everything. But it makes me think a little bit about Victor Ray's concept of the racialized organization in the way that um, different organizations, um, because of how they're structured, have a very particular view on, on race and it is not articulated.
It's a, you know, and it's a default to whiteness because of that's the way that, that, that these organizations work. And there are ways of having organizations that support different racial projects, but you'd have to radically rethink the organization.
Kerry McInerney: And, you know, I think that's such an important point.
And actually, Alex, I know you wrote a really fantastic piece about sort of thinking about structural whiteness and big tech specifically and then big tech organizations. And because I think part of the rationale of these hiring tools is they say, well, the easiest way to identify something like racism and to eradicate it is as you said, uh, in thinking about De Silva's work is just to erase race entirely from the conversation. Because if we don't have race, we don't have racism. But while, you know, we might see companies getting potentially, you know, again, jury's a bit out on this, better at engaging with these like very overt, very direct acts of racism where race is named and made very explicit.
There's so many different kinds of implicit ways, which I'm sure many of us have experienced in relation to gender and race and sexual orientation and many other attributes of ourselves where, you know, organizations have just been not created in ways that are hospitable to or fit with people who don't subscribe to this very narrow kind of white male heterosexual norm.
So like to give an example, um, you know, this can range from everything, say, in my job in an educational institution, um, everything from, say, oh, you know, um, if I celebrate, say, like, the Lunar New Year, that can be a very difficult thing to try to explain to an employer, like, oh, I need these days off, even though they're, say, in the university term, all the way through to kind of more extreme examples.
Like for example, during COVID, I remember trying to think about, okay, like I'm, for example, because of this sort of rise in anti Chinese and anti Asian racism, like I'm a bit anxious or nervous about walking to work or this kind of now being put these barriers in place, but how do you even like begin to have that conversation in the workplace?
Because that's not really something you're equipped to do with people who maybe aren't sharing that experience. And it's just something that they might not even have on their radar. And those are like, you know, from my kind of work experience, quite sort of minor examples. But I think what happens is you do see these kinds of things sort of building and codifying throughout the course of an institution and its lifetime.
And that means, um, when you're starting to recruit people into the organization, let alone once they're actually inside. And notice that's something none of these AI tools deal with, which is, you know, what's the point of bringing people magically in somehow if you're going to then drop them in the lion's den.
Um, you know, and so I think when you're dealing with complex problems like that, you're just not going to be able to do it with a tool that somehow claims to be able to toggle race or toggle gender on and off, which is what some of these tools do.
Emily M. Bender: Yeah, absolutely. And going back to the, this, this thing that we're still looking at on the screen here about, um, making the ad copy for the jobs more appealing to a more diverse base of candidates.
That's a slightly different thing. Like this is a, this is a silly way to describe it. And I don't know these tools, but it's a different thing because that is the company saying, how are we describing ourselves? How are we you know, and it might be a veneer, right? So if, if companies are using the Textio service to get more diverse candidates and then they managed to hire them, but then they don't change anything actually on the inside, then it's not going to help, right.
Um, but it's at least one minor step towards looking at company culture and the way that space is, is um, if not constructed, projected, um, that I think is, is a more sensible way to go about this than as you're saying, basically saying, well, we're just going to go color blind, gender blind, and so on.
I want to share a story of a, of a time where I think things went really well, um, about this, like, changing of frame that can help.
So I have, um, I'm in linguistics at UW and we are the home of the, um, American Sign Language program. So we have three deaf colleagues, um, and only a couple of the hearing folks in the department are proficient signers in American Sign Language. Um, I am a dilettante novice signer in American Sign Language, like I occasionally have managed to have a conversation that goes beyond hello, how are you and I'm proud of myself, but I have a couple colleagues who are hearing and sign well.
So we have interpreters at our faculty meetings, and a few years ago one day the interpreters didn't show up. And the thing that felt like an enormous success in that moment was that the entire group of faculty, hearing and deaf, related to the issue as we collectively have a communication problem to solve here because we collectively need to find a way around the fact that we're missing these interpreters.
Um, and that ended up being a combination of, um, me doing transcription, um, for the, uh, speaking, um, the, the audio stuff and, um, then my deaf colleagues were actually writing and then having a hearing colleague voice for them. So it worked out, but the thing that was really lovely was it wasn't, you know, oh no, our deaf colleagues have a problem.
It's like, no, we collectively have a problem. And I think that that kind of a reframing is what's needed in so many of these places. Like, how do we make this a space where we set it up so it's not just a space for the default person.
