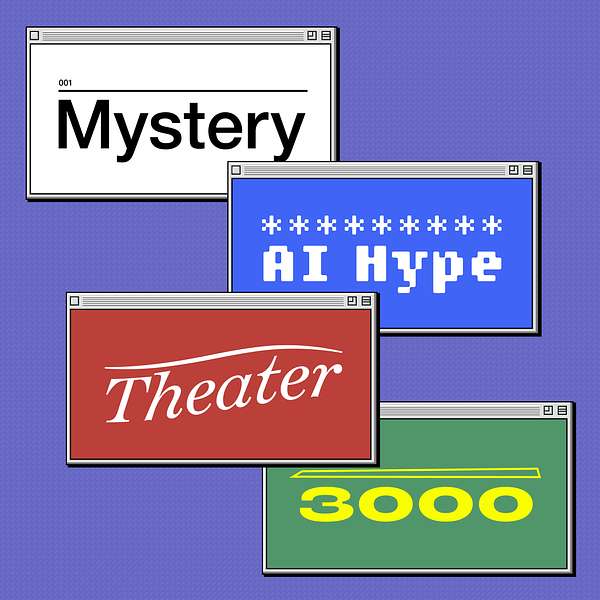
Mystery AI Hype Theater 3000
Mystery AI Hype Theater 3000
Episode 31: Science Is a Human Endeavor (feat. Molly Crockett and Lisa Messeri), April 15 2024
Will AI someday do all our scientific research for us? Not likely. Drs. Molly Crockett and Lisa Messeri join for a takedown of the hype of "self-driving labs" and why such misrepresentations also harm the humans who are vital to scientific research.
Dr. Molly Crockett is an associate professor of psychology at Princeton University.
Dr. Lisa Messeri is an associate professor of anthropology at Yale University, and author of the new book, In the Land of the Unreal: Virtual and Other Realities in Los Angeles.
References:
AI For Scientific Discovery - A Workshop
Nature: The Nobel Turing Challenge
Nobel Turing Challenge Website
Eric Schmidt: AI Will Transform Science
Molly Crockett & Lisa Messeri in Nature: Artificial intelligence and illusions of understanding in scientific research
404 Media: Is Google's AI actually discovering 'millions of new materials?'
Fresh Hell:
Yann LeCun realizes generative AI sucks, suggests shift to objective-driven AI
In contrast:
https://x.com/ylecun/status/1592619400024428544
https://x.com/ylecun/status/1594348928853483520
https://x.com/ylecun/status/1617910073870934019
CBS News: Upselling “AI” mammograms
Ars Technica: Rhyming AI clock sometimes lies about the time
Ars Technica: Surveillance by M&M's vending machine
Check out future streams at on Twitch, Meanwhile, send us any AI Hell you see.
Our book, 'The AI Con,' comes out in May! Pre-order now.
Subscribe to our newsletter via Buttondown.
Follow us!
Emily
- Bluesky: emilymbender.bsky.social
- Mastodon: dair-community.social/@EmilyMBender
Alex
- Bluesky: alexhanna.bsky.social
- Mastodon: dair-community.social/@alex
- Twitter: @alexhanna
Music by Toby Menon.
Artwork by Naomi Pleasure-Park.
Production by Christie Taylor.
Alex Hanna: Welcome, everyone, to Mystery AI Hype Theater 3000, where we seek catharsis in this age of AI hype. We find the worst of it, and pop it with the sharpest needles we can find.
Emily M. Bender: Along the way, we learn to always read the footnotes, and each time we think we've reached peak AI hype, the summit of Bullshit Mountain, we discover there's worse to come.
I'm Emily M. Bender, Professor of Linguistics at the University of Washington.
Alex Hanna: And I'm Alex Hanna, Director of Research for the Distributed AI Research Institute. This is episode 31, which we're recording on April 15th of 2024. Today's episode is going to be about science! And while we've talked about already about how LLMs are definitely not a replacement for human participants in social science research, there's unfortunately so much more bullshit out there.
Emily M. Bender: Think Google's claim that their DeepMind model could discover 'millions' of new useful materials, or the more general possibility of a, quote, 'self driving' robot-run laboratory. Even prestigious groups like the National Academies are buying into the hype, holding workshops to, quote, "explore the future of AI" as, quote, "an autonomous researcher."
Thankfully, we're joined today by two guests who can bring some much needed critique to this campaign to get scientists to place trust in AI. They're the co-authors of a recent piece in Nature examining the beliefs of scientists about the so called promise of artificial intelligence applications.
Alex Hanna: Dr. Molly Crockett is an associate professor of psychology at Princeton University. Welcome Molly.
Molly Crockett: Hi there. Thanks so much for having me on.
Alex Hanna: And Dr. Lisa Messeri is an associate professor in the Department of Anthropology at Yale University. Lisa just released a book about virtual reality, another much hyped technology, The Land of the Unreal.
Welcome Lisa.
Lisa Messeri: Hi, so great to be with you all.
Emily M. Bender: This is super exciting, and we got a lot to cover today, so I'm going to take us right into our first main course artifact here. Um, please confirm for me that you can see the Nobel Turing Challenge.
Alex Hanna: Yes.
Emily M. Bender: All right. (laughter) So, so much prestige in the title of this endeavor.
Alex Hanna: And I wanted to describe the, the image, too, the banner image is this, you know, Noble Turing Tech Challenge in this futuristic text uh, overlaid on a, um, kind of a space level view, orbital level view of lights at night, um, of the Earth. So yeah, just, just to give you a bit of a, an idea of the grandeur of the challenge.
Emily M. Bender: Yes, absolutely grand. It's the whole world, it's futuristic, it's in space, um, and we have in all caps, "The Nobel Turing Challenge is a grand challenge aiming at developing a highly autonomous AI and robotic system that can make major scientific discoveries, some of which may be worthy of the Nobel Prize and even beyond."
Um, and before we turn this over to our guests, I just want to say, I love the trope here of we're going to take sort of prizes and ways of doing evaluation of the work of people and use it to see how good the AI is, right? This, you know, 'could we win the Nobel Prize' or 'could this artificial scientist win the Nobel Prize' feels like it's just another example of 'did the AI pass the bar exam.'
Lisa Messeri: Yeah and I know-- I was gonna, I know that we're gonna get into the paper where this kind of emerges from, but kind of a nice little asterisk in the paper is it's not that the AI can win the Nobel Turing Prize. "In fact, we consider this successful if at least the Nobel Prize debates whether or not an AI can be, you know, can win a Nobel Prize." So there's there is humility in this project after all. (laughter)
Emily M. Bender: Um, okay, so let's, let's do a little bit more of the copy here. Um, so below that sort of headline we have, "Accomplishing this challenge requires a development of a series of technologies and in depth understanding on the process of scientific discoveries. From the system development perspective, the challenge is to make a closed loop system from knowledge acquisition and hypothesis generation and verification to full automation of experiments and data analytics."
