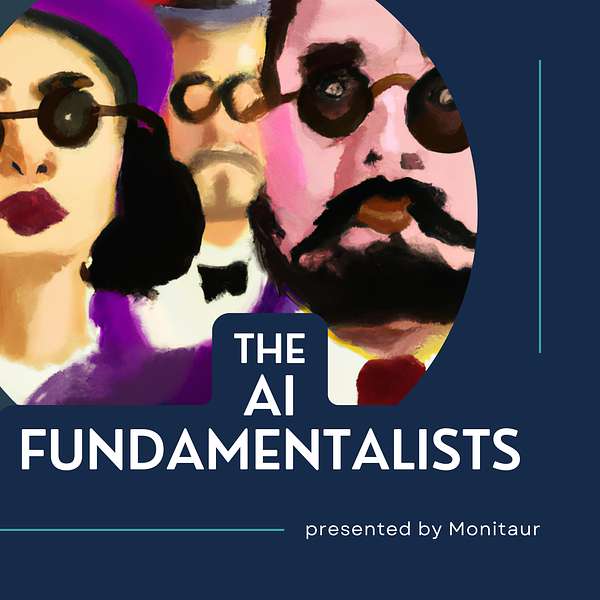
The AI Fundamentalists
A podcast about the fundamentals of safe and resilient modeling systems behind the AI that impacts our lives and our businesses.
The AI Fundamentalists
Data lineage and AI: Ensuring quality and compliance with Matt Barlin
Ready to uncover the secrets of modern systems engineering and the future of AI? Join us for an enlightening conversation with Matt Barlin, the Chief Science Officer of Valence. Matt's extensive background in systems engineering and data lineage sets the stage for a fascinating discussion. He sheds light on the historical evolution of the field, the critical role of documentation, and the early detection of defects in complex systems. This episode promises to expand your understanding of model-based systems and data issues, offering valuable insights that only an expert of Matt's caliber can provide.
In the heart of our episode, we dive into the fundamentals and transformative benefits of data lineage in AI. Matt draws intriguing parallels between data lineage and the engineering life cycle, stressing the importance of tracking data origins, access rights, and verification processes. Discover how decentralized identifiers are paving the way for individuals to control and monetize their own data. With the phasing out of third-party cookies and the challenges of human-generated training data shortages, we explore how systems like retrieval-augmented generation (RAG) and compliance regulations like the EU AI Act are shaping the landscape of AI data quality and compliance.
Don’t miss this thought-provoking episode that promises to keep you at the forefront of responsible AI.
What did you think? Let us know.
Do you have a question or a discussion topic for the AI Fundamentalists? Connect with them to comment on your favorite topics:
- LinkedIn - Episode summaries, shares of cited articles, and more.
- YouTube - Was it something that we said? Good. Share your favorite quotes.
- Visit our page - see past episodes and submit your feedback! It continues to inspire future episodes.
The AI Fundamentalists a podcast about the fundamentals of safe and resilient modeling systems behind the AI that impacts our lives and our businesses. Here are your hosts, andrew Clark and Sid Mungalik. Hello everyone, welcome to today's episode of the AI Fundamentalists. Today we have a guest with us, matt Barlin. He is the Chief Science Officer of Valence and we're honored that he's agreed to join us to talk about data lineage. But before we begin, matt's current work on designing Web3 also intrigued us because he's applying a systems engineering perspective and, as you'll remember, we've had a previous episode about the fundamentals of systems engineering on this podcast and we've invited him to share a few thoughts about that before we dive into data lineage. So, matt, welcome.
Speaker 2:Hi. Yeah, thank you, susan. Thanks for having me. I'm very happy to be here. Yeah, so I thought the systems engineering episode was very well done, and there were just kind of two points that I wanted to add about, one in terms of where it came from and another in terms of where it's going going back to the history is as. Andrew was discussing how it kind of came out of NASA and you know large scale, usually Department of Defense or government funded large scale engineering projects.
