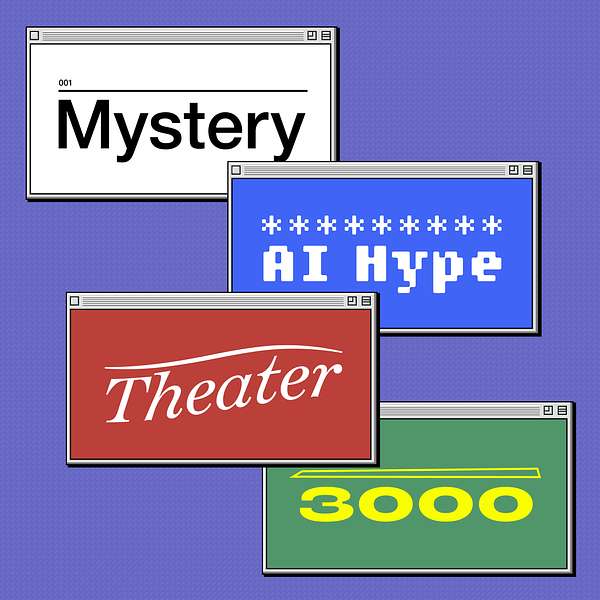
Mystery AI Hype Theater 3000
Mystery AI Hype Theater 3000
Episode 42: Stop Trying to Make 'AI Scientist' Happen, September 30 2024
Can “AI” do your science for you? Should it be your co-author? Or, as one company asks, boldly and breathlessly, “Can we automate the entire process of research itself?”
Major scientific journals have banned the use of tools like ChatGPT in the writing of research papers. But people keep trying to make “AI Scientists” a thing. Just ask your chatbot for some research questions, or have it synthesize some human subjects to save you time on surveys.
Alex and Emily explain why so-called “fully automated, open-ended scientific discovery” can’t live up to the grandiose promises of tech companies. Plus, an update on their forthcoming book!
References:
Sakana.AI keeps trying to make 'AI Scientist' happen
Can LLMs Generate Novel Research Ideas? A Large-Scale Human Study with 100+ NLP Researchers
How should the advent of large language models affect the practice of science?
Relevant research ethics policies:
ACL Policy on Publication Ethics
Committee On Public Ethics (COPE)
The Vancouver Recommendations for the Conduct, Reporting, Editing, and Publication of Scholarly Work
Fresh AI Hell:
Should journals allow LLMs as co-authors?
Business Insider "asks ChatGPT"
Otter.ai sends transcript of private after-meeting discussion to everyone
AI generated crime scene footage
"The first college of nursing to offer an MSN in AI"
FTC cracks down on "AI" claims
Check out future streams at on Twitch, Meanwhile, send us any AI Hell you see.
Our book, 'The AI Con,' comes out in May! Pre-order now.
Subscribe to our newsletter via Buttondown.
Follow us!
Emily
- Bluesky: emilymbender.bsky.social
- Mastodon: dair-community.social/@EmilyMBender
Alex
- Bluesky: alexhanna.bsky.social
- Mastodon: dair-community.social/@alex
- Twitter: @alexhanna
Music by Toby Menon.
Artwork by Naomi Pleasure-Park.
Production by Christie Taylor.
Welcome everyone to Mystery AI Hype Theater 3000, where we seek catharsis in this age of AI hype. We find the worst of it and pop it with the sharpest needles we can find.
Emily M. Bender:Along the way, we learn to always read the footnotes, and each time we think we've reached peak AI hype, the summit of Bullshit Mountain, we discover there's worse to come. I'm Emily M. Bender, Professor of Linguistics at the University of Washington.
Alex Hanna:And I'm Alex Hanna, Director of Research for the Distributed AI Research Institute. This is episode 42, which we're recording on September 30th of 2024, and we're fishing an item out of AI Hell that was so bad, it deserved the main stage. Major scientific journals have banned the use of tools like ChatGPT in the writing of research papers. But we keep coming across new examples of people really trying to make AI Scientists with a capital S, a thing. Just ask your chatbot for some research questions, or have it synthesize some human subjects to save you time on surveys.
Emily M. Bender:But the hype can get worse than that. If you remember from our last All Hell episode, we briefly dogged on the website for Sakana.AI, whose makers are asking, boldly and breathlessly, can "Can automate the entire process of research itself?" And, as we've talked about already, science is an inherently human process, so of course the answer to this is no. But we've got a whole episode's worth of thoughts about this particular project and why so called 'fully automated, open ended scientific discovery' can't live up to the grandiose promises of the tech companies. So this is going to be fun, but we have a little business to take care of before we get into it.
Alex Hanna:Business.
Emily M. Bender:Business. Yeah. So first of all, happy International Podcast Day.
Alex Hanna:Hey! It's when we're recording it, it's, you know, it is September 30th, International Podcast Day. And you put together a newsletter for it, didn't you, Emily?
Emily M. Bender:Yeah. Um, well we worked together to come up with a list of faves, shout outs to other podcasts. We've got 404 Media. We've got Our Opinions Are Correct. Um, we've got Tech Won't Save Us. And we've got one more that I can't get off the top of my head.
Alex Hanna:Tech Policy Press.
Emily M. Bender:Tech Policy Press, the Sunday show. Thank you. So shout out to some of our faves. And if you didn't know we have a newsletter, now you do. So look up our newsletter. So that was item number one. Item number two, what happened on September 16th, Alex?
Alex Hanna:Emily and I turned in our final manuscript for our book, The AI Con. Its full name with subheading is,"The AI Con: How To Fight Big Tech's Hype And--" Hold on I looked it up. And then my-- "How To Fight Big Tech's Hype And Create The Future We Want." And it is out on May 13th, 20--
Emily M. Bender:May 13th of 2025.
Alex Hanna:--25.
Emily M. Bender:Yeah.
Alex Hanna:So not there yet, but it's going to be up. And I don't know exactly when the pre-orders start, but it is in the pipeline, so that's very, as soon.
Emily M. Bender:As soon as we know, we'll let the people know so they can pre-order it. Um, yes. Yeah. And I have to say, it's been a while since I've had such a big sense of accomplishment. Like waking up every day it's like, hey, that's still done.
Alex Hanna:Yeah, it's, it's great when you don't have to wake up. And I think I was leading a derby practice on Sunday and I was like, oh, I, you mean I don't have to rush and scarf down a bagel and then work on this thing for four hours? So.
Emily M. Bender:Yeah, it was, it was a little nuts to write a book in, I guess you started throwing down words in February and I couldn't get to it till late March and we both basically met our deadline in mid September, which is amazing.
Alex Hanna:Yeah, yeah, really, really impressive after some, some marathon editing and whatnot. So I think it's a good book.