Kerry McInerney: I mean, I think that's just such a wonderful story. And I think it's such a powerful exemplar of like why we need to think about issues to do with access, to do with inclusion as kind of collective responsibilities, but also why trying to outsource these things to technologies is particularly doomed to fail.
Because you know, what's happening with, I think, some of these hiring tools and with other AI applications that are very technosolutionist is the complete opposite of that story. It's saying instead of us all saying, 'Actually, we're equally responsible for building a corporate or an institutional culture that genuinely allows people to be as seen or as hidden as they want to be,' it's saying, 'You know what, now it's the technology's problem and now it's the tools' problem. It's not my problem.' And again, I want to be really generous as to like why people buy in these tools. It's because they're very time stretched, have not been resourced well enough to try and bring about those cultural changes, but it doesn't change the fact, which is, you know, you're kind of just worsening a problem by removing accountability altogether.
And, you know, I think you can tell from the document on the screen, like who these products are for, like, they're not for the candidates. They're not for people who are minoritized and experience discrimination in companies. They are, um, just to quote directly from here, um, "By using technology to proactively minimize these biases, companies are able to not only avoid discrimination lawsuits," which is put as the first and most important thing, "but also better recruit competitive, diverse talent."
And I get that this is a marketing brief, which is going to be, you know, directed towards corporate institutions who are interested in purchasing these technologies, but I think just, you know, it's amazing to me how overt it is that this really isn't about improving candidate experience or employee experience.
Like this is about how companies can protect themselves from accusations of discrimination. And that, that to me is pretty disheartening.
Emily M. Bender: Yeah. So we've been talking about technosolutionism. I'm gonna be fast. Alex. Um, you've both brought up technosolutionism and how the, the people who really actually would like a problem solved or not consulted.
And to me, that feels really emblematic of technosolutionism, where the people proposing the technology are also claiming the right to define the problem and declare it solved.
Alex Hanna: Yeah. And one thing I wanted to mention is really harping on that, both what you said, Emily and Kerry, is that this marketing copy is written, you know, as kind of the solution to diversity, but there's this whole kind of way in which candidates have, you know, really taking to comporting themselves in these video interviews, right?
Where it really is, ucm, this kind of process of having to, um, really control their faces and control their body language and comport themselves in a particular sort of way. So, you know, you have this hyper vigilance about one's self and one's body and one's body comportment that, you know, you of course have to do in any kind of professional setting, but when you have a tool that is making some assessment of your, whatever, trustworthiness or your, um, your, your, your, um, uh, you know, reposed intelligence.
I don't, I don't know. HireVue has a lot of these terrible sort of metrics, but it does force you to enact a sort of type of professionalism that is then reinforced by these tools.
Eleanor Drage: Yeah, absolutely. And we all know that if you go to a fee paying school, often you get courses or classes or training in how to perform in these interviews, you know, not just what to say, but how to say it, how to phrase things, how to sit, where to look, what to wear.
Um, there's this, I, and one of--there's two anecdotes I heard about, um, about interviews that, I found really interesting and slightly shocking. And one of them was someone who went for an interview at the BBC. And these kinds of interviews are a bit odd because you neeccd to be casual, kind of TV, but not super casual.
You know, you don't want to put your feet up on the table, you know, when you're waiting for the job, but also you don't want to wear a suit either. And there's that kind of tricky balance you have to strike in how you respond to questions and how personable you are, um, you know, how you kind of meld well with a crew.
And that idea of culture fit, um, has of course been debunked and hiring, you know, as a kind of dirty term now, although you do still see it on these kinds of websites that we were looking at really explicitly. And so they're still looking at micro gestures as they call it how you tilt your head, the way that you say things and those things are racialized and gendered and classed. And so we really need to understand that better.
I think we just need to face up to things rather than just turn away. And they also, um, and you, both of you being tech people will be able to explain this better, but they're looking for, um, different hobbies that correspond with their idea of a perfect, perfect candidate, different ways of expressing themselves, different keywords, um, that correspond to their idea of what, what an ideal employee would, would be or look like.
I once heard a friend who went for an oil and gas interview, um, and they, having known nothing about it, but they asked him very quickly early on in the process, whether he went skiing. Um, and he was like, no, I've never skied before. And he didn't get the job, which I think he was pretty happy about, you know, just trying different things.
But this idea that, you know, you have a hobby that corresponds with other people's hobbies, um, is a kind of gross thing now, but it still happens, just AI is doing it instead. So we need to be really careful that we're not just doing the same thing as we used to do, just having a technology doing it on our behalf.
