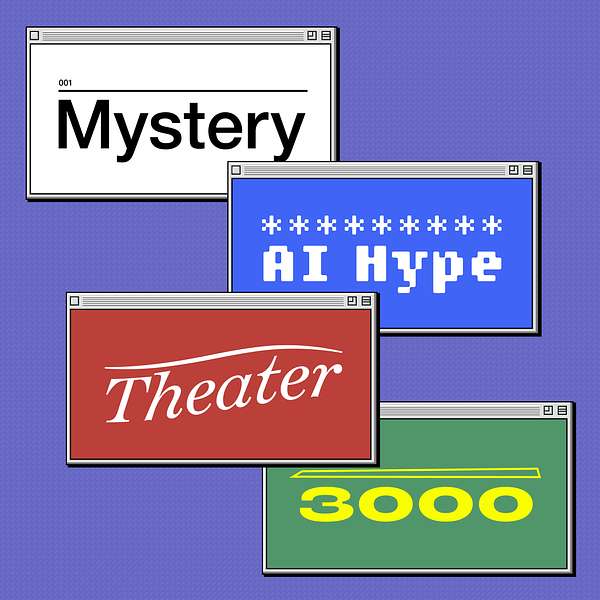
Mystery AI Hype Theater 3000
Mystery AI Hype Theater 3000
Episode 28: LLMs Are Not Human Subjects, March 4 2024
Alex and Emily put on their social scientist hats and take on the churn of research papers suggesting that LLMs could be used to replace human labor in social science research -- or even human subjects. Why these writings are essentially calls to fabricate data.
References:
PNAS: ChatGPT outperforms crowd workers for text-annotation tasks
- Beware the Hype: ChatGPT Didn't Replace Human Data Annotators
- ChatGPT Can Replace the Underpaid Workers Who Train AI, Researchers Say
Political Analysis: Out of One, Many: Using Language Models to Simulate Human Samples
Behavioral Research Methods: Can large language models help augment English psycholinguistic datasets?
Information Systems Journal: Editorial: The ethics of using generative AI for qualitative data analysis
Fresh AI Hell:
Advertising vs. reality, synthetic Willy Wonka edition
- https://x.com/AlsikkanTV/status/1762235022851948668?s=20
- https://twitter.com/CultureCrave/status/1762739767471714379
- https://twitter.com/xriskology/status/1762891492476006491?t=bNQ1AQlju36tQYxnm8BPVQ&s=19
A news outlet used an LLM to generate a story...and it falsely quoted Emily
Trump supporters target Black voters with faked AI images
You can check out future livestreams on Twitch.
Our book, 'The AI Con,' comes out in May! Pre-order your copy now.
Subscribe to our newsletter via Buttondown.
Follow us!
Emily
- Bluesky: emilymbender.bsky.social
- Mastodon: dair-community.social/@EmilyMBender
Alex
- Bluesky: alexhanna.bsky.social
- Mastodon: dair-community.social/@alex
- Twitter: @alexhanna
Music by Toby Menon.
Artwork by Naomi Pleasure-Park.
Production by Christie Taylor.
Alex Hanna: Welcome, everyone, to Mystery AI Hype Theater 3000, where we seek catharsis in this age of AI hype. We find the worst of it, and pop it with the sharpest needles we can find.
Emily M. Bender: Along the way, we learn to always read the footnotes, and each time we think we've discovered peak AI hype, the summit of Bullshit Mountain, we find there's worse to come.
I'm Emily M. Bender, a professor of linguistics at the University of Washington.
Alex Hanna: And I'm Alex Hanna, Director of Research for the Distributed AI Research Institute. This is episode 28, which we're recording on March 4th of 2024. And this week, the hype we're annihilating is very near and dear to our hearts as social science researchers.
Did you know the work of social science, of studying how people work and why they do what they do, can be made easier? All you have to do is invent some research participants out of thin air. At least, that's a claim from recent papers about the usefulness of LLMs in, quote, "simulating human samples."
Emily M. Bender: We've been knee deep in papers claiming LLMs might be the future of both processing and creating data about human behavior and experiences.
And as you might expect, we're very much in doubt that there's a practical or ethical path forward for either. You ready to get into it, Alex?
Alex Hanna: Oh gosh, let's do it. This stuff is pretty painful and something that we've been watching for quite some time. So let's do it.
Emily M. Bender: Yeah, so we're going to start with the one that's probably closest to home for me.
Um, and I think we can make pretty quick work of this one. So this is a short piece in Proceedings of the National Academy of Sciences, otherwise known as PNAS, uh, entitled "ChatGPT Outperforms Crowdworkers for Text Annotation Tasks," by Gilardi et al., uh, published in July of last year. So here we're looking at ChatGPT.
Next, we're looking at something that's looking at GPT 3. Um. And so, abstract, "Many NLP applications require manual text annotations for a variety of tasks, notably to train classifiers or evaluate the performance of unsupervised models." Absolutely, right? So, when you're using machine learning for something, you need some gold standard data to uh compare against and when you're doing machine learning for language technology, um, especially text based language technology, that is annotations over text.
So they're not wrong there. And if it's supervised machine learning, you need lots and lots of annotations, uh, to train the system.
Um, and then they say, "Depending on the size and degree of complexity, the tasks may be conducted by crowd workers on platforms such as MTurk, as well as trained annotators, such as research assistants. Using four samples of tweets and news articles, n equals 6,183, we show that ChatGPT outperforms crowd workers for several annotation tasks, including relevant stance topics and frame detection."
Um, hmm. (laughter)
Alex Hanna: So I mean, getting, yeah, getting into this, I mean, what they're doing--and I should foreground, you know, the folks who are doing this, these are political scientists at, um, University of, of, of Zurich, uh, ETH.
And so what they're trying to do is effectively, um, take a few studies here, looking at tweets, mostly tweets and news articles and, uh, effectively trying to do some pretty basic content analysis of them. Um, and so it's, interesting that they're doing this. I mean, this is kind of the idea of, you know, we have these basic things that we want to pull from articles and we want to characterize them.
Uh, and if you ever did content analysis, you know, you typically need to get a lot of humans to look at them to make some kind of evaluation of what these things are with regards to the content of them. Um, you know, and this is very common and say, political science research: is this, you know, pro or anti Trump or pro or anti abortion or something of that nature. And this is what they're using ChatGPT for in this case.