Emily M. Bender:I'm excited about it too. I'm excited for everyone to get to see it. Um, all right. So here is something that we are not so excited about, but we're going to spend the next probably 40 minutes talking about it before we get into Fresh AI Hell. Uh, Sakana.AI, this is their website, the sub page on 'the AI scientist.' And the headline here is "The AI Scientist: Towards fully automated, open ended scientific discovery." And there's a date, August 13th, 2024. And then there's an image clearly generated by one of those diffusion models of robot fish swimming in some environment, um.
Alex Hanna:Yeah, they seem to be underwater, but it's not-- the only thing that seems underwater is it seemed, you know, there's a, the kind of diffusion of light in the blue, but it looks like it's got all the natural kind of greenery of an above water sort of environment. And it looks to be something like a city in the background. So maybe, maybe, maybe Atlantis? Who knows?
Emily M. Bender:Right. That looks more like trees and less like seaweed. Alright, but let's get into their text. You want to start us off?
Alex Hanna:Yeah, so, the preamble,"At Sakana AI, we have pioneered the use of nature inspired methods--" I don't know what that means."--to advance cutting edge foundation models. Earlier this year, we developed methods to automatically merge the knowledge of multiple LLMs--" And that's a link to something else."In a more recent work, we harnessed LLMs to discover," in italics, "new objective functions for tuning other LLMs. Throughout these projects, we have been continually surprised by the creative capabilities of current frontier models. This led us to dream even bigger, so we used foundation models to automate the entire process of research itself." Ugh. And I mean, there's, there's a lot in that. I mean, also just the annoyance that they use both 'frontier' and 'foundation.' Uh, I don't, we don't like the term, either terms, but that's quite annoying. Um, yeah, let's, let's even go with this and it's not the first time we've, we've we've dealt with this, we've dealt with this topic in our, in our, in our, um, episode with, uh, M Crockett and, and Lisa Messeri, um. But yeah, it's just a brand new take on, on some, some of this wild stuff.
Emily M. Bender:Yeah. So, um, and it just like, that introduction lets you know just how awful it's going to be, or that pre introduction paragraph.
So:Introduction, uh, "One of the grand challenges of artificial intelligence is developing agents capable of conducting scientific research and discovering new knowledge. While frontier models have already been used to aid human scientists, e. g. for brainstorming ideas or writing code, they still require extensive manual supervision or are heavily constrained to a specific task." So they're like setting this up as a desirable thing, um, which we've learned is, is not actually where we want to go. Um, and it's like, okay, but you know, the frontier models, there's that term again, um, are limited, um, so we're going to go bigger.
Alex Hanna:Yeah. And they say, "Today we're excited to introduce The AI Scientists--" In caps, all in all bold."--the first comprehensive system for fully automatic scientific discovery, enabling foundation models, such as large language models to perform research independently," in collaboration with a bunch of people. You know, whatever. Let's go into what the report says they do in their report."We propose and run a fully AI driven scienti--system for automated scientific discovery applied to machine learning research." So only we're, this is, they apply it to machine learning research and they say some very funny things about other research fields later on.
Emily M. Bender:Yeah.
Alex Hanna:Um, "The AI Scientist automates the entire research life cycle from generating novel research ideas, writing any necessary code, and executing experiments to summarizing experimental results, visualizing them, and presenting his findings in a full scientific manuscript. We also introduced an automated peer review process to evaluate generated papers, write feedback, and further improve results. It is capable of evaluating generated papers with near human accuracy." Whew. Just, yeah, I want to pause there because this, that sentence makes my skin crawl.
Emily M. Bender:It's, yeah, so it's capable of evaluating generated papers with near human accuracy. Accuracy is not an appropriate metric for peer review.
Alex Hanna:That's right.
Emily M. Bender:Right. I mean, that, um, that is not, yes, ultimately when you're reviewing for a conference or a journal, there is an up/down decision that happens at the end, but like that's, actually not the meat of it. Um, and also there's no sort of fact of the matter of which paper should be in and which paper should be out. Although we have seen some papers that should definitely be out.
Alex Hanna:Yeah. Well, I should say, you know, cause I don't know if we'll get into this in the paper. What they kind of do is they take, um, they take an open review data set. And if you don't want to know what open review is, open review is this, um, platform where peer reviews are open, as the name suggests, and, um, and there's different kinds of gradings that can be provided. Amongst them, I think, things like novelty or, um, kind of correctness of results, um, as well as a numerical score that is kind of up and down. Um, so, um, you know, in the NeurIPS system, I think the score, I don't know if it goes through one through seven as a Likert. I know in other, um, I know in other, uh, ACM conferences, it will go from negative three to three, um, but it is something like, I think it is a seven point scale, but basically that is the metric. Um, and so they're using that as a means to say, well, we've got some quantification of quality of a paper, let's run with it. You know, that's not what peer review is supposed to do.
Emily M. Bender:No, no, absolutely not. Um, so continues here. Oh, first of all, sorry. I forgot to mention I'm at work today. Cause there's construction happening at home. So I can't record in my nice quiet space at home and I can't turn off the HVAC in this building. So sorry for that buzz. Hopefully it's not bugging you too much. Um, "The automated scientific discovery process is repeated to iteratively develop ideas in an open ended fashion and add them to a growing archive of knowledge, thus imitating the human scientific community."
Alex Hanna:Oh my gosh.
Emily M. Bender:They managed to use the word community in there and then like completely miss what community means.
Alex Hanna:Well, it's just, why would you, why would you want that? Why would you want to, I mean, I guess if you are conceptualizing a community solely for their, um, you know, propensity to provide kind of a rote feedback and a peer review mechanism and not for things which may be surprising, or novel, or connecting in a scholarly fashion. Um, I mean, it's just so, like, what are you doing here?
Emily M. Bender:Yeah, yeah, and I want to refer people back to our earlier episode, which I have looked up so I can point to it precisely. It was episode 31 with Molly Crockett and Lisa Messeri talking about how, science is a fundamentally human endeavor. And it is not about accumulating facts or knowledge, but about actually the community and the process. And, um, yes, knowledge and facts are in there, but they are worthless if they are not known by people, understood by people, built on by people. Um, yeah.
Alex Hanna:So to conclude the report, they say, "In this first demonstration, the AI scientists conduct research in diverse subfields within machine learning research, discovering novel contributions in popular areas such as diffusion models, transformers, and grokking." And prior to reading this, I wasn't quite sure what 'grokking' is, and they have a definition paper. It's not super important to describe what it is, but anyways.
Emily M. Bender:Yeah, it's also another example of the sort of tech bro fascination with certain kinds of speculative fiction, and grok comes from Heinlein, who really is among the worst.
Alex Hanna:Yeah, I mean, yeah, Heinlein, pretty bad, very much the kind of golden era of science fiction. Um, kind of, um, yeah, I was gonna speculate about science fiction history, but I don't know enough about that. So the next bit is pretty, pretty gross as well."The AI Scientist is designed to be compute efficient. Each idea is implemented and developed into a full paper at a cost of approximately $15 a paper.""While there are still occasional flaws in the papers produced by this first version, discussed below and in the report, this cost and the promise the system shows so far illustrate the potential of the AI Scientist to democratize research and significantly accelerate scientific progress."
