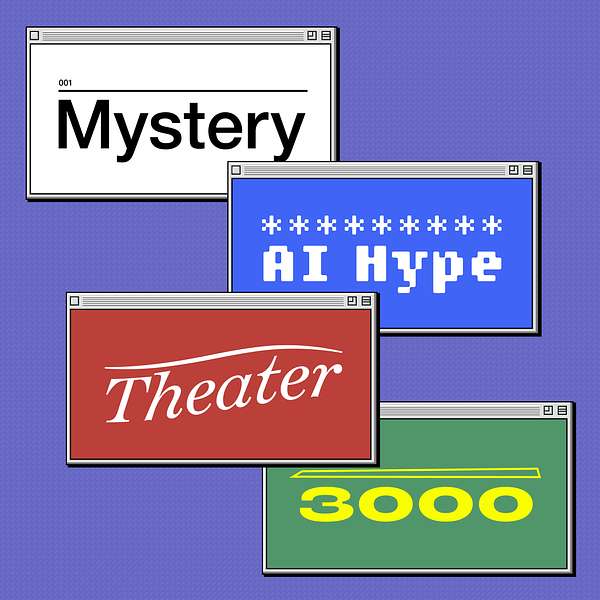
Mystery AI Hype Theater 3000
Mystery AI Hype Theater 3000
Episode 25: An LLM Says LLMs Can Do Your Job, January 22 2024
Is ChatGPT really going to take your job? Emily and Alex unpack two hype-tastic papers that make implausible claims about the number of workforce tasks LLMs might make cheaper, faster or easier. And why bad methodology may still trick companies into trying to replace human workers with mathy-math.
Visit us on PeerTube for the video of this conversation.
References:
OpenAI: GPTs are GPTs
Goldman Sachs: The Potentially Large Effects of Artificial Intelligence on Economic Growth
FYI: Over the last 60 years, automation has totally eliminated just one US occupation.
Fresh AI Hell:
Microsoft adding a dedicated "AI" key to PC keyboards.
- Dr. Damien P Williams: "Yikes."
The AI-led enshittification at Duolingo
- Shot: https://twitter.com/Rahll/status/1744234385891594380
- Chaser: https://twitter.com/Maccadaynu/status/1744342930150560056
University of Washington Provost highlighting “AI”
“Using ChatGPT, My AI eBook Creation Pro helps you write an entire e-book with just three clicks -- no writing or technical experience required.”
"Can you add artificial intelligence to the hydraulics?"
You can check out future livestreams at https://twitch.tv/DAIR_Institute.
Subscribe to our newsletter via Buttondown.
Follow us!
Emily
- Twitter: https://twitter.com/EmilyMBender
- Mastodon: https://dair-community.social/@EmilyMBender
- Bluesky: https://bsky.app/profile/emilymbender.bsky.social
Alex
- Twitter: https://twitter.com/@alexhanna
- Mastodon: https://dair-community.social/@alex
- Bluesky: https://bsky.app/profile/alexhanna.bsky.social
Music by Toby Menon.
Artwork by Naomi Pleasure-Park.
Production by Christie Taylor.
Alex Hanna: Welcome everyone to Mystery AI Hype Theater 3000, where we seek catharsis in this age of AI hype. We find the worst of it, and pop it with the sharpest needles we can find.
Emily M. Bender: Along the way we learn to always read the footnotes, and each time we think we've reached peak AI hype, the summit of Bullshit Mountain, we discover there's worse to come.
I'm Emily M. Bender, Professor of Linguistics at the University of Washington.
Alex Hanna: And I'm Alex Hanna, Director of Research for the Distributed AI Research Institute. This is episode 25, which we're recording on January 22nd, 2024. And it's time to talk about the workplace. If there's any universal anxiety about the so-called AI boom, it's that we're all going to lose our jobs as bosses adopt LLMs to do what we do.
But for cheaper and oh, by the way, also worse.
Emily M. Bender: The hypers would have us believe that "GPTs are GPTs": generative pre trained transformers are general purpose technologies. They can do anything. No more work for us. We're coming for those claims today. And if I may add, much more effectively than ChatGPT is coming for our jobs.
All right. So we've got a couple of our artifacts here for the main course. We're going to start, we decided with the one from OpenAI, which is where that "GPTs are GPTs" title comes from. Um, and then we've also got something from Goldman Sachs and I've just pulled up the OpenAI, um, uh, page here because it has the original date of this, which was March 17th, 2023, but if you go over to archive, um, the current version that's there is the version from August 22nd, 2023.
Did they fix all the ridiculousness in this paper in between? Well, I suppose it could have been more ridiculous. I didn't look at the first version, but.
Alex Hanna: I'm guessing that they didn't. Yeah. It's probably just updating a few graphs or something of that nature.
Emily M. Bender: Typos maybe. But the other thing that I have to say about both of these artifacts is that there's so much nonsense here that I'm afraid that we're going to run out of the hour and there's going to be something even worse that we didn't get to so just like disclaimer up front, just because we didn't talk about something in these papers doesn't mean we think it is at all reasonable.
Alex Hanna: Yeah, and before getting into the papers I also want to say that when both of these came out they got a lot of press, because these headlines are really catchy.
Basically the claim, first off, that GPTs are GPTs, that, um, generated preterm transformers are general purpose technologies. And then the other one that we're going to look at today is a report from from Goldman Sachs. It was actually quite hard to actually find this source material, while places like Forbes, the Financial Times, the Guardian, the Wall Street Journal, all the business press glommed onto this, even though the reports are pretty much as ridiculous as you'd expect.
So, well, let's, let's just get into it.
Emily M. Bender: Yeah. All right. So we're starting with, with the OpenAI one here. Um, and I think I'm going to skip the abstract because I have to rant first about the first line in the introduction. So it starts like this. "As shown in Figure 1, recent years, months, and weeks have seen remarkable progress in the field of generative AI and large language models" Parentheses, "(LLMs)." And I have to say, whenever I'm reviewing or reading something in this field, if the, if the motivation for the paper starts with, recently there's been lots of progress or increased attention or whatever, it's just like, I do not want to read the rest of this. And the inclusion of "weeks" in that made it even worse.
So I thought, okay, what's Figure One? Is it going to be some kind of a graph over time? Do you think there's a time axis in Figure One, Alex?
Alex Hanna: No, absolutely not.