But it's pretty questionable. I mean, it's you know, like you're saying, you know, this thing is doing the annotation, um. And what struck me about this is, well, a lot of things struck me about this, um, you know--Emily's highlighting something, so I'm going to let her go first because I think she--
Emily M. Bender: Yes, so they're going on about how this is so much work, um, and, uh, you know, you might already have available data, but probably in your own research, you need something different. So it says "More typically, however, researchers have to conduct original annotations to ensure that the labels match their conceptual categories."
And I'm like, yeah, that data work is often actually a big part of the work. Um, and then a little bit further down, "Trained annotators tend to produce high quality data, but involve significant costs. Crowdworkers are a much cheaper and more flexible option, but the quality may be insufficient, particularly for complex tasks and languages other than English."
Um, that 'languages other than English' thing surprised me. I guess maybe the annotation pool is just smaller. But, um, seems like you are also in--if you're not working in a colonial language, you're less likely to get annotators who are uh, socially distant from the text that you're trying to annotate.
Like, you know, um, but okay. So, uh, um, "explores the potential of LLMs for tax annotation tasks with a focus on ChatGPT."
Um, and then they--sorry, I'm looking at the PDF down where my notes are, and it's not formatted the same way, still a bit hard. So here's a bunch of, um, hype. "LLMs have been shown to perform very well for a wide range of purposes, including ideological scaling, the classification of legislative proposals, the resolution of cognitive psychology tasks, and the simulation of human samples for survey research," which I think might be our next artifact that we're looking at.
Alex Hanna: It is, it is. Yeah.
Emily M. Bender: And it's like--
Alex Hanna: And the thing is, yeah, I mean, the let--the ideological scaling thing, I don't think would necessarily be one that was questionable. Because ideological scaling is actually already done pretty well by existing methods. So, for instance, a very famous, um, political methodology for this is DW-NOMINATE, which what they effectively do is they take the bills that have been, uh, introduced, uh, I'm, I'm going to mess this up, but, but from what I understand is that the bills that that have been written and then co-sponsored by particular legislators, and they look at kind of, um, the kind of language used in those bills, and then they can assign to each legislature, a kind of estimate of their ideological position. But then it's, you know, so it's, it's not surprising that then these things which have ingested a lot of these kinds of scales and training data, um, or can replicate some of that, um, like categorization would do pretty well on that.
But I think it's then a pretty large jump to say they can be quite a big replacement for many, many different types of tasks that crowd workers are typically doing, right. Especially in the cases, as you point out, Emily, where the task is pretty complicated and defining what the problem itself is, is quite a, um, quite onerous in its own right. Right.
Emily M. Bender: And an important part of the work. Like that's where the science is. So for that ideological scaling application, it seems to me that that's something where text classification makes sense as, as an approach.
Um, I'm far from convinced that ChatGPT is the right kind of classifier to be using, but you know, it's a, it's less of a mismatch than this use case where you're trying to provide the ground truth so that you can test the text classifier, which is what they're proposing here. Um.
Alex Hanna: Yeah.
Emily M. Bender: So another thing that really jumped out to me is so they, they talk about how, uh, "In our previous study, the texts were labeled by trained annotators for five different tasks." Um, and then here they say, "Using the same code books, uh, we submitted tasks to ChatGPT as zero-shot classifications--" (unintelligible) Cats are upset too. "--as well as to crowd workers on MTurk." So they're taking something that was designed to work with trained research assistants and then just putting that as is up on MTurk for crowd workers who don't have the same training and into ChatGPT. Like that seems like terrible methodology
Alex Hanna: It's really, it's really interesting that they say that they could take the code book itself and, um, and submit it to ChatGPT in the same way.
I know from the development of many different, um, codebooks, um, you know, that I've worked with is that codebooks themselves can be very multifaceted. There's lots of exceptions. Things are meant to be human readable in particular ways, and, you know, trying to parse all that out takes a lot of contextual knowledge.
So, for instance, one other project that we do, I've been working with, uh, Ellen Barry a lot, um, for the past 7 to 8 years, is this project on coding protest events in in at college campuses. Um, and so even determining what a protest is very difficult. And I know there's a there's someone that I've talked with Neil Caren who tried to do this with ChatGPT and actually found that they were able to get very basic kinds of things out of it.
But it seems kind very curious, I--we had a little discussion about this at a, at a social movements workshop, but even determining what the boundaries of the protest are very, very difficult. And so it is, you know, it, the fact that you could sort of give a code back codebook to an LLM whole cloth and expect it to produce similar results is, is quite, it's quite odd.
So I just want to answer this question about what the method was for political science scaling, it's DW-NOMINATE, usually stylized all in capital letters.
Emily M. Bender: Cool. Thanks. So that, that situation you were just suggesting or describing where it's difficult to define the boundaries of what you're looking at, like what counts as a protest, for example. Um, but it's easy to get ChatGPT to give you some output. That's the danger zone, right? Where it's like, well, sure, this looks reasonable. We're going to say ChatGPT is doing a good job. It's, it's fabricating data. I really think that this is, this is a tantamount to fabricating data.
Um, so I want, there's a couple more things I want to say about this before we move on to the other one, which is maybe a bit meatier.
Um, "We then evaluated the performance of chat GPT against two benchmarks. One is accuracy relative to that of crowd workers and two is intercoder agreement relative to that of crowd workers, as well as our trained annotators." When I saw this, I'm like, what are they comparing? Is it--
Alex Hanna: Yeah, it's different and it's, it's actually different annotation runs.
So they actually say "lower--" well, they say "across the four datasets," and I don't know where this is also, cause I'm looking at the PDF, but it's in the results section, um, "Across the four datasets--" It's below the graph, I think Emily, so yeah, yeah. "--we report ChatGPT zero-shot performance for two different metrics, accuracy and intercoder agreement. Accuracy is measured as a percentage of correct annotations using our trained annotators as a benchmark--" And then here's the kicker, "--while intercoder agreement is computed as the percentage of tweets that were assigned the same label by two different annotators." So "between research assistants," this makes sense, "between crowd workers," this makes sense, "or ChatGPT runs."
So it's, it's kind of hilarious that they are then saying that they were calculating intercoder agreement for chat GPT between its runs. And then if you go and you actually look at figure, um, figure one, uh, the intercoder agreement between ChatGPT with temperature 1 and ChatGPT 0.2 is nearly a hundred percent. Incredible. So for looking that it would agree with itself is that's a, that's a bit of a, that's a, that's a kind of a false and inflated metric there.