Speaker 2:One of the things that the role as a system engineer and what system engineering was really about was being able to manage the documentation about all these different engineering efforts. And so that included, as Andrew mentioned like one of the best things that came out of systems engineering was requirements analysis, but it also had to do with managing
Speaker 2:change logs and updates where you know, computers may have been done for a certain specific task, but there was no central place to manage all the documentation that involved in, you know, engineering some complex systems, such as, you know, a naval vessel or a space shuttle type mission. So that was one thing, and then the other thing to mention was that, in terms of where this has gone, is that there is a specific branch that really Sid and Andrew were talking alluding to about. You know, that starts to define the tools that can be used, how you say, write different types of models and have their place.
Speaker 2:So you start with, say, a system model and then you can apply the certain, the different, branches say, like you know, for the physical system, a mechanical model and electrical model, or in you know a lot of the things that we're working on, where they're kind of cyber physical system, a mechanical model, an electrical model, or in you know a lot of the things that we're working on where they're kind of cyber physical systems then you might inject a behavioral model into these systems models, and so what that allows is you know all the things that Andrew and Sid mentioned, but also that you can like detect defects earlier and say how you're actually designing a system you know, before it gets to the very end.
Speaker 4:Yeah, love it. There's so much. Thank you so much for that perspective and for the audience. I learned a lot of my systems engineering, initially from Matt, so kind of full circle here a little bit. Yeah, great points, and I'm very much following the model-based system engineering stuff that's coming across. It's very interesting. And just your brief thoughts. And then let's get into the media episode, but kind of how that interacts with digital twins, which we've talked about previously on this podcast as well, and I know you personally have done a lot with.
Speaker 2:Yeah, sure, so that really kind of goes to those different types of models that we use in model-based systems engineering. Right Like being able to kind of model the plant or the system and then start to build in the different types of really kind of scenarios, but they may look like behavioral models or agent models and how they can start to more similarly replicate. You know what the deployed system might look like.
Speaker 2:And then even in certain, in some cases, that's that allows us or actually I'll say, in a lot of cases it allows us to do the kind of the verification and validation that also comes straight out of systems engineering, in order to build those kind of accurate predictions for the intended consequences of the system you're designing.
Speaker 1:Yeah, it's really interesting and thank you for allowing us this segue to talk a little bit about systems engineering and lean on some of the things that we discussed in that podcast, because I know as we go through our topic today on data lineage, your perspective in that space might creep in here as well, and we're hoping when you lean back on it and we get further into this, this will resonate with a lot of our listeners as we talk about data lineage as we talk about data lineage.
Speaker 3:Yeah, I think you know. Let's start off with basically give me like a high level description of what is data lineage and then maybe give us a small taste of what that might look like.
Speaker 2:Sure. So if I kind of segue from systems, engineering.
Speaker 3:You know one of the tenets in systems engineering where? You really started to think about the engineering life cycle you know, in data lineage one of the things I really start to consider is okay, what is the data?
Speaker 2:life cycle Right and so from there there are a lot of questions right About. You know who provided data. Who has rights to access that data?
Speaker 3:Who and how should you?
Speaker 2:verify the data, and then what is? Really the source of that verifier even, and can that be something that is controlled or be handed off to basically a computer that doesn't need to know that source independently?
Speaker 2:And so what that really drives us towards is really a new type of identifier for data that allows that identifier to be portable, so you don't have to recreate a new ID everywhere you go. It allows it to be interoperable, so you're able to go, bring this to other applications, and then you do want it to be provable, as I mentioned, like with verification, and then you know. Then future looking or even present looking could be okay. Could that be decentralized, so that it's not only relied on some?
Speaker 3:system where, if that?
Speaker 2:system goes down. That ID now is no longer active, so we're, you know, accessible. So those are kind of the the ideas about how data lineage and like identifiers need to need to exist need to exist.
Speaker 4:How would you compare contrast, because I've oftentimes heard lineage and provenance used interchangeably and kind of where do you see the similarities and or differences with those?
Speaker 2:Yeah, that's a good question, andrew. So I think you know provenance feels to me like it has a connotation that is a lot more about the verification of the data, not only just where did this data come from, but really about you know the actors along the way that really kind of like, say, attributed to the data, whereas lineage also, I think also includes more besides just provenance, and that includes, includes all the methods that can go into doing the verification, doing the validation, controlling the access. So it just encompasses more around the provenance of the data itself.