Emily M. Bender: So this Figure One, um, is, uh, captioned, "Exam results ordered by GPT 3. 5 performance." So this is not progress over time. It is a collection of results of what happens when you run a multiple choice exam through these systems.
Um, so anyway, that was just not off to a good start.
Alex Hanna: Abstract Tesseract saying that, "GPTs should be called gratuitous poppycock touters." And man, I, I think I like that. Any use of poppycock, like A plus in my book. Uh, yeah. So what they, what they're doing in this paper is that they're effectively trying to make the argument that GPTs are these quote, general purpose technologies.
And the kind of frame of these papers, both this one and the Goldman Sachs paper, is that they're taking kind of a labor economic approach to this. So Emily's highlighting now, um, where they say, "To complement predictions of technology's impact on work and provide a framework for understanding the evolving landscape of language models and their associated technologies, we propose a new rubric for assessing LLM capabilities and their potential effects on jobs." And so what they do is they, this rubric, which is in Appendix One, and we're going to spend a lot of time by the way, uh, talking about methodology. Oh, hold on. Let me--go back up so I can read. Yeah, no, yeah.
So, um, what they do is that they, um is that they, oh, there's this ru--so the sentence again says, "This rubric measures the overall exposure of tasks--tasks to LLMs following the spirit of prior work on quantifying exposure to machine learning." And then they cite a few people, including a few labor, uh, economists, um, Brynjolfsson is, uh, is a very famous one.
They say, uh, I know they also cite, um, Daron Acemoglu, uh, and a few other people in this domain, um, and David, David Autor. Um, so, "We define exposure as a proxy for potential economic impact without distinguishing between labor augmenting or labor displacing effects." Already kind of weird there.
Um, but then, but then yeah, "We employ--" Okay, this is the real kicker. "We employ human annotators and GPT-4 itself as a classifier to apply this rubric to occupational data in the U.S. economy, primarily so--sourced from the O*NET database."
Okay. And I, so first off, not only is it ridiculous that they asked GPT-4 on what types of jobs will be most exposed to automation, the human annotators that they used were only people working at OpenAI.
Okay. So anyway, go ahead, go ahead, Emily. I mean, it's ridiculous on the face of it, but like Emily, go off.
Emily M. Bender: Oh, absolutely. So, so basically the, the, and we scrolled past here and I guess it keeps, it comes later. They've got all of these like claims of like, we found that, um, "Our analysis indicates that approximately 19 percent of jobs have at least 50 percent of their tasks exposed when considering--" blah, blah, blah.
And it's like, no, your analysis is nothing because you basically just said, we're going to fabricate some data and then pretend that it means something and make some percents out of it. Like they literally asked GPT-4, and they did their prompt engineering to get it, to give them an answer. Um, you know, could, could, uh, an LLM, uh, and we can talk about what it is that they're asking for, but it's something like, does the LLM speed this up by a factor of two or not, right? Without decreasing quality.
But like GPT-4 does not have that information. It's not like they've queried a database, right?
Alex Hanna: Right. And the thing is interesting because I think this is, I mean, this is a problem. There's problems that are endemic with this kind of uh, forecasting that happens that has to do with automation and what labor economists do, um, it's very qualitative in nature.
And I'm not saying that to to ding qualitativeness, but it's dinging--it's basically an assessment. That is very. um, very subjective in its nature, but then it is given this veneer of, of quantitativeness in the kind of graphs that they present. Now, this O*NET methodology, I had a long conversation with a friend of mine from grad school, John Lattner, shout out John Lattner, uh, who is a economic sociologist.
I'm like, John, this methodology seems really nonsensical. You know, if you're trying to assess this, is it typically the case that you make subjective assessments of exposure to automation as deemed by labor economists or some kind of other, uh, you know, social matter, uh, social matter, uh, expert? And he's like, well, yeah, it's famously hard to do this, you're, you're trying to make kind of less bad predictions.
And I'm like, okay, I understand this is hard and you're trying to make estimations, but it seems like, you know, it seems quite pernicious to then make the people who are the assessments, the people working at OpenAI and the technology itself. Like what, what, uh, what a methodology design that just is. Just absurd.
Emily M. Bender: Yeah. And, and even if you had people who were qualified to make the judgments, making the judgments, there's other methodological problems here. So, um, I want to, first of all, I think we didn't actually read, did we read the full title of the paper or did we just jump in and say it's the OpenAI paper?
Alex Hanna: Uh I think we said "GPTs are GPTs."
Emily M. Bender: GPTs--that's enough of it.
Alex Hanna: Yeah, yeah, yeah.
Emily M. Bender: Okay, but they do say, "An early look at the labor market impact of potential of large language models." So that's what they're claiming to do. Um. And so I've scrolled this down to the methods and data collection part here, skipping over their literature review.
Um, and I, I noticed that, that Jeremy asked in the chat, "Hey, wait, is this another wall of metrics fallacy papers?" shout out to the episode that he guested on. And no, we don't have a wall of metrics. We have one really bad metric in a couple of guises that is poorly calculated. So it's a different different kind of problem here.
Alex Hanna: There's a lot of, there's a lot of graphs. Actually, before we get into the methodology, I would love to go into the, the results, because like what seems to be the most exposed, because it's, it's sort of like, okay, um. And so if you go down, so there's, there's some, you know, there's some results.
Yeah. So there's some smooth things and I'd love to go to the, like, there's this huge list. It's either in the appendix, um, of kind of like, what is the most exposed? Um, I think it's in the uh--I think it's in Appendix 1, um, of this. I don't have my notes in front of me. Um.
Emily M. Bender: I'll keep scrolling down.
Alex Hanna: Yeah, I think it's, it's, it's like there's a very, there's a very big, um, yeah, this, this one, this is the one.
So this, this is like, um, so this, for those of you who are listening, what this looks like, it kind of looks like an iceberg plot, almost. It's a, it's a rotated horizontal plot that, uh, looks like they use kind of a, uh, it goes from very light to very dark and kind of a rainbow metric. And I love graphs like this because once you start reading this, there's a big, what the fuck moment here.
So--
Emily M. Bender: Uh hold on. I was trying, I was trying to rotate it so I could actually read it.
Alex Hanna: Yeah, totally, totally fine.
Emily M. Bender: So you'll have to tell me what's there, Alex. So that's tiny and I can't see.
Alex Hanna: So can you zoom in? Cause I also can't read this. Let's zoom into the thing that's the most exposed. We're really getting to the nitty gritty here, folks.