Emily M. Bender: Yeah, math's-washing it.
Alex Hanna: Yeah, I would, I would say there, there was that one thing that I forgot if it was an Amazon tool, but I think they were, it was, it was the word, but it was 'lacrosse.' It was, if they had, you know, we're in the lacrosse, if they're had done lacrosse in university and it had a pretty high correlation with either maleness or, or, or, or as a class thing.
Um, any case.
Emily M. Bender: Yeah.
Eleanor Drage: In the States, isn't that really aggressive? And you like take your stick and, you know, it's like you really hit people with it. It's quite like a hardcore sport.
Alex Hanna: No, lacrosse, lacrosse is fascinating too because it's a kind of a bastardization of the indigenous stick ball. Right. And I mean, it's, and so it's, um, But I don't know enough about those lineages to speak with any kind of authority.
Emily M. Bender: It's associated with the sort of most prestigious, most expensive high schools and post secondary, is the thing in the US. So, and all of this is basically taking correlations in some training data set, which is going to be embedding lots and lots of biases, and then calling it AI and saying it's actually understanding and doing more.
And one thing that really jumped out at me in HireVue's copy here is under the heading "Casting a Wider Talent Net," it says "One specific way in which HireVue technology reaches more diverse candidates is by offering video interviews in 30 plus languages, making it easy for candidates to thrive during the hiring process, no matter their language of origin."
And I thought, what are they actually measuring in these interviews? Um, that they claim that this could work across all these different languages. And, you know, it's, it's probably. just random correlations that, that, and then they're packaging as if the system is listening to what people are saying. Um, and the other thing that is missing here and that I would advise anybody who's thinking about purchasing one of these services to look into is any information on how it was developed and how it was tested, right?
If you're, whenever you're looking at automation, you want to check and make sure that the evaluation, the validation of it was something that matches your use case and there's nothing here.
Kerry McInerney: Yeah. And if I could just add onto that, I think that's hugely important. I think this, this part about the language is also just very baffling to me because, you know, it's also, I think raises this question of where are these technologies being developed?
Because the location of where they're being developed really matters. And where are they being deployed? Because, you know, if you think about something like that, I look at a lot, which is racial categorization, how candidates are maybe self identifying, how they're being processed by these tools, that's going to look hugely different in the United States compared to say, well, I'm from Aotearoa, New Zealand.
Our racial categories and the labels that we use to self identify are really, really different. So there's like a huge number of issues that you might say, okay, well, when we tested on this US data set, people with different racial or ethnic self identifications were treated fairly, but then what's, what's the point of that data, if you're then going to deploy the tool somewhere else, like New Zealand, where you know, that data set just doesn't apply anymore. And I think too often that jump, um, is taken up or these tools are applied globally when they're actually really, really culturally, socially, politically, historically specific.
Yeah. I want to lift up something from the chat here from, um, we get the, so, uh, Style Loaf, um, I love the usernames in the chat.
Style Loaf says, "Really glad to hear ableism being discussed. So important, especially as we continue to be in an ongoing pandemic that is doubling as an under discussed eugenics project in the U. S. and U. K." Um, and I'm also being alerted that you can hear Euler purring on the mic. My apologies for that.
But I, I want to sort of bring that ableism issue around here because if you're talking about video, um, and you're talking about, um, you know, let's say neurodiversity, people might not make eye contact in the same way. Somebody who has a stutter is going to be evaluated very differently by one of these tools.
Somebody who, um, you know, maybe is hard of hearing, but, um, you know, still uses spoken language, they're going to sound different and they are not going to be evaluated I mean, nobody's being evaluated fairly by this, right? The thing is that it's, it's, it's fake, um, it's fake technology, right? That, that's taking input and giving output, but not in a way that matches what it's being sold as doing, but it is going to be particularly harmful, I think, to people with various disabilities that affect how they present themselves and how they're perceived.
Alex Hanna: And the one thing in the copy that says this is, you know, they, they say, "HireVue's data scientists, for example, work alongside a team of industrial organizational psychologists to ensure valid bias mitigated assessments that help to enhance human decision making while promoting diversity and equal opportunity."
And that's a pretty huge claim. First 'bias mitigated' doesn't have, you know, much of an agreed definition and second. If this is the case, then show us your, you know, show us your model cards, show us your, show us your, your work, show us your, um, actual transparency and what's happening here instead of going ahead and saying, just trust us.
So. Yeah.