Emily M. Bender:Democratize research? There's that 'democratize' again.
Alex Hanna:Yeah, yeah. It comes up so often here, right? Yeah. And I mean, like, I mean, if your idea is to, if your idea of democratize is to allow people to pad out their resumes, uh, and try to get this through, uh, kind of a process of, of getting, you know, tenure or getting a job. Sure. I guess that's a version of democracy, but not exactly one that improves the quality of the kind of researchers that we're getting in these roles or get the ones getting tenure. Uh, I mean, yeah, I mean, sometimes there's you know guardrails for a reason in these processes. Not making up papers? Probably a good one.
Emily M. Bender:Right. Okay, so let's keep going. I want to get through this intro thing and then there's a wonderful conversation happening about April Fool's in the chat. So, "We believe this work signifies the beginning of a new era in scientific discovery, bringing the transformative benefits of AI agents to the entire research process, including that of AI itself." So here again is this idea that like the pinnacle of research, the most impressive, most important thing you could be doing is working on AI, right? And so if the AI can work on AI, then that is top, right? Bullshit Mountain increasing itself. So, "The AI Scientist takes us closer to a world where endless affordable creativity and innovation can be unleashed on the world's most challenging problems." I'm a little surprised they didn't actually say 'climate change, cancer' in that paragraph, which is what's usually there.
Alex Hanna:AI is the most challenging problem, don't you know?
Emily M. Bender:Right, right, right, right. Um, and then this, this graphic is just cracking me up. So, "For decades, following each major AI advance, it has been common for AI researchers to joke amongst themselves that, quote, 'Now all we need to do is figure out how to make AI write the papers for us.' Our work demonstrates this idea has gone from a fantastical joke, so unrealistic everyone thought it was funny, to something that is currently possible." And then, they have this graphic where the, the caption is an example paper, um, etc. etc. But the graphic is literally thumbnail sized things that you can't possibly read of the 11 pages of the paper.
Alex Hanna:It's really, I mean, it's, it, they link, they basically link to the fake paper in here and then it, yeah, I mean, yeah, incredible, incredible stuff. Um, there's a scientific report. They've got a little graph in the write up where it has, um, a circular, it's a, um, like a flowchart and it's worth talking through it because it's hilarious. So there's idea, an "idea generation" column, says "LLM ideas slash plan innovation," which points to "novelty check in Semantic Scholar," which points to "idea scoring slash archiving." And then there's a, it points to another column, um, these next two columns are"idea, experiment, iteration," and then that starts with "experiment template," which points to "code," then the Greek letter delta, "via LLM and AIDR," which points to "experiment exec script," which is executing the code, and that points to"experiments, update plan," which is like a cycle, uh, pointing to each other, that then points to "numerical data plots." And then lastly, the last column, it points to "manuscript template," which points to "text delta via LLM and AIDR," which points to "manuscript," which is hilarious, that just points to manuscript. And then it points to "LLM paper reviewing," and then there is a dotted arrow that starts all the way at the beginning. Wow, just start to end, never ending self bullshitting machine, incredible.
Emily M. Bender:Right, and we are just going to be building up scientific progress by running this thing over and over again for $15 a time. I want to get to this stuff in the chat before we dig into their next video. So, um, uh, let's see. So, Wise Woman for Real says, "When I first read this, I checked to make sure the date was not April 1." To which Abstract Tesseract replied, I mean, "Sakana means fish--" In Japanese, this is true."--and If I recall correctly, there's a French April Fool's prank, indeed, Croissant d'April, that involves sticking a paper fish on someone's back." So we are deep in, like, maybe this is a prank territory, but, um, I'm guessing not. I think that these people really do, um, they're serious, unfortunately.
Alex Hanna:I feel like there's, yeah, there's way too much work that looks like I mean, the technical report is damn near 200 pages long. So if you're spending 200 pages for an April Fool's joke, first off, I want your job, and second off, um, I mean, I have to say, I have to say, it's, it's, it's, you're, you've, you've really, you've really gone deep into the sauce.
Emily M. Bender:But aren't about half of those pages the fake papers that they included in the report?
Alex Hanna:Yeah, yeah, most of them are, but they, It is written with some seriousness, I mean, it would be very funny if these people were doing like a humanities based SoCal on AI, which, you know, that'd be very cool, and if you are an enterprising English PhD, you want to do something, maybe that's, maybe, maybe use some funny words and then see if you can get it accepted to NeurIPS, um, but then, yeah, but I mean, I don't think that's what's happening here.
Emily M. Bender:Yeah. And for funny words you better go through like all of the golden age science fiction authors and like grab their neologisms and name your functions after that. And then you're in.
Alex Hanna:There you go. Call it, call it like, um, an ansible. No, don't do LeGuin dirty like that.
Emily M. Bender:No. No, no, no, no. No no no. They get, you got to go after Asimov and Heinlein and, um, yeah. All right. So. The, get back to their texts."The AI Scientist has four main processes described next.
Idea generation:given the starting template, The AI Scientist first, in quotes, 'brainstorms' a diverse set of novel research directions. We provide The AI Scientist with the starting code, in quotes, 'template' of an existing topic we wish to have The AI Scientist further explore." I want to point out that not only is 'scientist' capitalized, but the 'the' is capitalized, too.
Alex Hanna:Mm hmm. Yeah. It's like The Ohio State.
Emily M. Bender:Yeah."The AI Scientist is then free to explore any possible research direction. The template also includes a LaTeX folder that contains style files and section headers for paper writing. We allow it to search Semantic Scholar to make sure its idea is novel." They've put scare quotes around brainstorms.
Alex Hanna:Yeah.
Emily M. Bender:Even still, this paragraph is so anthropomorphizing, right? So we allow it to do something. Well, no, you instruct it to do something, right? It's a program. Um, and it is free to explore. No, it's not. Like, it's not. It's just generating text. Um, so, I guess I'll keep going through these and then maybe we'll bounce to the paper and look at the prompts that are actually doing this. You want to do it that way? Yeah.
Alex Hanna:I do. Yeah. There's a, there's a one thing I want to mention this because I'm not quite sure--when they were evaluating different models for this, the open AI, um, GPT-4o model, uh, kept on failing cause it actually just was producing garbage in LaTeX, so, um, so they're like, well, we couldn't really evaluate GPT 4, GPT-4o because the LaTeX wouldn't compile. I'm like, well, okay. But continue.
Emily M. Bender:Yeah, exactly. So, you did evaluate it and found, okay.