So the first thing, and I'm craning my head sideways so I can read this. So 'data processing, hosting and related services' is the most exposed. The second is 'other information services.' Okay. Third is 'publishing industries except internet.' I love the except internet here because that like is the kind of 'I work in tech. I'm not as exposed to this.'
Insurance carriers and related activities. Credit intermediation and related activities. Securities, commodity contracts, and other financial investments and related activities. Professional, scientific, and technical services. Lessors of nine financial intangible assets, except copyrighted works. Broadcasting except internet. Monetary authorities, central bank, fund trusts, and other financial vehicles. Wholesale electronic markets and agents and things, and then telecommunications.
Okay, this is, there, there's, again, I think there's a thousand job, a thousand and nineteen different job categories in the O*NET database.
Already, just in reading the description, and then they have this kind of coefficient of, of, of exposure. Um, and, and this is, and this is as graded by GPT-4. Already, this is setting off a little, a lot of alarm bells.
Um, so given that insurance carriers are very exposed, securities and exchange contracts, other kinds of legal services, Emily, these are a lot of the jobs that we've really been worried about, you know, talking about this kind of automation that you have things like legal contracts, or you having things like insurance carriers doing any kind of adjustment to this. And these are the things that are most exposed to automation. Just my, like, my alarm bells are just, like, just blaring at this point.
Emily M. Bender: And the exposure that we're worried about, to be very clear, is that somebody is going to decide that this is an okay thing to do. And then, you know, people who are trying to get their medical expenses reimbursed are going to be told, well, GPT-4 said that that's actually not eligible or whatever. Like that's the, that's the exposure that we're worried about.
I just want to say this 'except internet' thing here might not be these authors. It might be that these are categories that are in that O*NET thing. Oh look there's two of these.
Alex Hanna: Yeah. One's the GPT-4 grade and the other is the, um, is the, um, OpenAI, the human annotators. Yeah. And I think they have pretty high correlation between them, which is, which is, yeah, which is why they're valid.
They're, they're also trying to validate GPT-4 as, as a valid human rater here, right?
Emily M. Bender: Yeah, we need to, we need to talk about their interannotator agreement stuff, which is also nonsense. Um, and we'll, we'll get to that, but I think we have to talk about their, their methods and data collection in some detail.
So, um, section 3.1, "Data on activities and tasks performed by occupation in the US: We use the O*NET 27. 2 database--" I don't know if it's O-NET or O-star-NET. But whatever.
Alex Hanna: I don't, I don't know.
Emily M. Bender: "--which contains information on 1016 occupations, including their respected respective detailed work activities and tasks.
And I've got to say, first of all, that sounds like somebody sat down and tried to systematize something so that you could do studies of, um, you know, how people move through occupations or, you know, different kinds of things. So, you know, respect for attempting that, but also this is one of those, 'the map is not the territory' things, right?
So. "A DWA is a comprehensive action that is part of completing task--" Ungrammatical. "--such as study scripts to determine project requirements. A task, on the other hand, is an occupation-specific unit of work that may be associated with 0, 1 or multiple DWAs. We offer a sample of tasks and DWAs in Table 1."
Um, so I was looking at these. And, you know, task ID, um, 4668. 0, occupation title is 'gambling cage workers.' And the, uh, detailed work activity is 'execute sales or other financial transactions.' And the task description is 'cash checks and process credit card advances for patrons.' And it's like okay, yes, but when you've got a person who's standing there in the gambling cage, um, and they're doing these tasks, they are also probably keeping an eye out for people who are getting disruptive or like there's a bunch of stuff they're doing in parallel, um.
The last two things here are educational tasks, so 6529, the occupation title is kindergarten teachers except special education, and then 6568, elementary school teachers except special education, and there's no DWH, just a hyphen. And then the task description, yeah, isn't that weird?
Alex Hanna: Yeah.
Emily M. Bender: Involves [inaudible] volunteers, whatever, yeah.
Alex Hanna: Yeah, I don't know why that necessarily is. And they're, they say a little bit and I'd love for any kind of like folks who are in the labor econ field to sort of get on it because they're, they're O*NET itself, I think is a separate database, but then they're also doing a mapping here to the Bureau of Labor Statistics, the BLS.
Um, and so what, uh, so it looks like what they're doing is that they, the DWAs come from the BLS. Um, which for our non American listeners is our, um, is within, is BLS, do the BLS statistics come out through the Department of Labor or through Commerce? I forget. Um, if anyone knows, drop it in the chat, uh, but they say "we use the BLS recommended crosswalk to O*NET to link the O*NET task in the DWA dataset to the BLS labor force demographics, which is derived from the current population survey," um, which I don't know if that's, um, that's something that comes from Census or not.
Um, so "Both of these data sources are collected by the US government and primarily capture workers who are not self employed, are documented and are working in the so called formal economy."
So that's also a pretty huge, um, exclusion if you're talking about people who are not documented and are also not working in, are not working in the informal economy, but like, you know, you collect data. You know, use the data that you have. You come to the, you come to the labor econ, uh, you know, fight with the, uh, with the revolver you've got.
Emily M. Bender: Yeah. So, so, uh, they talk about then defining exposure. "We present our results based on an exposure rubric in which we define exposure as a measure of whether access to an LLM or LLM powered system would reduce the time required for a human to perform a specific DWA or complete a task by at least 50%." Now, thinking back to kindergarten teachers, elementary school teachers, anybody who's doing caring work, um, you don't speed that up, right?
A day at school is a day at school. If you, if you somehow did it in half a day, you haven't like increased productivity. You've just spent less time educating the kids like it doesn't.[laughter] You know.
Alex Hanna: Yeah.
Emily M. Bender: It doesn't make any sense.
Alex Hanna: And I think a lot of the, and I think at the same time, I think in those big, and I don't, you know, I can't look at this automatically and we want to spare our viewers who are seeing this, that, that absolutely tiny chart in the, the iceberg plot in which, um, Uh, Abstract Tesseract, Nick, Nick M said, "Matplotlib was-- lib? Libe?
How do you say I, I looked this up. "Matplotlib was like, I'm sorry, Dave. I'm afraid I can't do that."
And yeah, it was just the, the plot itself being, um, but probably that's why it's in an appendix. Um, but yeah, basically some, some of these things are really, you know, can't be automized.
Okay. Let's talk about this exposure rubric.