Emily M. Bender: All right, I think it's time for us to move to our second argument.
Eleanor Drage: Just very quickly before, I'm sure Kerry has something to say about this as well, but what I, I was always a bit afraid of giving definitions of race, gender, and disability when giving these presentations. And then actually at the FT, I was like, nah, just go for it.
And just for, you know, both, for companies to know that disability is something that is produced through disabling infrastructures is so important, but also for them to be, you know, acquainted with ideas about race as the product of racism and not vice versa as Paul Gilroy sees it or gender is something that is citationally produced and then embodied.
You can explain those in ways that are actually quite simple, um, and that are really understandable. So that's one thing. And then the second thing is opting out and consent. So important and so easy to do, I think, but it does involve HireVue, for example, talking to the client and saying, how are you enabling people to opt out if they're uncomfortable and how do they know for sure that they will not be discriminated against based on their opting out and doing it a different way. And the having the security to know that, okay, I can choose not to use this system and I can use a human and I won't be, you know, marked down or something, that is so important because you're in such a bad position as a job seeker.
You know, you really need this job. You're really worried that they'll think worse of you if you don't use their like normal system. Um, and it has to be okay to not do that and to be reassured of that and to have a process in place. And you know, there's no, there's lots of kind of exposes that have been done around disability in relation to AI hiring tools.
Um, we don't know for sure what the, what the experience is, and I don't think even HireVue can tell you, um, you know, how disability relates in its many different forms to its video hiring tools. But just as an initial building block, the ability to opt out and to know for certain that you're not going to be downgraded because you want to do that.
It's so important.
Emily M. Bender: Yeah, super, super important. And, and I think opt out on one level is easy, but on another level really does require commitment on the side of the company. And then the sort of a clear, um, articulation of that commitment in a way that actually comes through so that people don't say, oh, but, but surely they're, they're just saying that because they have to.
So I'm still going to go with this uncomfortable thing.
Eleanor Drage: If they don't show the commitment, then at least, you know, that you're going to have an employer that doesn't care about you. So in some ways that can be, you know, quite indicative of your experience at the organization later on.
Alex Hanna: Right.
Emily M. Bender: Yeah.
Alex Hanna: All right.
Shall we move on?
Emily M. Bender: Yes. So our next artifact is not itself a hype artifact, but it is talking about something really awful. And, and thank you again, um, to our guests for bringing this to our attention. So "The BAMF's. controversial dialect recognition software: new languages, and an EU pilot project." That's the headline.
This comes from a publication called Algorithm Watch. Um, and the author here is Josephine Lulamae. Um, and, uh, well, um, so Kerry do you want to take us into this one and tell us what we're looking at?
Kerry McInerney: Yes, sure.
So this is an example that actually came from a paper and research study that Eleanor did with Dr. Federica Frabetti, who is a lecturer at the University of Roehampton, thinking about different kinds of border control technologies that are AI enabled or AI powered, and how they reproduce kind of these much older histories of structural discrimination, particularly around racism and sexism, around many other things, though, as well, and how new technologies are being used to reproduce and entrench these histories of border control.
Um, and you know, I think that this particular application of AI is especially concerning to me as someone, you know, who not only is very interested in, um, the relationship between AI and border control as someone who did, you know, all my PhD research was specifically on immigration detention and thinking about these new carceral regimes of, of border control and why they are so damaging.
Um, but also as someone, yeah, who personally migrated to the UK when I was 18. My family immigrated from various parts of the world. Uh, and so I think for many of us who have histories of migration of travel, um, of experiencing kind of in very lived and bodily ways, these forms of control and checking, the way that these technologies are now increasingly being rolled out and deployed, um, is incredibly concerning.
Um, and so the particular artifact we've put here, I'll leave Eleanor to go more into this sort of technical details, um, is a tool that, um. supposedly can identify where someone has come from based on their voice on their dialect and, you know, I'll leave Eleanor to claim whether or not we think it can actually do that.
But the reason why, like, I see this tool being very much part of kind of a broader issue to do with hype and trust is because um, this idea of trust and truth plays such a central role in different asylum processes. So I know much more about the UK case than about the German case, but it is this constant battle between, um, asylum seekers who are constantly disbelieved, constantly portrayed as liars, and constantly having to make impossible truths visible to border control authorities.
And, um, this, this kind of application of new technologies, I think it's just a further hurdle that asylum seekers have to face. So again, that's not saying, um, you know, that these tools are sort of creating a new problem, rather that they, I think, are exacerbating an existing issue, which is this sort of, sort of, these impossibilities of proof when it comes to trying to make your case towards a hostile asylum system.