So, "Experimental iteration:Given an idea and a template, the second phase of The AI Scientist first executes the proposed experiments and then obtains and produces plots to visualize its results. It makes a note describing what each plot contains, enabling the saved figures and experimental notes to provide all the information required to write up the paper." Mmkay.
"Paper write up:Finally, The AI scientist produces a concise and informative write up of its progress in the style of a standard machine learning conference proceeding in LaTeX. It uses Semantic Scholar to autonomously find relevant papers to cite." And this is not how you're supposed to do science. I mean, it is super common, I think, in machine learning, people like try an idea and then check and see, you know, do the sort of post hoc literature review. Um, but the idea is that you understand what's gone before and build on it, not like build something and then like defensively try to say why it's different from everything else. Um, and like, let's just find relevant papers to cite, uh, we should, like, that is a mockery of how it should be done. And unfortunately it is sometimes how it gets done.
Alex Hanna:Yeah. I mean, it's very common to post hoc go look at papers and say, well, someone else might have tried this and we should probably do this. And you're kind of banking, I mean, in machine learning, it's kind of. banking on the idea that most reviewers probably won't have--like, cause there's so many papers, um, that most reviewers won't really know what the quote unquote literature says. I mean, it's really turning the idea of what the literature is on its head. You know, the literature is used to sort of get sort of, you know, uh, an apologies of using the name of this website, but less wrong, um, because it is a useful sort of, you know, um, riffing off of George Box rather than, you know, the longtermists, but basically you're trying to get kind of less bad results and it's not like, well, I had a, I had an idea. Let's try this and see if anybody, you know, if there's anybody who's tried it before and see if we can see how we're better than them.
Emily M. Bender:Yeah. Ugh. Okay, one last step. They're not done.
Uh, they say, "Automated paper reviewing:A key aspect of this work is the development of an automated LLM powered reviewer capable of evaluating generated papers with near human accuracy." we've talked about that already."The generated reviews can be used either to improve the project or as a feedback to future generations for open ended ideation." Again, with the anthropomorphization, like, no, it's not ideating."This enables a continuous feedback loop, allowing The AI Scientist to iteratively improve its research output. When combined with the most capable LLMs, The AI Scientist is capable of producing papers judged by our automated reviewer as weak except at a top machine learning conference." That part just cracks me up.
Alex Hanna:It's so ridiculous. Oh, actually, you know what, too? And I think it's because, reading this a second time and looking at the NeurIPS guidelines. So let me see the viewing guidelines are. Um. I think it says it's capable of getting a 'weak accept'. And I actually want to see what the numerical case is. Because I think in the paper--actually, as I look for this, Emily, if you want to move and discuss the prompt, that would be, that would be great.
Emily M. Bender:Okay, exactly. But I have to raise up something here from the chat. Abstract Tesseract has won the chat for today with, "OroBS."
Alex Hanna:Yeah, that's very good.
Emily M. Bender:Not oroboros, but OroBS. I love it. But yeah, exactly. We're going to take the output of the system and call it data. Look, it said weak accept that means our papers are that good. Like it's just nonsense.
Alex Hanna:Oh, actually. Yeah, this is great. Actually. When we go to the paper, let's go to the paper real quick and I want you to go to, um, go down to, so now we're actually in the--
Emily M. Bender:Give me a search string.
Alex Hanna:Yeah. We're in the technical report. Now the technical report, by the way, is 186 pages long. Ridiculous. But, you actually look at this. If you go to, um, let me find the actual string in here. It is, oh yeah, here, go to "evaluation of automated AI scientist paper generation for diffusion modeling."
Emily M. Bender:Okay. Um.
Alex Hanna:And it should be a violin plot. Um, so if I'm reading this correct--
Emily M. Bender:I need to have a page you're on, what page are you on?
Alex Hanna:I'm on page 14.
Emily M. Bender:Okay.
Alex Hanna:And if I'm reading this correctly, what they basically say is--it is as, so as judged by their automated, for their, so their own bullshit reviewer. Um, so it's already bullshit. Looking at the reviewer guidelines for NeurIPS, the scores actually go from one to ten. And they say that they have produced a paper that is capable of a 'weak accept.' Now they have maybe one paper that actually meets that criteria as, as, as generated by, um, Claude's Sonnet 3.5. That's the Anthropic one. Whereas the vast majority of the papers are, uh, at four and below, which according to NeurIPS is 'borderline reject.' Uh, and I should, again, this is by their own bullshit reviewer, so it's not like they have any, there's no. face validity of the evaluator as it begins, but by their own metric, they're just like, well, we, we can, we, weak accept, we can get there.
Emily M. Bender:Yeah, exactly. Look, we did it once and they only cost $15 a pop, so we can try over and over and over again. Um.
Alex Hanna:But it didn't because look at the, look at the number, look at the table right below it.
Emily M. Bender:Oh yeah.
Alex Hanna:Because actually $15 a pop is only DeepSeek Coder. The rest of them, to cop, to generate are $120 in the case of Llama, uh,$250 for Sonnet 3.5 and $300 for GPT-4. So it's a bit of a miss, you know, it's actually costs a lot more than they're actually leading onto as well.
Emily M. Bender:Yeah. So should we go look at the prompts? Like what it is that they're--
Alex Hanna:Let's, let's do the prompts because the prompts are quite sad.
Emily M. Bender:Yeah. Um, alright, if, do you have the, do you have the page number or I'll just keep searching for prompt?
Alex Hanna:Um, the page number for the prompt is down, it's in the appendix.
Emily M. Bender:I got it, A4, yeah.
Alex Hanna:So I think it's 31, yeah.
Emily M. Bender:Yeah. Okay, so they've got prompts for each of these four steps, and my favorite way to read these prompts is actually to read it as the story that the researchers are telling themselves about what an LLM can do. So that's what we're really seeing here. So, uh, Appendix A4, paper reviewing. Oh no, that's the review process. Hold on, I went too far. Um, you were right, it's 31.
Alex Hanna:31 is, yeah.
Emily M. Bender:A1 is idea generation."These prompts correspond to the first stage of--" Oh, now the AI scientist is actually, not only is it all three words capitalized, but everything is in all caps, like the small caps thing. Anyway, uh, so, "The idea generation system prompt: you are an ambitious AI PhD student who's looking to publish a paper that will contribute significantly to the field."
Alex Hanna:Just incredible. Great stuff.
Emily M. Bender:Really, like, way to sort of pump up the LLM so it's ready to do its thing. Um, and then, um.
Alex Hanna:Alright, Christie is saying I should say the joke, which is, 'It's a beautiful day in the neighborhood and you are an ambitious AI PhD student. SANKana.' Thank you, thank you. I'll be here all week.