Emily M. Bender: Yeah. So to review, the reason you do intercoder agreement is basically to evaluate the effectiveness of the codebook. Is this something that's clearly defined enough? Is the task clearly defined enough and the instructions well enough written that different annotators will give consistent answers to each other. And you can also do intercoder agreement where it's the same person, but across time, like are people applying this thing consistently. That is evaluating the codebook, it is not evaluating the annotators. Although I guess once you've moved to the MTurk world, it's like, okay, are these, are annotators with insufficient training going to be doing this well enough?
Um, and I kept reading this going, surely they're comparing ChatGPT to the people doing annotation, but no, they're comparing to itself saying, look, it's consistent, so therefore it's good, which is not what that means.
Alex Hanna: Yeah. So there's a question, there's a question in the chat and I just want to, I was typing out a response, but it was not between ChatGPT and the coders, but typically because intercoder reliability is done between hypothetically, it's two different raters from the same rater pool.
So you wouldn't necessarily compare a coder and ChatGPT or a, uh, with the metric. That's why they're taking accuracy statistics as kind of a metric measure of that rather than doing intercoder, because then it would just probably just be very low. Um, so it does, yeah, hypothetically it's not, or, or, or kind of empirically, it doesn't make sense to do this.
Emily M. Bender:
Yeah. None of this makes sense. But there's, there's something from BacterX that I want to bring up. Were you going for that one too?
Alex Hanna: No, no. Go ahead. Go ahead.
Emily M. Bender: Okay. Um, I think this sums up the paper well. So BacterX has, uh, in quotes, "Getting high quality annotations is difficult because untrained people can give you poor quality annotations." And then right arrow, -->. And then in quotes, "We tested our methodology against untrained people." So yeah, uh, here's something that doesn't work. Here's something else that probably doesn't work, but it seems to work a little bit better than the other thing that doesn't work, like.
Alex Hanna: Yeah, I mean, I mean, I will say, you know, I will grant this paper a little bit of credence just because the, um, you know, these are rather simple classification tasks, right?
And I mean, classification tasks themselves are somewhat often very rote, especially if there's kind of language that it keys in on. And, you know, classification itself is a, is a, you know, pretty mature kind of, um, you know, it's one of the kind of foundational things of much of machine learning. And if you're developing some kind of a language model that's doing this in such a way that it can guess a class, you know, sure, you know, more power to you.
I would also then, but I would say that the way that this is really, I mean, first off, this paper got massively covered in a lot of tech press on Twitter and effectively the top line of it was 'ChatGPT replaces crowd workers.' And a lot of crowd workers got incredibly mad at this, rightfully so.
They're like, okay, is it actually going to replace crowd work? No, it actually can't because these are very narrow applications of a tool like this, um, and, um, TurkOpticon, and we should drop this in the show notes too, put out a, uh, wrote a letter, uh. TurkOpticon being the, uh, an organization that represents MTurk workers. But they had, they put out something in the TechWorker Coalition newsletter called, "Beware of the Hype: ChatGPT didn't, didn't replace human data, data annotators," rightfully pointing out, you know, it's you know, data annotators do a lot more work, or excuse me, crowdworkers do a lot more work than just annotate, you know, whether a particular frame or topic is in a news article or a tweet, especially for political science research. Um, and these workers do a lot more foundational work.
Um, you know, you really can't go ahead and reduce and say crowdworkers are going to be put out of business because, you know, this ChatGPT tool seems to work from pretty narrow set of classifications.
Emily M. Bender: So I want to push back a little bit on your credence there, Alex.
You're going to give this thing a little bit of credence.
Alex Hanna: Go ahead.
Emily M. Bender: So yes, text classification is a mature field. And yes, that's something that you can do. But you cannot replace the creation of gold standard data with the classifier itself.
Alex Hanna: Yeah, no, I agree. I agree with that.
Emily M. Bender: That's what they're proposing here.
That's what they're saying. They're saying we don't have to actually do the expensive thing of hiring trained annotators or the less expensive thing of hiring crowd workers. We can just throw ChatGPT at it for much less money in order to create the gold standard, which is basically just proposing fabricating data.
Um, and as, uh, SPDeGabrielle says in the chat, "So they have identified an efficient and cost effective methodology to commit academic fraud." Um, and I think yes, although with the twist that it is above board, that is, they're not pretending not to do this, they're bragging about doing it. So it's not data fabrication or academic fraud in the sense of like hiding the data fabrication, but I think it's still fabricated data.
Alex Hanna: Yeah. And BacterX asked also had a good question, which is, you know, "In your opinion, is this a failure of just science journalism or framing by the journal and the authors?" I mean, I think that the authors are pretty, are admitting quite well what they're doing. I wouldn't even say science journalism really, you know, globbed onto the story.
It was really, it was really kind of a lot of tech publications that glommed on the story and they really ran with it. And, um, there was a good push back there, um, linked from, um, the TWC, um, uh, newsletter that, uh, Chloe, um, Chloe Xiang from Vice, uh, had written about this, uh, but it didn't, it didn't replace, you know, all the AI hypers and Twitter just going ham on the story and really, you know, losing their shit.
Emily M. Bender: Yeah. And then we see other people pointing to this in like, well, look, we don't have to use real annotators anymore. We can just get ChatGPT output and use that. Like I've seen this cited in other places and that's super frustrating. Um, or this one or some arXiv papers that are similar. All right.
Alex Hanna: Yeah.
Emily M. Bender: Um, worth pointing out that this was not NLP people. As you said at the beginning, this was political scientists, and now we're going to see some more political scientists.
Alex Hanna: This is political science. Yeah. This is political--
Emily M. Bender: Wanna start us off on this one?
Alex Hanna: I'll do it. Yeah. So this is political scientists with computer scientists at Brigham Young University.
Um, this is published in the Journal Political Analysis, which is the, uh, kind of flagship journal of politic, the political methodology section of the American Political Science Association. Um, lots of good work in this journal typically. Um, and so the title of this journal, uh, this journal article is, "Out of One, Many: Using language models to simulate human samples."
Lead author is Lisa P. Argyle, um, and the abstract says, "We proposed and explored the possibility that language models can be studied as effective proxies for specific human subpopulations in social science research. Practical and research applications of artificial intelligence tools have sometimes been limited by problematic biases, parenthetical, such as racism or sexism, unparenthetical, which are often treated as uniform properties of the models. We show that the, quote, algorithmic bias with one such tool, the GPT-3 language model, is instead both fine grained and demographically correlated, meaning that proper conditioning will cause it to accurately emulate response distributions from a wide variety of human subgroups. We term this property algorithmic fidelity and explain its extent in GPT-3."