What should we be thinking about in that very dense, two sentence paragraph?
Molly Crockett: I think it's such a good example of this vision that Lisa and I encountered in our research where scientists are so excited about the possibilities of AI to replace humans at every stage of the research pipeline, right? From hypothesis generation to experiments to analysis, and even to peer review and evaluation of the quality of the science, or in this case, should it, should it win a prize? And what's been so fascinating to us about looking at these visions is the ways in which it sort of, um, highlights the institutional pressures that scientists are facing, right? We're really pressured to be as productive as possible and to accomplish these superhuman feats of understanding increasingly large data sets that incidentally are being provided by AI tools and the regime of big data.
And, um. the intervention that we're hoping to accomplish is to help scientists see that actually the the human contributions to science are really valuable. And many decades of, uh, research and science and technology studies has revealed that.
Emily M. Bender: Yeah, absolutely. Um, all right. So do we, this, this is a live thing. Right here we have news they're, they're doing a workshop coming in July. "AI4Science and Nobel Turing Initiative Workshop," um, at the National University of Singapore. Oh, there's lots of things in 2024. There was a workshop in Tokyo in February, um, and then we're--now we're back to 2023 at Carnegie Mellon. So they seem to have looped in the interest of pretty prestigious institutions in many different locations.
Alex Hanna: Um, I would love to like read the actual challenge at the end, the copies at the bottom of the page.
Emily M. Bender: Yeah. Okay.
Alex Hanna: Yeah. So they say, "Overview: the Nobel Turing Challenge is a grand challenge for artificial intelligence and related discipline of science that aims at quote, 'developing AI Scientists--'" And AI Scientists is notably in caps. So these are "agents capable of autonomously carrying out research to make major scientific discoveries worthy of the Nobel prize and beyond by 2050," end quotes.
Okay. "The Nobel Turing science-- the Novel Turing Challenge is a challenge and a question. To develop--" So here's the challenge. "To develop AI Scientists--" In caps again, "--that perform research fully or highly autonomously and make significant discoveries. This involves defining research questions, understanding state of the art and relevant areas of science and technology, generating hypotheses, planning experiments, executing them, verifying hypotheses, and generating a series of new questions and further iterations. The successful development of AI Scientists--" In caps, should, I need to say this at every, every turn, "--shall enable us to make significant scientific discoveries at scale."
And so, you know, here, and then the question is, is, is, is, um, "Would AI Scientists behave like the best human scientists so that the Nobel Prize selection committee or peer scientists do not notice it is a machine rather than a human being? Or does it behave very differently so that AI Scientists--" all caps, "--making discoveries are very obvious?"
And so in this case, it is it is a a mimic of the Turing test. That's the where the the Turing is coming in, uh, and, and, and, and, uh, Emily and I were on another podcast, the Our Opinions Are Correct podcast direct, um, talking about the Turing test and the original, uh, 1950 paper that Alan Turing wrote, um, but it is a, a, a, a scientific copy here.
Now there needs to be judges to determine "whether the science that is done is fully AI or it is indistinguishable, indistinguishable from humans."
So, yeah. What are your thoughts on that?
Lisa Messeri: I mean, so, so many thoughts. So the, and this, this question, the question paragraph that you read, Alex, is so fascinating because it's this idea that like, so bought into this idea that the 'AI Scientist,' caps Scientist, is so autonomous, right is highly autonomous such that it's going to be also submitting its Nobel Prize docket or whatever, however you get onto the like visibility of the Nobel Prize committee without any kind of human intervention, or even that it's not a human directing an AI scientist, 'Oh, why don't you look at this problem space or that problem space.'
It's really the full fledged idea of this is an AI scientist who is working completely, you know, outside of where the, um, of any kind of scientific knowing and but then you get you stopped reading I think before it gets to like the caveat, right, which is if "If AI scientists exhibit a capability very different from human scientists, we may consider it as an alternative form of science or an alternative form of intelligence."
And that opens up a whole epistemological can of like, allowing this AI scientist as conceptualized by this like, you know, the people putting out this challenge could still be like, you know, um, something productive, even if it's doing something like wild over on the other side, because maybe it's just a different form of science.
And then it just raises the question so what do we even mean by science? Right? What is what is the what is being probed here? The you know, reading this web page, reading the other materials associated with it, is this notion of science is knowing the world, right? That's kind of what is undergirding this quest, is can AI know the world better than human scientists or in conjunction with human scientists?
And truly, what does that mean?
Molly Crockett: It's also interesting how this passage flips back and forth between the human like and the superhuman, which is a theme that we see a lot in all of the visions that we have looked at. Um, and in this case, you know, behaving like the best human scientists or differently. And, um, I think that those, um, those sort of two ideas, human like and superhuman are interestingly in tension here.
Alex Hanna: Yeah.
Emily M. Bender: I was already--
Alex Hanna: Go ahead, Emily.
Emily M. Bender: Um, the part that really caught my craw first was also, um, under the challenge, uh, "shall enable us to make significant scientific discoveries at scale." So I'm thinking, of course, of, of, you know, Alex has wonderful things to say about why scale is such a problematic goal, but also thinking about, okay, so we've gained a lot, um, across societies, um, if we only look at the positive, lots of good things have come out of scientific inquiry.
Not that there aren't problematic things as well. So, you know, more of those good things, yes, better. But, uh, that scale thinking is, I think, really problematic here, right? If we want to have more scientific discoveries happening, then, well, how do we think about education? How do we think about science funding?
How do we think about supporting the work of scientists and the communication amongst themselves of scientists? And not how do we automate it so we can just, you know, machine go brrr.
Alex Hanna: Yeah. And I mean, I think I was just touching on this, this thing I mean, you brought up Lisa is that the kind of idea and you brought it up before uh, we start recording, but the idea that the work of science is inextricably human, and I mean, what does it mean to necessarily scale something out that you can't really have humans involved in? I mean, how is that going to work in the human welfare? And what does that I don't, I don't know what the, the get is for scaling, you know, what is I think very much, very much, I don't want to call it necessarily a craft enterprise, but it is one in which, in which there is an element of discernment about what is important, right?
And that, that's quite, that's quite critical.
Um, I also want to point out this last sentence in the question, which is, "Then a collaboration between human scientists and AI scientists may perform better than human or AI scientists alone because of diversity in the form of intelligence."
And it's such a like kind of a throwaway line at the end of the sentence, the question, because you know, what the hell does it mean to say diversity in this sense? Especially when large language models are trained on a bunch of existing text, and that text is mostly in English.