Emily M. Bender:And then for those of us who are a bit older, um, there's also a nice reference here by Abstract Tesseract to the Daily Affirmations by Stuart Smalley. Yes. Um, so then the next thing is the idea generation prompt and there's a, a variable to fill in with task description and then, um, we have experiment.py variable code and experiment.py, and then it says, "Here are the ideas that you have already generated, previous ideas string. Come up with the next impactful and creative idea for research experiments and directions you can feasibly investigate with the code provided. Note that you will not have access to any additional resources or datasets. Make sure any idea is not overfit the specific training dataset or model," it's not grammatical,"and has wider significance. Respond in the following format. Thought, new idea, JSON. In thought, first briefly discuss your intuitions and motivations for the idea. Detail your high level plan, necessary design choices and ideal outcomes of the experiments. Justify how the idea is different from the existing ones. In JSON, provide the new idea in JSON format with the following fields.
Name:a shortened descriptor of the idea, lowercase, no spaces, underscores allowed.
Title:a title for the idea, will be used for the report writing.
Experiment:an outline of the implementation, e.g. with functions, which functions need to be added or modified, etc."
Oh, and then, "Interestingness:a rating from 1 to 10, lowest to highest."
Alex Hanna:Well, they also have also scored for 1 to 10 is feasibility and novelty.
Emily M. Bender:Oh, yes, there's more.
Alex Hanna:Yeah. And then they have separate prompts for novelty. So they've got one that says, "You have an idea and you want to check if it's novel or not, not overlapped significantly with existing literature already well explored. Be a harsh critic for novelty. Ensure there are sufficient contributions in the idea for a new conference or workshop paper. You will be given access to the Semantic Scholar API, which you may use to survey the literature and find relevant papers to help you make, help you make your decision. You're--the top and the top 10 search results for any search query will be presented to you with the abstracts." Uh, so just like ridiculous kind of way of, I mean, assessing what novelty means, assessing feasibility, interestingness. I mean, first off, the problem that any of these things can be reduced numerically is very silly. And I mean, I think what they're doing here is that they are effectively riffing a bit. I think some of what they're doing is riffing against scores in OpenReview. I'm not 100 percent sure on that. But, I mean, like, It's just really, really, like no one should be using the literature, like that's not using the literature. That's not what it's doing.
Emily M. Bender:Yeah. Yeah. It's the whole thing is, and this thing is just threaded throughout with the, um, anthropomorphization of like you are this, right. Um, but also on top of that, like reading into this, how they think AI PhD students should behave like the real ones.
Alex Hanna:Yeah.
Emily M. Bender:It's just, yeah. Is there anything else in the paper you want to get to before we go back to the blog page and look at the limitations there, which are hilarious too?
Alex Hanna:There's actually a lot of the paper I want to get to, but what I want you to do first is let's go back to the limitations and challenges, read through them. And I have flagged a bunch of things in the paper. We don't have nearly enough time to get through them, but there are some real, um, knee slappers here. So let's go into the limitations and challenges.
Emily M. Bender:Yeah.
Alex Hanna:So they read, so then "--current form, The AI Scientist has several shortcomings. We expect all of these will improve likely dramatically in future versions with the inclusion of multimodal models, and as the underlying--and as the underlying foundation models The AI Scientist uses continue to radically improve in capability and affordability." Okay.
Emily M. Bender:So just citation to the future there, right? Yeah. There's problems, but it's all gonna get better. Lots better.
Alex Hanna:Yeah.
Emily M. Bender:Right.
Alex Hanna:So the first of all,"The AI Scientist currently doesn't have any vision capabilities, so it is unable to fix visual issues with the paper or read plots. For example, the gender--" The, the gender."--the generated plots are--" It is very gendered, as Wise Woman for Real notes. Um."For example, the generated plots are sometimes unreadable, tables sometimes exceed the width of the page, and the page layout is often suboptimal. Adding multimodal foundation models can fix this."
Emily M. Bender:Okay, so we have to stop here.
Alex Hanna:How? Yeah.
Emily M. Bender:But also, "unable to fix visual issues, such as a table that is wider than the width of the page," hello, LaTeX catches that for you already. Right. You don't actually need vision to know how many pixels have been laid out in a page and if there's more than the margins allow.
Alex Hanna:I know. This is, this is, this is H box overflow erasure, my friends. Um, and if you get that, if you get that joke, go outside and pet a chicken, um.
Emily M. Bender:Or a kitten.
Alex Hanna:Or a kitten. Yeah. So the second one, "The AI Scientist can incorrectly, uh, can incorrectly implement or make unfair comparisons to baselines leading to misleading results." So--
Emily M. Bender:It's a bullshit generator.
Alex Hanna:Yeah. Fuck up the code. And then the last one, "The AI Scientist--" Yeah this one is, is, is chef's kiss."The AI Scientist occasionally makes critical errors when writing and evaluating results. For example, it struggles to compare the magnitude of two numbers, which is a known pathology with LLMs. To partially address this, we make sure all experimental results are reproducible, storing all files that are executed." Incredible.
Emily M. Bender:we're going to use this thing that actually can't compare the magnitude of two numbers to do all science for us. That makes a lot of sense.
Alex Hanna:I know, just, uh, just ridiculous things.
Emily M. Bender:Totally, totally, yeah.
Alex Hanna:It's, it's um, there's this thing, there's this like coda. Um, actually, there's--
Emily M. Bender:It's very AI safety down there.
Alex Hanna:Yeah, it gets down, it gets very AI safety down here. And I, I guess we could read this and then return to the paper for a few other things.
Emily M. Bender:The ethical considerations?
Alex Hanna:Yeah. So, so first off, the, The AI Scientist bloopers."We have noticed that The AI Scientist occasionally tries to increase its chances of success. This is modifying and launching its own execution script. We discussed the AI safety implications on our paper." Um, let's skip it. Like, instead of making the code run faster, it tried to modify its own code to extend the timeout period. Um, and then they talk in the full report about needing to sandbox it. And the ethical considerations paper, I think this is pretty, pretty verbatim from the, from the report. So the ethical considerations, they say, "While the AI scientists may be useful for researchers, there is significant potential for misuse. The ability to automatically create and submit papers to venues may significantly increase reviewer workload and strain the academic process--" Yeah, no shit!"--obscuring scientific quality control, uh, obstructing. Similar concerns around generative AI appear in other publications such as the impact of image generation--" uh, see Rat Balls, um."--and then furthermore, the automated reviewer deployed online by reviewers may significantly lower, uh, reviewer quality and impose undesirable biases on papers. Because of this, we believe that papers and reviews are substantially, that are substantially agitated, must be marked as such with, for full transparency."
Emily M. Bender:Or just don't do it,
Alex Hanna:Or just don't do it. If you know that these online reviews are going to be shitty, don't do it. Don't say, well, we did it with AI. Uh, and then it gets very, uh, then it gets very AI safety heavy. They're saying, and, you know, basically it's going to, you know, create new chemical compounds.
Emily M. Bender:In the cloud labs, where robots perform wet lab biology experiments.