Speaker 3:And in this world of responsible AI, we often talk a lot about, you know, managing data lineage, managing provenance, knowing where your data comes from. But why should we really dig in and care about data lineage? Like what does it allow us to do what is like impossible without it? What is this providing for us beyond a checkmark?
Speaker 2:You know, so obviously in the world of AI, we start to care. Maybe this is like one of the later things that we care about, but we certainly care about data quality, right. So we care about if this data not only is it verifiable and correct, but is this data actually useful? Because training costs money, right, and so if you're training on poor quality, data even if it was verifiable oh, this data is correct but it actually didn't get me the result I was looking for in a model, then that's a poor result, right?
Speaker 2:Separate from that is the integrity, the integrity of the data, whether it was actually true or not, and whether it is able to be used in a model which actually brings us to you know the larger reasons which are really around, like governance and compliance.
Speaker 2:So you know governance thinking about more about how organizations are allowed to share data, what permissions and really what legal agreements they have in place around what they're able to share and like whether it's just raw data, pii, or you know insights about data data and then on the, you know the the other side, which I know you guys know quite a bit about on compliance and auditing and being able to actually have logs and records about how data was accessed and how, when it was onboarded, and who who got it and who did what with that data.
Speaker 3:We understand that data lineage also enables us to verify the credentials, basically, of the data holders, and so I guess we're curious to hear a little bit about what types of methods are used to implement this. There's probably some cartography involved. If you're comfortable, we could use some technical detail. Just understanding what are some of the techniques being used under the box.
Speaker 2:Yeah, so in this kind of changing world for regulations, when we're talking about compliance, you know there's. We all know about the EUAI.
Speaker 3:Act.
Speaker 2:We know that there's GDPR CCPA, gdpr CCPA and then as we move kind of outside of say sharing PII and into say how different media is financial data. Then we've got CPFB. What this kind of all is screaming for is a need for not only this record, but this record for data to be tamper-proof and verifiable. Yeah, that's really interesting. Can you tell us more about what this verification looks like and what are some of the techniques that are?
Speaker 3:going into that.
Speaker 2:Yeah, so I'll talk about um, verifiable credentials and kind of taking two steps back on, kind of where that comes from is so there's something called the w3c, the worldwide web consortium, and they're a long-standing body from tim berners-lee know so, going back to 1994, of really the standards and regulations body for the internet, and so one of the recommendations that they put out an architecture for was around what are so what I've kind of pieced together as DIDs, the Essentials Identifiers, verifiable Credentials and Verifiable Presentations.
Speaker 2:So what the Verifiable Credential is is really a JSON document of your data, that work, data that has been chosen to be provided, and what it contains in that document is it contains the permissions around where it can contain the permissions around, the data that was shared, and then it also contains effectively, you can think- of it as metadata around that data that is, showing the proof of who provided that data via this decentralized identifier, and data integrity proof to show that this data, as it sits now, had to be what was untampered with in order to generate the proof for the hash of the proof that exists on that JSON document.
Speaker 3:Great and it's interesting to hear that, like, basically, this is very similar to like checksum checking, hash checking, right. So it has a lot of the flavor of how we do like document signing effectively, but for data now. So, if we use this type of technique, what does this let us do? What does this enable? Yeah, so right away.
Speaker 2:I mean the first thing that it does is like number one. You know you can tamper with it. If you made a change it would be shown up right away.
Speaker 2:Someone say to come in with a right to access that data, or and actually only some of that data, and allows them to actually get what's called a verifiable presentation, which is, you know, effectively a subset or a derivative of the data in that credential. So that can be say, you could have your data in this credential and only some of that, only what I'm allowed to see is given to me in a separate document which also contains all the proofs that show that lineage, all the way back to not only who provided that data as a decentralized identifier, but also say who provided that data as a decentralized identifier, but also say like who authorized that.
Speaker 3:And that can also contain say not the data itself, but say a computation of that data.
Speaker 2:So, for instance, would be that you say have uploaded your driver's license to yourself and you have a credential to yourself and I'm allowed to only ask for your age. Or actually even better would be to say, just tell me whether you're over 21 or not and me not knowing how to do me not having to know any other information, so what?