That surely does not signal any kind of real diversity. It's, it is, it is more of a reinforcing mechanism. Um, and, you know, just which is which is degrading over time. And yeah, that's not, you know, when we kind of say talk about like diverse teams as being more successful, this is certainly not what we're talking about.
Lisa Messeri: So in our, in the paper that Molly and I have written, this is one of the points that like, we were most kind of stunned by in many of these, in many of these papers, part of what we're doing is we're saying, okay, let's say that this AI scientist comes to be, let's say that all of these visions that scientists have for how AI can benefit science, let's say let's not get hung up on hallucinations and the current kind of technical issues.
Let's say you can achieve these kinds of autonomous agents producing science at scale. What does that mean for the world that we then live in? What does that mean for the project of science that we then live in? And one of the things that we kind of propose is that one of the big risks, epistemic risks that remain is that we might be in a monoculture of knowers where it seems as though, because these agents are multiple and, and, and, you know, working with humans that, that this illusion of diversity seems as though the box is checked.
Yes, we still have a multiplicity of knowers, but precisely Alex, for what you just said about these AI models being trained on very particular data sets. And by machine tool makers who have themselves a very particular way of imagining solutions to problems. Instead of this diversity, you in fact get a narrowing, but the fact that in this proposal, diversity is being emphasized is exactly the illusion that our paper goes back to, right?
And that where we see the main risk is that people think they're bringing diversity into the system, that they're including diversity. But the way AI gets cast as these human and superhuman is exactly what allows these illusions to pervade.
So it's this incredibly tricky um, cycle that we see in these, in these proposals.
And to the point about scale, another piece that we came across--and again, the work we were surveying, they're all in Science, Nature, you know, these top um, top tier journals-- was someone who was imagining, okay, yeah, let's say we have these AI scientists, let's say we have these, you know, at scale, you know, AI bots producing science left and right.
That, that research scientist went into the, kind of stumbled into the obvious, which is, oh, well, they're going to therefore be talking to each other in a way humans ourselves can't fully understand or process. And so the whole notion of, say, even the journal article goes away and all we have are repositories where AI scientists can kind of deposit their knowledge in the language they understand and other AI scientists kind of pick it up and bring it back out, as if this is a good thing.
Alex Hanna: Yeah.
Emily M. Bender: Is it, is it even science if it isn't communicated among scientists like real scientists?
Alex Hanna: Right.
Emily M. Bender: Ah, okay, so this, we were just looking at the web page, which is continually updated for the Nobel Turing Challenge. There's also, um, an article in NPJ Systems Biology and Applications, which is a Nature property, um, from June of 2021.
And it's, it's kind of interesting to, you know, to put ourselves back in the mindset. There was already AI hype going on. Um, this is, you know, the, everyone was full steam ahead on large language models, but not so much on large language models as synthetic text extruding machines, um, we're still, you know, a, a, a year and some from the release of ChatGPT, and yet here's this article, um, full of this, this idea that we could, we could build an AI that could do our science for us.
So I'm going to, I'm going to start us with the abstract here. "Scientific discovery has long been one of the central driving forces in our civilization--" 'Our civilization,' I love that singular there, right? "It uncovered the principles of the world we live in and enabled us to invent new technologies, reshaping our society, cure diseases, explore unknown new frontiers, and hopefully lead us to build a sustainable society."
Um, so again, we've got science as um the agent in all of this, rather than scientists, which is icky.
Okay. "Accelerating the speed of scientific discovery is therefore one of the most important endeavors. This requires an in-depth understanding of not only the subject areas, but also the nature of scientific discoveries themselves. In other words, the science of science needs to be established and has to be implemented using artificial intelligence systems to be practically executable."
So.
Alex Hanna: This one is, this one is, I mean, first off there already is a field called 'science of science.' Um, and it's, it's kind of like, um, the sociological study of science kind of with computational methods.
Um, and, and so for instance, I know Bernie Koch is someone who I've co authored with, um, you know, is very interested in kind of the architecture of like deep learning and how deep learning and benchmarking kind of co evolved, um, and or were enabled by that.
Uh, and so it's the use of those data sets, uh, and uses of kind of other tools from science and technology studies and methodologies, uh, so it exists.
Um, but then--
Emily M. Bender: And it doesn't have to be implemented using AI systems.
Alex Hanna: Yeah, that was my next thing. It has to be executed with this, which I'm like, well, that is quite, quite a, quite a, you know, a demand. But yeah, I just needed to get that in.
Emily M. Bender: No, I, I figured we'd be breaking there. Um, Lisa, Molly, anything you want to say about the first half of that abstract before we continue?
Molly Crockett: I, I want to talk about the, the inevitability that is just sort of, oozing through all of the language here and also the idea about accelerating the speed of scientific discovery, which again sort of feeds back into this like push for more and more and more and more and more and more and more productivity.
And, you know, speaking of science of science, I think one of the pieces of the literature that Lisa and I have been interested in throughout this project is that, you know, more science does not necessarily lead to better of, better understanding of the world. So the sort of tagline of our paper is 'producing more while understanding less.'
And there have been some really interesting papers suggesting that as, um, as you see a sort of deluge of publications entering the literature, um, that this, in, in many ways, can reduce innovation, reduce creativity and sort of scientists who are faced with the deluge of literature just tend to cite, uh, cannon, um, and, um, narrow the, the scope of their investigation, sort of fixating on the, uh, widely accepted principles as opposed to innovating.
So I think we do want to question this, whether more productivity, whether that is assisted by AI or not, is something that actually does help us understand the world better.
Lisa Messeri: And what a good point about, like, um, citing canon, because in the, you know, in the first paragraphs of this paper, which I hope we don't have to go to in too much detail, because, uh, the citations that he does use is Karl Popper, Thomas Kuhn, Paul Feyeraband: like he took an intro to philosophy of science probably when he himself was an undergrad.
And like, if you are at all a serious thinker about the science of science, you would put a huge asterisk by these thinkers of themselves operating in their own paradigm and their own moment when we were trying to understand science. It's like citing Einstein in, you know, in a physics paper, which is like, yeah, it's relevant, but it's not where you're going to get innovation.
It's not where you're actually going to push your understanding of how science works in this case.
Alex Hanna: Yeah, that's such a good point. And it's sort of, you know, like, going back to this, and then also kind of going back to--the next paragraph, then cites a number of different kinds of projects, which I think are, I think they've, I mean, are these are any of these still in operation?
So it says, "Not surprisingly, scientific discovery has been a major topic in artificial intelligence research, which dates back to DENDRAL, META-DENDRAL, followed by MYCIN, BEACON, AM and EURISKO." Um.