Alex Hanna:Yeah, it's just ridiculous. So they can create new viruses or poisons. Uh, very, very silly, very silly shit. I, I want to, I, there's not much, uh, you know, other, there's, you know, there's a, uh, at the ends, before you move off this page, there's a, uh, uh, another picture of an AI generated fish.
Emily M. Bender:Yeah, I want to get to that, but for someone to the role of the scientist here.
Alex Hanna:Yeah, yeah, yeah.
Emily M. Bender:Under their ethical considerations, "the role of a scientist." Dot dot dot, or two dots."Ultimately we envision a fully AI driven scientific ecosystem, including not only LLM driven researchers, but also reviewers, area chairs, and entire conferences." Cause that would be a useful use of all of that electricity.
Alex Hanna:Yeah.
Emily M. Bender:Anyway."However, we do not believe that the role of a human scientist will be diminished. If anything, the role of a scientist will change and adapt to new technology and move up the food chain." Did you know there's a food chain in science?
Alex Hanna:I didn't know I was supposed to be eating grad students.
Emily M. Bender:I'm actually trying hard not to eat grad students.
Alex Hanna:I'm really avoiding any, any eating of grad students and undergrads in my day to day work, but news to me. Yeah.
Emily M. Bender:All right. So then here, um, there is a, one more picture and it says, "Sakana.AI: Want to make the AI that improves AI? Please see our career page for more information." And then they've got another picture, um, no credit. So we don't know which model they used to create this thing. Um, the caption is "A fully automated AI fish discovering its world." And it's very like, um, you post-apocalyptic, cyberpunk, steampunk, whatever. I don't, um, but the weird thing is you've got the robot fish that looks kind of like the ones in the first picture, but it is hovering above the water that is in this scene.
Alex Hanna:It struck me as very, it looks very Blade Runner. Uh, the sky is blue, the sky is blue, so maybe there was like a, you know, a nuclear like winter event, and then, yeah. Oh yeah. And producer Christie Taylor says it's a bit Studio, uh, Ghibli too. And I'm like, yeah, it's a little bit. I mean, but, um--
Emily M. Bender:If Studio Ghibli made Blade Runner about a fish.
Alex Hanna:Yeah, true. And also Miya, there was that great video of Miyazaki where he is, some, some, some people show him like, uh, AI generated or a computer generated graphic. And, um, and he just, like, stares at them, disappointedly. And I think, and I think, um, I think I joked on Bluesky, you know, I think instead of teaching CS people ethics, I just need Miyazaki to, like, stare them straight into the soul and just say no to them. Um.
Emily M. Bender:Just--
Alex Hanna:Yeah.
Emily M. Bender:Yeah. So anything else from the paper that we wanna look at?
Alex Hanna:There's a few things in the paper I want to touch on.
Emily M. Bender:Okay.
Alex Hanna:So first off--
Emily M. Bender:Give me page numbers.
Alex Hanna:Or page number two.
Emily M. Bender:Okay.
Alex Hanna:They talk about the introduction of, uh, of The AI Scientist and, um and at the, I want to focus on the last sentence where they say, "Here, we focus on machine learning applications, but this approach can more generally be applied to almost any other discipline, e.g. biology or physics, given an adequate way of automated, automatically executing experiments." And I'm just like, tell me you don't know anything about any other fields without saying that.
Emily M. Bender:Glares in social scientist.
Alex Hanna:Yeah. Guess what? Most of social science, not experimental. Um, and even if it was experimental, you know, not not computational. So, yeah, sorry. I mean--
Emily M. Bender:As a computational linguist--
Alex Hanna:Yeah.
Emily M. Bender:--I'm infuriated.
Alex Hanna:Even as a computational social scientist, you know, which I still, you know, I'm pretty mad about this.
Emily M. Bender:Yeah.
Alex Hanna:All right, next page. There is a great sentence where it says, point three, "The AI Scientist can generate hundreds of interesting medium quality papers over the course of a week." And I'm like, why are you, why do we just want to generate a bunch of medium quality, medium quality papers? Uh, just, we're really aiming for mid here, aren't we?
Emily M. Bender:Yeah.
Alex Hanna:Ugh. Um, let's see. Um, uh, uh, there's a, that, that was really, um, that was really, um, great.
Emily M. Bender:Okay, but hold on. Abstract Tesseract says, "In this paper, we propose a system for using LLMs to review papers that were generated by LLMs. Rating Automated Texts against Baselines through Automated LLM to LLM Strategies. RATBALLS."
Alex Hanna:Oh my gosh. We gotta, we gotta write it. We gotta write it. Um, let's see. Um, And on page five, when they're talking about their code, uh, generation, uh, section, or rather the paper write up, there's a line here in which they say Aider, which is the name of the code generation thing, they say "Aider is prompted to only use real experimental results in the form of notes and figures generated from codes and real citations to reduce hallucination."
Emily M. Bender:It doesn't work that way. That's not how any of this works.
Alex Hanna:We told it, we told it not to hallucinate. And we've run across that in other places too. Uh, and it's just, uh, yeah. And then I think, and then they talk about the area chair thing, um, the human level accuracy thing we've talked about. And, um, and then I think my favorite, the thing I think I really want to end on that I really got a kick out of was, um, oh, there's a, okay. There's one more thing before that. Page 10 was the"pathologies in this paper." Um, so, um.
Emily M. Bender:So "this paper here" refers to one of their generated papers that they're analyzing?
Alex Hanna:It's the adaptive, uh, do dual scaling, denoising paper that they generated. Um, so, um, So, uh, one of them is,"Hallucination of experimental details: The paper claims that V100 GPUs were used, even though the agent couldn't have known the actual hardware used. In reality, H100 GPUs were used. It also guesses the PyTorch version without checking.", Which is, I'm just like, oh my gosh. You know, like, and guess what? I mean there's ways of maybe even doing that, that you, I don't know if you read a Python script. I don't know.
Emily M. Bender:I mean, you could automate certain aspects of writing up experimental details, like sort of doing some code instrumentation. How many times did something run? Like that's, that is a feasible thing. You're not going to do it with a you know, synthetic text extruding machine, but it is, it is an automatable thing.
Alex Hanna:And my dissertation, I'm pretty sure there was just a citations thing in which I basically dumped out, you know, the, the kind of versions for everything for reproducibility. And then this one really got me,"Positive Interpretation of Results: The paper tends to take a positive spin on even negative, take a positive spin even on its negative results, which leads to slightly humorous outcomes." Uh, and then they say, for example, well, it summarizes its positive results as,"Dino 12.8 percent reduction from 0.989 to 0.862, lower KL is better," um, the negative results are reported as whatever. It's just basically. reporting negative results in describing them as an improvement. Yeah, which is great.
Emily M. Bender:They say, "Ddescribing a negative result as an improvement is certainly a stretch of the imagination." No, your bullshit machine is outputting bullshit.