Speaker 2:this enables is us to use zero knowledge proofs and which is really kind of what I just described without having to know anything more about that information and so like. So we can kind of thank all the advances in not only just compute and storage but also in cryptography that are allowing us to be able to to have like really kind of a new way to not only secure data but also like access data, and so you know some of the techniques that can involve allowing for, say, proofs of inclusion, which means like, oh, like.
Speaker 2:I know. I know I had my credential, I know what my DID is, and so I can know that if you went and access the data, I can know that it was there as part of that record.
Speaker 2:Or, on the flip side, we can have a proof of exclusion and we could say like, hey, I think my data got breached and you know, I can bring it and find out that it was not included in this data set. So it allows for, you know, everything that we see on a new data breach. Every, you know, every couple of days we can see that there is like a new way to kind of also really kind of attack that, or at least be aware, right, like you never actually know. Now you say, oh, there's a data breach and now you're just like crossing your fingers and hoping that you weren't involved in it, right yeah.
Speaker 1:And I know we're going to discuss a little bit more about responsibility and responsibly, and especially in terms of AI, later on, but are there any other benefits that maybe aren't AI?
Speaker 2:Oh, yes, you know, we haven't talked much about well as I mentioned a decentralized identifier and so like these can also kind of the records and what happens to your data can also be, say put onto a decentralized or distributed ledger, you know, so it could be a blockchain, or it could be some other ledger, and so what that can allow for is, you know, economic participation, say, in the sharing of your data, that where you are actually able to have control right and and be willing to be willing to share and be willing to get paid if you choose to share your data and so that can improve really you know, like, what are the effects of that?
Speaker 2:Like, well, that can improve, you know, not only incentives for people, but it also can improve the quality of data that's being shared.
Speaker 3:You're describing a really nice and broad range of, basically, capabilities that this gives us right Things that are part of this data lineage, part of ai modeling. Is there anything else that really stands out to you? Or like these techniques are used, or like where, where else we might get value from these? Uh, let's say in like even on the training side. You know, one of the things that we think a lot about is value attribution.
Speaker 2:So really, how much that data contributes to say whether it's training a model and how you know how different actors or you know data sets that were provided in doing so you know how well did they help. Help to say, either just make that outcome, you know it can, it doesn't have to be specific predictions, but it can be actually just building the model and and training and getting the weights for your parameters.
Speaker 3:Yeah, I think that's that's really great and exciting. I think that that basically enables us to do a lot of things, and especially in this age where you know we have a lot of data out there that's getting used to train these models and we don't know if it's ours. We don't know if it's our images, our text, our video. This type of cryptographic data lineage would allow us to actually source back and understand where this data is coming from and where it's going to.
Speaker 2:Yeah, precisely.
Speaker 1:We've been strictly sticking to data lineage and, from a data perspective and really the foundations of that, we are going to switch gears in a little bit to talk a little bit more about this data lineage in the context of building robust and responsible AI systems Before we make that switch. Is there anything else you want to share?
Speaker 2:systems before we leave that, before we make that switch. Is there anything else you want to share? Uh, yeah, so just really kind of about, um, how this can participate in one of the one of the other aspects and kind of the changing landscape is around third-party cookies and how they're going away, and advertising and so-.
Speaker 1:I was gonna say this is my baby. Third-party cookies, marketing and advertising.
Speaker 2:Yeah, so every kind of ad tech company you'll see is discussing first-party data and how can, how they can, you know, build a build on a campaign on the cookie list world, and so this is also an application where kind of where these verified credentials and having this record for data becomes, you know, really important in terms of both having all the other things that I mentioned. The rights to the data and the value to the data and the attribution are all kind of, you know, at the forefront in the changing in the updates to ad tech.
Speaker 1:I'm interested to hear that. You hear that from both a professional's perspective and in terms of data and AI and where it's going, because we've heard that, as a marketer, we've heard this is coming for years and almost jaded because they pushed the deadline, but it is interesting to see how people are. What's going to evolve, like how are we going to be able to reach audiences? And that's the perfect setup to move into modeling and ai. As far as the ai, goes.