Emily M. Bender: I think if we look at the dates on these, it's gonna be 1980s.
Alex Hanna: Yeah, yeah, yeah. These are quite old.
Emily M. Bender: Yes, 1984.
Alex Hanna: These are quite old like expert, uh, like expert systems.
Emily M. Bender: Yeah. Here's One from 1993.
Alex Hanna: Oh, there you, there you go. There you, yeah. 1993. DENDRAL. The first--
Emily M. Bender: I'll take us back up to where we were.
Alex Hanna: The first expert system, and then this continues to be the ma-- one of the main topics of, of AI. And then they set that the Gill paper, which we're, we're gonna address next time.
And then, then I actually two papers from Gill. I'm, I'm surprised he didn't, um, you know, cite a, um, uh, Galactica.
Um.
Emily M. Bender: Well, uh, because this is 2021. This is pre Galactica.
Alex Hanna: Oh, that's right, that's right.
Emily M. Bender: By a year.
Alex Hanna: Oh, yes, yes, yes. Yeah. And so it's, it's, but it, yeah, exactly. So there's this kind of huge, huge jump here.
These things in the 80s, and then, you know, has there been any kind of development in the interim? Um, which is, yeah, I mean, well, I guess in the 80s, then you had this kind of, you're, you're getting into AI winter territory right there.
Lisa Messeri: And, you know, there's an interesting, like, question of why is this person writing this paper at this time?
Also, who are creating the visions of AI for science? Is it scientists who are just excited about, like, hoping that they can kind of overcome where they know their own field's limitations are? Or is it AI researchers who are like, we need to use, like, here's a great use case, you know, let's, let's kind of explode it onto the scene.
And I, I didn't do enough, sorry, like research on Kitano. He's a, he's like on the border of these things, cause actually if you like scroll he's at the moment that he writes this, this conflict of interest notes that he is in fact, the editor of "Systems Biology and Applications," but he also seems to be more robustly trained as an AI scientist.
So, you know, he's someone who no doubt is passionate about AI's potentials for science. But if you're like, but what does it mean to be writing that claim when your position is as an AI researcher versus when you're writing that claim as a scientist? And again, the visions converge, like AI, AI researchers and scientists without AI training do kind of stumble upon similar imaginations for the, how AI could develop their, um, could help develop their portfolio, but it really matters that this person also is associated with Sony, um, and is coming from a corporate setting in addition to no doubt his genuine academic interests and, um, you know, possibility uh potentials.
But yeah, so it says that at the moment, the author is editor-in-chief of this journal in which this is, this is published in, which is fascinating.
Emily M. Bender: And you wonder why Systems Biology and Applications would be the place to publish something that's supposed to be across all science, right? It seems very specific.
Um, and you know, when you say, is this coming from scientists who feel a need to improve their practice of science, or is it coming from AI researchers who are looking for a way to prove how smart their AI is, um, and I think that you hit on it by, by referencing Sony here, is that, when we talk about AI researchers, we absolutely have to keep in mind that a lot of that is happening in industry and sort of for corporate interests.
Um, I got to do this thing, speaking to deans of the Pac-12 universities. Um, and there's this question in the air of what happens to this meeting next year when the Pac-12 is no more, but anyway, I got to speak with them. And one of the points that came up because they're all trying to figure out what to do about this.
And I got to say to them, this is becoming your problem right now, not because there's been some big breakthrough in technology, but because there's a whole bunch of money pushing this across lots and lots of sectors. And I think it helps, I think we're better able to react if we see where this is coming from more clearly sort of pushing aside the hype.
Um.
Alex Hanna: Yeah, and it's clear. I mean, it's not, I mean, Kitano is not just the affiliated with Sony. He's CEO of Sony AI. Um, so I mean, it's, you know, it has a clear vested interest in this. And yeah, I mean, this point you make Lisa is great. I mean, if it was the case that there were a set of scientists who were looking at a very well scoped problem, um, approached some researchers and said, we would like some aid in furthering this in a particular sort of way in developing some tools, um, in a pretty narrow way, maybe not necessarily to develop hypotheses, but to see if we could take this kind of intractable computational problem and, um, you know, process and churn some data with it.
Yeah. I mean, I think that's a pretty good use of AI tools, but in this case of we need an end-to-end closed loop solution in which robots are talking to each other, yeah, then it looks like something completely different.
Emily M. Bender: It's, it's not a good use of AI tools 'cause AI tools aren't a thing. Right. Um, it's a good use of, of automated pattern matching in some cases, right? And arguably, I mean, you all probably know this better than I do, but I think that's a good description of what was going on with AlphaFold. So there's lots and lots of data that's been carefully curated by humans doing science, um, mapping between protein structures and amino acid sequences, if I've got the underlying details right.
And the question is, okay, given that, given the patterns in that, can we use automated pattern matching systems to come up with a, a, good sort of starting point for the next ones that we're looking at. So is that--
Molly Crockett: Yeah, there's a really great commentary that I saw recently by Jennifer Listgarten in Nature Biotechnology. The title of the piece is, "The perpetual motion machine of AI-generated data and the distraction of ChatGPT as a scientist," and she talks about AlphaFold as a really good example of an extremely rare use case for AI that actually just worked out super well because there was this very particular data set that lent itself well to the kind of, of, of problem that that class of models can solve, but that it's, um, a mistake to sort of generalize beyond that one case because there were so many highly specific features that contributed to its success and that most problems in science are not going to be like that.
Alex Hanna: Yeah.
Emily M. Bender: Yeah. And when you call it AI and then you call ChatGPT AI, it looks like this is all the same thing. We found, um, this is a few episodes back, I'd have to dig to see which one it was, but we found a paper that was doing a review of the use of ChatGPT in dentistry, and in the abstract they claimed that it could read dental X-rays.
This text processing system.
Um, and eventually dug through and found out that they were pointing to another review article that was looking at applications of AI in dentistry, and there was some computer vision applications looking at dental X-rays, and they equated AI and so on, and just, you know, yeah.
Lisa Messeri: Well, and that's the problem with 'the AI scientist.' Like it, it groups into all of these very discrete things and it makes it really hard to figure out where are the good applications if you're allowing AI to be this monolith and not breaking it down into its different functions its different possibilities.
It really is a hindrance to actually trying to do something interesting or useful with these, you know, with these modes of processing.
Alex Hanna: Yeah
Emily M. Bender: Yeah. All right. So scaling science is bad for science and scientists, AI science is bad for science and scientists, and AI science is bad for the application of, you know, automated pattern matching in science.
Alex Hanna: Yeah. Yeah.
Molly Crockett: Yeah. I mean, I think especially I might, I might especially say like narratives about AI, right? Like Lisa and I, I think are pretty clear in our paper. We're, we're not saying that tools that would fall under the large umbrella of AI are never going to be useful for science. Like, obviously, they are.