Alex Hanna: And before we get into this, it's helpful just to read this and I'll go ahead and read the first two paragraphs.
So the article says, "For several years, Germany has been the only country to use automated language and dialect recognition in asylum procedures, purportedly to help authorities verify a person's claims about where they are from. According to the privacy advocacy group European Digital Rights, this is a technology in line with AI claiming to predict someone's sexual orientation or political beliefs and should be banned."
Um, and then, uh, "Now an inquiry by MP Clara, uh, Bünger reveals that since July, 2022, the software is being used to recognize Farsi, Dari, and Pashto. And in addition to Iraqi Arabic, Maghrebi Arabic, Levantine Arabic, Gulf Arabic, and Egyptian Arabic. Not everyone is convinced by this new development. The American computational linguist Mark Liberman, while emphasizing he's no expert on Persian languages, he told us he is skeptical about training a machine class--to classify spoken Farsi, Dari, and Pashto." And then Lieberman is director of the Linguistic Data Consortium.
Emily M. Bender: That's actually a really interesting detail there. So the LDC is one of the original clearinghouses for linguistic data for linguistic and computational linguistic research. But this parenthetical says, "Liberman is the director of the Linguistic Data Consortium at the University of Pennsylvania, where the German Federal, German Federal Asylum Agency or BAMF gets the majority of the training data for its software."
And so the person who's like in charge of the organization providing that training is like, no, this is, this is not how this is to be used.
Doesn't make sense.
Alex Hanna: Yeah. Doesn't work. It's really interesting too.
And I mean, this is, this is an aside, but I mean, there's a bunch of things about kind of. organizations like the LDC organizations that have repositories of, of, of data for computational study going on saying, mmm, this is not meant to do any kind of forecasting and prediction and why are you doing this?
Um, anyways, that's for another episode. Sorry, go ahead. I know, I know Kerry called onto Eleanor, so I'll, I'll pass it on.
Eleanor Drage: Yeah. These have been amazing introductions to this paper, which is really about a piece of software that is a combination of many different kinds of technologies. And I don't think we said this in relation to the hiring technologies before, but it's not just one thing.
It's a cluster of lots of different kinds of systems, and they can be clustered together through the shared assumption that, for example, personality can be spotted on a person using AI, or that the point of origin of a migrant can be spotted through their voice. And what is really important about this, um, about why this technology doesn't work is exactly what Kerry said.
It's not a truth unless it corresponds with what the system believes the world looks like. So you have to create a story every time you fill in a visa application or every time you say who you are or where you come from, you're doing it in a way that fits with the way that border control sees the world.
And it doesn't matter whether it's strictly true or not. Or it strictly aligns with your experience of, of your childhood, of the way that you moved, of migration patterns. Um, but it must correspond with how border control sees the world. And so what we were trying to prove, Federica and I, was that these kinds of systems performatively produce citizenship. Authentic citizenship.
And by performatively produced, we mean quite simply that instead of just identifying or recognizing a truthful migrant, and here you can see this politics of suspicion, right? It actually produces the truthful migrant through the technology that is being used. And I'm really indebted to the work of Pedro Oliveira, who's this fantastic sound engineer that I met at the AI Anarchies conference in Berlin.
And he is um, uses different kinds of sound technologies to, um, really kind of take the piss out of these, these technologies by saying, where is the point of origin in the voice? Is it in the tone, is it in the frequency? How do you pull apart the voice and then piece it together in a way that makes sense to border control?
It's a ridiculous idea. And when I was writing this, I was living with a friend who is an opera singer, and they were trying to make their voice sound as authentic as possible. Um, there's a way of singing called bel canto, and it is basically, um, a way of retraining the voice to sound very natural. And what that kind of taught me was that it's about perceptions of naturalness.
It's about sitting in the audience at a concert and saying that voice sounds like a natural voice to me. Of course, it's not natural. It's been trained. It's been taught to sing in a particular way. So it's all about the hear, the hearing person, the listener. It's not about the person speaking at all. So how we how we take in migrants at border control has everything to do with our own ideologies, our own, um, preconceptions about what authentic, um, uh, migration stories sound like, and really nothing to do with the people themselves.
And that is so dehumanizing. That is, that is the horror, really, um, of these kinds of technologies.