Cause I, cause it's kind of the same methodology that's in the Goldman paper. And I'd love to go to the Goldman paper too. Cause the claims are just as fantastical as this paper.
So they say, it's a little box here. So the summary, summary of the exposure rubric. "No exposure, E0 in parentheses, if using the described LLM results in no or minimal reduction in the time required to complete the activity or task while maintaining equivalent quality," uh, with a, uh, footnote here that says, "Equivalent quality means that a third party, typically the recipient of the output would not notice or care about LLM assistance."
Okay.
Emily M. Bender: So we, we can get away with throwing synthetic text in here and no one's going to notice. That's what they say.
Alex Hanna: Right, exactly. That's equivalent quality. "Or using the described LLM results in a decrease in the quality of the activity, task, uh, or task output." So actually going to get worse, as no exposure.
That's interesting as it only goes positive and there's not a negative exposure. Anyways, "Direct exposure, E1. If using the described LLM via ChatGPT or the OpenAI Playground--" and I say this because I think they might have assessed via ChatGPT and GPT-4 prior to its integration in ChatGPT. Remember this was released, this was written in March uh, 2023, uh, "--can discrete, can, can discrete, can decrease the time required to complete the DWA or test by at least half." So that's kind of partial exposure. And then "LLM plus exposed," which sounds like, um, some, you know, girls gone wild for chat bots, um, or "E2. Access to the described LLM--"
Sorry for that joke, uh, I really apologize.
"Access to the described LLM alone would not reduce the time required to complete the activity test by at least half, but additional software could be developed on top of the LLM that could reduce the time it takes to complete the specific activity task with quality by at least half. Among these systems, we count access to image generation systems."
And then there's another footnote. Uh, "in practice as can be seen in the full rubric, in Appendix A.1, we categorize access to image capabilities separately, E3, to facilitate annotation, though we combine E2 and E3 for all analysis. That was so much to say. Emily, take it away. I need to catch my breath.
Emily M. Bender: Yeah, so a couple of things in here. One is. I know I've lost the terminology for this, but when you're talking about data, there's, there's like continuous data where like 1.5 is related to one in the same way that 2.5 is related to 2. There's, um, discrete buckets that are nonetheless ordered. And then there's just, you know, discrete buckets that do not stand in any ordering relationship to each other.
And that's what this is. Like, they make it look like E2 is somehow more than E1, but it's not right. Yeah. So that's, that's an issue here. Um, but the--so this is, this is what they're trying to claim that they're measuring and they're trying to come up with numbers or cat--sorry, they're trying to label each of those, I guess, tasks or DWAs as E0, E1, E2, and then sort of saying, okay, well, then this profession has this many of its tasks in these categories, um.
But um, okay. This gets fun. So just below that box, it says "We set the exposure threshold at a potential 50 percent reduction in time required to complete a specific DWA or task while maintaining consistent quality. Um, we anticipate that adoption will be highest and most immediate for applications that realize a considerable increase in productivity. Although this threshold is somewhat arbitrary, it was selected for ease of interpretation by annotators. Moreover, regardless of the chosen threshold, we guess that real world reduction in task time would likely be slightly or significantly lower than our estimates, leading us to opt for a relatively high threshold."
So basically they're saying we picked an arbitrary number to have people make a guess about, and we put it at 50 percent because that was maybe easier to pretend that they were accurately guessing. I, um, I don't, I don't know.
Um, but then they have their Table 2, which is supposed to be showing us agreement. And they've got, uh, three different sets of numbers here. So there's comparison between GPT-4 using rubric version one, which is the one in the paper, and human; GPT-4 rubric version two and human; and then GPT 4 using the two different rubrics.
Problem number one.
Alex Hanna: So agreement, agreement with itself.
Emily M. Bender: Agreement with itself.
Alex Hanna: And they should note this, these, these numbers aren't very good. So they've got, um, uh, what is this letter? Is this a lowercase?
Emily M. Bender: You have alpha, beta, and then--
Alex Hanna: Well, what's the one in the header?
Emily M. Bender: Oh, the header, that's a gamma, isn't it?
Alex Hanna: That's a gamma, yeah.
Emily M. Bender: Yeah.
Alex Hanna: Is that a gamma? But is it the third one, a gamma too? Is it an uppercase? Oh, gosh. I don't know. I'm sorry.
Emily M. Bender: We're not physicists.
Alex Hanna: Please, feel free, yeah, feel free to dog me in the chat for not knowing my Greek letters. So they got an alpha, beta, gamma, and then they have different weightings. The percent agreements between GPT-4, it kind of is, is pretty, is, is, is above about 65.
But then the Pearson coefficient is, is pretty terrible just for E1 between GPT-4 and human. It's, which is--
Emily M. Bender: But hold on, they're measuring nothing that makes any sense at all here. You say just for E1 and they've got two more lines, but If you're talking about inter annotator agreement or inter rater agreement, then you say, okay, for all the data, to what extent did these two raters get the same answer?
And if you're doing it right, you're using a chance corrected metric, um, like Cohen's Kappa.
Alex Hanna: Right.
Emily M. Bender: For all of the data. They are looking at only the things that were labeled E1 or things that were labeled E1 plus E2 with some weight. So they're combining this like weighted score that they want to do and their agreement metric.
But the agreement metric should have been just about the underlying data. And, and there's a huge omission here that Jeremy has picked up in the chat. They didn't talk about inter rater agreement among the people who were doing the real annotation.
Right?
Alex Hanna: Yeah. Right. If you're not even, if you're not doing, yeah, if you're not even seeing how much you can agree between your different annotators, then, you know, how stable is that going to be once you start comparing it to GPT-4?
Um, so failure on agreement metrics, please go ahead and read a textbook on content analysis. Krippendorff has a nice one from 2004. Uh, You know, go ahead.
Emily M. Bender: Krippendorff of Krippendorff's Alpha, right? Which is, I think, in that same chance-corrected space as Cohen's Kappa.
Alex Hanna: As Cohen's Kappa, that's right. Yeah.
Okay, we could, oh my gosh.
Emily M. Bender: I think maybe we should bump over to Goldman Sachs here. Oh, no, hold on. There's something that I have to do here, though. They do have a limitations section. So without reading this in great detail, they say, "subjective human judgments." Basically we had people guess, that's a limitation. "Measuring GPT-4." Oh, they're going to recognize it as a weakness to fabricate data. Well, not quite.