"We create quote, silicon samples by conditioning--" which--
Emily M. Bender: Eew eew eew.
Alex Hanna: Yeah, it's, it's, I hate, I hate this term. It just, it makes me want to, it makes me want to, um--
Emily M. Bender: It's creepy.
Alex Hanna: It's creepy. Yeah. It's got a, it's got a big ick factor.
"--by conditioning the model on thousands of sociodemographic backstories from real human participants in multiple large surveys conducted in the United States."
Then they compare the two, they say it's nuanced, et cetera. Um, yeah. Yeah.
Emily M. Bender: Let's get the date on this.
Alex Hanna: Yeah. This was pretty recent. Yeah.
Emily M. Bender: A year ago.
Alex Hanna: Early--a year ago. Yeah. So February 21, 2023 is when this is published.
Emily M. Bender: Yeah. And it's important to note that they are, they must have done the research prior to November of, of '22.
So they're using GPT-3 and not ChatGPT, which is, you know, effectively the same technology, but without the, like, conversational overlay. Um.
Alex Hanna: Right.
Emily M. Bender: Not that that makes it any more plausible. It's just sort of making sure that we're thinking about the right thing.
Alex Hanna: Um, yeah. Yeah. Yeah. So, so this is this, there's so much, there's so much in this article that I mean, is bizarre. And also the terminology is also kind of wild. So what they're effectively doing here, so first off they say kind of in the first, the first graph. Yeah.
Emily M. Bender: Oh, I want to take us to the samples here so we can see what they're doing, but we can go back to the first graph.
Alex Hanna: Yeah. Well, this one of the things they're doing, right, is that they, this is one of their tests. So the, one of the tests that they have here, and for those of you listening, what we're looking at is a two by two table, and--in the proud social science tradition. And what they're doing is that they're providing these kind of vignettes here, uh, and then, and then giving, uh, giving these things, uh, these responses from GPT-3.
So, um, there's basically a way of, if you are a Democrat, how you describe a Republican and vice versa. So. Uh, for Democrats, we have--this is a strong Republican. They, they wrote, "Ideologically, I described myself as conservative politically. I am a strong Republican racially. I am white. I am male. Financially, I am upper class in terms of my age. I am young. When I am asked to write down four words that typically describe people who support the Democratic party, I respond with, 'liberal--'" And this is the generated text, "'Liberal, socialist, communist, and atheist.'"
And then if we go and the more--and then I want to go to the, the, describe the strong Democrats describing Republicans. Ideologic--because it's more interesting. "Ideologically, I described myself as extremely liberal. Politically, I am a strong Democrat. Racially, I am Hispanic. I am male. Financially, I am upper class. In terms of my age I am middle aged. When I am asked to write down four words that typically describe people who support the Republican party, I respond with, 'ignorant, racist, misogynist, and homophobic.'
Yeah, go ahead. So, Emily, what do you think?
Emily M. Bender: So, so the reason I wanted to, so--yeah. We'll get back to sort of the headline of what they're doing, but I wanted to put this in the context because when they say something about coming up with first person backstories, that's what this is.
So, they're creating prompts where they, using first person language, describe um, a position in the social space defined in this other survey they're working from, but written out in words. And then they sort of leave off with, so "I respond with," and then it's colon one dot, right? So they're, they're basically prompting GPT-3 to come out with a list of some number of words.
I'm not sure what about this makes it stop at four. Maybe they just cut it off at four. Um, but this is, this is not like elsewhere in the paper. They're going to talk about how there's not just one bias, there's actually multiple different biases, and we can disentangle it by creating these silicon samples, and the silicon samples are basically prompts describing somebody.
And it sounds like they think that therefore this is accessing the words that people matching this description, so liberal, Hispanic, middle aged, upper class, and male, um, and strong Democrat. This is somehow indexing the part of the training data for GPT-3 that came from people without identity, which is bullshit.
Like that, that's not what's happening here.
Alex Hanna: Well, I think that, I mean, I, I, I do think that there's enough of, you know, enough carefulness, I would say in this, not to say it's necessarily described accessing kind of an output of. Individuals in those data, it's more like I think that it's more like they're saying the biases that exist are--there's not just one kind of bias, which sure, you know, why would there be, but there's multiple different types of biases in the text and there, if you kind of prime this, you will have then some kind of association in which predictive test texts will then come out from that priming.
And then there's, but then there's also kind of elements of this--and I, and I want to go down to some of these. First off, I, I, let's go back up to the top.
Emily M. Bender: Yeah, sorry.
Alex Hanna: There's a few parts of these that, that kind of really sent a lot of, um, annoyance, uh, through, through my, through my, through my bones. So one of them is in this first graph, um, they write, um, we, "When trained at scale--" So they describe basically what model LLMs do, but then they say something, they say, "When trained at scale, they exhibit a remarkable ability to capture patterns of grammar, cultural knowledge, and conversational rhythms present in natural language." And I'm like, uh, that's, that's a curious thing to say, to say that they are exhibiting cultural knowledge, uh, I'm really, and I'm really disturbed by that kind of characterization of the language model.
Emily M. Bender: Yeah, one of these things is not like the other. Patterns of grammar, sure, that's a property of language form. Conversational rhythms present in natural language, okay, sure, that's a thing about language form. Cultural knowledge, very different.
Alex Hanna: Yeah, yeah, yeah, for sure. And then that, the second part that got me was this second paragraph, where they say most discussions of this algorithmic bias treat it as a singular macro level feature of the model and seek ways to mitigate negative effects. And that, to me, is annoying in the way that they that they are thinking about what bias means. And to me, one of the things, the signals is it is, it is possibly not just their failure.
It's possibly a way that fairness as a field tends to characterize bias, where it's sort of one like bias equals bad rather than being very specific about the ways that we talk about racism, sexism, uh, you know, misogynoir, uh, transmisogyny, homophobia, all the things that we know are different axes of domination, but then do get reduced to this idea of I think what I quote singular macro level feature of the model.