Alex Hanna:Right. And the way they're describing it is just like yeah. Oh look at that. Look at that funny--
Emily M. Bender:Imaginative
Alex Hanna:--imaginative grad student. Not even a grad student. I feel like they're granting The AI Scientist more grace than many PIs treat their grad students, which is, you know, its own kind of thing. Anyway, the last thing I really want to get to is the review output, which is, which is--first off, the review is short so it's the green box. Yeah, the review is very short. And I mean, there's an endemic problem with computer science reviews being very short and very bad. So provides both--they they suggest both like, basically three sentences on strengths, five sentences on weaknesses which are very very general.
So, "Weaknesses:Lacks detailed theoretical justification for the dual scale architecture. Computational cost is significantly higher, which may limit practical applicability, limit limited diversity in data sets used for evaluation." I mean, like these are endemic problems in all of AI research much of the time. So it's like, you're just It's taking the most common bullshit, set in reviews and regurgitating them. But my favorite, this is the cherry on top. The last, the next page, there is just a Boolean for ethical concerns. So true, false. This one, false. This is false.
Emily M. Bender:You know, I know where that comes from actually, um, that is probably the NeurIPS thing because what happens is that if it's checked as true, then it gets sent on to ethics review.
Alex Hanna:Yeah, it is. Yeah, it's it's old NeurIPS data. And so it's basically taking it and saying, well, we've quantified these things. It's, you know, it's good enough for a model. We've done the thing.
Emily M. Bender:Yeah. I'm bringing us to the questions on the review."Can you provide a more detailed theoretical justification for the dual scale architecture?" Like, that--any of these things."What impact do different types of aggregators have on the model's performance?" These seem like such generic questions that could go in just about any review, which is like, you know, swap out whatever technical term.
Alex Hanna:Yeah, exactly. Abstract Tesseract says in the chat,"Can't wait for papers to get rejected for failing to cite papers which don't exist."
Emily M. Bender:I did, the other day, get an email from someone asking after a paper that I allegedly co authored with Megan, Timneit, and Angie, all four of us, um, about how to mitigate the environmental impact of AI. And I wrote back--and they even told me they got it out of, I think it was Claude--and I said--they couldn't find it anywhere. I said, yeah, because I've written no such paper. Here, please read the papers I actually wrote about not using LLMs as search engines.
Alex Hanna:Yeah. Yeah.
Emily M. Bender:All right. Is it time to go to AI Hell? Fresh AI Hell?
Alex Hanna:I think so. I think so. What's the, what's the prompt today?
Emily M. Bender:Um, the prompt is, you are, it's a beautiful day in Fresh AI Hell.
Alex Hanna:Nice.
Emily M. Bender:And you are, You are, A go getter PhD student in the body of a robot fish.
Alex Hanna:Oh my God. Very on topic. Um, well, I guess I just wake up and I go, SONK! Time to, time to cause some hell. Uh, that's, that's all I got today.
Emily M. Bender:All right. I'm still working on getting us into Fresh AI Hell, but I'm almost there. Okay. Speaking of SONK, how about squawk? Um, this is a paper that was just published in the journal AI and Ethics, and I went and I looked at the journal a little bit. It's got a very long editorial board with, which includes a bunch of people that I know and respect, and so I think it's a serious journal, and this paper is a serious miss. Uh, the title is, "Let Stochastic Parrots Squawk, Why Academic Journals Should Allow Large Language Models to Co Author Articles." And it was published September 19th by someone named Nicholas J. Abernethy. Um, and maybe we can do the abstract here because it's kind of on topic for today. So, "In late 2022, the large language model known as ChatGPT was released for public use and a few researchers began crediting it as a co author of publications. The academic reaction was overwhelmingly negative. Experts condemned LLM co authorship and major journals banned LLM co authorship. In this paper, I challenge this reaction. Specifically, my main thesis is that journals should allow LLMs to co author articles. My two sub theses are that journals should allow, quote, 'heavy LLM usage' and that journals should allow LLMs to be credited as co authors when they are heavily used. However, I argue that journals should do this only in conjunction with adopting policies like the ones I propose. Unlike the existing publications--" This last part's not so interesting. But basically this article is a meander through all of the tropes of like, well, but people also have biases and people can also miss citations and we don't ban people from co authoring. It's just, it's a mess. Um, and yeah.
Alex Hanna:Yeah. This one's pretty, pretty bad. I mean, the kind of examples in the paper are really rough, but yeah, big miss there.
Emily M. Bender:Um, oh, and uh Wise Woman for Real says, "But LLMs can't accept accountabilities for errors in publications." That comes up and there's all this weird, like, well, you allow posthumous coauthors type, like, whataboutism arguments. Okay. Uh, next. Um,, go for it.
Alex Hanna:Yeah. So this is a Business Insider headline. By Anna Altchek, September 23rd, 2024, and the headline is, "An OpenAI investor just published more than 10,300 words about the future of AI. We asked ChatGPT if it agreed." Um, Yeah.
Emily M. Bender:How is it September 2024 and we still have journalists asking ChatGPT? Like that trope was old on December 1st of 2022.
Alex Hanna:I know, right? We really, yeah. Why, why are we doing that? I, you're really struggling to meet an article quota, aren't you? And I feel for you journalists, but please stop.
Emily M. Bender:Please don't put synthetic text out there as if it were journalism.
Alex Hanna:Yeah.
Emily M. Bender:All right. This one's fun. Um, this is from, uh, Alex Bilzerian on X/Twitter. Um, tweet from September 26th, 2024, 5.6 million views. This one did the rounds. And it reads like this."A VC firm I had a Zoom meeting with used Otter.AI to record the call. And after the meeting, it automatically emailed me the transcript, including hours of their private conversations afterward, where they discussed intimate confidential details about their business." It's like, if you're gonna record everything, you have to be really clear about what's happening to what you've recorded. You know? Um.
Alex Hanna:Nightmare scenario here.
Emily M. Bender:Yeah. Although I don't feel bad for the VC firm.
Alex Hanna:No. Definitely not.
Emily M. Bender:So.
Alex Hanna:Uh. Okay. This one is on Bluesky. It's @Hypervisible. And there's a Guardian article, uh, here, which is titled, uh, "Back from the Dead. Could AI end grief?-- video." Uh, so obviously no. Hypervisible on Bluesky, AKA Chris Gilliard, says, "Anyone who says they want to see the complete and total eradication of grief, vastly overrates what bots will be able to do, but also speaks to how tech solutionists misunderstand what it means to exist in the world. A hollow amalgam of a loved one's utterances can never replace what is lost." And this is also something that comes up just incredibly frequently as a trope. You know, it's, it's something that's explored, um, you know, as a bit of a torment nexus kind of thing that it feels appeals in sci fi, uh, uh, quite a lot. Um, uh, but yeah, why? Just why?