Um, they say the outcomes, first of all, they, they cite something else from open AI saying Rrecent research indicates that GPT-4 serves as an effective discriminator, capable of applying intricate taxonomies and responding to changes in wording and emphasis," which is just bullshit, right? Um, they continue, "The outcomes of GPT-4 task classification are sensitive to alterations in the rubric's wording, the prompt's order and composition, the presence or absence of specific examples in the rubric, the level of detail provided, and the definitions given for key terms." Which is a lot of words to say, It's all nonsense. It's not data. We fabricated data, but here we're going to just sort of talk about how we didn't, we, we have to be sensitive to the fact that the fabricated data might come out slightly differently if we asked for it slightly differently.
Alex Hanna: That's right. That's right. Yeah. And it's, and it's really, yeah. And I mean, it's sort of the, um, yeah.
And again, in the subjective human judgments, a paragraph before, they say, "In our study, we employ annotators who are familiar with LLM capabilities," AKA people who work at OpenAI. And, but then they, they acknowledge, however, "this group is not occupationally diverse." Yeah, no shit, Sherlock. "Potentially--" That's not in the paper, obviously.
"--potentially leading to biased judgments regarding LLM's reliability and effectiveness in performing tasks with unfamiliar occupations."
You know, I would love a study that asked workers how well they thought these things could do their jobs. And I'm sure you would get quite different metrics.
Emily M. Bender: Yes. Yeah.
Oh, okay. So this is, this is, um, as course is saying in the chat, absolutely unscientific. It is nonsense. Um, but guess what? It's not the only one. So our other artifact comes from Goldman Sachs economics research. Um, I like how this has not only a date, but also a timestamp. So March 26th, 2023 at 9:05 PM Eastern daylight time.
Alex Hanna: Oh, gosh. They, yeah. Well, sorry for them, you know, that also signals Goldman Sachs, probably not a great place to work if they're releasing reports at 9 0 5 PM Eastern Daylight Time. I digress.
Emily M. Bender: Yeah, there's that. Okay. So this is the title. It is a global economics analyst. I guess that's the. Um, heading that it comes under.
And then the title is, "The potentially large effects of artificial intelligence on economic growth." Parentheses, Briggs/Kodnani, who are, I guess, two of the authors. There's then four authors listed um, with incidentally their phone numbers. Um, all right. And so there's the, this executive summary, which we need to read because it's got lots of nonsense in it.
And then we can get into the methodological nonsense. Um, how about I do the first one and then--
Alex Hanna: Yeah, go for it.
Emily M. Bender: Okay. Uh, "The recent emergence of generative artificial intelligence, parentheses AI, raises whether we are on the brink of a rapid acceleration and task automation that will drive labor costs and raise productivity."
Not quite grammatical. Uh, "Despite significant uncertainty around the potential of generative AI, its ability to generate content that is indistinguishable from human created output and to break down communication barriers between humans and machines reflects a major advancement with potentially large macroeconomic effects."
So generate content that is indistinguishable from human created output is not actually a good thing. Right?
Alex Hanna: Yeah.
Emily M. Bender: Um, and you were laughing before about how breakdown communication barriers between humans and machines is ridiculous, right?
Alex Hanna: Yeah. As in, as, as if machines have this kind of internal kind of mind and we need, we just need to understand each other better, man.
And so, so the next, the next point says, "If gener--if generative AI delivers on its promised capabilities, the labor market could face significant disruption. Using data and occupational tasks in both the US and Europe, we find that roughly two thirds, two thirds of current jobs are exposed to some degree of AI automation and that generative AI could substitute up to one fourth of current work."
These are like, these are monumental cataclysmic sort of claims and is not very different from what OpenAI said in which they said 80 percent of jobs would be exposed with about 19 percent to be completely replaced.
They continue, "Extrapolating our current estimates globally suggest that generative AI could expose the equivalent of 300 million full time jobs to automation."
Okay, so if you're taking, I don't know what you know, how many people work in the formal economy worldwide, say it's, I don't know, two thirds of, of, of how, what's the current global population, eight, 8 billion?
Emily M. Bender: 8 billion I think yeah.
Alex Hanna: Yeah. So that's, so that's about six. Um, so you're talking about 6 billion people, I mean, 300. What's, what's the math 300 million, uh, of six, a pretty minuscule, uh, kind of, uh, percentage, I'd suppose, but, uh, but given that they are extrapolating here from US and Europe data to, um, the global majority, that seems questionable. But, uh, yeah, that's, let's move on.
Emily M. Bender: Yeah. Yeah. Um, and this is, I mean, the rest of this is sort of more of that hype, um, on this page.
Um, but I think we need to, to get to their, um, explanation of generative AI. So this is the Goldman Sachs folks telling their audience, heading is "Generative AI Explained. We first discuss the current state of AI development and its key capabilities. Exhibit 1 provides an overview of generative AI in comparison to its predecessor machine learning methods, sometimes referred to as narrow or analytical AI.
In our assessment, the generative AI technologies currently in focus, such as Chat2PT, DALL-E, and LaMDA, are distinguished by three main characteristics. One, they're generalized rather than specialized use cases. Two, their ability to generate novel human like output rather than merely describe or interpret existing information.
And three, they're approachable interfaces that both understand and respond with natural language, images, audio, and video."
Um, this next paragraph has weird, uh, gratuitous shout out to Microsoft. So they talk about MS DOS to Windows to Office as their examples. Just like there was other software companies in the meantime, but this graphic, Exhibit One, is ridiculous.
So they have step one, training data to neural networks, step two, neural network to AI output, step three, AI output to human interface and step four, applications. And then in each of these boxes, there's previous ML methods, uh, contrasted with generative AI. And at each point it's just like, yes, people were using machine learning, you know, somewhat problematically, but at least sensibly, you know, like with a, with sort of a defined purpose and now we pretend we have magic.
So in the first box, "Previous ML methods: data trained on specialized databases for specific purposes, e. g. make statistical predictions about election results, answer questions about my biomedical literature, et cetera."
Um, yeah. So data trained on specialized databases for specific purposes. Sounds like the first step to a reasonable application of machine learning.
Alex Hanna: Yeah.
Emily M. Bender: Right. Contrast that with, "Generative AI: data trained on large generalized databases, i. e. the entire internet--" Put a pin on that. "--thus one, wider range of use cases and two, more easily able to spawn complimentary innovations with specialized use cases, in parentheses and quotes, 'deepening of AI'."