We can point to a lot of examples where they're useful. And I use, I use machine learning in my own research. Um, but I think that what we're really worried about are narratives about what AI is and what it can do that are being, um, in many, um, in many senses, like driven by industry that are then capturing the imaginations of scientists and putting this sort of institutional pressure on to scientists to incorporate these tools into our research pipelines, oftentimes, without a good sense of, um, what their limitations are, and without the space and time to really have the conversations that we need to have to determine when it's going to be useful and when it might, um, actually lead us astray.
Alex Hanna: Yeah. And I mean, this is the thing that I think we run up against a lot. And I mean, I, I appreciate Emily raising that Pac--that Pac-12 discussion. There's a lot of this rush, you know, from from deans or other university administrators and saying, how can we teach you know, students, how to use these tools, lest they be left in the dust.
And we hear this from different quarters, too. And we're kind of like, well, you know, these things could be if these were scoped down pretty narrowly, you could have someone kind of help you do these things. But then the way that these are marketed, then they appear to be, you know, there's actual conversations in which an equity concern that was said is that like, oh, people have, you know, equal access these to these tools.
I'm like, well, you know, like that's, that's not, that's not what, that's not the point. You see, these tools are going to exacerbate equity in other types of ways, especially, you know, according to bias in the way that these things will perpetuate bias around social social lines. Um, but it's the case that if you actually need this stuff to work, you need to actually then have kind of bespoke uses of certain pattern matching tools.
Um, and then if you're left with a ChatGPT, you're going to get this pretty crummy type of mimicry of whatever garbage it produces.
Emily M. Bender: Just hearing from a student about a colleague that he's working with who was using ChatGPT to look up formulas and, and code the formulas. And this is a, a, a former student of mine who, uh, went and double checked and it was doing things like replacing a divided by with a times and getting stuff wrong.
You know, it's not it's not helping the progress of science there. All right, you're you're co--go ahead Molly.
Molly Crockett: Oh, no, I was just saying yeah that that is absolutely terrifying and and I think you know the the worries about hallucinations and errors in AI systems are something that all scientists are thinking about.
Um on top of that though it's important to recognize that even if engineers solve the problems of errors and hallucinations, we're still going to have risks to science because, as Alex was mentioning, um, there's a lot of prestige around using AI in science right now. There's a lot of funding being poured into it.
And I think in the social sciences in particular, there's a real risk that because there's so much hype and prestige around AI that scientists using those, those approaches in their work are going to, um, get more resources, get more power, and crowd out or foreclose the types of research questions that you really just can't answer well with AI models.
Like, you know, there's, there's so many, um, aspects of human behavior that just don't lend themselves well to computational approaches. And, um, what, what we talk about in our paper is, is what we call the illusion of exploratory breadth, where scientists come to mistake the, um, full set of questions you could ask about the world with those questions that you can ask using AI approaches.
And so the worry then is that because of the hype, because of the prestige of AI, scientists forget that there's this whole set of questions that we could be exploring that need other methods to address those questions. Um, and that's, that's contributing to, you know, monoculture of knowing.
Alex Hanna: Yeah.
Lisa Messeri: And this is also going back to Alex's point about the hazards of 'at scale,' of this, being able to scale, right.
My, my primary method is ethnography, which is a method that does not scale. Um, but I still think the long duration, close collaboration with humans as a mode of generating knowledge is an incredibly important, um, kind of knowledge production. I mean, it is why anthropology is on the bottom of the, uh, the pecking order of the social sciences, because we can't scale, um, but it would be, a tragedy to lose that and other forms of knowing, all of which help pursue what I think science is about, which is, you know, better reckoning with the world, not knowing what the world is or isn't, but providing heuristics that we can use to navigate what is a deeply complex place in which we all live.
Alex Hanna: I'm really, I'm really, um, regretting the day, which I know I feel like I'm going to speak this and I hope it doesn't speak it into the world, but surely there will be someone who takes those little Humane AI pins and then puts it in the room--
Emily M. Bender: No, no, no, no.
Alex Hanna: --and then says it's doing ethnography or something.
Yeah.
Emily M. Bender: Ok. So I want to give a shout out to our newsletter, um, podcast listeners, if you haven't noticed, we have a Mystery AI Hype Theater 3000 newsletter now. And the most recent post as we're recording this, um, is me imagining my ideal response of a university to all the AI hype, which is to double down on funding across disciplines and especially the humanities and social sciences as ways of knowing as the correct response to all this rather than funneling all the money through AI, which means through computer science. All right. Um, so picking up, there was a really great segue, Molly, a little bit back, um, to this National Academies thing where you were talking about pressure um, in our institutions to do this.
So we have a little bit of time to look at how, um, the National Academies for Science, Engineering and Medicine, at least some portion within them basically got sucked into this challenge. Um, so this is the, the PDF from NationalAcademies.org describing, "AI for Scientific Discovery -- A Workshop," that was held in Washington, D.C. last October. Um, and I think it might be helpful to read the, I'm going to read the, the, the goal for this meeting. So the goal for this meeting is "to explore the future of AI in terms of its role as an autonomous researcher performing scientific discovery. This includes where AI stands, where it needs to go, and which disciplines should invest more in utilizing AI scientists. The workshop will also explore the ethical aspects and potential pitfalls that loom for AI scientists."
And by AI scientists, they don't mean scientists researching AI. They mean these, um--they didn't capitalize though.
Alex Hanna: Yeah, they didn't capitalize it.
Emily M. Bender: "This workshop is intended to be inclusive to the global community and will engage with international partners."
Um, and so they're, they're, um, it's a two day thing. They have, "What is the goal of an AI scientist?" In parentheses, "in conducting independent research." And that one, um, Hiroaki Kitano, our hero from the first part of this episode, um, is one of the speakers.
And then, "Global scientific discovery in the AI age."
Um, "Enablers - what are the gaps in AI that would prevent us from achieving independent scientific discovery?" Um, uh, "Societal aspects of AI: Barriers and opportunities, inclusive of regulation."
Um, Um, so this is the like, oops, we got to do ethics session. Um, "What are the gaps in automated physical experiments and experimental data collection and curations that would prevent us from achieving independent scientific discovery?"
That's just the conceptualization of it is, but, okay. Sorry. Um, And then on day two, "Examples of grand challenges, AI applications, domains. What is impossible currently in supporting the AI researchers slash scientist, small steps in the direction of grand challenges, examples of AI pilots and small wins," and then "Opportunities and where to make investments in AI."
So, in just a few minutes, what do we want to dig in here the most?