Alex Hanna: Yeah, I really appreciate this. That's such a amazing way of putting it that the voice is a site of producing citizenship, right? And it makes me think a little bit of, uh, Dorothy Santos's work, um, her particular work, uh, The Cyborg's Prosody, which is, um, kind of, uh, flipping this idea of, of, um, accent reduction, um, in her work, particularly with, with, with, with this kind of cottage industry of especially Filipino workers who staff call centers and there's these bevy of accent reduction apps so they can respond to the kind of global demand for for, for, for, for tech support and phone-based tech support. And it kind of turns it on this head where there's this app and there's this training where then there's these different stories where they're listening in English um, with a reader as a, I'm reading from, from her site, who has a Filipino accident, but, but an accent.
And then the last one requires a person to, um, um, repeat excerpts of the story told in Tagalog. Um, and so it's sort of like, how are you actually forcing someone to produce a kind of fromness or an origin. And I love this thing you said, Eleanor, where in the voice is where you're from located.
Emily M. Bender: Yeah. This is for me, um, resonating. I was just at the Linguistics Society of America annual meeting and, um, got to hear some from, um, Nicole Holliday, who works on what does it mean to sound Black in the US. Um, and she does this as, um, uh, like acoustic analysis, but also perception studies. And it is very much on the listener side. What is it about voices that people will use to make certain social judgments?
And all of those are really interesting questions. And they have no place in these life and death decisions that are faced by people who are coming from just terrible experiences, only to be further dehumanized.
Kerry McInerney: Absolutely. And I think something that comes out of both of these technologies, but of this case in particular, is this idea that, you know, something like the voice is always the same and there can be somehow this kind of essential truth about someone when we know, you know, that these are things that change throughout your life.
We know age changes your voice,you know very fundamentally, we know say some kind of vocal trauma might change your voice fundamentally but also we code switch, we speak in very different ways in you know different settings. We pick up--some people pick up accents wherever they go around the world, if they've traveled and moved a lot.
Some people don't. My brother and I have lived across the world in different places. He has a very strong Kiwi accent. My accent is not very strong. We just have different levels of, you know, absorption when it comes to voices. But yet, you know, what these tools do is they kind of recodify these ideas that something about us is--has to be this biologically essentialist trait that itself is indicative of other things about us that can never change. And so to me, there's something fundamentally very racializing and very racist as well about this idea of always trying to push you back to your point of origin. Because it's a little bit to me like the technological version of saying, but where are you really from?
Which is one of those questions, which again, if you're an immigrant, you get quite a lot and it's never, you know, it can be well intended. It tends to not be received particularly well. Um, because it's this idea of, you know, oh, are you saying I'm not from here? Even though I was born in this place or even though I've lived here for a long time, um, and it's something that I think, you know, again, a lot of people of color and minorities experience in any sort of white majority society is people kind of trying to relocate you in a way.
And it doesn't give you much authority over your own story, over who you are, to kind of come back to this point about being dehumanizing, like, it's a difficult experience. And that's just on the kind of everyday level. Imagine just how much more terrifying that is when, you know, your life or death decision about your asylum is literally dependent on whether or not you've managed to code switch successfully into a voice that a border guard or the border understands as being authentic.
Like it's a hugely distressing experience.
Emily M. Bender: Yeah. And you have to be both legible to that border guard. And authentic at the same time, which might be a completely impossible thing. All right. I'm going to take us over to Fresh AI Hell, uh, Alex, you okay for a musical cue this time?
Alex Hanna: Oh, yes. Uh, let me, we, we had a mention of opera earlier, so...
Emily M. Bender: Yes, I was going to go for opera if that's okay.
Alex Hanna: Oh, no, no, don't, don't, don't opera me, don't, don't opera me.
Emily M. Bender: Musical style of your choice. This time you are a data worker and your job is labeling news stories as Fresh AI Hell or not. And you are passing the time by singing about them as they come by.
Alex Hanna: Interesting, interesting. Um, oh gosh, okay. [Singing][ This hell, that hell, this hell, that hell, this hell, that hell.
This one detects origin based on voice, that's hell. This one tells us whether chat GPT can be used in schools, that's hell. [Speaking] I'm just thinking of like a Willy Wonka style, you know, I, I, if I was, if there was a visual element of this, I'd be dressed in lederhosen that was green and paint my face orange.
Emily M. Bender: I love it. Thank you so much. One of my highlights the podcast every time is trying to come up with something bizarre and then Alex hits it out of the park. All right.
Alex Hanna: It's, it's, it's, it's a pers--it's my personal bane of my existence, but I love it anyway.
Emily M. Bender: Yeah. And then you got me back in our fresh, in our all-Hell episode at the end of the year.