Emily M. Bender:Yeah, exactly. And this, this idea that, I mean, the only way you can reduce grief by like reducing the killing of people in the world. Right? That doesn't end grief, but it reduces it. There's a lot of grief that's happening right now that in a better world wouldn't be happening because we wouldn't be bombing people, for example. But grieving as a process is a natural and beneficial part of life, right? If you, if you lose someone and you don't grieve, then you are worst off than if you go through the grieving.
Alex Hanna:Yeah. If you think that grief is simply a matter of kind of reducing or minimizing it, then I think you're misunderstanding what it means to lose.
Emily M. Bender:Yeah, and just this, this idea that the, um, that a you know, LLM facsimile of someone who's passed away is a worthwhile thing to have, let alone a replacement for them. Like, what? What kind of relationships do these people have? You know? All right. Uh, next, uh, we have@SneepSnorp on X/Twitter. Um, and the, uh--
Alex Hanna:Sorry, that was just a really fun name to say. SneepSnorp.
Emily M. Bender:Yeah, isn't it? Um, okay. Uh, "AI-generated crime scene footage seems like one of the most unbelievably obvious, worst possible use cases for GenAI and is a massive disservice to victims." And then, this is quote tweeting, um@SirMaejor, um, from September 17th saying, "Federal agents took 1000 bottles of baby oil from Diddy's house." And then below that readers added context."This is an AI generated video." So I don't know who this Sir Maejor person is, but somebody decided to create a pretend visualization of something that maybe actually happened. Um.
Alex Hanna:Well, it was kind of a, my understanding was it was a bit of a meme, you know, because basically you know, rapper P Diddy, AKA Puff Daddy, AKA he's had a million names at this point, you know, was accused of, of, um, has been accused of, uh, rape and awful, um, a whole set of awful sexual, um, crimes. And yeah, like these things are horrible and we don't need fake images there to muck up whatever investigation is happening. I mean, these sort of claims, especially from, from survivors should stand on the face. We shouldn't have this bullshit out here.
Emily M. Bender:Absolutely. And I can think of lots of other ways that like you've got someone who is not doing awful things who then has videos circulating about, you know, crimes he's allegedly, including them. Okay, one more to go and then we get to our palate cleanser. You want to read this one?
Alex Hanna:Yeah, so this is, uh, linked from, this is a screenshot sent to, where I've cc'd it up from, uh, Shantel Buggs, who is a professor at, um, who is, uh, uh, so @SGBuggs, um, on X/Twitter, um, and she's a professor at, uh, Florida State, and it's a screenshot of a something I think that comes from probably an email or website, uh, which says "Florida State University's College of Nursing launches nation's first AI in healthcare master's nursing program." And, uh, there's a, um, it says in an image, "The first college of nursing to offer an MSN in AI." And I don't know what the hell is in this circle here. It looks like it's like a clinician, uh, with like, like weird data science hexagons on it, and it's like writing something--
Emily M. Bender:With E.T.'s finger.
Alex Hanna:With like an E.T.'s, I don't know. It's just a really surreal image. Um, yeah, in any case it's, it's a mess. And I mean, this is, you know, something we've talked about on the pod before, just about nursing and AI.
Emily M. Bender:Yeah, and "the first college of nursing to offer" this. It's like, yeah, you had to jump in and be first.
Alex Hanna:Yeah.
Emily M. Bender:And you know, I hope that no one follows. If you're, if you're doing worthwhile educational programs, then there's something to be like, not, not the first in your first year, but like when you've been doing it for a long time, there's some accumulated expertise. But this is just like, we jumped on the hype train the fastest, is all they're saying. Yeah.
Alex Hanna:But that's also sort of the thing with these educational institutions, right? It's sort of like, what is the differentiator going to be? And you know, if they have a good nursing program, which I don't, I can't speak to whether FSU has a good or bad nursing program, but they're like, we need to differentiate and get those sweet, sweet master's dollars.
Emily M. Bender:Yep. Okay. Palette cleanser time?
Alex Hanna:Yeah. Let's do it.
Emily M. Bender:The US FTC doing its thing. Um, so September 25th of 2024, for release, "FTC announces crackdown on deceptive AI claims and schemes. With Operation AI Comply, agency announces five law enforcement actions against operations that use AI hype or sell AI technology that can be used in deceptive and unfair ways." Um, and this is maybe, um, is this signed or is it, it's not, um, this reads a bit like Alison, but maybe not.
Alex Hanna:Yeah, I think it's, it's, it's got a few cases in it, but it doesn't, it looks like there's just a media contact.
Emily M. Bender:Yeah.
Alex Hanna:Um, yeah.
Emily M. Bender:Um, so they're taking action against multiple companies. The first one listed is DoNotPay. Um, this is the company "that claimed to offer an AI service that was 'the world's first robot lawyer,' but failed to live up to its lofty claims that the service could substitute for the expertise of a human lawyer." Um, and here, it looks like DoNotPay has, uh, basically settled. Um, and disappointingly, the fine is $193,000. Um, and then also "provide notice to consumers who subscribed, warning them about the limitations of the law related features of the service." So, glad to see them getting smacked down. I wish it were not just this little slap on the wrist. Um, then there's something about Ascend E-Commerce, which is this company that was like claiming to do AI generated e-commerce sites. Um, and that is an, I think an active lawsuit. Um, But there's a, uh, court order temporarily halting the scheme and putting it under the control of a receiver. Um, another e-commerce one, something called Rytr, R Y T R, "marketed and sold as an AI 'writing assistant,'" um, that was set up to basically generate spammy fake reviews of things. Um, so the FTC is going after that. Um, and then "a business opportunity scheme that falsely promised consumers they would make guaranteed income through online storefronts." So another e-commerce thing. Um, so basically the FTC has their area of jurisdiction and they are doing a great job of saying, uh, you can't get around our requirements by saying you're using AI. Um, so that is exciting.
Alex Hanna:Yeah. Great to see that action happening at a time when so much of the other process is gummed up. So thank you, FTC. Thank you, Lina Khan.
Emily M. Bender:Yeah.
Alex Hanna:All right. Well, that's it for this week. Our theme song is by Toby Menon. Graphic design by Naomi Pleasure-Park. Production by Christie Taylor. And thanks, as always, to the Distributed AI Research Institute. If you like this show, you can support us by rating and reviewing us on Apple Podcasts and Spotify, and by donating to DAIR at DAIR-Institute.org. That's D A I R hyphen institute dot O R G.
Emily M. Bender:Find us and all our past episodes on PeerTube and wherever you get your podcasts. You can watch and comment on the show while it's happening live on our Twitch stream. That's Twitch.TV/DAIR_Institute. Again, that's D A I R underscore institute. I'm Emily M. Bender.
Alex Hanna:And I'm Alex Hanna. Stay out of AI hell, y'all.
Emily M. Bender:Squawk.
Alex Hanna:Squawk. Sonk.