Lisa Messeri: Well, let's talk about the ecology of how this comes to be and the connections with what we were just talking about, right? So the piece that appeared in one of the Nature journals about the Nobel Turing Challenge written by Kitano, it is now breathed into, not only in Kitano being an invited speaker at this panel, but the language of 'AI scientists.' Of 'autonomous discovery,' right? All the language that is in that piece that we just read and dissected now here is naturalized by being part of a National Academies of Science, um, of science program, and even to talk about the ethical risks or any of the other, you know, problems in the conceptualization already takes the conceptualization as a kind of a productive way forward.
So, you know, the deflation of the hype has come too late, uh, because it, you know, it has already had all these after effects. But I find it just totally stunning that you can write an article in like, you know, a random Nature journal and then two years later have it be the subject of a National Academies workshop.
Like, there's some really interesting power that is, that had to be mobilized to go from point A to point B. And as an ethnographer, I would love to dig into it, um, but it wasn't all readily apparent via Google. You would actually have to be there and be talking to people to figure out some of these answers.
Emily M. Bender: In, in real time without scaling, right?
Lisa Messeri: (laughter) Yeah.
Alex Hanna: I mean--
Lisa Messeri: It would have taken two years.
Alex Hanna: It's so curious too. I mean, just to see the different partners here as well. I mean, because it's not just National Academies on the header. There's also the Office of Naval Research and the, also the NSF. Um, and these have been organizations that have provided legitimacy to different types of-- different types of AI projects. Um, and so, but then you also look at the titles of people. Um, you know, some of them are university based, but then there's also, um, different, um, different companies, different startups, um there was someone from Palantir speaking on day two. Um, yeah, their, their, uh, their, uh, head of--Anthony Bak, head of AI and machine learning.
Um, there's Google, um, head of Google Research Kenya. Um, I will shout out a, uh, kind of--
Emily M. Bender: I love how the global partners are Google.
Alex Hanna: Yeah. Well, I would, I would say that one, you know, one person that he involved was Vukosi Marivete, who is chair of data science, data science at the University of Pretoria. And had also been very involved in the, in the, uh, Masakhane project, um, for African languages.
Emily M. Bender: Yeah, Vukosi's amazing. I was sort of surprised to see his name here. I hope it wasn't a terrible experience for him.
Alex Hanna: Right.
Lisa Messeri: And Deb Johnson also is an amazing, uh, ethicist of technology.
Alex Hanna: Yeah. And we have these individuals, though, in the, 'Oops, we must pay attention to the social risks,' um, but embedded within the larger kind of scope of 'we need these AI capital as scientists, uh, creating their own labs doing X, Y, and Z.'
Um, and it's, yeah, it's infuriating that there's at least infrastructure provided here. Um, and there's air given to this, this kind of, this kind of project.
Emily M. Bender: Yeah. Molly, anything you wanted to add on this one?
Molly Crockett: Um, I, I think that what is especially interesting to me about this workshop is that, um, and I tuned into a little bit of it.
And my impression was that it, it really was a group of, of people coming together, um, from different sectors, science, government, industry, but there was a strong focus about where should we put our money? Where should we invest to reap the best payoff of AI scientists? And, um, I, I thought that was really, really interesting and, um, and consistent with the way that, that Lisa and I have been sort of forming impressions about this space.
Emily M. Bender: That also sounds like the logic of effective altruism on the other TESCREAL stuff of how do we, how do we optimize.
Molly Crockett: Oh yeah, that's, that's a really good point.
Emily M. Bender: So I've just, I've just highlighted one thing here under "Global scientific discovery in the AI age" and like, I have written a paper where I've had to put the title "in the age of AI," and hated doing it after the fact, but, um, like sort of labeling it this way again, sort of says, this is what's going on now. Everything else, it feeds into what you're talking about before Lisa of, um, you know, this is, uh, anything you can't questions you can't approach with these methodologies sort of end up devalued and unexplored.
But okay. So, uh, there's this claim, "AI has already made a substantial impact on the research enterprise in disciplines spanning materials research, chemistry, climate science, biology, and cosmology." Um, so picking up already what we were saying about calling all of this AI sort of blurs together distinct approaches and lends credence to things that, that shouldn't have it because of the shared naming, but also we wanted to call out that the material stuff ain't all that.
Um, so there's this reporting from 404 Media, um, I think reporting on, um.
Alex Hanna: It's a reporting on a on another paper. Um, well, if you go down a little bit more, it's by, um, these two individuals.
Um, oops. Skip this newsletter thing. Um, this Perspective paper published in Chemical Materials. So Anthony Cheetham and Ram Seshradi at the University of California, Santa Barbara. Um, so they report, they're, they're, they're looking at this one DeepMind paper that had published in Nature, in which they had produced-- DeepMind and some researchers from Lawrence Livermore, um, had said that they had generated 2.2 million new, um, materials. And so then this paper by Cheetham and Seshadri says they took a sample of 380,000 of them and said that none of them meet a three part test of whether the proposed material is credible, useful, and novel. Uh, they believe what DeepMind found are crystalline inorganic compounds and should be described as such, rather than using the more generic label material, which they say is a term which would be reserved for things that quote "demonstrate some utility," which is, woof, really harsh.
Emily M. Bender: And requires a human perspective, right? Because utility to whom? Utility to some human purpose.
Alex Hanna: Yeah. And there's a great quote in this article by Cheetham in which he says, um, "If I was looking--" And this really highlights the kind of nature of needing humans in this process, but he says, "If I was looking for a new material to do a particular function, I wouldn't comb through more than 2 million new compositions as proposed by Google. I don't think that's the best way of going forward. I think the general methodology probably works quite well."
So he's not saying like, yeah, maybe this pattern matching could be good, "but it needs to be a lot more focused around specific needs. So none of us have enough time in our lives to go through 2.2 million possibilities and decide how useful that might be."
And so, yeah, basically, you know, really taking this and effectively saying, and then there's a little bit more down in this article, uh, in which there's another, um. In which the author of this article, which I think was Jason Koebler, and he says, "AI has been used to flood the internet with lots of content that can't, cannot be easily parsed by humans, which makes discovering human generated high quality work a challenge. It's an imperfect analogy, but the researchers I spoke to said something similar could happen in material science as well. Giant databases of potential structures don't necessarily make it easier to create something that is going to have a positive impact on society."
And this is a, like, really a great point here.
From, um, and I think this is kind of from Palgrave. Um, he's another material scientist. "You're basically creating junk at scale and taking humans out of the process." He's just saying, you know, you don't know what's actually useful here. And so what are you doing when you're saying having AI capital S Scientists?