And it's a bit indicative of the things that they cite here. I mean, they say, um, you know, Solon Barocas, and, and Andrew Selbst's paper on, um, big data's disparate impact. But even in that paper, or I mean, you know, Solon and Andrew are at least nuanced enough to talk about disparate treatment and disparate impact with regards to race and sex, upon which there is the most anti discrimination case law. Um, but then they also aren't, aren't, you know, citing things like Gender Shades, citing things in which you're talking about particular sorts of axes of domination. And so I, I do want to flag that just because I think it's at least sort of a criticism that starts from a bit of a straw man.
And I do want to flag that just to start.
Emily M. Bender: Yeah. So I want to come back to the thing I was saying about how they, they seem to suggest that they can decompose this and then find the biases of different people. It's in this paragraph or one of the places. So, "It is possible to select from among a diverse and frequently disjoint set of response distributions within the model, each closely aligned with a real human subpopulation."
So they, they really seem to think that by poking at this model, by priming it with, I'm a this, I'm a that, and then getting different answers out, they are accessing the, uh, kinds of biases, um, held by the people that they're describing in that prompt. Right. Which is not, you know--no.
Alex Hanna: Well, there, I think what they're, yeah, go ahead.
Sorry.
Emily M. Bender: Oh, no, I was going to move the next thing. So go ahead.
Alex Hanna: Well, what I'm thinking that they're doing, and I mean, I think a lot of what they're doing is that they're taking, they are sort of taking a particular prior methodology. Right. So they say on, in this paragraph, "We obtained this evidence by quote 'conditioning--'" and, and I, this kind of term conditioning is a little weird. "--GPT-3 on thousands of socio demographic backstories from real human participants in multiple large surveys in the United States to 2012, 2016, 2020 waves of the American National Election Studies survey--" Which is a very large, uh, multi-year um, I think it's panel, a panel survey. Uh, although they might do some cross-sectional things there. "--and Rothschild at al's Pigeonholing Partisans data."
And so they're effectively saying, you know, and, and in the two by two table, they're effectively conditioning is that they're replacing, you know, particular demographic and, uh, you know, in--and, uh, identitarian properties of the subjects, and then they are then trying to show what stereotypes may exist there, right? And so, sure, if you're doing that kind of slot filling, you know, they're sort of, you know, then trying to retain, you know, return these sort of things that you would get in the survey data, right?
But it is, you know, shouldn't be treated as this kind of subject. I mean, it is a subject in so far as one is a subject and can be reduced to a certain class of data points in survey data. Um, but it's, it's, it's, but it's really, I mean, it's this line that they're trying to ride and it's very odd in speaking of this way, especially in the using this terminology of 'silicon subject.'
Emily M. Bender: Yes. So they start the same paragraph we're looking at with, "High algorithmic fidelity in language models is crucial for their use in social science, as it enables researchers to extract information from a single language model that provides insight into different patterns and attitudes across many groups."
And I'm going to stop there for a second. This presuppose their conclusion. Like when they say "High algorithmic fidelity language models is crucial because it--"
They're basically presupposing that this thing exists, which they are trying to establish. That really got me. Um, and then they talk about there's, uh, so "provides insight into the different patterns of attitudes and ideas present across many groups." And then their sample here is women, men, uh, we're missing some genders there. White people, people of color, millennials, baby boomers, et cetera. And I just have to like, once again, say, what happened to GenX?
Alex Hanna: Oh, you're Gen--they didn't catch that. Cause you talked about that on another podcast.
Emily M. Bender: Another podcast. Yeah. When we were on The Daily Zeitgeist I'm like, Gen X, underrated. Everyone skips over us. Here it is again. Um, but then worse than that, um, and, and missing genders is worse obviously, but then they say, "and also the combination and intersection of these groups." So Black immigrants, female Republicans, white males, et cetera.
This feels to me like an appropriation of a misappropriation of the notion of intersectionality. Um, and you know, they're not citing Kimberlé Crenshaw here, so they're not doing like the worst version of it, but like intersectionality is not like the set intersection where people happen to have multiple, um, of these identity characteristics, but actually the experience of getting discriminated against along multiple lines.
And so whenever anyone uses intersection, I'm like, do you really know what you're talking about?
Alex Hanna: Yeah. I mean, that's a, I mean, that's a classic thing that happens. I mean, even even for political methodologists, I mean, people that are really in, you know, do that do a bunch of political anti-oppressive work often think that it's kind of "add identities and mix" without really focusing on the, the notion of, uh, of, you know, the kind of the quote unquote basement element of this, that the, the oppressions are unique and qualitatively different rather than just additive.
Emily M. Bender: Yeah. Um, yeah.
Alex Hanna: One thing I do want to touch on here is the, uh, their four, their four criterion, uh, or criteria for the, um, what they call algorithmic fidelity. Um, so first off, they defined algorithmic fidelity earlier by saying, "We define algorithmic fidelity as the degree to which complex patterns of relationships between ideas, attitudes, and sociocultural context within a model accurately mirror those within a range of human subpopulations. The core assumption of algorithmic fidelity is that texts generated by the model are selected not from a single overarching probability distribution, but from a combination of many distributions. That structured--and that structured creation of the conditioning context can induce the model to produce outputs that correlate with the attitudes, opinions, and experiences of distinct human subpopulations."
So yeah, so effectively what they're saying is that the probability distribution of words, um, effectively can be conditioned. There's many different distributions of words over the training data and those have an association with actually existing things people would write of human populations. And so that's. That's, you know, you know, I, I, I'm having a bit of trouble parsing this and you selected this other thing that I also selected in the text, Emily, and so I want you to, like, get into that a bit more.
I have my, my, my, um, um, beef with this, which I think is a lot more maybe fundamentally epistemically in, in, in argument with these folks, but I want you to go ahead.
Emily M. Bender: Yeah. So, so first I want to say that there are valid social science questions to ask. I think that you could use a trained language model to answer, if you had a known and curated data set that the language model was trained over.
Alex Hanna: Sure.
Emily M. Bender: But if you want to say things like, what do we see in the distribution of output in this language model? And, um, people like Dan Jurafsky, and I forget the first author on that paper, have done that where they've like selected texts from different periods in history and looked at how the word associations change and you can trace things about societal attitudes moving, but that's only valid if you've sampled the text in order to answer some specific research question, rather than whatever garbage is inside GPT-3 that OpenAI won't tell us about.
So that, that's one thing. The thing that I've highlighted here--oh, and in the paragraph above that we skipped over, it says, "Many of the known shortcomings and inaccuracies of large language models still apply, Bender et al, 2021. Marcus, 2020." That's the stochastic parrots paper. They're like, yeah, yeah, yeah, stochastic parrots.