Speaker 2:I think one of the the things that I think about is that, um, you know, kind of every couple months, but I think the original paper goes back about two years where, but I mentioned that AI is running out of training data and so you know there's different forecasts of like, okay, for different types of generative AI models. You know there's maybe two years left of humanated training data for AI.
Speaker 2:And so this kind of goes back to the point about how valuable actually human-generated data is, and so when we talk about responsible AI, then when this first started coming out and really mentioning like the EU.
Speaker 3:AI.
Speaker 2:Act. Back then it was like okay, we need to actually have these extra checks in place to say like, okay, how did you actually get your data from right?
Speaker 2:And so then we started looking at or kind of like people started looking at RAG systems, the retrieval augmented generation, where it does an extra check basically on a source of its data or, you know, or a refresh in some cases, depending on how it's implemented, and so you know, this is where those systems now, if they're doing that extra check on a source, this is where something like verified credentials and data lineage feeds right into that type of system and so where you can have this double check or this refresh on how it was used in training, definitely, and that's where I think there's a huge need for that and any type of well-governed modeling system having that ability to see the lineage and have that flow through of knowing where your data came from.
Speaker 4:How do you know its quality. All of the things are super crucial to everything we talk about here on of models. So it's interesting to see. And if you haven't had a chance to read the EU AI Act I'm goofy like that where I enjoyed reading the 450-page document, but I'm not normal If you just go look at the highlights, there's a lot of great summaries out there. Ey has a good one, things like that. And if you look at the generative AI writers that they added recently, right before ratification that regenerative AI writers that they added recently, right before ratification that would be a good area to see, like how valuable this type of work work can be.
Speaker 4:You gave me some homework, andrew, I didn't realize I didn't.
Speaker 2:I did not read the full act. I read the few pages that kind of came out that were kind of like almost the fait accompli about what, what was going to be included in the in the act?
Speaker 4:So there's a lot of boilerplate. You can go to very specific things like some of the articles are good, the very specific articles in the middle, and then there's like a generative AI, almost appendix, that says a lot of this stuff, which I mean for your expertise and interest. I think you'd really enjoy, and probably be as I do with some of the other sections. Yes, when you see some of the things that they have in there specifically around, it's like a softball pitch to everything you're talking about right now.
Speaker 3:Well, I think this is a great discussion about data lineage and how it's really going to fit into this responsible AI framework. I think we talk a lot about data lineage, but I think this really highlights a lot of the ways in which it allows us to understand our models and how they're built, what data goes into them, and potentially even like how it made a decision in that case of the RAG, yeah, so I'd love to ask if you have any, you know, closing thoughts or anything you want to say, or if you'd like to take one thing away from this. I guess, what would you want to highlight for them?
Speaker 2:Yeah, sure. So I mean kind of what I think about what we've been working on with data lineage. Is that really kind of kind? Of resetting a foundation that allows us to build for the future.
Speaker 2:So, like you know, there's a there's a future that that involves fully homomorphic encryption and multi-party computation, where you know computers or you know really kind of cloud devices can compute on data without ever, actually ever having to see the data or know anything about it, and you know. So what we really needed was this kind of new kind of building block. One of the things that we've probably one of the phrases that we've all probably heard is data is the new oil right and really what happens?
Speaker 2:oil, not oil is the same, and it also needs a lot of refinement before it actually gets to anything that's useful. And so, really, what we're talking about here with data? Lineage is okay. How do we take? Raw data and refine it in a way that is now able to be used in this new future world of training models, generative AI models and, you know, decentralized ledgers.
Speaker 1:Matt, first of all, thank you for being with us and for those remarks. Matt, first of all, thank you for being with us and for those remarks. We really appreciate your thoughts on data lineage in the world of responsible AI and, really you know, taking us back to the basics on data lineage with your expertise. We're looking forward to seeing more of what you do and might have you back on a future podcast to answer some more questions. What do you think?
Speaker 2:This is so much fun. I'm so glad to be a part of it, and I've loved your previous episodes, so I'll be happy to come back For our listeners.
Speaker 1:Thanks for joining us today. To check out this podcast and all of our previous episodes, please visit our homepage at wwwmonotarai. Slash AI dash fundamentalists. Until next time.