I just want to say probably again on this podcast, if somebody says that ChatGPT or anything else is trained on the entire internet, you immediately know, they do not know what they're talking about. Because the internet is not something you can go and download. Um, so huge red flag there. Uh, and you know, it doesn't get any better in the rest of these boxes.
Is there any of one that you want to dig into Alex?
Alex Hanna: It's, it's all bad, but you know, the, the, the next one, I mean, the step two is, is pretty bad. I want to highlight that. And then I want to like really get into some of the really ridiculous graphs here because they, because graph, uh, there's one graph in here, which is the most, uh, uninterpretable graph I think I've seen in, in probably a few years, uh, but the second box here, step two, neural network to AI output. And it says "Previous ML methods models generate statistical predictions based on relationships and training data." Okay. "Generative AI models seek to generate new information that is indistinguishable from human data."
Oof. Okay. "Achieved via the introduction of a second 'discriminative, and this is in quotes, neural network,' which evaluates the output of the primary quote, 'generative neural network' for authenticity relative to human output."
What the, like, this is--to me, I, I, I, I, if you see that, um, Breaking Bad meme where like, um, like Jesse says something really in this like ridiculous and out of context, my response is Brian Cranston going, what the fuck are you talking about, Jesse?
Um, because I have the idea what they're saying by talking about two different neural networks that operate in this ways. Then they completely you know, misuse a term by saying, quote, "This adversarial neural networks approach forces the generative network to revise its output and learn to consistently fool the discriminative network network."
So what I think they're describing here is reinforcement learning with human feedback, but it's like worst description I've ever.
Emily M. Bender: Actually, I don't think so. I think this is the GAN stuff that was used for image generation. And it's not to my knowledge--yeah.
So you had, you had one thing that was making an image and then the other thing I had to decide if it was a actually occurring human captured image or something.
Right. And so. Yes, a lot of the sort of progress in making these photorealistic images came through the scan training setup, but that's not there in the LLMs and yeah, you're, you're thinking it's maybe a bit like the RHLF, um, nope, RLHF segment in ChatGPT, but it's, it's not right. Like it's--
Alex Hanna: Yeah, it's, it's really, yeah.
All right. I'd love to go down to Exhibit 3, which is there--one of their things--there's, there's a, there's a lot bad in this paper and it, it, it, it received absolutely no criticism in the press.
I found it actually quite hard to even find this paper, which was only linked, like I could find not from the Goldman Sachs site, but from this weird Italian, uh, key4biz.it from some WordPress site. Anyhow, most of the, most of the, most of the business press didn't even link to the paper, really weird stuff.
Anyways, Exhibit Three is, "Management teams are increasingly focused on opportunities from AI on corporate earning calls. And more mentions of AI predict higher, um, capital expenditure or CapEx."
And so basically the first one is this mentions of AI in calls. Uh, and so not surprising they're mentioning it more, hype seems to make your investors happy, right? But then the second panel of this graph is like is not interpretable. So the X axis is a logged "mentions of AI on 2019 to 2022 earnings calls, so in 10-plus mentions of AI. And then the Y axis is "cumulative change in corporate uh, capital expenditures" and it's, and it's, and it's not, and then I think they're just doing a cross section here. And then there's a size of the bubble, which it goes completely undefined, and then in the actual graph, they've got, um, an average of the S&P 500 companies, which is around 50, cumulative change in corporate expenditures. And then there's a trend line and then they write out the coefficients for the line. And then the R-squared is tends out to--turns out to be 0.12, which one is not very big and like, what the hell is happening in this graph? If I got this in an undergraduate methods assignment, I'd go, what, what are you even showing me here?
Emily M. Bender: Yeah. And that line in the middle. So the average for S&P 500 companies with less than 10 mentions of AI is basically the average for the companies that are not represented on this graph, because this is only the ones that have more than 10.
Alex Hanna: Yeah.
Emily M. Bender: And it's kind of right in the middle. Also.
Alex Hanna: Yeah. And also I probably, they probably drop then everything that's less than 10 just because it would probably would completely flatten this trend line as well.
Yeah. So, yeah, absolutely nonsense. And then the last thing I'd love to highlight, I know we still need to get to, to hell, uh, is this, is this, is this thing where they actually get into the jobs. You know, I find it helpful to always get into the jobs and see what, what the hell they're talking about. So Exhibit Five or, um, here, uh, sorry, uh, yeah.
So these are the jobs that could be completely automated here. So, um, So the first one in the U.S. is, and I'm assuming they're using, um, kind of a mapping of O*NET to BLS or, or, um, or, um, uh, uh, or some kind of, um, mesh of the two. So the first one is office and administrative support, 46 percent of the jobs. Legal, 44 percent of the jobs, again, legal.
Architecture and engineering, 37 percent of jobs. Now this one is funny. 36 percent of life, physical and social science jobs could be automated. And as a social scientist, I'm like, really? Okay. That's, that's, that's really interesting that you say such a thing. Uh, 35 percent of jobs--
Emily M. Bender: Do they mean jobs like one person's work entirely automated so you have three people working instead of four. Is that the is that what they're saying here?
Alex Hanna: Think of, think of three social scientists. Think of, if you're a social scientist in the audience, think of two of your friends, which one of you is going to be automated? Um, yeah, but then it just gets into, then it's community and social service jobs, which is fascinating. And then management, which is also actually quite funny. Um, because I think, um, I think there was a tweet a few weeks ago, uh, in which they said, why aren't we thinking about automating CEO jobs? It seems like they don't actually do much and maybe, maybe it'd be most, most up for that.
But I just find this kind of, these kinds of general buckets to be both big and surprising.
Emily M. Bender: Yeah. I think we should talk about their methodology because they did not, it appears, ask GPT-3 or 4, um, but what they did instead was they basically took a classification of, um, tasks by difficulty from that same O*NET thing where there's apparently a scale of difficulty. So someone else has gone through and rated these things on difficulty and basically just said we are going to assume that anything up to difficulty level 4 can be automated.
And then we're going to run these numbers based on that. So it's also fake data.
Alex Hanna: Yeah.
Emily M. Bender: Um, where the, there's no basis for the claim that whatever this rating scale was of difficulty relates to automate ability, they're just making the assumption.