Um, but they say, um, talking about breaking down the sort of macro level bias into something more specific, they say, "It suggests that the high level human-like output of language models stems from human-like underlying concept associations." That seems false to me. There's a big jump there, right? It's giving human-like output.
Well, why? We don't know why. You can't assume that it's the thing that you want. But even if that were true, the next sentence doesn't follow, despite their assertion. So they say, "This means that given basic human demographic background information, the model exhibits underlying patterns between concepts, ideas, and attitudes that mirror those recorded from humans with matching backgrounds."
Like, I don't think those two things, I don't think that follows from the previous one, and I think the previous one is also false.
Alex Hanna: Yeah. And I mean, I think my, in my, this does such an--my big thing that's sticking in my craw here, which I think is, I mean, this, this kind of line, and I mean, this, this is more of a--
Oh, sorry. My cat is--I'm like, why is my PDF scrolling? And it's because I've got a cat on the space bar.
Uh, and, and so, I mean, I think, you know, my, something that's really in my craw here, and I think this this is more of a critique of more of, you know, a large swath of political science research rather than rather than read this paper in particular, but I think that the kind of idea, sort of an ideological, there's sort of an ideological output that stems from demographic identity and from identity writ large, right? And I mean, a lot of this is kind of what has given rise to, you know, much of the work and polling and the kind of Nate Silver industrial complex of the, of, of, of thinking that you can really, you know, that, that identity is very much kind of destiny in political opinions, which, you know, if you're looking at things on the whole, there's going to be some, some trends that sort of track, um.
And then, but then they are making the jump here that the kind of language is, and I think this is, this is really coming from the initial assertion here, "The high level human-like output of these language models stems from these concept associations." And then that tracks to this demographic background. Right? Um, and. Um, we should probably take quite a, you know, that's, that's quite the, that's quite the assertion. Right?
Emily M. Bender: Yeah. That, that your background, your demographic background, um, determines patterns between concepts, ideas, and attitudes. And all we have to do is basically input that into the model and then we can get stuff out that is useful data to do theoretical or empirical work with in political science.
Like this is once again, advocating for data fabrication. You wanted to get to their criteria though.
Alex Hanna: I wanted to get to the criteria, mostly because I wanted to get to the criteria one, which they call the "social science Turing test," which I which I hate.
Emily M. Bender: Yes.
Alex Hanna: I hate, I hate on a guttural level. But the social science Turing test, which they say "generated responses are indistinguishable from parallel human tests."
So effectively, what they're doing is comparing the outputs here, um, to what they have, and I think in the Pigeonholing Partisans paper, the Rothschild et al paper, and I actually was curious on the results from this.
So in study 1, where they have free form partisan texts, they do the comparisons here, um. And on page 342, which is page six of the PDF, um, they are--yeah, yeah, it's after this graph, although I kind of want to come back to this graph because it's kind of funny. Um, but they, at the end, the last sentence on the page is, "We find evidence in favor of both criteria--" In this case, this is the Turing test and also the quote 'backwards continuity test,' uh, which we didn't, we didn't describe what that is, but they said, "We find evidence in both, in favor of both criteria. Participants guessed--" And these are, um, I believe MTurk workers, uh, again, "--61. 7 percent of human generated lists were human generated, while guessing the same of 61%, uh, 61. 2%."
So this is, and then two tailed difference of, uh, p equals 0. 044. So first off, not only were these pretty abysmal results in either assessing whether this is human or computer generated, and note that this is published in 2023.
Uh, you know, before the widespread adoption of, uh, and, uh, of ChatGPT, which means that they had to be doing, um, a lot of this work, uh, prior to, um, that actual general release. Um, but it's already really, and so people are probably quite--much more adept at guessing that now. And also just statistically, I mean, it's wildly very statistically, I mean, you know, have your, have your critique of frequentist, you know, statistics and, and P, uh, P value, uh, you know, testing and whatever.
But even if you accept all that, the P value is, is quite terrible here.
Emily M. Bender: So are they saying, I think they're saying that these numbers are not significantly different. And that's what they're giving us that P value.
Alex Hanna: Oh, okay. Okay, great.
Emily M. Bender: Not super clearly written, but I think they're saying--
Alex Hanna: No, no. You know, so the difference between the two is not, is not significant.
Emily M. Bender: Yeah.
Alex Hanna: Okay, great. Thank you for clar--
Emily M. Bender: They're not bragging about that P value.
Alex Hanna: No, no. I was going to say, are we bragging about that? It's, it's terrible, right? No, but.
Emily M. Bender: No, it, yeah.
Alex Hanna: But regardless, yeah, the numbers are quite, thank you for correcting me. I will admit my, you know, my, uh, my failure there. Um, but yeah, the values are not that great.
And you can imagine if they did the same thing these days, it would be, it'd be pretty bad, right?
Emily M. Bender: Right. But even, even if this shows what they want it to show, um, my note says, 'This is pointless. What they're establishing is the capacity to mislead, not the validity of anything.' Like their, their social science Turing test is, can we create output? Can we produce the form of something such that a person reading it can't tell if it came from a machine or not? That's got nothing to do with whether it's actually useful data for your social science questions.
Alex Hanna: Yeah. Yeah.
Emily M. Bender: Right. It's, ugh. Um, okay. So, where, where are we in this? Is there anything else we need to, you wanted to go back to the graph, or the figure here.
Alex Hanna: The graph, the graph is, is, is, there's just a lot of bubbles basically comparing words that come out. I mean, there's nothing, there, there's some weird ones that emerge, like, uh, describing uh, Democrats. Uh, GPT-3 seems to say that they're people uh uh a lot of the time, which is weird. I mean, this is, uh, so it's like, "I would just describe Democrats as people," which is kind of hilarious.
Um, and then there's some curious ones here, as in the humans in the study. If you identify as a Democrat, um, and you, um, and you are describing Democrats, uh, you are more apt to describe them as caring and educated.
Now I'm just talking about the study and the actual, but, but the actual interesting thing too, is that there's, even if you look across some of the most used words, it doesn't even seem to be a, like a lot of variation across the, the GPT-3. Like the bubbles seem to be kind of the same size across many of these things, like there's nothing, anything that's super surprising. Whereas that there's a lot more of this variation across the human, the human descriptions, which I found to be interesting.
Emily M. Bender: So this is the, the other dimension here is extremely conservative, something independent, something Likert scale between conservative and liberal.