So didn't make me sing though. I refuse on that.
All right, so our first element of six in the segment this time, um, from Chalkbeats New York, which I guess is a, uh, school focused publication, um, the title is, "Can artificial intelligence help teachers improve? A network of New York City schools wants to find out."
This was published on January 2nd of this year by Michael Elsen-Rooney, um, and basically what's going on here is, um, so, "Urban Assembly, a network of 21 schools, is working with the American Institutes of Research to develop an AI powered tool that can help instructional coaches analyze videos of teachers delivering lessons and offer feedback according to network leaders."
So, more surveillance tech, basically recording what's going on in the classroom, and then handing that data to yet another party, the instructional coaches who are then using it to help the teachers become better teachers, I guess. And they, yeah.
Alex Hanna: This is a means of, you know, already being in a place where teachers are you know, surveilled and mandated to do even more and more. Um, I mean, having something in the classroom that's going to surveil you and then also passing it off to a third party to take that content and do something with that is, is just awful. And I'm, I'm imagining these public high schools aren't unionized if they were, I mean, they would--this is a provision to push back against those.
Emily M. Bender: One, one would hope. All right. I'm going to keep us moving cause we've got a lot that I want to get to here and this one um, yeah, Alex, you want to, you want to present this one?
Alex Hanna: Sure.
So this one is, yeah, this is a sad one.
So this is a tweet thread by Dr. Laura Robinson, who says, "Man--" and she tweeted this at the end of the year, last day of the year, December 31st. Uh, "Man, I gotta say, I'm sure other people are getting something out of this, but all my direct contact with AI has been so depressing. For, for example, I used to be part of a bunch of crochet groups and boards on social media and I've quit them all because they are now 90 percent people posting AI art that's obviously not a photo of a real crochet project and everyone's praise everyone praising them for it because they can't tell the difference. It's so annoying It feels so feels like so much creativity has gone out of the world.
The next photo, "Like what the hell is this?" And it's like these three princess type crochet looking things. Um, and she says "Like, what the hell is this? This is obviously not really yarn. And honestly, it depresses that you could have touched yarn in your life and think this is real."
Is there any, is there anything next in this thread as well?
Emily M. Bender: Yeah, it goes a bit longer.
Alex Hanna: Um, yeah, yeah, yeah. Um, and I think these are, these are helpful. Um, you used to be, "You used to be able to, and maybe there, there still are some have spaces, which--where people would share art and patterns and tutorials and try to improve together.
But if all the, um, amigurumi uh, is this hyper glossy too pair--too perfect AI art and people stop sharing their real art because they can't compete. People who realize that the board is entirely faked now stop participating. A lot of these photos lead to for-pay patterns that are also created by AI or just stolen from actual artists that won't even approximate what's in the photo, so scams, and that the whole board just becomes another social media content mill that doesn't attract anything other than likes or clicks because there's no actual person behind the dark--art to lead you or show you how they did it."
And then, "*Plinkett voice* Now everything sucks."
So yeah, just another kind of, uh place where These tools are being used basically to churn and optimize for engagement in certain places where that actually can be monetized like, you know, Twitter Blue or like Facebook pages, etc. So really sad when craft pages turn into this.
Emily M. Bender: Yeah,
Eleanor Drage: [Unintelligible] an amazing crocheter.
So.
Kerry McInerney: I was going to say, I was like, that makes me so sad. I've actually not come across this though. So I'm not going to like keep an eye out for it, but I like, and how that in that picture, not only are that, uh, amigurumi, like, you know, not very realistic looking, but the bottom one has actual hair, like human hair.
Alex Hanna: Oh yeah.
Kerry McInerney: Compared with the others, uh, it's quite a dark take on, on crochet that's been generated.
Emily M. Bender: Oof, yeah. All right.
Eleanor Drage: Would this Improve with AI literacy. Or are we doomed to be sending people, I mean, Kerry just sends me her crochet images. Maybe that's just the way it's going to head.
Emily M. Bender: Yeah, maybe we're going to go back to local things.
Okay, I'm going to take us really quickly through the next couple here. Um, so, uh, Ferris State University, this is in the Holland Sentinel, headline, "Students' classmates at this Michigan university could be AI." And, at Ferris State University in Michigan, they have created two virtual students to "enroll" in quotes in classes in the spring semester.
The students are named Anne and Fry, and they will, in quotes, "enroll," quotes are mine, "as freshmen, and be part of hybrid classes where they'll interact with classmates and complete assignments." And apparently this is some kind of, like, study of how to make something, but this is, this is for research of some kind.