Alex Hanna: Yeah, right. That's right. Yeah, there's such, such interesting things here, like, um, things that would be in context, not really something that you could go and give to an LLM, for instance, interpreting the meaning, uh, of information for others and then in the right column, the easiest task would be 'interpret a blood pressure reading.'
Okay. Yeah, I can interpret a blood pressure reading. If it's probably, uh, north of 100, you're probably doing decent. If it's, um. if it's, if it's higher than, than 160, you probably got a problem.
Uh, but the people who are doing that in context are EMTs, nurses, doctors, medical staff, uh, they're not going to go to their ChatGPT calculator and put it in. And that's not going to make their job any easier because they're pretty easy metrics there. Why do you need an LLM for this? You don't. Why are you going to introduce it into that?
Especially for frontline workers, you're not going to.
Emily M. Bender: Yeah. Oh, and then I'm looking at this last one here. So, um, the "AI-exposed work activity column," uh, the value is performing administrative activities. And then the examples of tasks by difficulty, difficulty 4. So automatable, according to this assumption, "complete tax forms for a small business."
Like if you care about the accuracy and presumably you care about the accuracy of your tax forms, you do not want the synthetic tax extruding machine in there doing it for you.
Alex Hanna: There's amazing ones here. There's this great. I just, I want to just read one. One is "check to see if baking bread is done."
Really? I'm going to, I'm going to just, I'm going to. Hey, if you could put an LLM in a, in a baking thermometer or whatever, I'm not a baker. I don't know how baking works, but like, then, then go ahead. Anyways. Yeah. Let's, let's move on.
Emily M. Bender: All right. I got to give you a prompt about musical or not musical this time, Alex.
Alex Hanna: Hey, let's go for musical.
Emily M. Bender: Okay. So somebody in the chat just said something. "Plot twist." This is Abstract Tesseract. "The difficulty scale is the same one they use for the songs in Dance Dance Revolution." So a DDR style song with lyrics. About the difficulty of job tasks.
Alex Hanna: Oh my gosh. All right. I have to think about this.
All right. This is my cover of 'Can't Stop Falling In Love.' Um, I, I played a lot of DDR in my youth.
[singing] It's like my life was empty with you before that you came along. Then I got in. LLM to check if my bread was done. Sometimes I really want to be sure that it is proofed. But now I know with this large language model that it's ready to peruse. Can't stop eating my bread and using an LLM, can't stop--
Uh, I can't think of how to finish that.
Emily M. Bender: Oh, that's the best one yet, Alex.
Alex Hanna: Thank you. I, I thank you for mentioning Dance Dance Revolution. My wealth of, of, of playing that in the basement of the student union at Purdue is paying off.
Emily M. Bender: All right. Can't stop eating my LLM bread.
Okay. We have, we have six things here under Fresh AI Hell. I really want to get to them. The first one I picked because it's, it's, uh, seems very relevant to this week's topic. So this is from ZDNet, um, and, uh, the category is home slash tech slash services and software, headline, "Create an ebook using AI for $35. Using a--" Subhead, "Using ChatGPT, My AI eBook Creation Pro helps you write an entire ebook with just three clicks, no writing or technical experience required."
Alex Hanna: Oh gosh. Oh yeah. Yeah. Because, because we, we write just, you know, just to, you know, just to create eBooks, you know, no thoughts, brain empty.
Emily M. Bender: And I wish I knew who said this, but I heard from somebody like, if you couldn't be bothered to write it, why should I be bothered to read it?
Like, this is that. Okay. Next.
Um, I've got something on X. Oh, yeah.
Alex Hanna: Um, yeah. So this is, this is one, this is a post by Reid Southen, who's a visual artist, um, and has been very vocal against AI. So he writes, "Duolingo laid off a huge percentage of their contract translators and the remaining ones are simply reviewing AI translations to make sure they're acceptable. This is the world we're creating, removing the humanity from how we learn to connect with humanity."
So just like nightmare material, like even if you're doing Duolingo, uh, and you're trying to learn a different language, you don't even know if the translations are correct or not.
Emily M. Bender: Yeah. And it's like also, again, this was interesting work apparently for a pretty broad range of contractors.
And now they've all just been said, you're redundant because we can make a fake version of this, that the people trying to learn this are not in a position to tell the difference. So we're going to get away with it.
Um, the next one is another tweet. On the quote tweet of that one, um, from Dean Tāne, um, "It's not just the translations, it's the voice recordings. The Irish Duolingo course a few months ago switched to AI generated voices that has pronunciation all over the place, and in many cases makes up entirely new sounds that don't exist in any Irish dialect. It's terrible."
So--
Alex Hanna: There was a follow up. There was a follow up on this was this that was pretty interesting.
It might be the second tweet in this where it's--yeah it's this one.
"So Duolingo loves to pride itself on having more people--and having more people learning Irish than there are Irish speakers in the world." And then they say, "We see this fact all the time when the app is loading, but this--if this is what they're doing now, they are already causing untold damage to the language. Um. It would be like the American kid who wrote the vast majority of the Scots Wikipedia with no knowledge of the language and just made everything up, but much worse."
And it reminds me of some of the kind of work in language revitalization, which I'm sure you can speak a lot more to Emily, but kind of like people like, um, uh, there's this one professor who's revitalizing, uh, Native Hawaiian and the way that, you know, there was establishment of certain schools for that.
But imagine if, I think Hawaiian might even be a language on Duolingo, but imagine that you're just kind of mangling the language via AI translations. I mean, you're not going to preserve that language. You're going to do irrevocable damage to it.
Emily M. Bender: Yeah, yeah. And, and there's already so the folks at Te Hiku Media in Aotearoa New Zealand are big on like, this is our community's data, we should decide what happens with it and we should benefit from it if there's if it's being used in a way that's generating benefits, and you know financial or otherwise.
And this is like, not, not only are we going to stop employing anybody who speaks this language, we're just going to just, you know, keep reusing that data to, to make money while creating a product that's worse, but the people who are using the product can't tell, or so they hope. Very sad.
Alex Hanna: Yeah.
Emily M. Bender: Okay. This next one is something from my own institution that I've just wanted to rant about a little bit, um, on the pod for a while.
Now, this came from the office of the proto--provost, um, at the very end of last year. Um, Okay. "Provost Tricia Serio: um, Dear colleagues, as this year draws to a close and I reflect on my first quarter as provost, I am inspired by and grateful for your contributions to the University of Washington's Michigan, sorry, mission to preserve, advance, and disseminate knowledge." Et cetera, et cetera.