And you see like things going from bigger to smaller in the humans ones in some places. Um, but not, uh, yeah, and boy, does GPT 3 like outputting people to talk about Democrats down here too. Yeah. This is, hold on.
Alex Hanna: This is the people. So this is the, if you describing, this is GPT-3 describing. This is hard to describe on audio. So if you are, yeah, and someone in chat says, "This is extremely US-centric." It's a, it's a, it's an American, it's based on an American national election study. So it was 100 percent US centric. So, I mean, they're not, they're, it's not a, Uh, political scientists, uh, are, you know, they, they will, if you studied not-America, you are called a comparativist or you do a study in international relations.
Otherwise you are an Americanist. Uh, it is the most, and it is the most boring part of political science. Um, and yet there's, you know, one of the big five sections or the big five classifications in political science is Americanism.
Emily M. Bender: Alex, can you help me with something? Yeah.
Alex Hanna: Yeah, go ahead.
Emily M. Bender: Can you help me with this, with this figure?
So, yeah, at the top we have describing Democrats, describing Republicans.
Alex Hanna: Yeah.
Emily M. Bender: And then on the, the Y axis, so that sort of X axis, we have most frequent words to describe, to use to describe Democrats and most frequent words used to describe Republicans.
Alex Hanna: Yes.
Emily M. Bender: So, what are the quadrants of this thing?
Alex Hanna: Oh. So the quadrants, so the quadrants are how you're, like, the tool or the person is primed.
And so, so if the, so in the humans part of it, it is, if they identify as extremely, uh, conservative, um, and it's not quite, it actually is a Likert scale. So it is a seven point scale from "extremely conservative" to "extremely liberal." And so that is actual humans. And then the, in the GPT-3, it is how the LLM is actually quote unquote "conditioned."
So the thing where it said, I am extremely conservative, I would describe Democrats as that and then the yeah.
Emily M. Bender: But these two big rows here are "most frequent words used to describe Democrats" and "most frequent words used to describe Republicans," which they pulled out to display this, I guess. Um, and they don't say most frequent frequent--relative frequency of word occurrence.
In which data set? Or I guess we're going to assume it's the human data set.
Alex Hanna: The original--yeah. In the Pigeonholing Partisans data set.
Emily M. Bender: Okay. That's the original.
Alex Hanna: Yeah. That's the original data set. That's the human data set. Yeah.
Emily M. Bender: So I think I need to take us out of this. IrateLump is hilarious here: "Democrats are definitely party."
Alex Hanna: Yeah, it's, it's kind of, yeah, it's, it's, it's interesting, interesting errors here in this, in this, um, when you see this, just kind of a, you know, and they are definitely people, uh, and just some, some weird, some weird errors at the margins here.
Emily M. Bender: "Republicans are conservative." All right. So I think we can leave this behind.
I've got two other artifacts that are sort of in the same space that I just want to say a couple words about each and then we'll go on over to AI Hell. Um, first one is a paper that appeared in January '24. "Can Large Language Models Help Augment English Psycholinguistic Datasets?" And here they're talking about the practice of norming, where you ask a bunch of speakers of a language, a bunch of questions about things like which words are more similar, um, which words are more frequent than others, and then you can compare that to what's happening in the corpora. And these people are apparently suggesting replacing ChatGPT, sorry, replacing those human judgments with ChatGPT, which is basically a representation of a large corpus. So ditching the part where you compare to what people think.
The other one, this is published in Wiley, um, and the title is, "The Ethics of Using Generative AI for Qualitative Data Analysis," um, and it is a largely multi author study.
You want to say something about this one?
Alex Hanna: It's published in Information Systems Journal. Oh, okay. Just correcting that. Yeah.
Emily M. Bender: Yeah, yeah. Wiley is the publisher. Yeah, Information Systems Journal. Yeah.
This is apparently a bunch of the editors of this journal who had a big conversation and decided to turn it into an editorial. And if you get into the, the introduction here, they're basically saying, we couldn't agree on whether or not this would be good science, but we agreed that it's got ethical issues.
So we're going to talk about those ethical issues.
Alex Hanna: Yeah. I mean, and it's, and it seems like what they're doing is that they're, um, they, it seems like, I mean, this is more of a problem here, and I haven't read this one in detail, but it says the editor, one of the editors, uh, was contacted by an associate there who explained that the qualitative data analysis tool ATLAS.ti, which is, you know, one of the kind of big one or two, um, uh, qualitative analysis tools, including uh, and, uh, NVivo and, um, and, uh, and, uh, Dedoose, uh, was offering a free of charge analysis of research data--if the researcher shared the same data with ATLAS.ti For training purposes for their, uh, uh, generative AI analysis tool.
Uh, so just, you know, just, you know, yeah, we agree, and we agreed with, uh Petttere in the chat, this is probably fine. But it's also the fact that, you know, we're like, and we should talk about this at some point on the pod in the future, all these companies are rushing to take any kind of text trove that they're sitting on and to sell it to OpenAI or sell it to some third party, whether that's Reddit, whether that's been, um, I uh DocuSign--
Emily M. Bender: WordPress.
Alex Hanna: WordPress, uh, and, and Automattic their, their parent company, uh, Tumblr.
Um, there's a great episode of the 404Media podcasts where they talk about, uh, WordPress and Tumblr. And this wasn't even an official release. I think they had a Tumblr employee leak it. Um, so anybody who says any, anybody sitting on any kind of textual data, it's like a, it's like a gold rush to cash in on it.
I've been particularly pissed off because, um, a tool I use and I kind of love, PDF Expert, now offers like an AI summation of the PDF you're reading. No, if I needed an AI summation of the PDF reading I was reading, I wouldn't be reading it. Get this shit off my, off my tools.
Emily M. Bender: All right, gotta take us over to Fresh AI Hell.
Um, and I think we need another single. So the second hit single from, uh, Ethical Autonomy Lingua Franca, the, uh, post punk twee band with their breakout hit, Rat Balls, um, is, I don't know the title of it. You'll have to tell me the title of it, Alex.
Alex Hanna: Oh, yeah. It's obviously Silicon Samples.
Emily M. Bender: Okay, Silicon Samples, and I was going to say the content is, um, lyrics based off of effectively Silicon Samples.
So, go.
Alex Hanna: Yeah, yeah. All right, I gotta go back to that text. Uh, it starts with this really kind of whiny intro where it goes, (singing) I am liberal. I am 30. I am a white male. I am upper class. The four words I would use to describe my dad are ignorant racist misogynist and rich! Da da da da da da da da. Thank you.