It's like, where's your IRB? You are subjecting all of these classmates to, yeah. Alright, uh, next, from the literal internet of shit files.
Alex Hanna: Oh yeah, so this is from The Verge, uh, the title being, "Enjoy talking to your new voice controlled smart bidet. Kohler's PureWash E930 bidet seat helps smarten up your existing toilet by adding new sprays, app connectivity, and voice control."
This is by Victoria Song, uh, and yeah, it's a picture of a toilet. Um, "For most people, flushing $10,000 down the drain for an entirely smart toilet is a bit too much, but if you always wanted a fancy toilet, Kohler's kicking off CES 2024 with a more accessible option." Uh, oh yeah, and it says it's--they've had, [unintelligible] ... highlighted this bit where it says the difference is that it has Amazon Alexa and Google Home compatibility.
Emily M. Bender: So you have your choice of sending your bidet usage data to either Amazon or Google.
Alex Hanna: Yeah, right.
Kerry McInerney: But also, what if someone hacks it and then gains control over your bidet functionality? It's like, that to me is a serious, like, security risk.
Emily M. Bender: Apparently it's got remote control. Anyway, this is nonsense. Okay.
Eleanor Drage: Also, random bidet facts, um, it means 'pony' in early French. Maybe you know this, Alex, which is weird, but also they were originally popular in Catholic countries, which is messed up. No one wants to know why. Um, but yeah, I love a bidet and I would have definitely been one of those like 18th century and 19th century Americans being like, please, to my husband, bring me a bidet back from France on your travels.
Emily M. Bender: Yeah, no, bidets are fabulous. They do not need to be hooked up to the internet.
Eleanor Drage: No, they do not, not bidets, definitely.
Emily M. Bender: So people watching saw me just drop something out. It's really funny, but it needs time to be read aloud. And I wanted to save time for this one last thing because it's particularly timely. I just got back from the Linguistic Society of America annual meeting, which is held jointly with the American Dialect Society's annual meeting.
And the American Dialect Society does the original Word of the Year. They've been at it since 1990 and the voting for the word of the year happens live in the meeting and you get to like advocate for and against certain words and it's loads and loads of fun.
And the, under the, the antidote to Fresh AI Hell here, I want to, um, brag that, um, they have special categories. So there's the overall word of the year, but then there's specific ones. And there was an ad hoc category this year for AI related word of the year. And though I'm very sad that we needed such a category, I'm quite proud that what won was 'stochastic parrot.'
Alex Hanna: [cheering] Amazing.
Emily M. Bender: And it was loads of fun to be there at the ADS meeting saying, I can speak to the origin of this phrase. Like it was the first time that the coiner of the phrase was in the room to talk about it. So that was, that was really cool.
Alex Hanna: I do love some of the other things around here, like acronym of the year, fuck around and find out, "FAFO," that's pretty great. And then, most creative of the word, k k k-"Kenissance," uh, renaissance in the wake of Barbie's movie depiction of Ken, and then "assholocene," scene, which seems actually quite great, uh, I I feel like, you know, that's like, uh. [crosstalk] [laughter] But congrats on the stochastic parrots, AI-Related Word of the Year, amazing stuff.
Emily M. Bender: That was a fun highlight of the weekend for me.
And that's it for this week. Dr. Eleanor Drage is a Senior Research Fellow at the Leverhulme Center for the Future of Intelligence. Dr. Kerry McInerney is a Research Fellow at, again, the Leverhulme Center for, uh, and the AI Now Institute. Thank you both so much for joining us.
Oh, thank you for having us. It really was delightful to get the chat about all the crazy and hellish, frightening things happening in the world of AI and also the smartbidets. So thanks again.
Eleanor Drage: Hey delulu!
Alex Hanna: Amazing. Our theme song was by Toby Menon, graphic design by Naomi Pleasure-Park, production by Christie Taylor, and thanks as always to the Distributed AI Research Institute.
If you like this show, you can support us by rating and reviewing us on Apple Podcasts and Spotify, and by donating to DAIR at dair-institute.org. That's D A I R hyphen institute dot org.
Emily M. Bender: Find us and all our past episodes on PeerTube and wherever you get your podcasts. You can watch and comment on the show while it's happening live on our Twitch stream. That's Twitch.TV/DAIR_Institute. Again I'm Emily M. Bender.
Alex Hanna: And I'm Alex Hanna. Stay out of AI Hell, y'all.