Lisa Messeri: And this is like the whole process problem of de-skilling that goes alongside in these conversations, right? What does it mean to de-skill science and scientists in this way, such that, because right now you have the great quote of someone who has the intuition to know of the 2.2 million, you know, of that, problem space, um, I as a scientist who's been trained and thought about this for decades, I actually kind of know where to look, right. Which is something that when the AI scientist is approaching this problem thinks is not possible, right? Oh, no, the most efficient way is clearly to search the whole space as efficiently as possible, as opposed to kind of heuristically narrow it down.
And what happens when you get more and more reliant on AI earlier and earlier in the process? What happens to that kind of intuition, which is so much of what scientific work is.
And again, like this becomes the really important interventions that come from like feminist science studies, which is to say that science is a human process is not to delegitimize it. It's actually to show where its strengths are and understanding that humans do science. And the training that goes into that is what makes science a creative and impactful endeavor. Um, and to assume that all of that can just be replaced by these, um, these programs really misunderstands what science is.
And also if you begin, I mean, this is something that Molly and I talked about isn't in the paper, but something that I've been like, you know, waking up in the middle of the night over, like if all of a sudden what it means to be a scientist is to be an AI minder, who's going to want to be a scientist?
And what would that mean therefor, for what science becomes in the future? So I just, I'm just also nervous just about the like uh, what this, what this is, what this is doing to the impression of what scientists are in the broader public and to the kids who I really want to still become scientists.
Alex Hanna: Yeah.
Emily M. Bender: So Lisa, you've just articulated what I was going to prompt Alex with for, um, for her segue into Fresh AI Hell.
So Alex, I need a musical genre in which you would like to sing frustration today.
Alex Hanna: Wait, you wanna, you wanna, I should provide the genre?
Emily M. Bender: You provide the genre and then I'm gonna tell you what you're singing about. But it's a genre for frustration.
Alex Hanna: Oh, absolutely Riot Grrrl.
Emily M. Bender: Okay, so you are singing in the style of Riot Grrrl.
You are one of these AI minders working with an AI, capital S, Scientist collaborator who's getting it all wrong. Go.
Alex Hanna: Oh gosh, okay. Um, I'm trying to think of what, uh, uh, oh gosh.
(singing) You think you're so cool with your AI tools. You want to go back to school and make materials, but you're not gonna, they're all junk crystalline inorganic compounds.
Most of them are fake. Most of them are fake. You're a fake.
(speaking) Okay. That's all I got. I did, I sang Decepticon by Le Tigre at Live Band Karaoke this weekend. So I was very primed for that.
Emily M. Bender: Love it. Love it. Okay, that brings us to Fresh AI Hell. The first thing, a very on topic, if we think about Galactica, which kind of only came up earlier, there's this piece in Forbes written by Bernard Marr, um, whose tagline here is contributor. I'm not entirely sure they're a journalist. Um, anyway, this is a headline, "Generative AI Sucks: Meta's Chief AI Scientist Calls For A Shift To Objective-Driven AI." And this is coverage of a, um, I think a talk by Yann LeCun. And what I particularly enjoyed in here was this Yanni-come-lately moment. Um, so under the subhead, "The Shortcomings of Generative AI," uh, midway down the paragraph.
Um, "Yann LeCun makes the point that large language models, the foundation of today's generative AI tools, are capable of producing text with superhuman abilities in narrow domains, but fundamentally operate by predicting the next word based on preceding input," you don't say, "a process that lacks the ability to genuinely understand context or engage with the physical world, leading to outputs that can be impressively fluent, yet often devoid of factual accuracy or common sense understanding."
So says Yann LeCun in 2024, but check him out in 2022, with the release of Galactica, uh, he quote tweets Papers With Code. This is November 15th, 2022. So just pre-ChatGPT. "A large language model trained on scientific papers. Type a text and Galactica.ai will generate a paper with relevant references, formulas, and everything."
So. (laughter) And I believe that yeah, go ahead.
Alex Hanna: Well, yeah, no, I mean, it's, it's, um, I, I, I want to make this hashtag Yanni-come-lately, just because I think you, there's a lot of instances in which, um , just Yann LeCun himself has sort of backtracked without acknowledging his own, uh, you know, his own mistakes, um, but yeah, I mean, he, he was very defensive of Galactica, I think there's some other, your next tweets here, Emily, uh, of him where he said, um, yeah, so it says "following a text Galactica--" Um, oh, what happened?
What happened?
Emily M. Bender: What happened?
Alex Hanna: Oh, yeah. "Following a text, Galactica spits out a prediction of what a scientific author might type, thereby saving time and effort. This can be very helpful, even without being completely accurate." What?
Emily M. Bender: What? "The usual disclaimer applies, garbage in, garbage out, prompt it with lunacy, get lunacy."
This is back in November 2022 when he was all defensive. It's like, no. It's fundamentally synthetic text. It is always garbage out. Just sometimes it happens to look like it makes sense. And then this one is, uh, from this year. Um, yes. Yann LeCun says January 24th, 20--oh no, sorry. 2023: "Galactica happened. We saw to Twitter mediated backlash and knee jerk prophecies of impending doom fueled by anti-Meta prejudice. Yet Galactica doesn't make shit up," shit bleeped, "any more than ChatGPT."
Alex Hanna: Yeah, yeah.
Lisa Messeri: They're both terrible is what this is saying.
Emily M. Bender: Yeah.
Alex Hanna: So yeah, effectively people hate it on us because we're we're Meta, and and then but you know, both of them are bad like why doesn't you know and so right here we just got the it's just the full sour grapes.
Emily M. Bender: Yeah. Anyway, I thought, I thought this was hilarious. And like now he's saying, Oh yes, AI is going to happen. We're still going to have all this great stuff, but it has to be done differently. And so the question is, is that actually acknowledging that the hype was a problem? Or is it trying to like, is it reading the tea leaves that this bubble's about to burst and trying to like drag it out for a bit longer?
Alex Hanna: Yeah.
Emily M. Bender: We'll see.
Okay.
Molly Crockett: I think these are so interesting and such a good example of the vision of AI as an oracle, that you can type in a question and it will give you back an objective and comprehensive answer and what, what might be an interesting feature of this commentary on the vision is that even as there is some acknowledgement of the limitations of the current iteration of the vision, there is nevertheless a deep faith in the vision itself, right, the idea that, well, one day we will get to AI as an oracle, and that is a good vision to have.
Alex Hanna: Yeah, yeah, 100%.
Emily M. Bender: Like, as you're saying, that it's A, possible, B, inevitable, and C, beneficial. And we want to disagree on all points. All right, also under the rubric of scientific applications of AI, this is an article from January um, on CBS News under the tagline Health Watch, the headline is "Mammography AI can cost patients extra. Is it worth it?" Um, and basically there's this upselling.