Um, and then there's this paragraph a little bit further down. Um, "I'm looking forward to the new year and new quarter and the promise they hold. Is that the part that I'm looking for? Hold on.
Um.
Alex Hanna: Is this, is this the right one? I'm looking for some GPT stuff.
Emily M. Bender: Yeah, no, it's not GPT. It's, um, so they, there's a bunch of stuff in there about how great it is that, uh, faculty, staff, and students come together to create art and literature, conceptualize performances, reveal discoveries, and develop technologies, right?
All the stuff that we're doing at the university. And then. In this penultimate paragraph, "I hope you'll join me at the Provost Town Hall in February, more details to follow soon, when I will discuss the state of the university from the academic perspective and highlight some of the ways faculty and staff are leveraging artificial intelligence to transform their research, innovation, teaching, and impact."
And I just felt so let down.
Alex Hanna: I mean, maybe, maybe, um, maybe Provost Serio will invite you to be on the, on the, in this town hall. Maybe.
Emily M. Bender: Yeah. Yeah. Usually it's my colleagues in computer science who get invited to these things, not me. Okay.
Alex Hanna: All right. So this is on, on, on, is this Bluesky? Uh, yes. So this is Dr. Damien P. Williams saying. Uh, and, and so this is, um, uh, uh, quote tweeting, uh, um, or quote, posting, quote
I don't know if you call it, uh, Nash, who says, "Speaking of shitification--" Shout out to Cory Doctorow, um. "Microsoft has gone so balls deep on 'AI'" in quotes "they're forcing keyboard manufacturers to add a new key to open their shitty quote 'AI' function in Windows. Meanwhile, users are either not using it or trying to get it off their computers."
And then, uh, Dr. Williams is saying, "Yeah, Microsoft spent too much dollars on AI hype without real robust use cases before seeing what the public could or would actually do with it. And now, rather than admit they were very, very wrong to do so, they are literally, they're trying to literally, literally manufacturing consent via a whole new keyboard form factor. Which. Yikes."
Emily M. Bender: Yikes indeed.
Alex Hanna: And it's. Yeah. All right.
Emily M. Bender: All right. And then to take us--to take us out of AI Hell I have some comic relief here. This is a Tumblr post by someone named ddwayne. Sorry, reblogging something from an account called Fuck Customers.
Um, and they are--
Alex Hanna: [laughter] Sorry, just a very funny Twitter, Tumblr name, not Twitter.
Emily M. Bender: Um, so the thing says, "Half rant, half story. I'm a physicist. I work for a company that helps develop car parts. Essentially, car companies come to us with ideas on what they want from a part or material, and we make slash test the idea or help them make slash test it.
Usually, this means talking to other scientists and engineers and experts, and it's all fine. Sometimes, this means talking to business people and board execs, and I hate them. A bit ago, when AI was really taking off in the zeitgeist, I went to a meeting to talk about some tweaks Car Company A wanted to make to their hydraulics, specifically the master cylinder, but it doesn't super matter.
I thought I'd be talking to their engineers. It ends up just being me, their head supervisor, who was not a scientist slash engineer, and one of their executives from a different area, also not a scientist slash engineer. I'm the only one in the room who actually knows how a car works and also the lowest level employee, and also aware that these people will give feedback to my boss based on how I quote, represent the company whilst I'm here.
I start to explain my way through how I can make some changes they want, trying to do so in a way they'll understand, when head supervisor cuts me off and starts talking about AI. I'm like, oh well, AI is often integrated into the software for a car, but we're talking hardware right now, so that's not something we can really-- 'Can you add artificial intelligence to the hydraulics?'
'Sorry, what was that?'
'Can you add AI to the hydraulic system?'
Can I fucking what mate? 'Sir, I'm sorry. I'm a little confused. What do you mean by adding AI to the hydraulics?'
'I just thought this stuff could run smoother if you added AI to it. Most things do.'"
It goes, it goes on.
Alex Hanna: It just goes on. Yeah, that's, that's, that's incredible.
I just, I, it's the kind of magic sheen on all of this and it just absolutely infuriating. I mean, it would be like, if you asked a system to generate, let's say, a new engine design, uh, like, could you? It would probably blow up, you know, right away, given that these are combustible systems.
Um, and my lord, like, the kind of, you know, and I think it's just the kind of, thing that's happened to all kind of management.
Yeah, I just, I just, I just can't my brain. I'm just not computing right here.
Emily M. Bender: I have to like just underscore this last little bit here. "So he was--" This is from that same post. "He was seriously asking. I've met my fair share of idiots, but I was sure he wasn't genuinely seriously asking that I add AI directly to a piston system, but he was, and not even in the like, 'Oh, if we implemented a way for AI to control that part' kind of way, he just vaguely thought that AI would make it better.
Alex Hanna: Yeah, so, so just rub on, rub on some AI, just amazing. Yeah. Yeah. And Medusa Skirt saying, "Can you add an AI to the spring to calculate the Boltzmann constant in real time?" And I'm like, ugh, oh my gosh.
Emily M. Bender: I mean, clearly pistons have a job that is definitely exposed to LLMs and they're gonna either increase their productivity or lose their jobs in the near future. Right?
Alex Hanna: Hey, how hard is it to actually calculate whether, you know, an engine will explode or not? It can't be that hard.
Emily M. Bender: Let's ask GPT-4.
Alex Hanna: Let's do it, y'all. Uh, all right. I think we're, we're headed out. That was a fun one. I'm sorry for all the, uh, all the fits and starts to begin this. But yeah, these are, this is cathartic.
Those are papers I've been wanting to go after for a while.
So that's it for this week, our theme song by Toby Menon, graphic design by Naomi Pleasure Park Production by Christie Taylor. And thanks as always to the Distributed AI Research Institute. If you like this show, you can support us by rating and reviewing us on Apple Podcasts and Spotify, and by donating to DAIR at DAIR-institute.org.
That's DAIR hyphen institute.org.
Emily M. Bender: Find us and all our past episodes on PeerTube and wherever you get your podcasts. You can watch and comment on the show while it's happening live on our Twitch stream. That's twitch.tv/dair_Institute. Again, that's D A I R underscore Institute. I'm Emily M. Bender.
Alex Hanna: And I'm Alex Hanna. Stay out of AI Hell y'all.