Emily M. Bender: (laughter) Love it.
Thank you. Okay, um, we are now in Fresh AI Hell, starting with, article published in BBC, Glasgow and West by Morven McKinnon titled, "Police called to Willy Wonka event after refunds demanded," from a week ago. Alex, what do you want to say about this one?
Alex Hanna: This one has just been sweeping the nation. I mean, I guess the world because it's based in the UK know, this went quite viral.
You know, the, the Willow, the Willy Wonka, AI generated hellscape, uh, that people got so mad at that they had called the police, and was it, was it Glasgow or Glastonbury? Um, where this happened, it was, it was, uh, it was in, uh, Glasgow yeah, yeah. And so, you know, they, they had used these AI tools to, uh, you know, create these really magical, uh, type of, um, visuals for the event and everything.
And they ended up being in a warehouse. Um, they hired uh, actors to recite AI generated scripts. No one had actually, uh, you know, they had given the scripts a few, a few hours before. Um, and then the, the most, the most depressing part of this is that, like, some of them, some of the kids couldn't get in. When they came in, they had to ration jelly beans. They were given, um, I think one to three jelly beans, according to which actor you, you talk to, and a quarter of a cup of lemonade.
Um, there was an absolute, an absolute kind of devastating and like hearing the actors, you know, actually recite these types of things. There was this one AI generated character who's called The Unknown.
Uh, and it was supposed to be this this, this, this chocolate maker who hid in the walls and there's this video clip of this, this thing with this dollar store mask on and it comes out and all the kids start crying immediately. And, and it's just, and then there's this, the woman who plays the Oompa Loompa, uh, you know, and, and, and she looks like she absolutely is having the worst day of her life, and they did an interview. Vulture did this interview with her. And it's just, uh, it just, everything speaks to what's at the core of AI hype. Like just the hellscape of, you know, uh, of, uh, whole events they're supposed to be filling, done cheaply, uh, and taking, uh, I think they, they charged 35 pounds to get people to come in.
So, yeah, it's so much to that, to the character of AI and you know, late capitalist hellscape.
Emily M. Bender: Yeah, it's all hype, and we're going to do really terrible working conditions for the poor people who are involved with it. Okay. We're not getting through all this hell today. We have to deal with the AI Hell backlog soon, but I definitely want to get to this one and then that one.
Um, so this is something that happened to me. I found a quote attributed to me in a news site called Bihar Prabha, which is from Bihar in India. Um, and it was not something I'd ever said. It was an article about, um, BlenderBot 3. And so I emailed the editor saying, this is fake, take it down, print a retraction.
And they did that, they replied very quickly, and they took out the quote. And then in their email to me, they admitted that they prompted an LLM to generate the piece. Which was just like, you know, this is all happening early in the morning for me, and it like didn't even occur to me that that's what it was.
But, um, yikes. And also it means that anybody who's been, you know, quoted in the news recently is at risk of having fake quotes attributed to us. Um, because that's how they're doing these things now. It's gross. Um, and--
Alex Hanna: Absolute nightmare.
Emily M. Bender: Yeah, unfortunately I didn't take a screen cap before they changed it, because like I said, early in the morning.
But, um, they--I also had the email they sent owning up to the thing. All right. I think we got to go straight to this one, Alex. We'll get we'll do a Fresh AI Hell thing recently. This one is from today, BBC title is "Trump supporters target Black voters with faked AI images," and the byline is BBC Panorama and Americas, whatever that is, and the author is, the journalist is Marianna Spring.
Um, and the title, before I get you to describe this horrendous image, uh, Donald Trump supporters, sorry, "Donald Trump supporters have been creating and sharing AI generated fake images of Black voters to encourage African Americans to vote Republican." What do you see here Alex?
Alex Hanna: Yeah, so I mean trying to describe this for folks who are audio only.
So this is Donald Trump in the middle of a group of Black people. He's got his arms around two Black women. There's all kinds of terrible aI artifacts here. The hands are all fucked up. One guy's ring finger is tiny. Um.
Emily M. Bender: I mean that, that could be a developmental difference, but probably not. Right.
Alex Hanna: Yeah.
Emily M. Bender: It's more likely an like AI artifact.
Alex Hanna: Yeah. There's got, um, like, uh, Trump's left hand is kind of webbed. Um, one woman is wearing a bonnet on her head, but the bonnet looks like a t-shirt or something weird piece of kind of things. A guy has a hat on there's there's illegible text on it. There's also like way too much fidelity in everybody's faces here and that so, you know there's kind of faces that are all in focus, yeah, it's it's just a classic piece of, of AI Hcell.
And I, I do want to mention the, you know, disregarding, um, the, the kind of election integrity thing. There was a recent piece put out by, um, Julia Angwin, Alondra Nelson, and, and Rina Palta um, that was, uh, in Proof News and also the AI Democracy Project, uh, entitled, "Seeking reliable election information? Don't trust AI."
Uh, and they've really, uh, I think this, the focus here, it was primarily on, I think, language models, but just goes ahead and further, you know, further proves the point. If you are a listener of this podcast, you don't need the convincing, uh, but they empirically went ahead and tested these models for typical election, mis- and disinformation.
Um, so yeah, anything around the election, it's just going to get worse and worse and worse.
Emily M. Bender: Yeah. Yeah. I think that the most hellish thing about this, um, BBC article is that we have now, you know, another clear example of people using deepfakes to try to influence things. And in this case, it's apparently not external actors, but actually American voters who are doing this.
Um, so form your networks of trusted people, find the people you trust, the sources you trust, be very clear about where you get information and, you know, build up that value of authenticity because we're going to need it.
Alex Hanna: All right. With that, I think we're at time. So I'm going to take us out. Our theme song is by Toby Menon, graphic design by Naomi Pleasure-Park, production by Christie Taylor.
And thanks as always to the Distributed AI Research Institute. If you liked this show, you can support us by rating and reviewing us on Apple Podcasts and Spotify. And by donating to DAIR at DAIR-institute.org. That's D A I R hyphen institute dot org.
Emily M. Bender: Find us and all our past episodes on PeerTube and wherever you get your podcasts.
You can watch and comment on the show while it's happening live on our Twitch stream. That's Twitch.TV/DAIR_Institute. Again, that's D A I R underscore institute. I'm Emily M. Bender.
Alex Hanna: And I'm Alex Hanna. Stay out of AI Hell, y'all.