I have all these pop ups, so the, the start, I don't have a journalist name here, do I? Yes, Michelle Andrews. It's Michelle
Alex Hanna: Andrews, yeah.
Emily M. Bender: Yeah. Uh, so Michelle Andrews writes, "As I checked in at a Manhattan radiology clinic for my annual mammogram in November, the front desk staff reviewing my paperwork, asked an unexpected question. Would I like to spend 40 dollars for an artificial intelligence analysis of my mammogram? It's not covered by insurance, she added." So there's this, like, upselling, um--
Lisa Messeri: Could I spend 40 dollars to not have AI read my mammogram?
Alex Hanna: I know, right? I know.
Emily M. Bender: Tell me exactly what it is that you're using, how it was developed, how it was evaluated, and then I'll decide, right?
Alex Hanna: But this is really interesting, the next part of it, which is says, "I had no idea how to evaluate that offer, feeling upsold. I said, no, but it got me thinking, is this something I should add to my regular screening routine? Is my regular mammogram not accurate enough? If this AI analysis is so great, why doesn't insurance cover it?"
And there's no question being like, why do we need to add this at all? Is this, why are, you know, this, and it seems like if insurance isn't covering it, it's I mean, insurance doesn't cover a lot of things, but if it's if it's not supposed to, I mean, it seems like a cash grab by the, the radiology clinic.
Lisa Messeri: You know, it is a startup company who got VC funding, who said, here's a use case, AI for mammograms, went around, sold it to these clinics and then the clinics are in turn selling it. So we can go, I mean, like, it's just so clear what's happening behind the stage here, which has happened in so many other instances. But I think you're right, Alex, like that, seeing how this journalist or this writer is reasoning through what this means to even be offered it, adds like a whole wonderful dimension to why we need to be so vigilant about these, you know, these, um, startups that might be like shit posting on Twitter, but then actually work their way into this clinical setting and have actual people ask, oh, should I be asking for this?
Right, so it's the same way we saw the paper, the Nature paper leading to the National Academies of Science workshop. Here we see this kind of like startup company leading to the front desk of a hospital without any one asking, is this possible? Is this good? You know, what kind of caveats do we need to accompany these claims of knowing?
Molly Crockett: Yeah, and also the way that the introduction of this AI analysis makes the patient question whether the regular mammogram is not accurate enough and wondering whether, again, adding more AI gloss to science will then make any approach, any method that's not using AI to seem like it's less scientific.
Emily M. Bender: And the marketing leans heavily into that.
So in this, couple paragraphs down, there's another person um, who was handed a pink, of course, pink pamphlet that said, "You deserve more: more accuracy, more confidence, more power with artificial intelligence behind your mammogram."
Alex Hanna: The, the pink, the pink pamphlet, just really leaning into the, um, breast cancer marketing industrial complex, you know.
Emily M. Bender: 100 percent.
Alex Hanna: Which is awful in its own right, but--
Emily M. Bender: All right, we got a little couple of things here that are sort of comic relief. So we're a bit over time, but I want to take us to the comic relief. The first one is, this is from Ars Technica on Mastodon, um, a ways back January 30th, 2024. Um, with a picture of a clock that says, "With steam that rises, a cup so fine, 9:41, it's coffee time." Almost rhymes. And the, the, the toot here says, "Rhyming AI powered-clock sometimes lies about the time, makes up words." And then, uh, "Poem/1 Kickstarter seeks 130k for fun, ChatGPT fed clock, sorry, for fun, ChatGPT fed clock that may hallucinate the time."
So what I love about this is is how it makes very concrete to a person who sees or uses this clock just how unreliable that technology is. I think it's actually a good piece of performance art in that way, and also hilarious.
Alex Hanna: Is the actual image maybe um, AI generated, it looks really, oh no, I don't, I think there's, the text looks too legible for that.
Emily M. Bender: The text is too good, yeah.
Alex Hanna: Yeah.
Emily M. Bender: All right. And then one last sort of comic relief, although less so, um, again, Ars Technica from, this one is February 23rd, 2024 by Ashley Belanger, Belanger. Um, So, uh, "Stupid M&M Machines," and then, "Vending machine error reveals secret face image database of college students," subhead, "Facial recognition data is typically used to prompt more vending machine sales," and what's going on here, um, "University of Waterloo students discovered that there was an M&M-branded smart vending machine, um, that was collecting facial images without their consent, consent as they were making purchases."
So, surveillance by M& M.
Alex Hanna: I'm wondering if it's, I mean, Waterloo is kind of the, the tech university of, of, of Ontario. So it's, it's very funny that this is where it is, but also like, good God. And the image here, I go to the image because it's, it's got kind of like the, the sexy brown M&M in like a lens reflected in this.
Um, so I don't know where they got this image. I'm very, I'm very tickled by it.
Emily M. Bender: And I can't tell if that M&M is being surveilled or looking through a peephole. What do you think is, is going on there?
Lisa Messeri: I think that M&M is surveilling.
Alex Hanna: The M&M is surveilling. So it is the voyeur brown M&M. Got it.
Emily M. Bender: That probably explains the glasses too, right?
Alex Hanna: Yeah.
Lisa Messeri: Yes, exactly. I mean, we would never see the green M&M in this compromising position. Let's just be clear.
Alex Hanna: The green M&M, just way too, way too above board. And, uh, I'm just, I'm going to end this despite introducing M&M lore and say almond M&M, right? Anti fascist, you know, just, just want to say it.
Emily M. Bender: That's canon?
Alex Hanna: That's canon. Red M&M? Communist.
Lisa Messeri: Clearly.
Emily M. Bender: Ah, so much fun. Good to start with some, some humor, even if it is building off of surveillance. Um, all right, so we are past time. Thank you so much for joining us. That's it for this week. Dr. Molly Crockett is an associate professor of psychology at Princeton University, and Dr. Lisa Messeri is an associate professor in the Department of Anthropology at Yale University. Thank you so much, um, both, for joining us.
Molly Crockett: Thank you so much.
Lisa Messeri: Thank you guys.
Alex Hanna: Our theme song was by Toby Menon. Graphic design by Naomi Pleasure-Park. Production by Christie Taylor. And thanks as always to the Distributed AI Research Institute.
If you like this show, you can support us by rating and reviewing us on Apple Podcasts and Spotify. And by donating to DAIR at DAIR-Institute. org. That's D A I R hyphen institute dot O R G.
Emily M. Bender: Find us and all our past episodes on PeerTube and wherever you get your podcasts. You can watch and comment on the show while it's happening live on our Twitch stream.
That's twitch.tv/DAIR_institute. Again, that's D A I R underscore institute. I'm Emily M. Bender.
Alex Hanna: And I'm Alex Hanna. Stay out of AI Hell, y'all.